Handwriting Recognition for Form Processing
Home » Case Study » Handwriting Recognition for Form Processing
Project Overview:
Objective
Handwriting Recognition for Form Processing: Our primary goal was to transform the way industries like finance, healthcare, and education handle form data. By automating the extraction of handwritten information, we aimed not only to speed up data processing but also to minimize errors that are common in manual data entry.
Scope
It enables the conversion of analog handwriting into digital text, supporting industries such as finance, healthcare, education, and government in streamlining data entry, reducing errors, and enhancing data analytics capabilities.
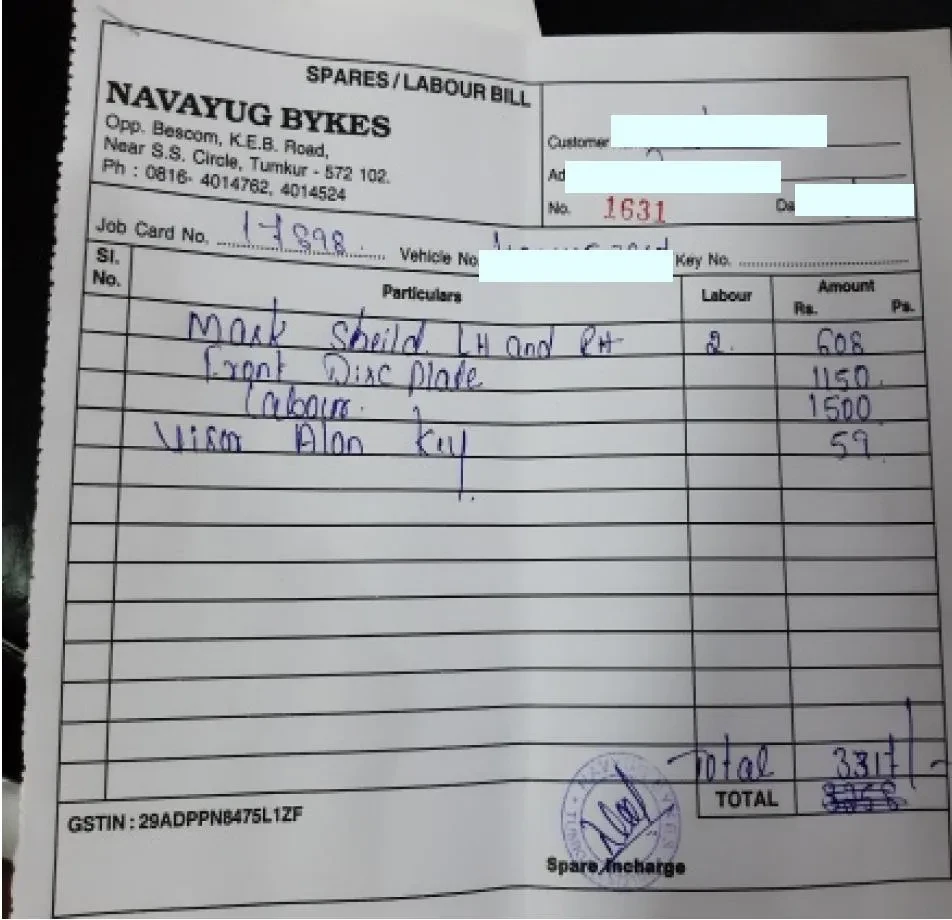
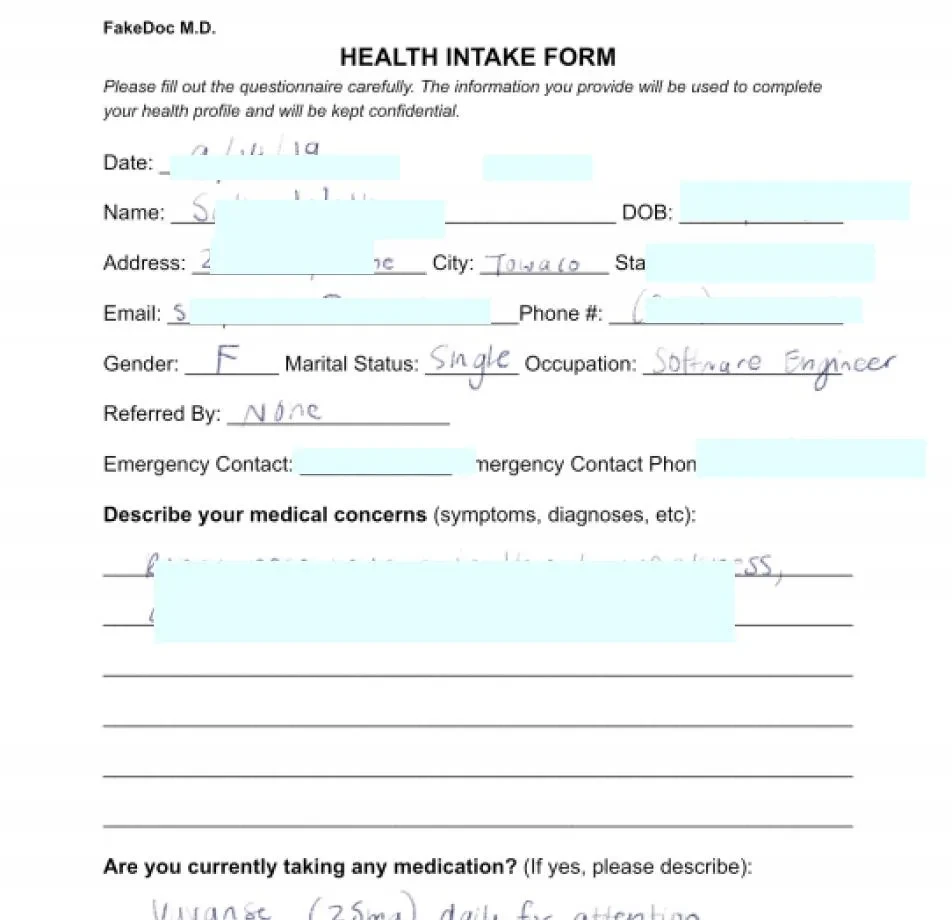
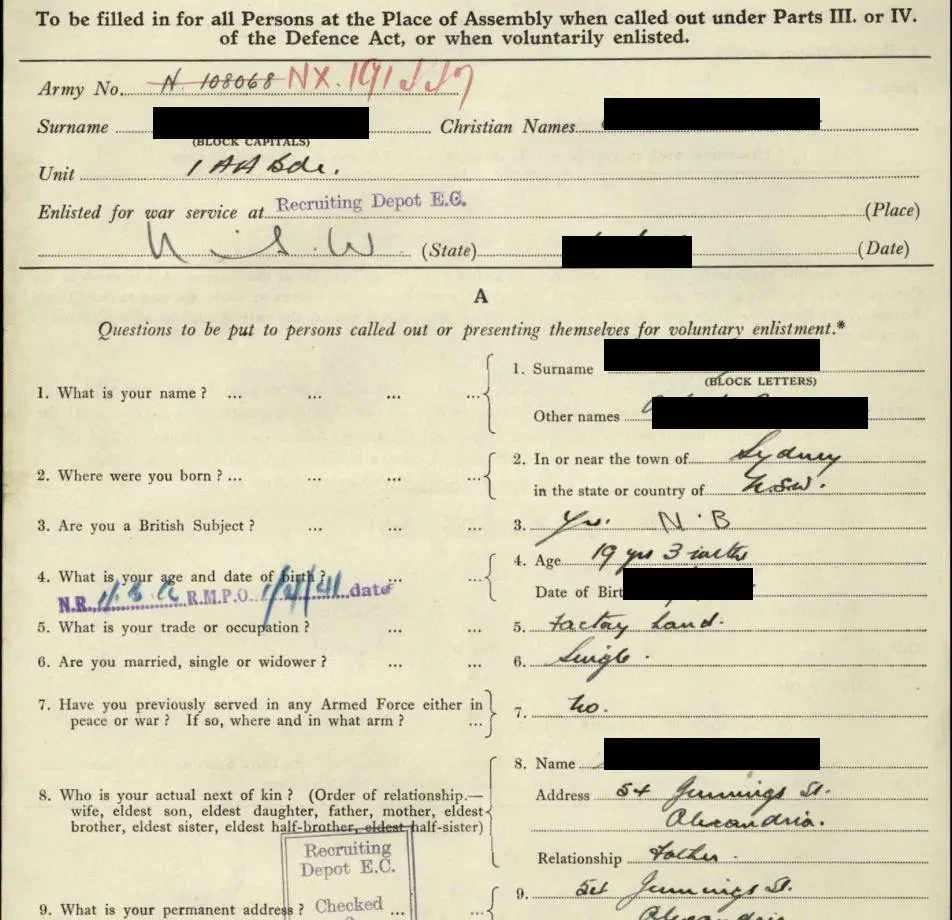
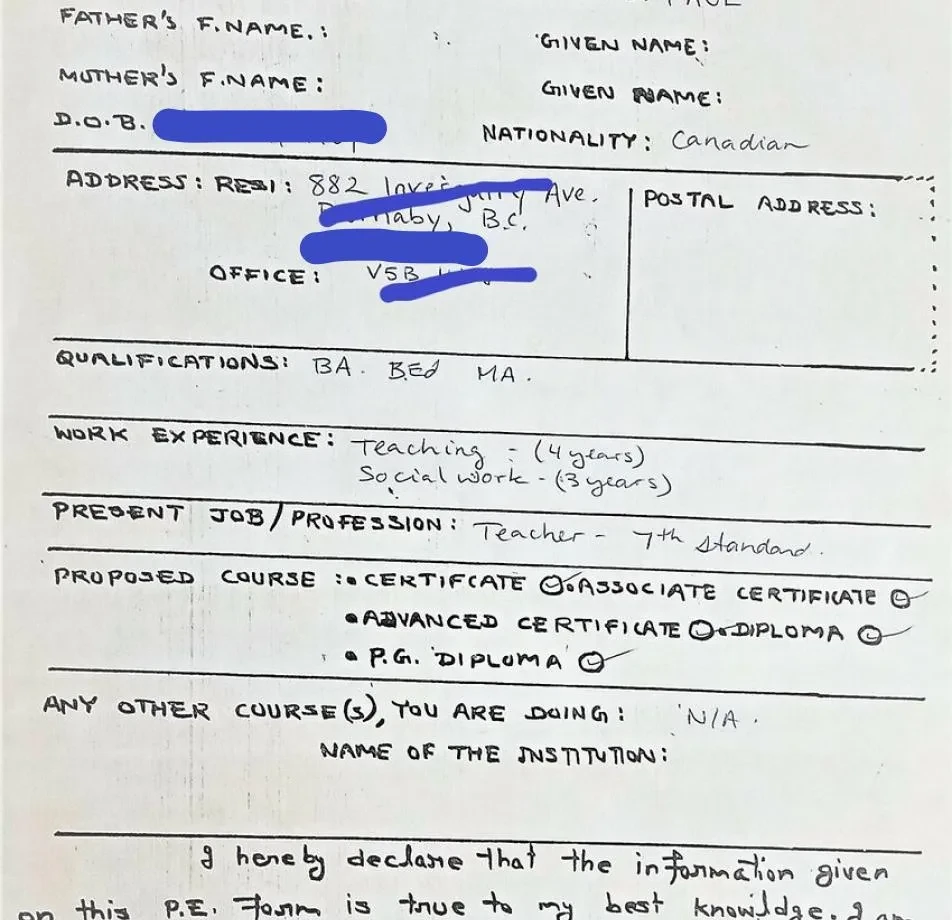
Sources
- Academic Research: OCR technology has significantly advanced, enabling machines to convert different types of documents, such as scanned paper documents, PDFs, or images captured by a digital camera, into editable and searchable data. Recent studies focus on enhancing the accuracy and efficiency of OCR systems.
- Industry Reports: Moreover, handwriting recognition, which is a subfield of OCR, has garnered considerable attention in recent years. Scholars are exploring various methodologies to enhance the accuracy of recognizing handwritten characters and converting them into digital text.
