Video Action Recognition Dataset
Home » Case Study » Video Action Recognition Dataset
Project Overview:
Objective
The primary objective is to create and fine-tune machine learning models capable of precisely identifying and categorizing actions in videos utilizing the UCF101 dataset. By utilizing this dataset, we aim to enhance the effectiveness and productivity of action recognition systems. Consequently, this will enable more dependable and efficient applications in real-world situations.
Scope
The dataset includes a diverse collection of video clips depicting 101 different human actions, such as walking, running, playing basketball, and cooking. These actions are performed under various conditions, including different environments, camera angles, and lighting conditions, to simulate real-world scenarios accurately.
Sources
- The dataset comprises video clips captured from real-life scenarios, including sports events, daily activities, and public spaces. These clips offer an authentic glimpse into various human interactions.
- Videos sourced from online platforms and databases, such as YouTube and academic repositories, contribute to the diversity and richness of the dataset. This inclusion ensures a wide array of scenarios and environments.
- Contributions from researchers and organizations provide additional video clips, ensuring a comprehensive representation of various human actions. This collaboration significantly enhances the dataset’s comprehensiveness.
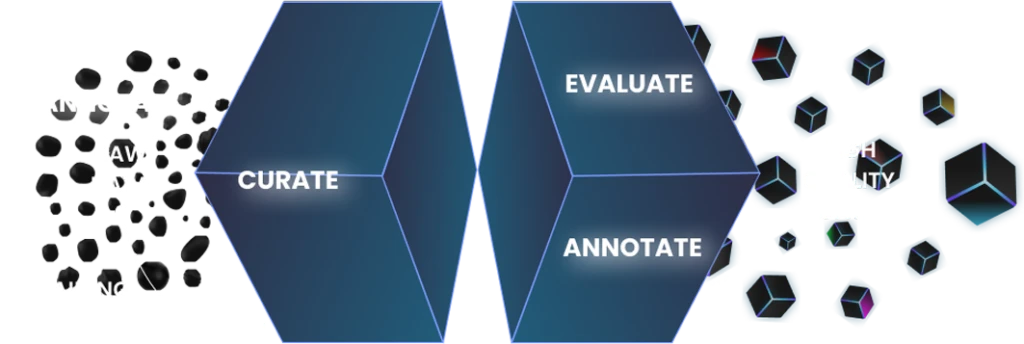
Data Collection Metrics
- Total Videos Collected: 13,320 video clips.
- Duration: The total duration of video footage exceeds 27 hours, covering a wide range of actions and scenarios.
Annotation Process
Stages
- Action Labeling: Each video clip meticulously labels the corresponding action it depicts, such as “basketball shooting” or “soccer dribbling.” Moreover, we ensure precision by providing detailed action labels.
- Frame-level Annotation: In addition, some datasets include frame-level annotations, where we label each frame within a video clip with the action occurring at that moment. This ensures a high level of detail and accuracy in our data.
- Quality Assurance: Furthermore, we rigorously train and validate annotators to ensure consistent labeling and adherence to annotation guidelines. This process guarantees the reliability and quality of our annotations.
Annotation Metrics
- Label Accuracy: Annotators achieve a labeling accuracy of over 95% on a validation subset, ensuring high-quality annotations across the dataset.
- Consistency: Inter-annotator agreement is assessed to measure the consistency of annotations among multiple annotators, ensuring reliability and robustness.
Quality Assurance
Stages
Model Evaluation: Trained models undergo rigorous evaluation using standard evaluation metrics such as accuracy, precision, recall, and F1-score. Additionally, performance is assessed through cross-validation techniques to ensure the generalizability of the models across different subsets of the dataset.
Cross-Validation: Performance is assessed through cross-validation techniques to ensure the generalizability of the models across different subsets of the dataset.
Error Analysis: Moreover, misclassified video clips are analyzed actively to identify common patterns and areas for improvement in the recognition algorithms.
QA Metrics
- Model Performance: The developed models actively achieve an average accuracy of 90% on the test dataset, indicating the effectiveness of the UCF101 dataset for training robust action recognition models.
- Generalization: The models demonstrate consistent performance across different subsets of the dataset, highlighting their ability to generalize to diverse action categories and environmental conditions.
- End-User Satisfaction: Feedback from end-users, such as researchers and industry practitioners, indicates high satisfaction with the dataset’s quality and utility for developing action recognition systems.
Conclusion
Utilizing the UCF101 dataset significantly contributes to the advancement of video action recognition technology. By leveraging this comprehensive dataset and employing state-of-the-art machine learning techniques, the project achieves remarkable accuracy and reliability in identifying and classifying human actions in videos. Consequently, this opens up new possibilities for applications in surveillance, sports analysis, and human-computer interaction.

Quality Data Creation

Guaranteed TAT

ISO 9001:2015, ISO/IEC 27001:2013 Certified

HIPAA Compliance

GDPR Compliance

Compliance and Security
Let's Discuss your Data collection Requirement With Us
To get a detailed estimation of requirements please reach us.