Traffic Sign Detection for Autonomous Vehicles
Home » Case Study » Traffic Sign Detection for Autonomous Vehicles
Project Overview:
Objective
As a leading data collection and annotation company, moreover, we have successfully executed a comprehensive project focused on traffic sign detection for autonomous vehicles. First and foremost, our primary objective was to meticulously collect and annotate a diverse array of road signs, thereby ensuring our data aids in the real-time, accurate interpretation of these signs.
Scope
Our project’s scope was extensive; consequently, it encompassed the identification and categorization of a wide range of traffic signs, from speed limits to warning indicators. We dedicated ourselves to providing an exhaustive dataset, essential for the nuanced needs of autonomous driving technology.
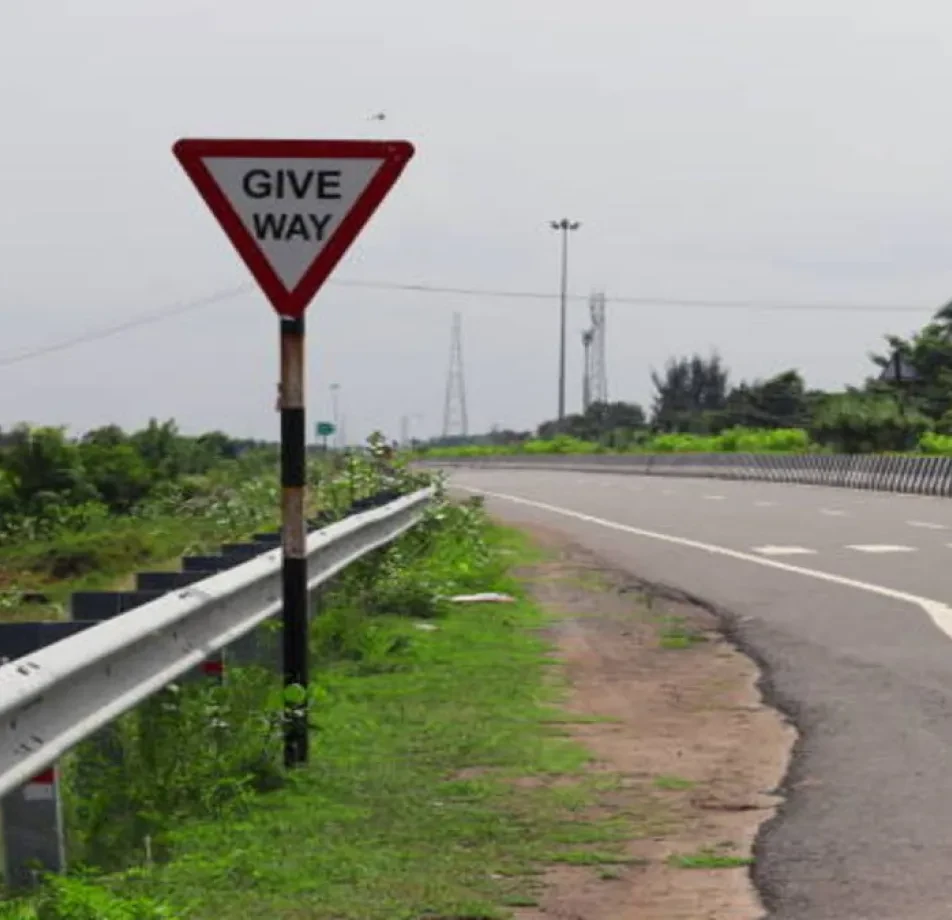
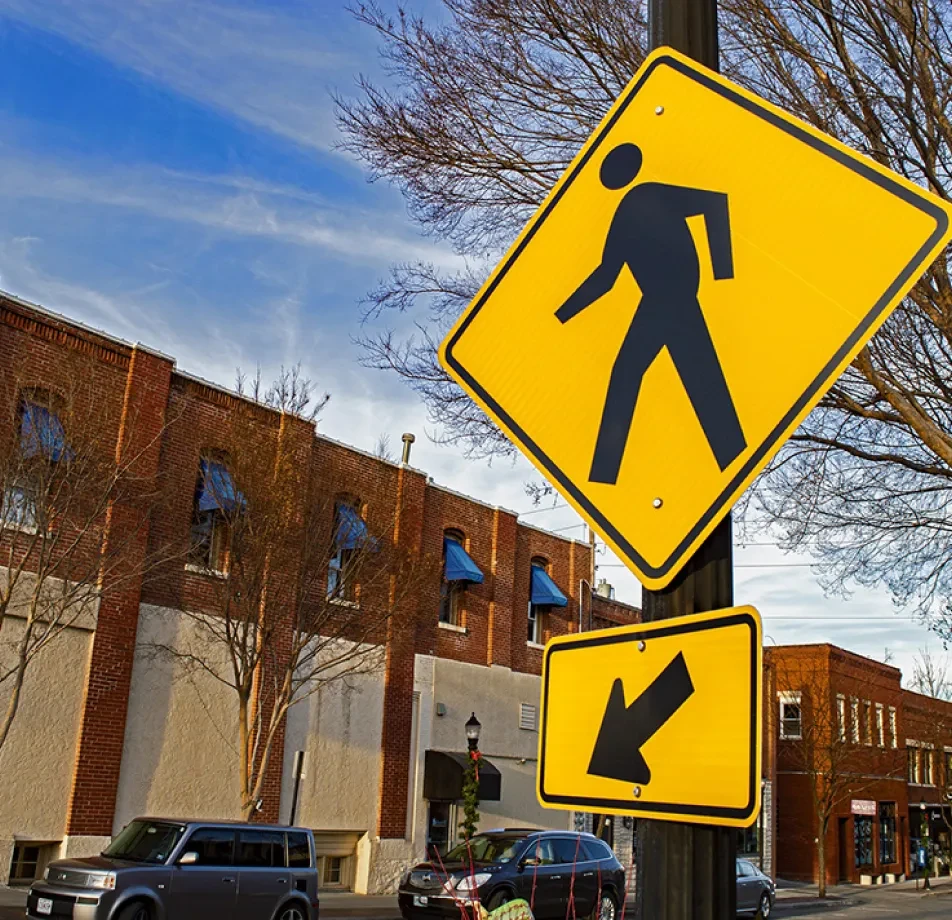
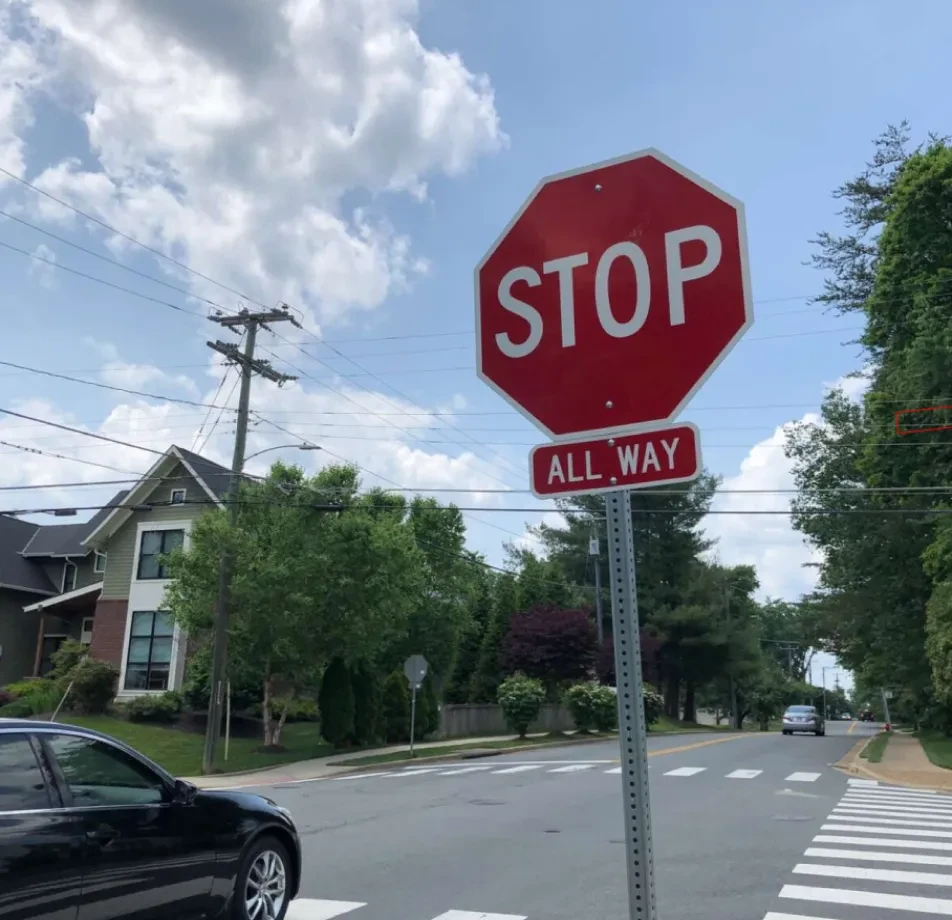
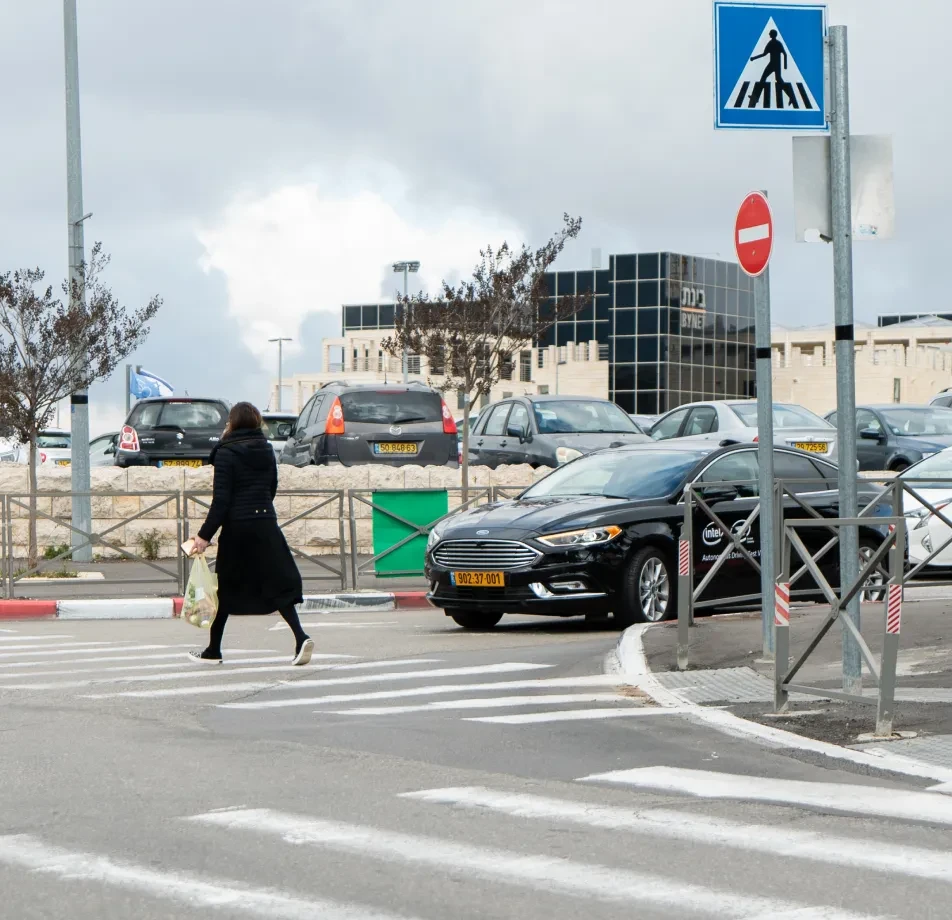
Sources
- Academic Research: Journals and conferences in computer vision and AI.
- Automotive Industry: Manufacturers and tech companies’ R&D efforts.