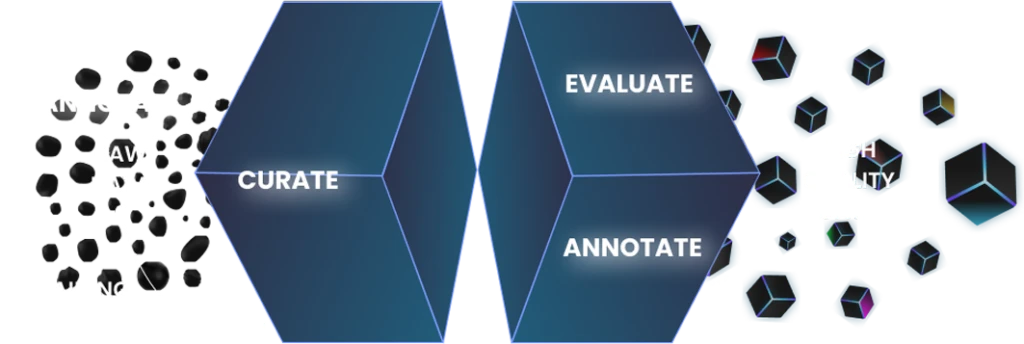
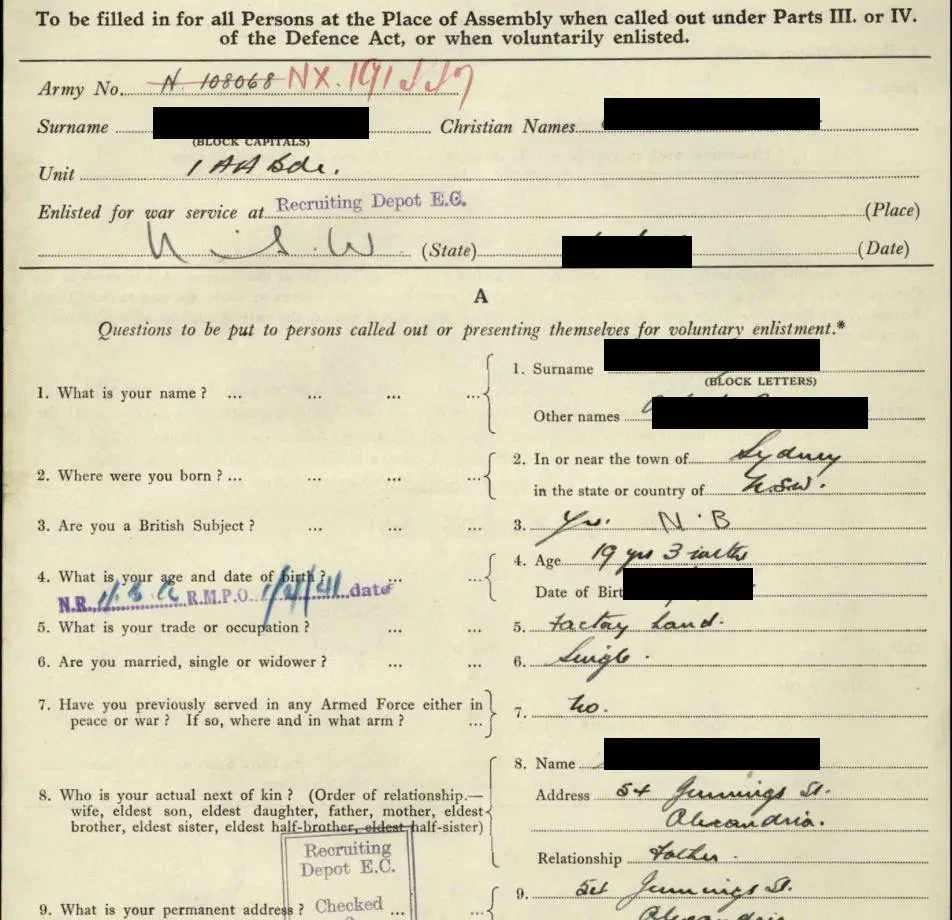
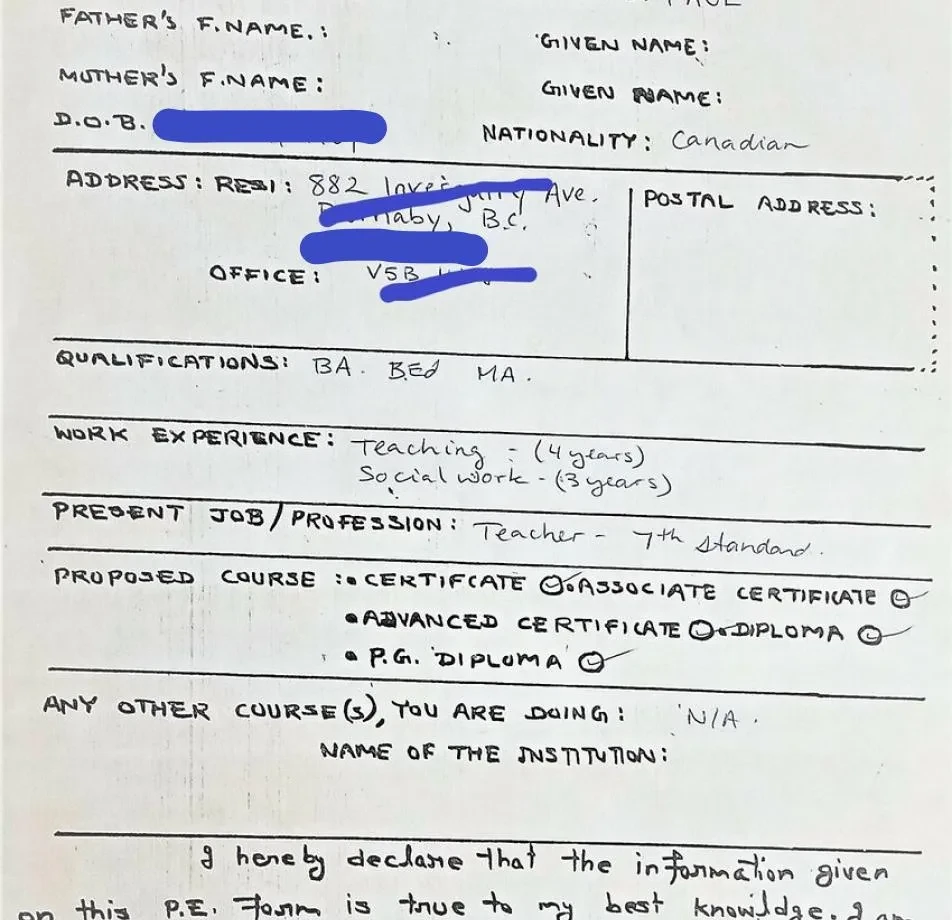
Data Collection Metrics
- Data Volume: Quantity of collected handwritten form data.
- Data Quality: Accuracy and completeness of the data.
Annotation Process
Stages
- Data Collection: Gathering handwritten forms is essential, especially when it includes various document types and handwriting styles. First and foremost, collecting diverse forms allows us to understand different handwriting patterns. Consequently, this aids in developing better recognition systems. Moreover, by including a variety of document types, we ensure comprehensive data coverage.
- Data Preprocessing: First, gather all the collected forms and ensure they are organized and free from damage. Next, proceed to clean the forms, removing any dirt or smudges that might interfere with the digitization process. Once the forms are ready, carefully scan each one using a high-resolution scanner. This step will convert the physical forms into digital images, preserving every detail accurately.
- Feature Extraction: Extract relevant features, such as character shapes and patterns, for recognition.
- Model Training: Utilize machine learning algorithms to train the handwriting recognition model.
- Recognition and Verification: Apply the trained model to recognize and verify the handwritten text.
- Data Integration: Integrate recognized data into digital systems or databases for further processing.
- Quality Assurance: Implement quality checks to ensure accurate recognition and resolve any discrepancies.
- Feedback Loop: Continuously update and refine the recognition model based on user feedback and evolving handwriting patterns.
- Scalability: Ensure the solution is scalable to handle large volumes of handwritten forms efficiently.
Annotation Metrics
- Inter-Annotator Agreement (IAA): To ensure consistent and reliable annotations, we measure the level of agreement among human annotators when labeling handwritten data through Inter-Annotator Agreement (IAA). Furthermore, we evaluate the precision of handwritten data annotations by assessing the percentage of correctly labeled characters or words within the dataset, thereby determining Annotation Accuracy.
