Obvious Objects Segmentation Dataset
Home » Case Study » Obvious Objects Segmentation Dataset
Project Overview:
Objective
Scope
Compile an assortment of images capturing various commonplace settings where certain objects naturally stand out due to their size, color, or importance. Each image will feature pixel-wise annotations isolating these obvious objects from the background and other less prominent items.
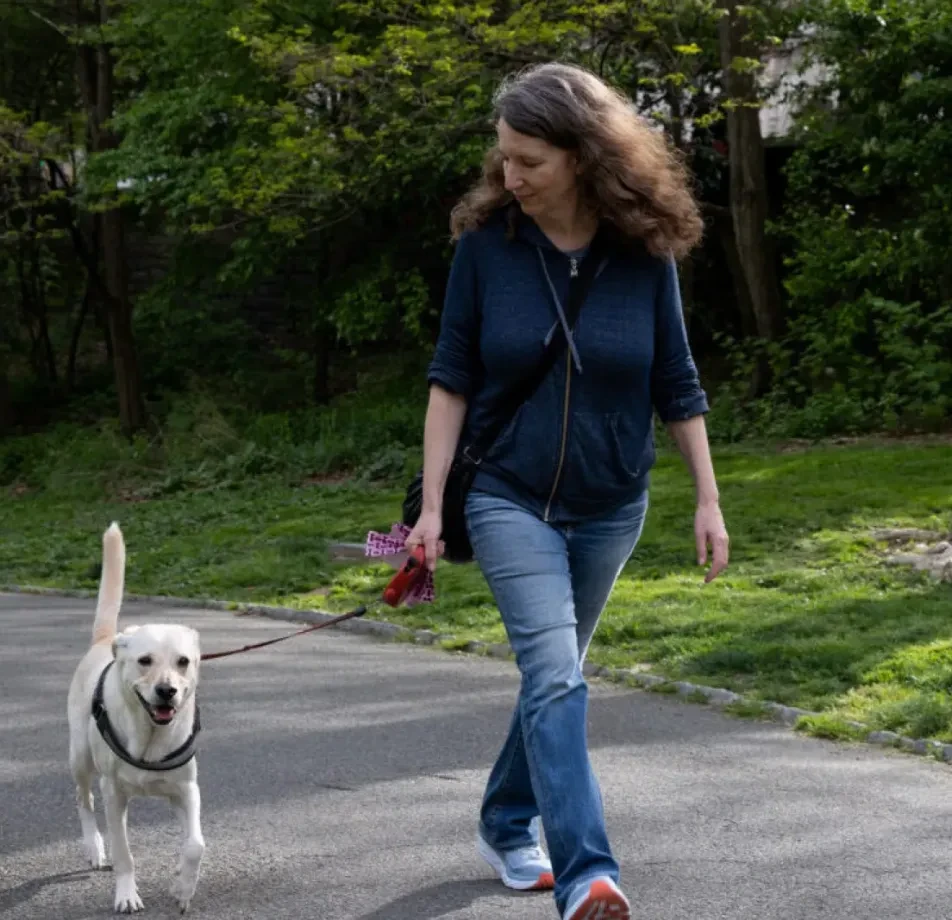
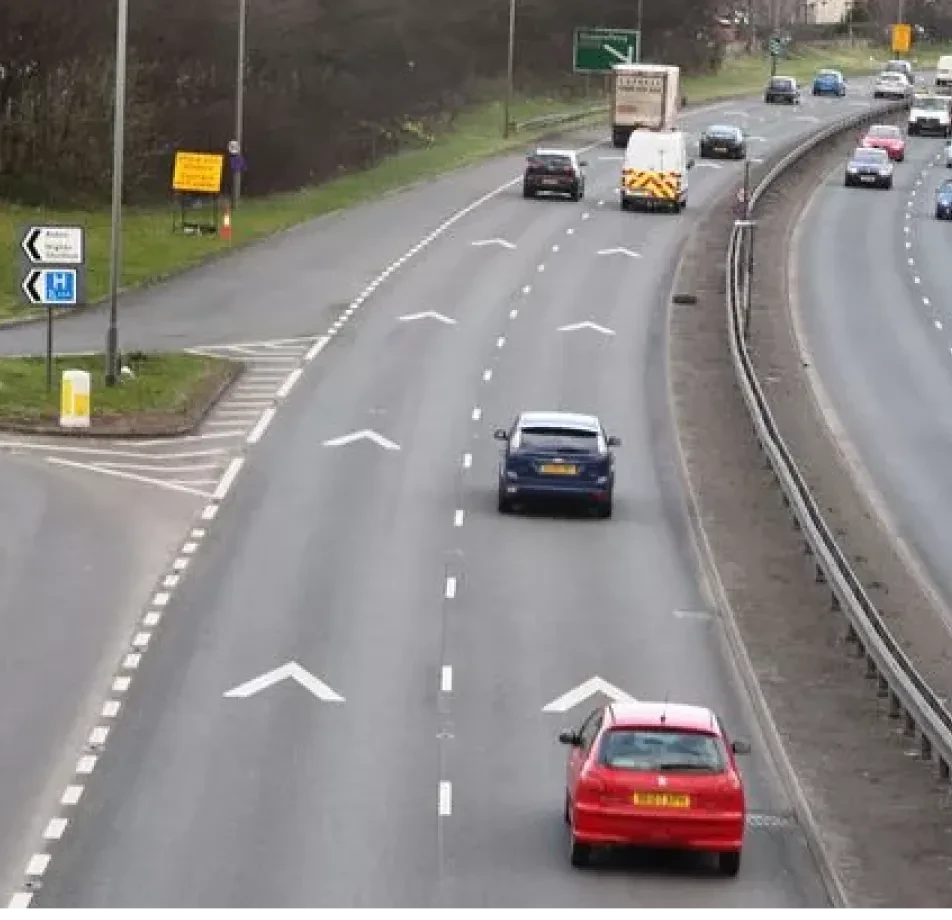
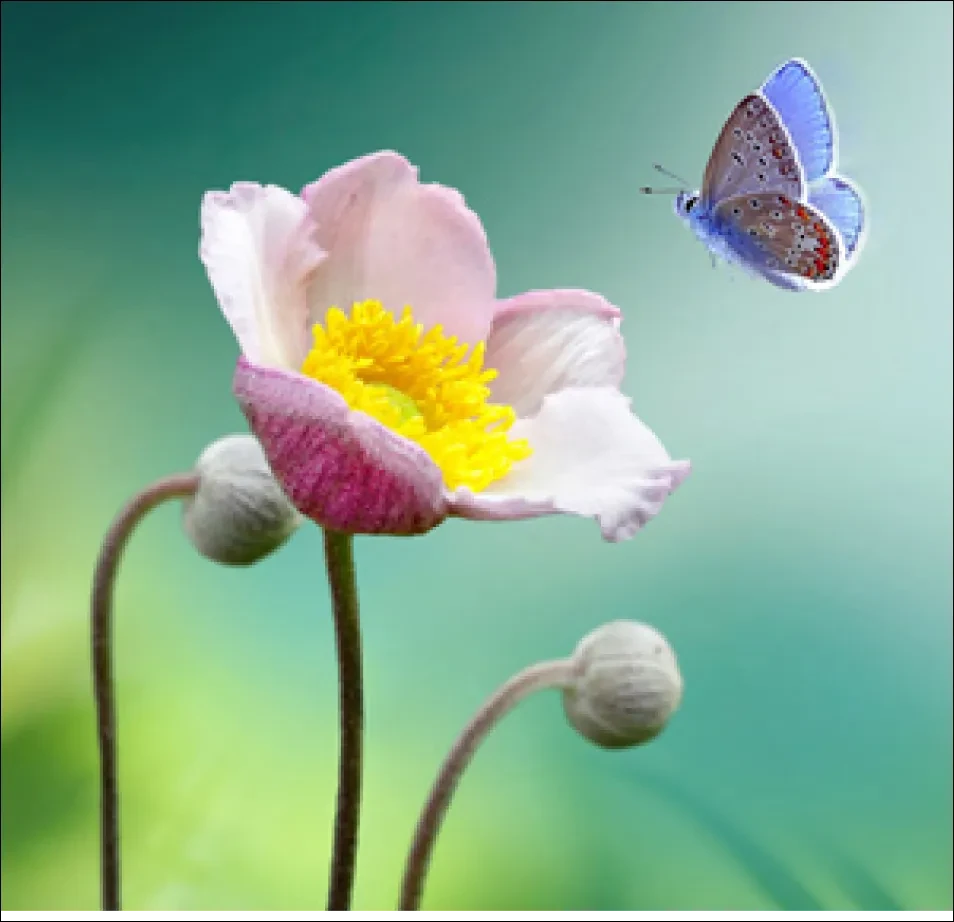
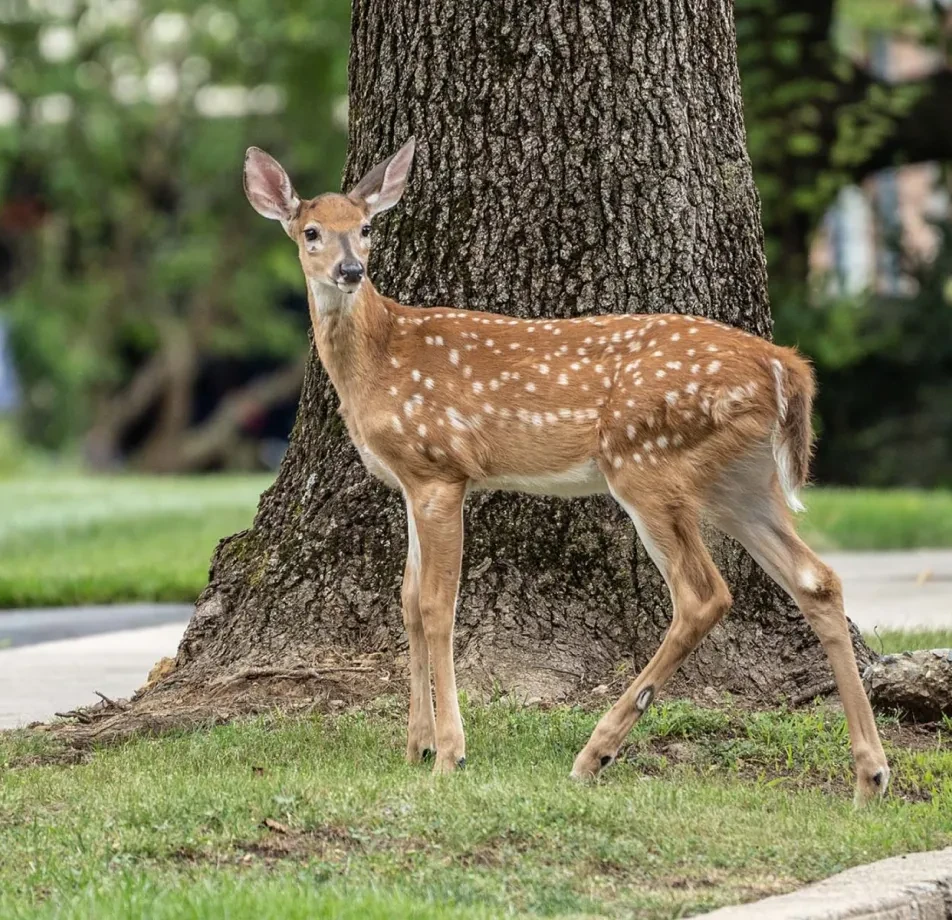
Sources
- Public and private spaces, such as parks, squares, living rooms, and kitchens.