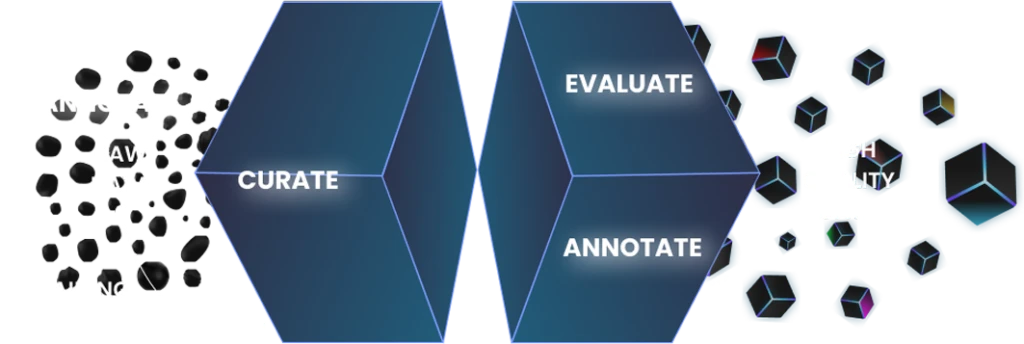
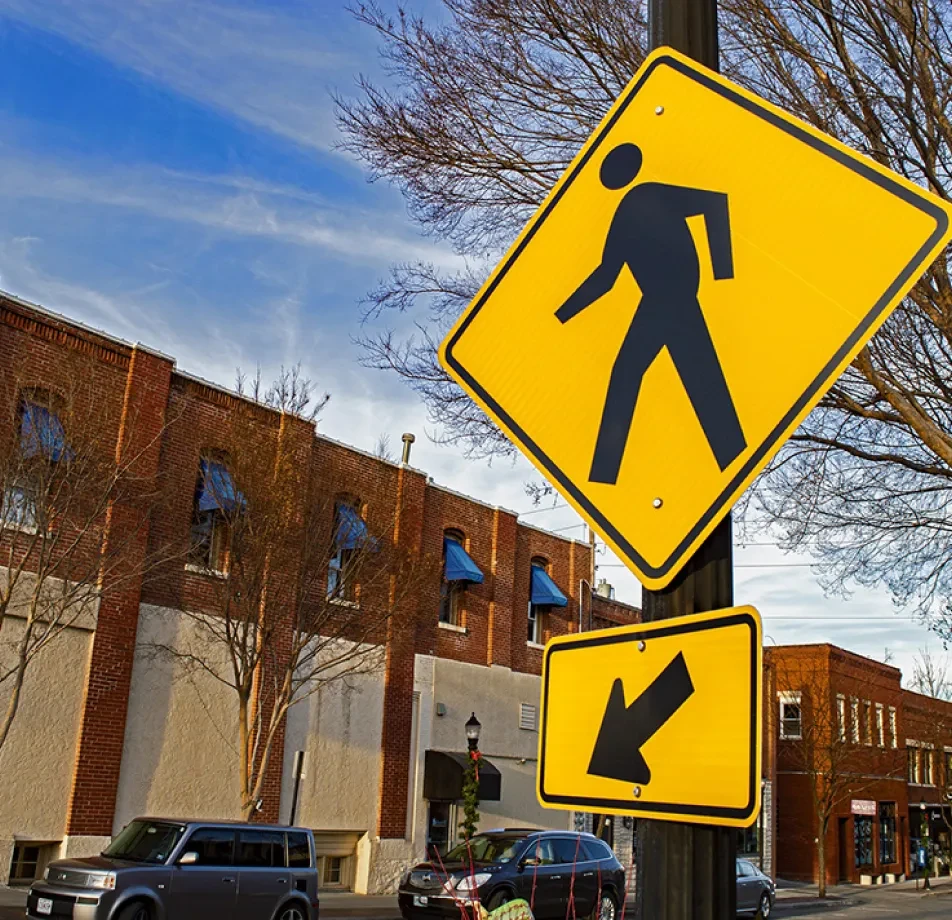
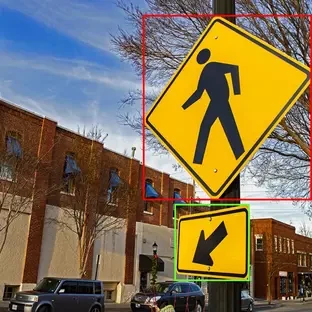
Data Collection Metrics
- Dataset Size: Amount of annotated data for training.