- Annotation Accuracy: Evaluate the precision of handwritten data annotations by assessing the percentage of correctly labeled characters or words within the dataset.
- Annotation Efficiency: Additionally, we assess the speed and cost-effectiveness of the annotation process, ensuring that it is efficient and scalable for large handwritten form datasets, thus focusing on Annotation Efficiency.
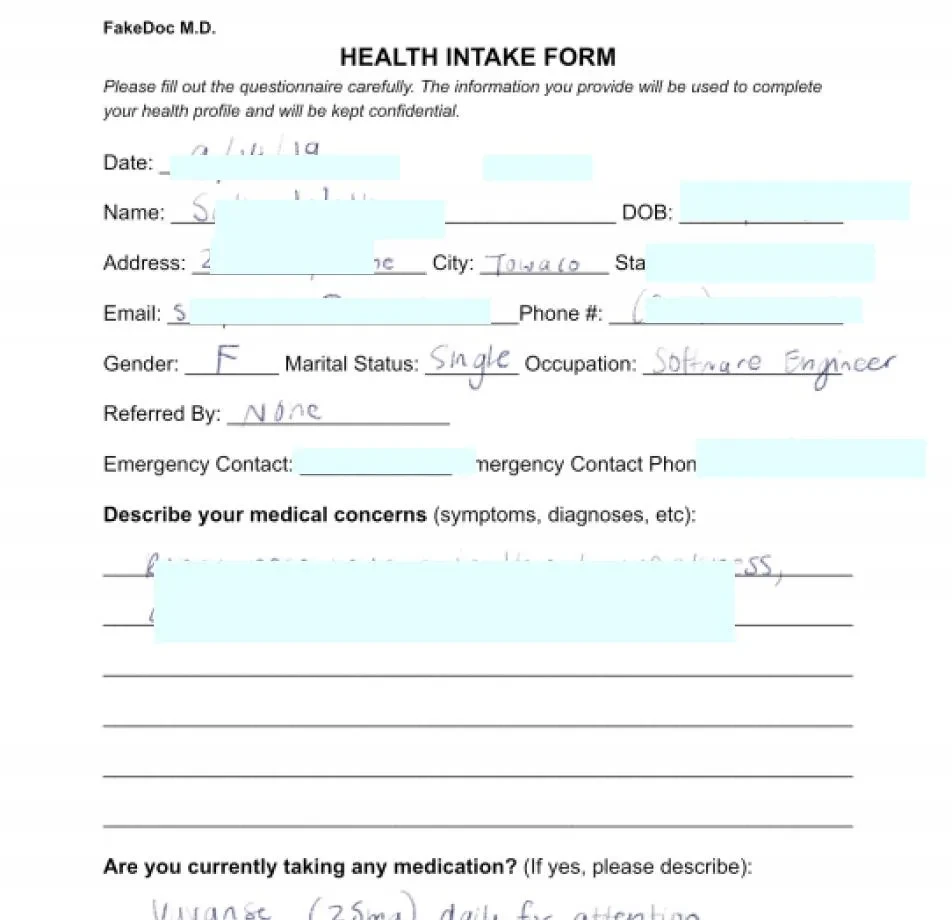
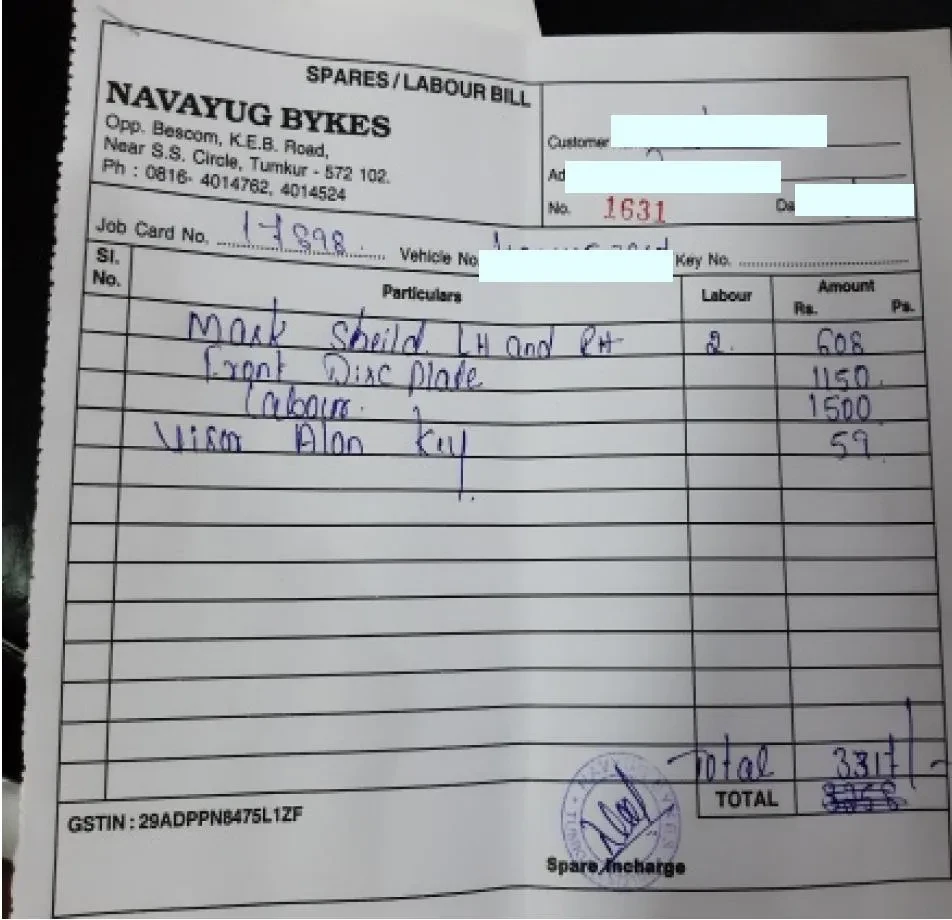
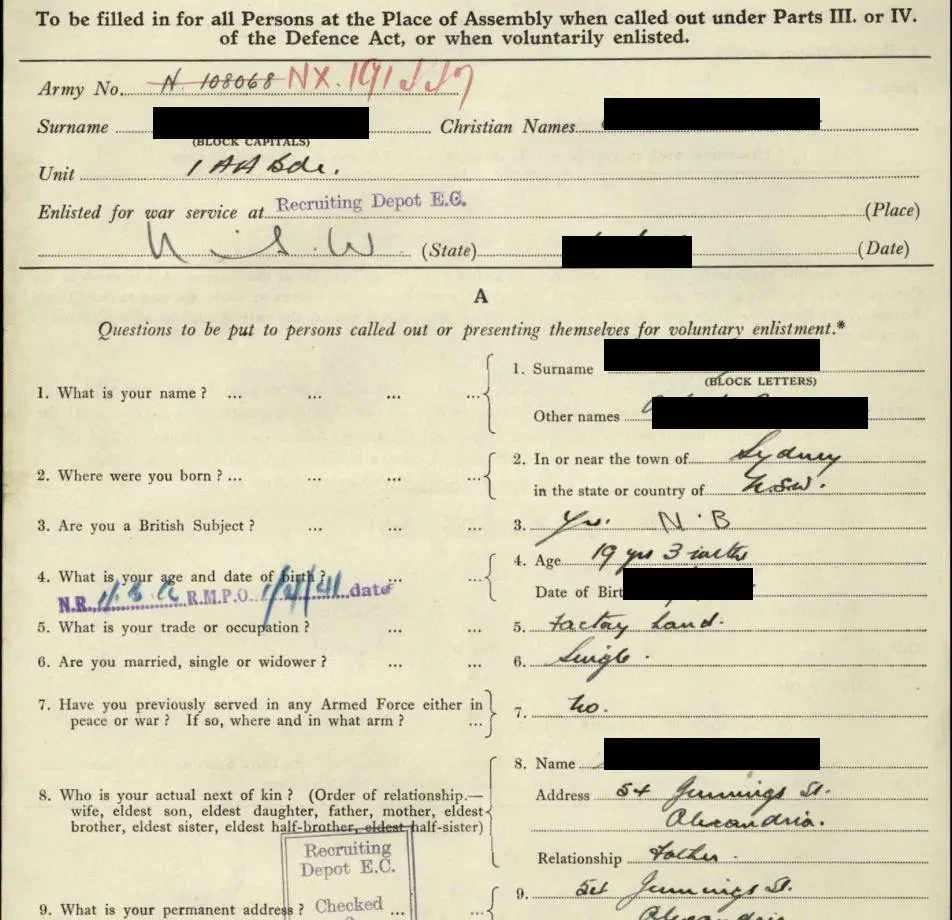
