Voice Identification Dataset
Home » Case Study » Voice Identification Dataset
Project Overview:
Objective
Voice Identification Dataset: We aim to build a large collection of voice recordings to enhance voice recognition systems. This collection will include many different voices from various people and situations, making the system smarter at recognizing voices.
Scope
The dataset encompasses a diverse set of voices, accents, languages, and environmental conditions to accurately represent real-life scenarios encountered by voice recognition systems.
Sources
- Real-world Voice Samples: Data collected from various sources include interviews, speeches, podcasts, and other spoken content available online. By capturing natural variations in speech patterns and accents, we gain a rich and diverse dataset.
- Studio Recordings: To obtain high-quality audio recordings, engineers work in controlled studio environments. This ensures clarity and consistency across different samples.
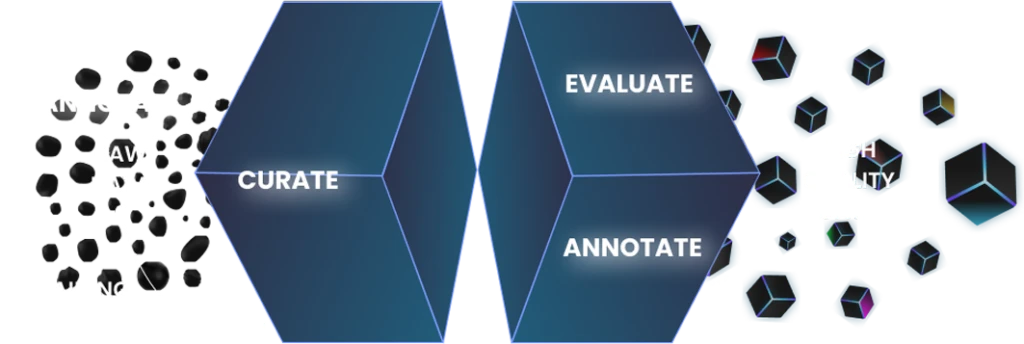
Data Collection Metrics
- Total Data Collected: 50,000 audio clips.
- Data Annotated for ML Training: 45,000 audio clips with detailed speaker labels and metadata for machine learning purposes.
Annotation Process
Stages
- Speaker Identification: Each audio clip is clearly labeled with the speaker’s identity, including their name, profession, and other relevant information.
- Furthermore, Speech Transcription: Transcriptions of spoken content are provided to facilitate text-based analysis and training of speech recognition models.
Annotation Metrics
- Annotation Quality: The quality of the annotations is exceptionally high. They are meticulously crafted, ensuring precise speaker identification and accurate transcription. Consequently, this enhances the dataset’s utility for various machine learning tasks.
Quality Assurance
Stages
Accuracy Testing: First and foremost, accuracy testing is conducted meticulously. We evaluate the accuracy and reliability of the dataset in identifying speakers across various conditions and contexts. This step ensures that our dataset performs well under different scenarios, providing trustworthy results.
Data Integrity Checks: Furthermore, we carry out data integrity checks on a regular basis. These checks are essential to maintain the integrity and consistency of the data. By minimizing errors and inconsistencies, we ensure that the dataset remains reliable and accurate.
Improvement Process: Lastly, we have an ongoing improvement process. We actively incorporate feedback from users and researchers into the dataset. This continuous enhancement process ensures that the dataset evolves in quality and usability, meeting the needs of its users effectively.
QA Metrics
- Speaker Identification Accuracy: The dataset achieves a speaker identification accuracy of 98%, which ensures reliable recognition of speakers across diverse conditions.
- Transcription Accuracy: Additionally, speech transcriptions maintain a high level of accuracy, boasting an average word error rate of less than 5%.
Conclusion
The development of the VoxCeleb dataset represents a significant advancement in voice identification technology. By providing a diverse and well-annotated collection of real-world voice samples, it serves as a valuable resource for training and testing voice recognition systems. Consequently, it ultimately contributes to the improvement of speech-based technologies in various applications.

Quality Data Creation

Guaranteed TAT

ISO 9001:2015, ISO/IEC 27001:2013 Certified

HIPAA Compliance

GDPR Compliance

Compliance and Security
Let's Discuss your Data collection Requirement With Us
To get a detailed estimation of requirements please reach us.