Outdoor Objects Semantic Segmentation Dataset
Home » Case Study » Outdoor Objects Semantic Segmentation Dataset
Project Overview:
Objective
Construct a comprehensive dataset tailored for the semantic segmentation of various outdoor objects. This dataset is intended to push forward advancements in environmental studies, smart cities, and augmented reality navigation.
Scope
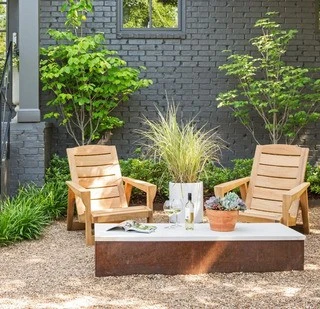
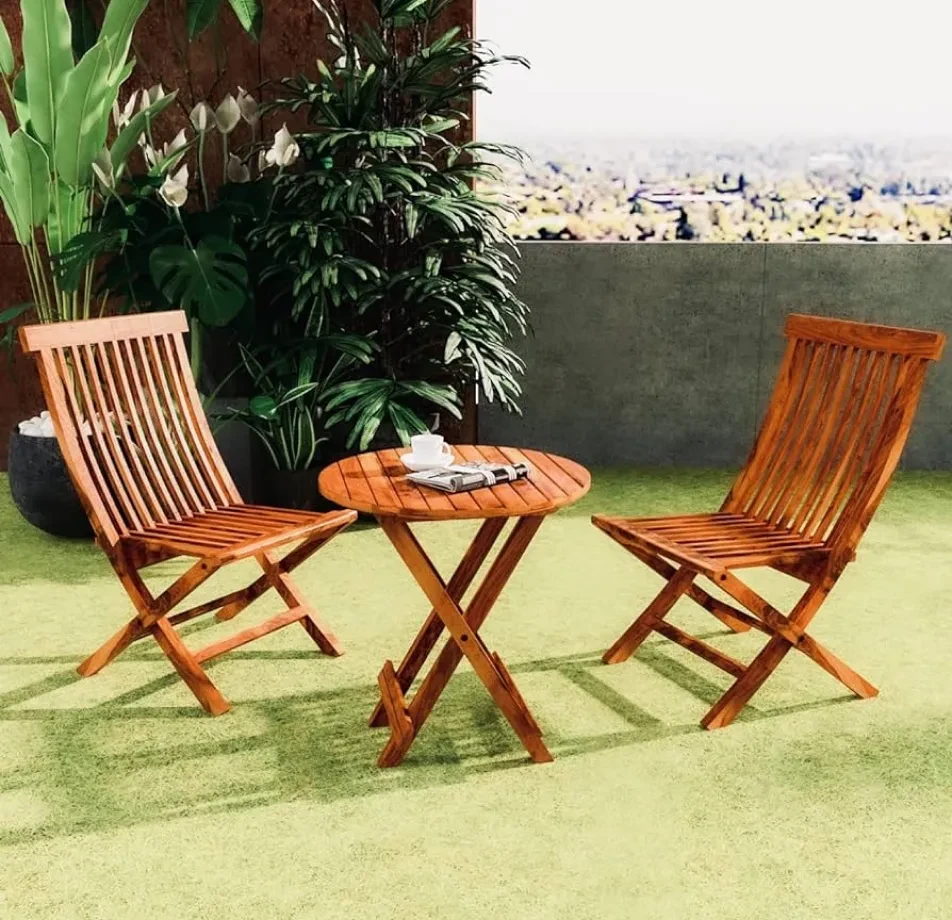
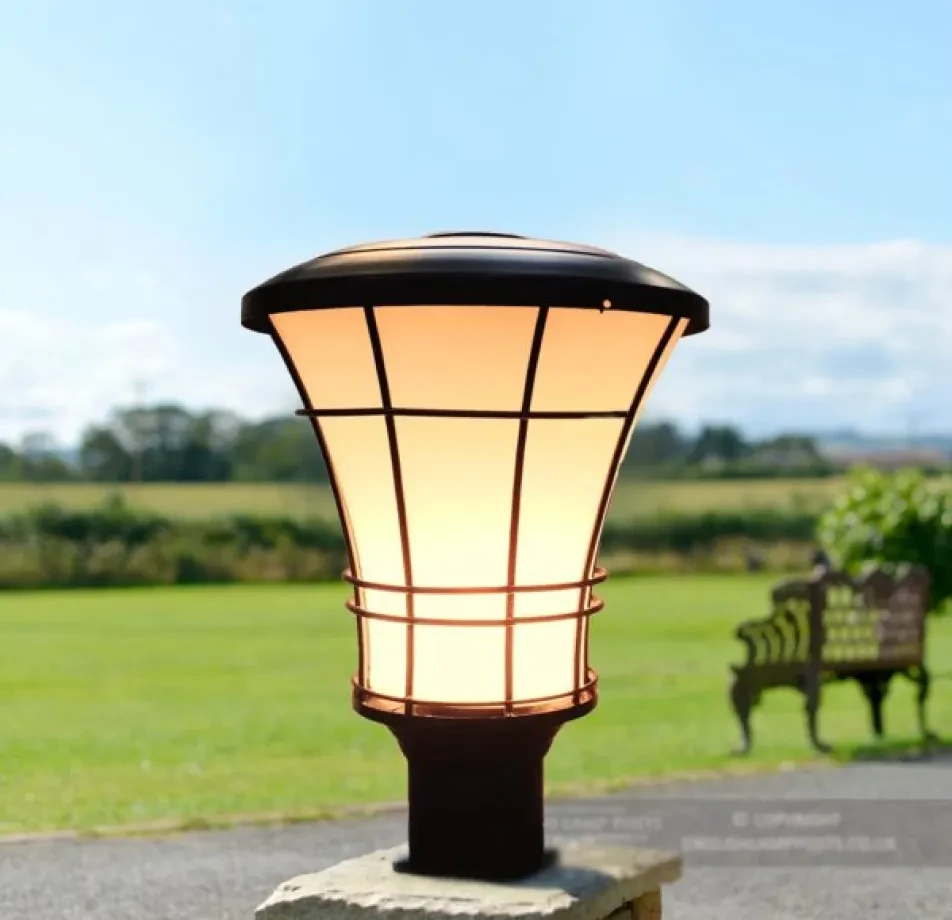
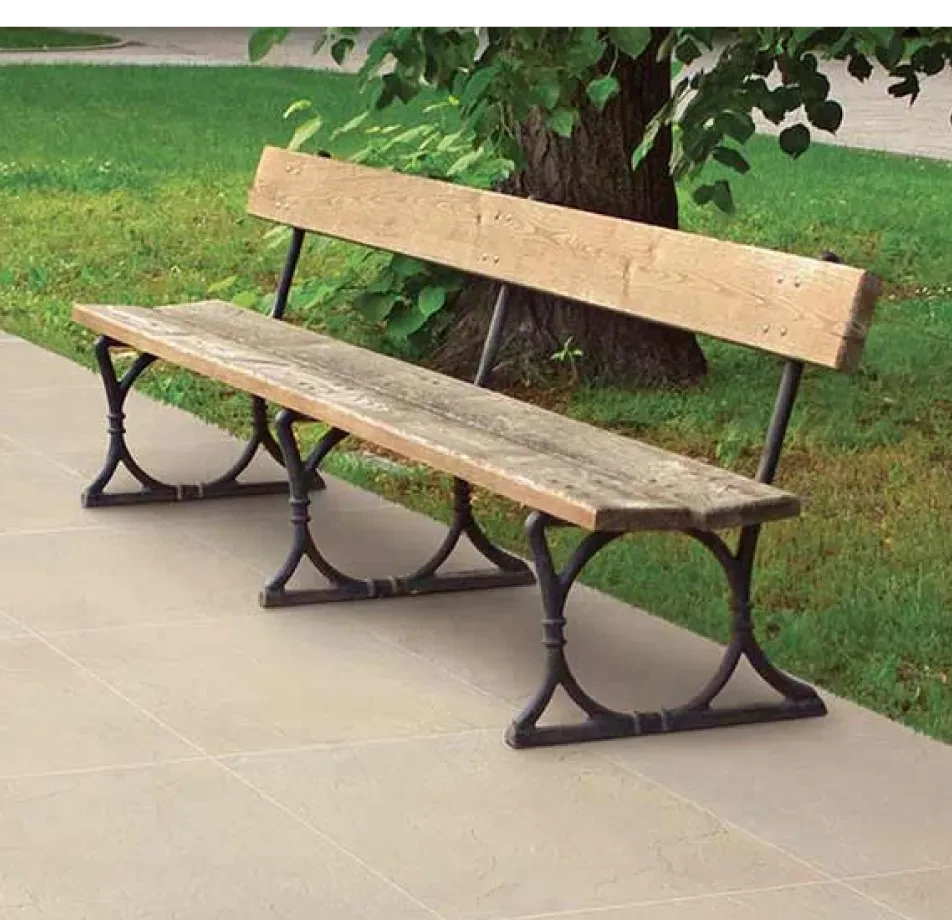
Sources
Collaboration with urban planning commissions and forestry departments can greatly enhance the data collection process. Moreover, their expertise ensures accurate and useful data for various applications.