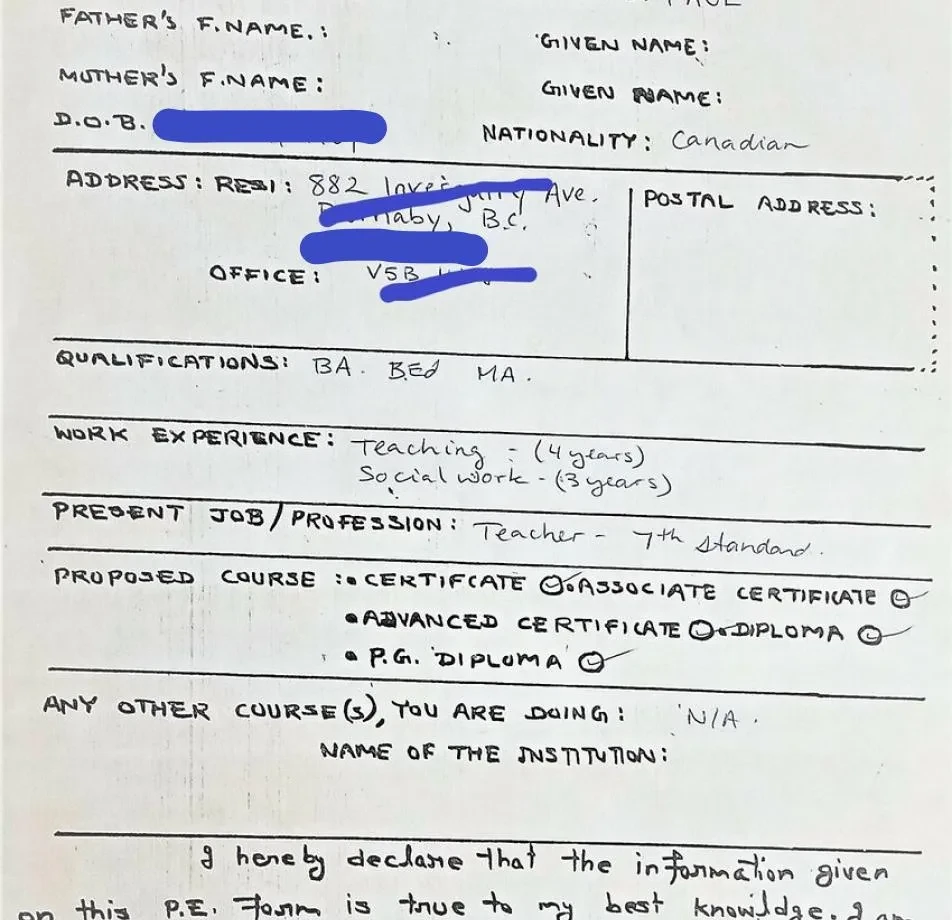
Quality Assurance
Stages
Data Privacy: Secure handwritten data and comply with privacy regulations.
Quality Control: Ensure accuracy and reliability of annotations and recognition.
Ethical Practices: Adhere to ethical guidelines in data handling and processing.
QA Metrics
- Recognition Accuracy: Measure text recognition precision.
- False Positive Rate: Evaluate false recognition instances.
Conclusion
Handwriting recognition for form processing is a transformative technology that streamlines data entry and document management. By automating the extraction of handwritten information from forms, it enhances efficiency, reduces manual errors, and accelerates data processing in various industries, from finance and healthcare to education and government.

Quality Data Creation

Guaranteed TAT

ISO 9001:2015, ISO/IEC 27001:2013 Certified

HIPAA Compliance

GDPR Compliance

Compliance and Security
Let's Discuss your Data collection Requirement With Us
To get a detailed estimation of requirements please reach us.