Image Segmentation for Medical Imaging
Home » Case Study » Image Segmentation for Medical Imaging
Project Overview:
Objective
Image segmentation in medical imaging is to precisely delineate and quantify anatomical or pathological regions within medical images, aiding in diagnosis, treatment planning, and workflow efficiency while advancing healthcare with advanced technology.
Scope
Image segmentation in medical imaging encompasses various applications. These include delineating anatomical structures and pathological regions in medical images. This technology benefits fields such as radiology, pathology, and surgery by automating tasks and improving accuracy. Moreover, ongoing advancements in technology continually expand its potential.
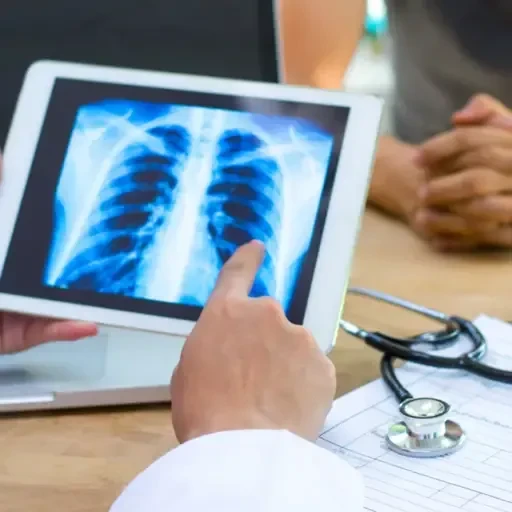
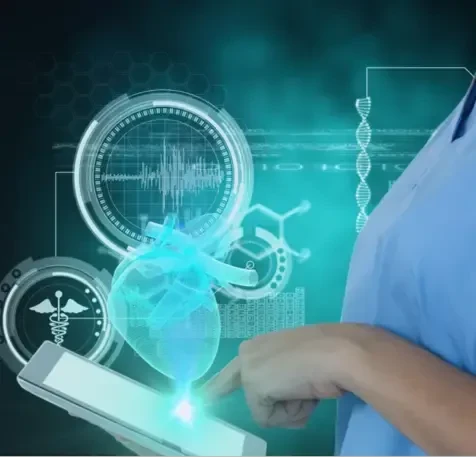
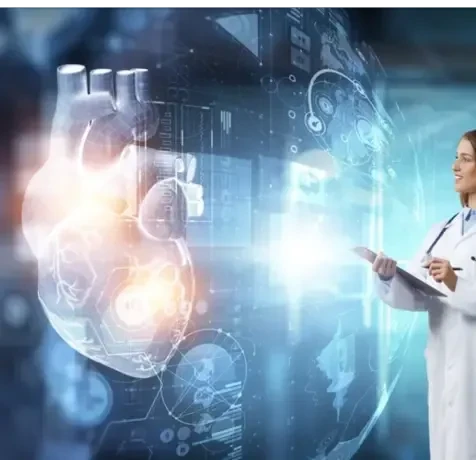
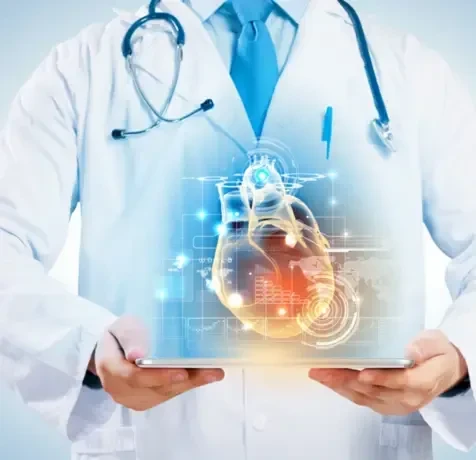
Sources
- Deep Learning: Deep learning techniques, notably convolutional neural networks, have revolutionized medical image segmentation.
- Clinical Impact: Consequently, image segmentation is widely applied in clinical contexts for tasks like tumor detection and precise diagnosis.
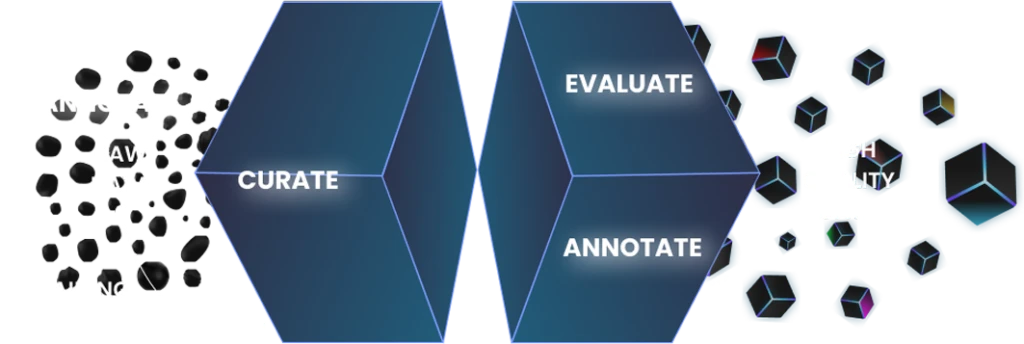
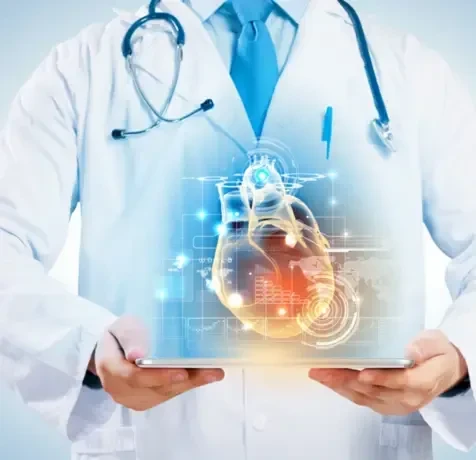
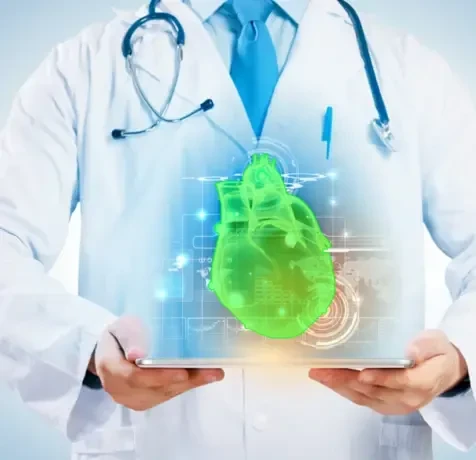
Data Collection Metrics