- Environmental Diversity: Range of conditions covered in the dataset.
Annotation Process
Stages
- Data Acquisition: Our team excelled in gathering images and videos of traffic signs from multiple, varied sources.
- Data Annotation: We meticulously labeled each piece of collected data, specifying the precise location and type of each traffic sign.
- Preprocessing: Our process included enhancing image quality through resizing, noise reduction, and color correction.
- Model Training: Utilizing advanced machine learning algorithms, we trained the detection model on our carefully annotated data.
- Real-time Detection: The trained model was implemented in autonomous vehicles for immediate traffic sign recognition.
- Integration: We ensured the seamless integration of detected signs with the vehicles’ navigation and control systems for optimal safety.
Annotation Metrics
- Annotation Consistency: Measuring the agreement level among multiple annotators when labeling the same set of traffic signs is crucial. This ensures uniformity in the annotations. Additionally, by adding transition words, such as ‘furthermore’ or ‘moreover’, it can help clarify relationships between ideas. For instance, ‘Furthermore, it assists in identifying discrepancies in annotations.’ This emphasizes the importance of consistency in the labeling process. Moreover, using transition words like ‘consequently’ or ‘therefore’ can highlight logical connections.
- Annotation Accuracy: When evaluating the precision and correctness of annotations, it’s crucial to consider several factors. Firstly, we need to assess whether the annotations accurately identify the sign’s type. Secondly, we must determine if they correctly pinpoint its location. Additionally, we need to evaluate whether the annotations accurately define the boundaries of the sign.
- Annotation Efficiency: Evaluating the precision and correctness of annotations, in terms of correctly identifying the sign’s type, location, and boundaries, requires meticulous attention to detail. Additionally, it demands a comprehensive understanding of the context and significance of each annotation. Moreover, it necessitates thorough documentation of any discrepancies or uncertainties encountered during the evaluation process.
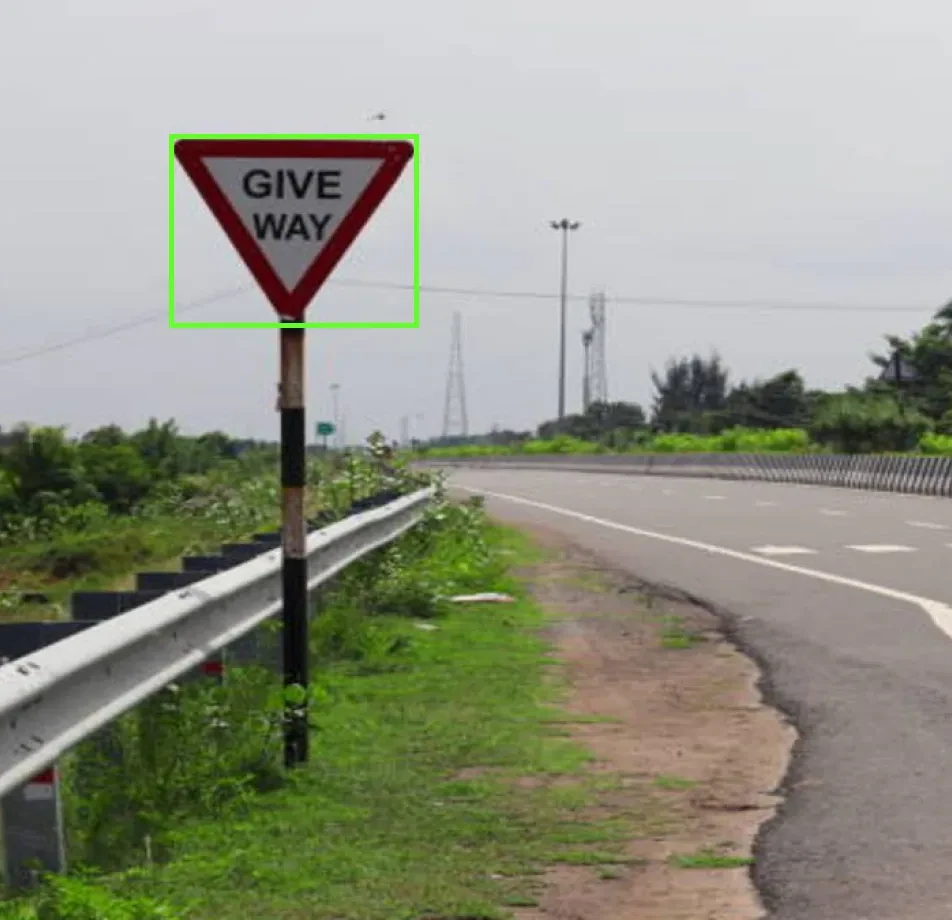