Drone-captured footage of parks, forests, and urban areas provides detailed and up-to-date imagery. Additionally, this method allows for comprehensive coverage of large areas quickly.
Street view images from mapping services offer a ground-level perspective. Furthermore, they provide valuable insights into urban infrastructure and layout.
Contributions from community-driven photography initiatives add a diverse range of images. Not only do they supplement professional data sources, but they also engage the community in data collection efforts
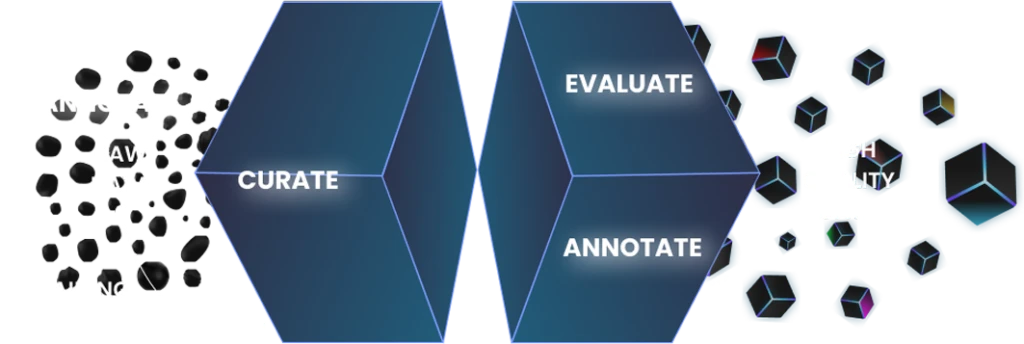
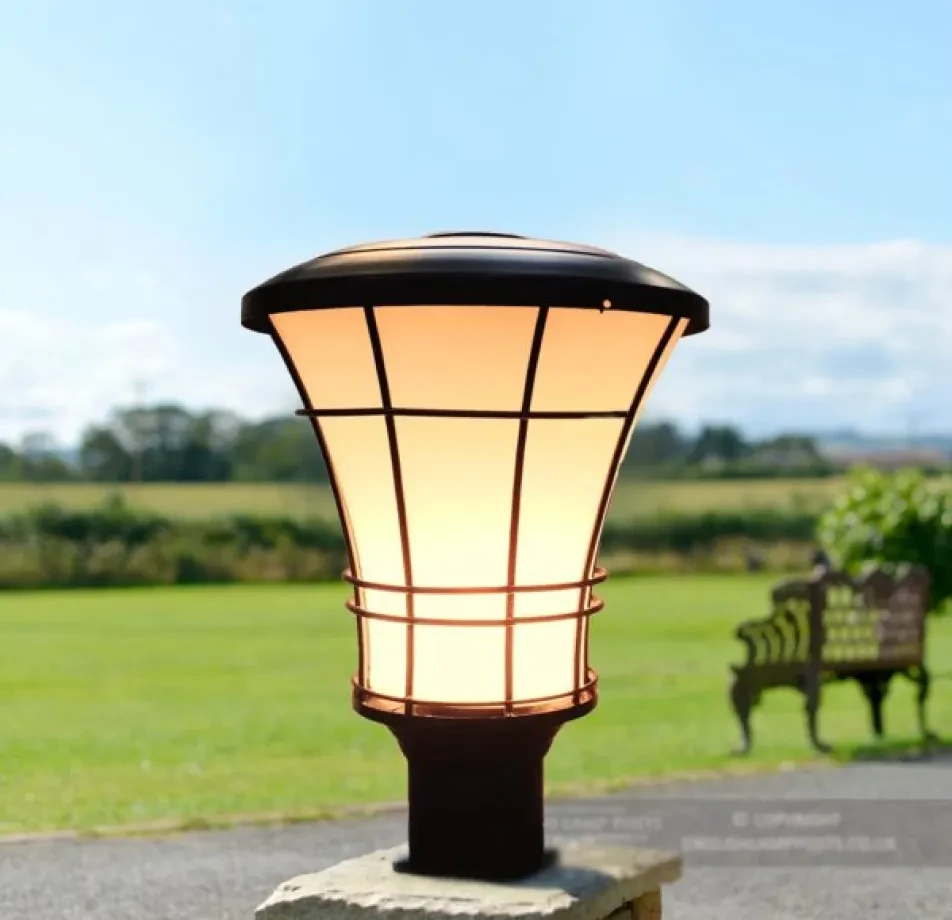
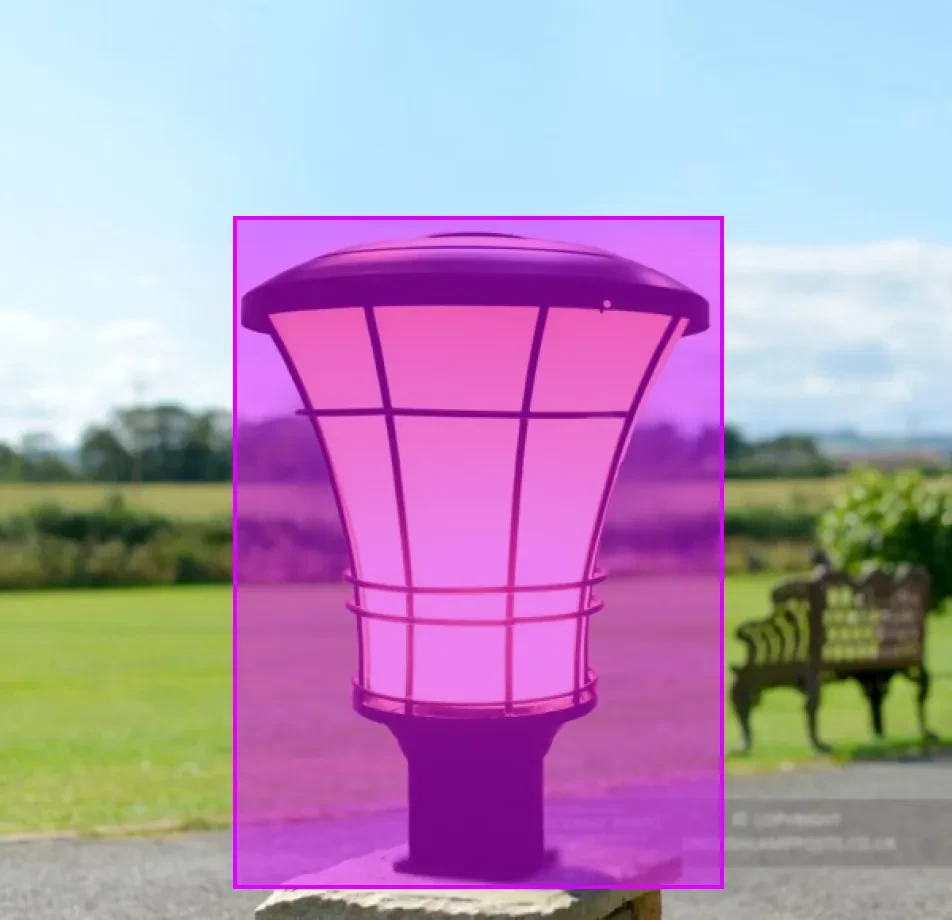
Data Collection Metrics
- Total Images: 25,000
- Urban Landscapes: 10,000
- Rural Settings: 5,000
- Forest Areas: 6,000
- Waterfronts: 4,000
Annotation Process
Stages
- Image Pre-processing: Initially, standardization for light balancing, resolution enhancement, and size normalization is performed to guarantee uniform image quality.