- Image Quantity: Total images collected.
- Annotation Quality: Accuracy and consistency of image annotations.
Annotation Process
Stages
- Preprocessing: Enhance image quality and reduce noise.
- Feature Extraction: Identify relevant image characteristics.
- Segmentation Algorithms: Divide the image into distinct regions.
- Post-processing: Refine segmented regions for accuracy.
- Validation and Evaluation: Measure segmentation quality against ground truth data.
Annotation Metrics
- Dice Coefficient: Measures the overlap between predicted and ground truth regions.
- Similarly,Jaccard Index: Quantifies the similarity between segmented and reference regions.
- Furthermore, Sensitivity and Specificity: Assess the classifier’s performance in detecting and excluding regions of interest.
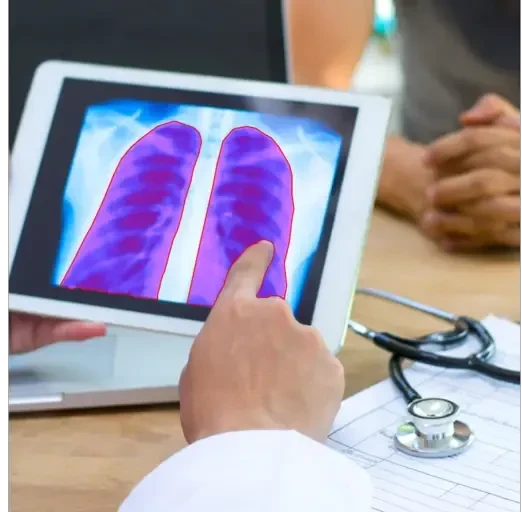
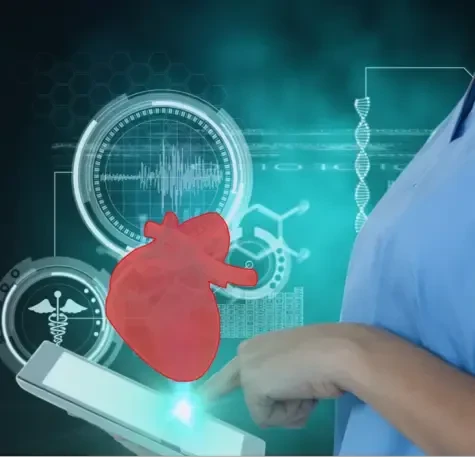
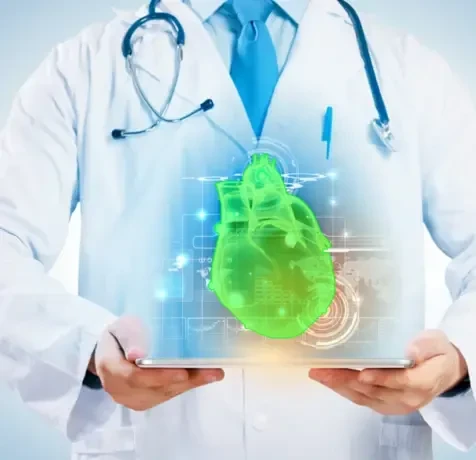
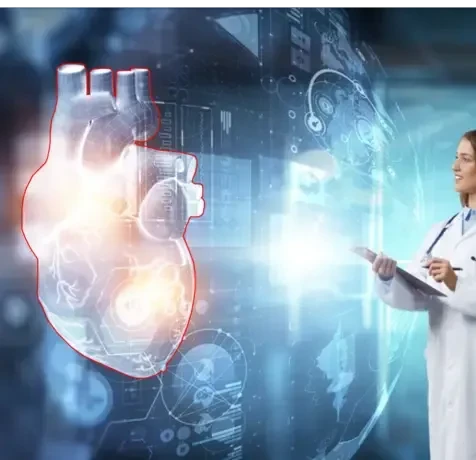
Quality Assurance
Stages
Transcription Verification: Transcription verification is vital in ensuring the accuracy of transcribed content by cross-referencing it with the original source. This process is crucial for precision and reliability, particularly in various fields.
Privacy Compliance: Similarly, privacy compliance involves adhering to laws and regulations to protect individuals’ personal data. By doing so, trust, legal adherence, and data security are maintained.
Data Security:Furthermore, data security plays a pivotal role in safeguarding digital and physical data from unauthorized access. It ensures confidentiality and integrity, which are critical for information safety.
QA Metrics
- Defect Rate: Measures the number of defects or errors in a product or process, indicating its quality.
- Customer Satisfaction: Reflects how well a product or service meets customer expectations, a critical quality metric.
Conclusion
Image segmentation in medical imaging stands at the forefront of technological advancements, holding immense clinical significance. Despite challenges related to data quality and algorithm complexity, the integration of deep learning techniques, especially convolutional neural networks, has significantly improved the accuracy and efficiency of segmentation tasks. This automation not only enhances the precision of diagnoses and treatment planning but also streamlines healthcare workflows, allowing medical professionals to focus on critical decision-making processes.

Quality Data Creation

Guaranteed TAT

ISO 9001:2015, ISO/IEC 27001:2013 Certified

HIPAA Compliance

GDPR Compliance

Compliance and Security
Let's Discuss your Data collection Requirement With Us
To get a detailed estimation of requirements please reach us.