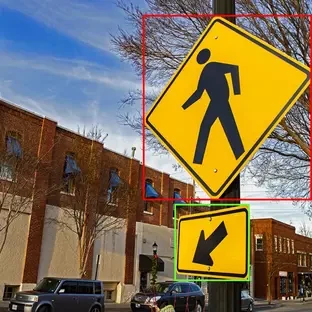
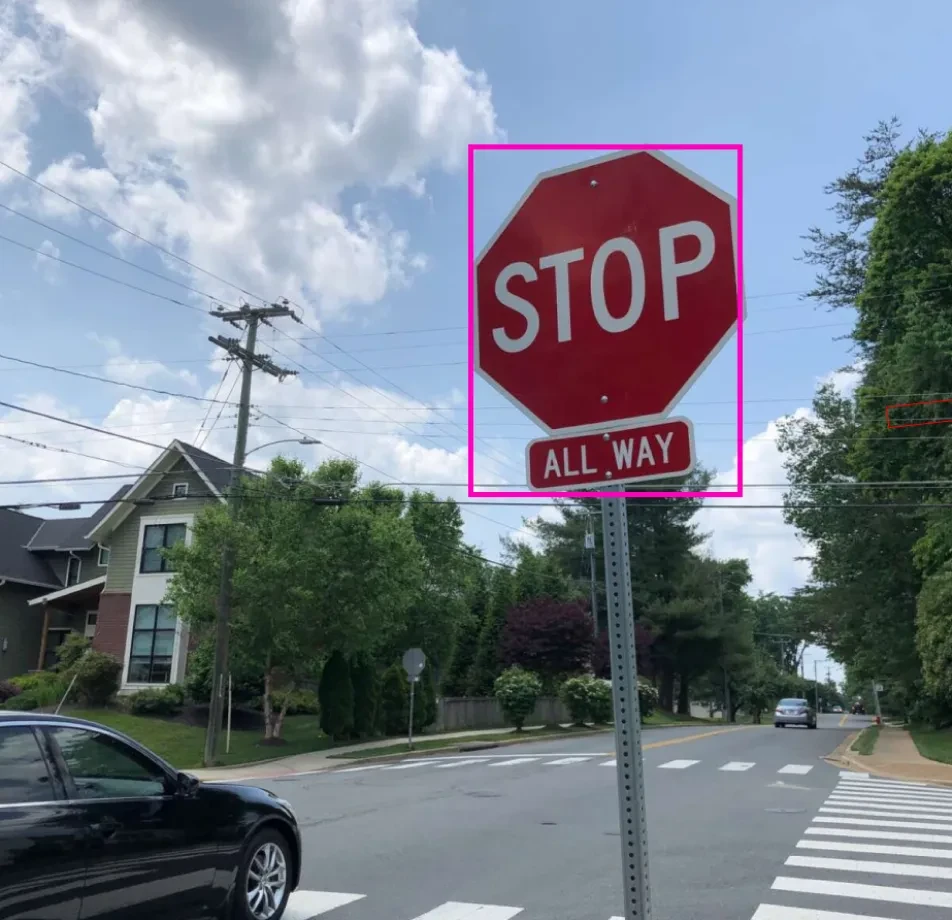
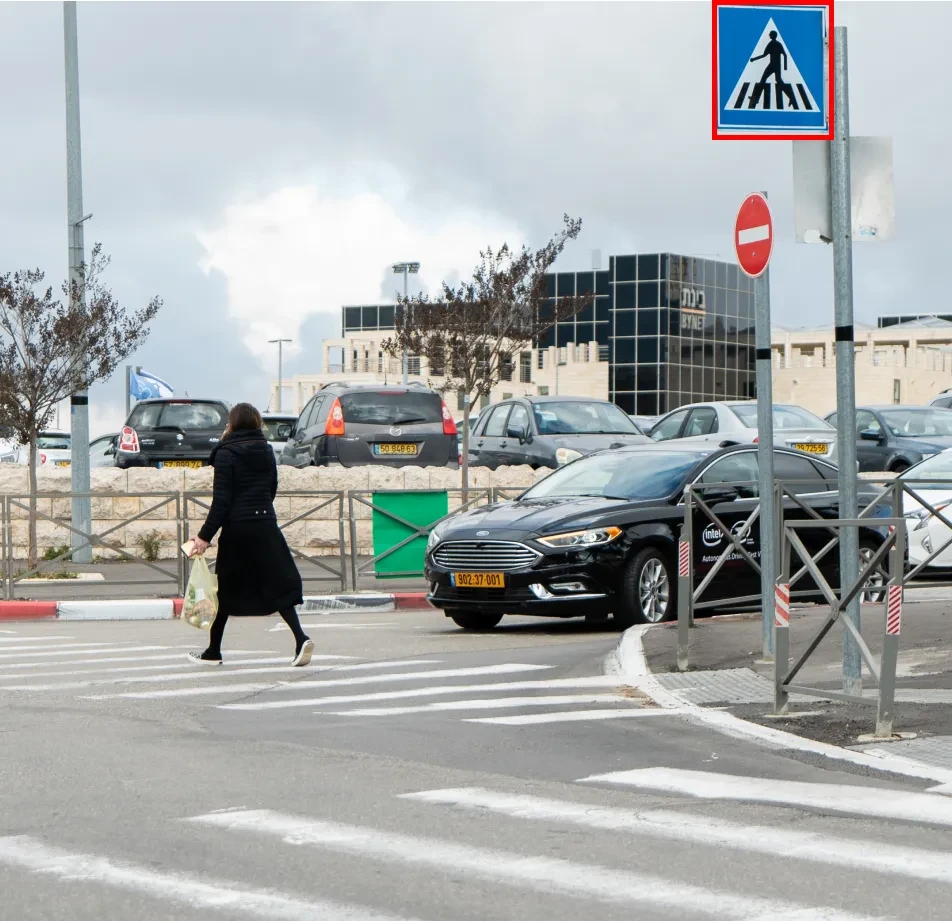
Quality Assurance
Stages
Data Privacy: Protecting personal information in collected data.
Quality Control: Ensuring accurate annotations for reliable models.
Access Restrictions: Limiting access to sensitive traffic sign data.
QA Metrics
- Accuracy Rate: Percentage of correctly detected traffic signs.
- False Positive Rate: Proportion of incorrectly identified signs relative to all detections.
Conclusion
This traffic sign detection project underscores our capability and expertise in data collection and annotation for autonomous vehicles. By providing a comprehensive, accurately annotated dataset, we ensure that autonomous vehicles interpret and respond correctly to road signs, promoting safe navigation and adherence to traffic regulations.

Quality Data Creation

Guaranteed TAT

ISO 9001:2015, ISO/IEC 27001:2013 Certified

HIPAA Compliance

GDPR Compliance

Compliance and Security
Let's Discuss your Data collection Requirement With Us
To get a detailed estimation of requirements please reach us.