- Pixel-wise Segmentation: Subsequently, annotators use specialized software to meticulously define every pixel associated with distinct outdoor objects.
- Validation:Â annotations undergo verification by a secondary team to ensure precision and consistency.
Annotation Metrics
- Total Pixel-wise Annotations: 25,000 (One for each image). Consequently, this ensures comprehensive coverage and detail for each image.
- Average Annotation Time per Image: 30 minutes. This is due to the complexity and diversity of outdoor elements. Moreover, the detailed annotation process enhances the accuracy and utility of the dataset.
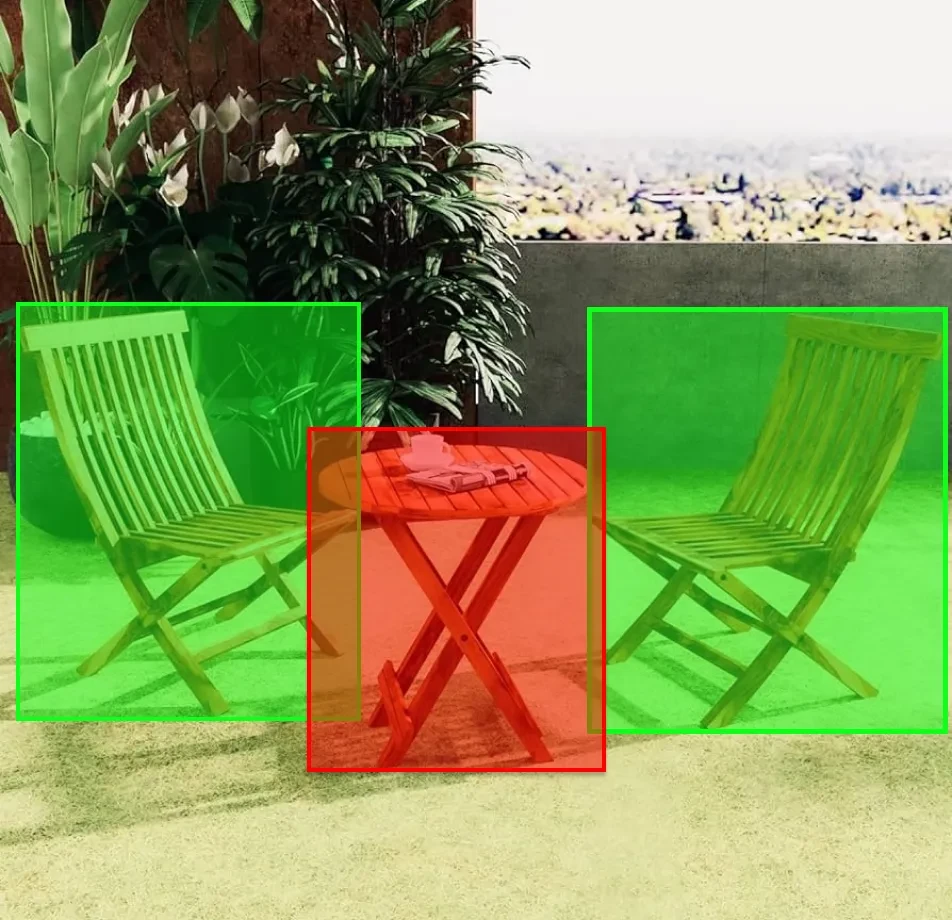
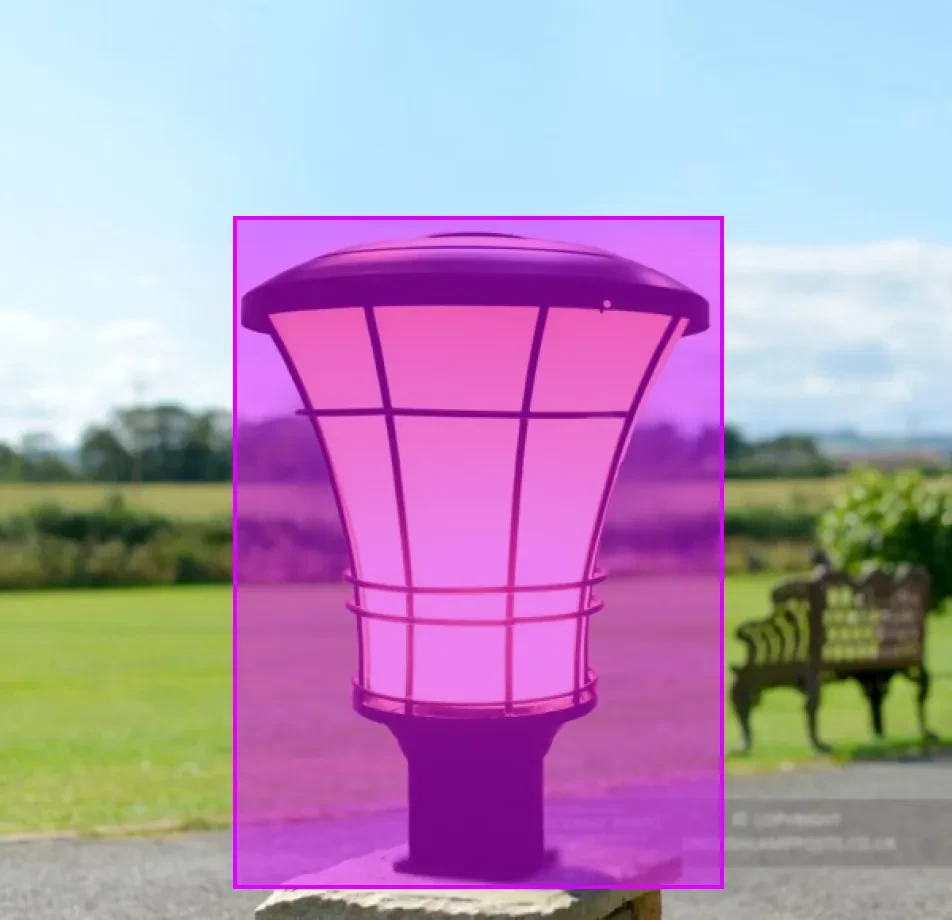
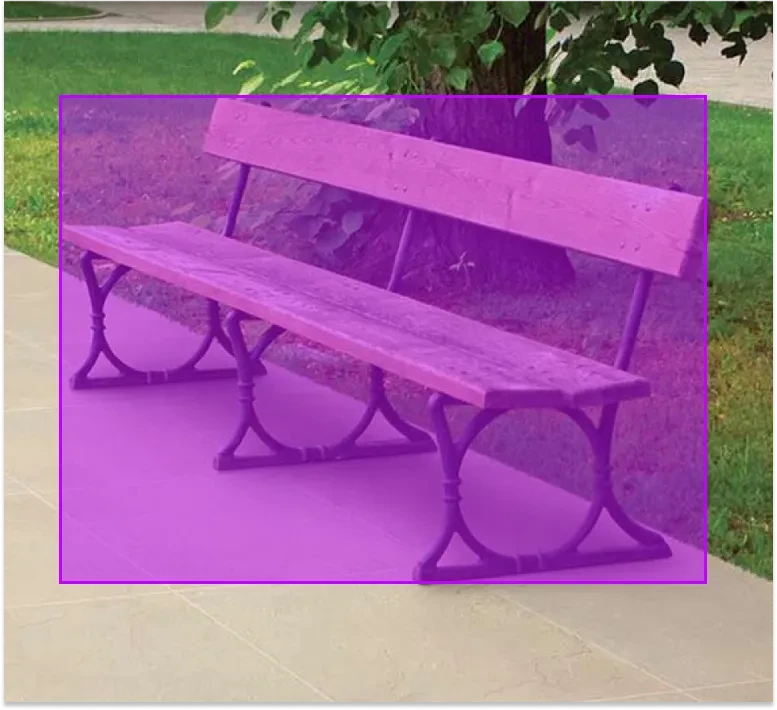
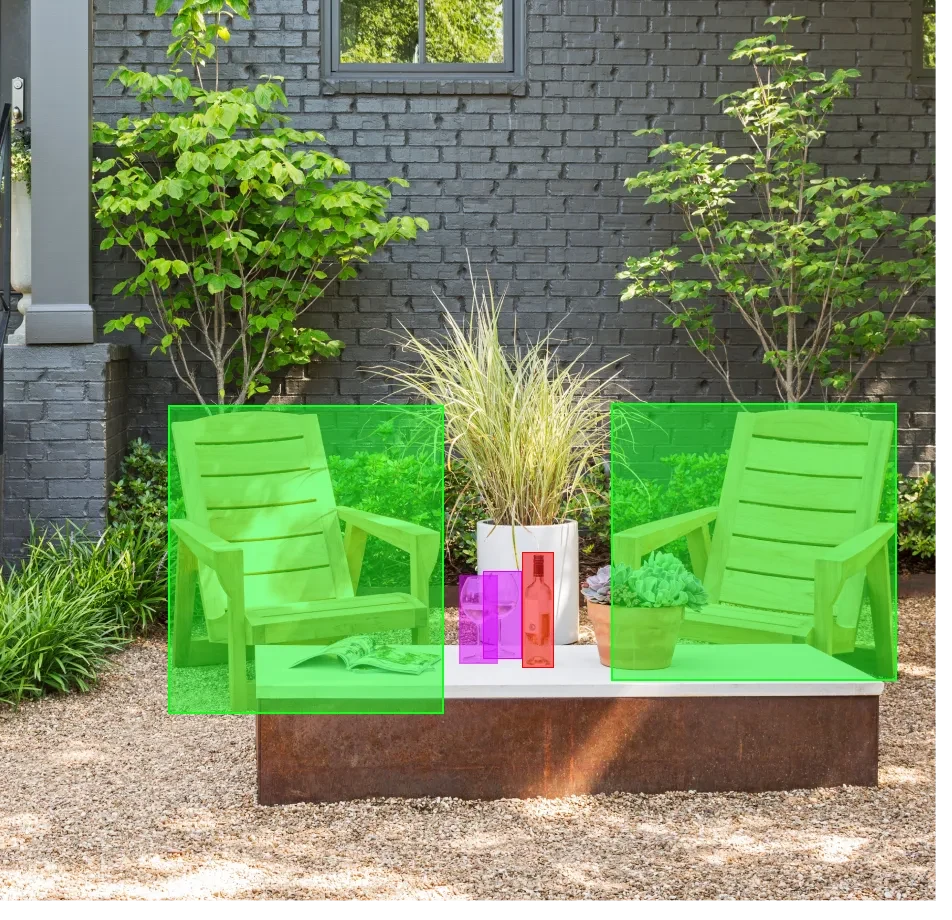
Quality Assurance
Stages
Automated Model Comparison: Preliminary semantic segmentation models aid in contrasting annotations, thereby identifying possible mismatches.
Peer Review: A subset of the dataset undergoes rigorous review by senior annotators to confirm consistent quality.
Inter-annotator Agreement: Select images are annotated by multiple individuals to maintain and ensure a consistent quality threshold.
QA Metrics
- Annotations Verified using Models: 12,500 (50% of the total images). Consequently, this process ensures a high level of accuracy and reliability in the dataset.
- Peer-reviewed Annotations: 7,500 (30% of the total images). Additionally, peer review adds an extra layer of validation, enhancing the overall quality.
- Inconsistencies Identified and Addressed: 750 (3% of the total images). Moreover, addressing these inconsistencies improves the dataset’s integrity and usability.
Conclusion
The Outdoor Objects Semantic Segmentation Dataset represents a groundbreaking advancement in computer vision, particularly addressing the expansive realm of outdoor environments. Whether utilized for environmental conservation, the development of smart urban infrastructure, or the creation of immersive AR experiences, this dataset lays a solid groundwork for the forthcoming wave of outdoor-focused technological innovations.

Quality Data Creation

Guaranteed TAT

ISO 9001:2015, ISO/IEC 27001:2013 Certified

HIPAA Compliance

GDPR Compliance

Compliance and Security
Let's Discuss your Data collection Requirement With Us
To get a detailed estimation of requirements please reach us.