- high-traffic areas like train stations, airports, and shopping malls.
- crowdsourced inputs through a dedicated platform soliciting diverse environmental captures.
- collaborations with city planning and public transport authorities
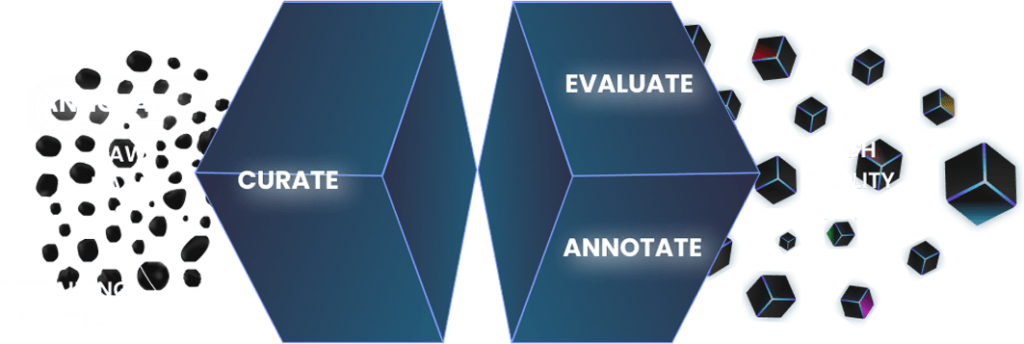
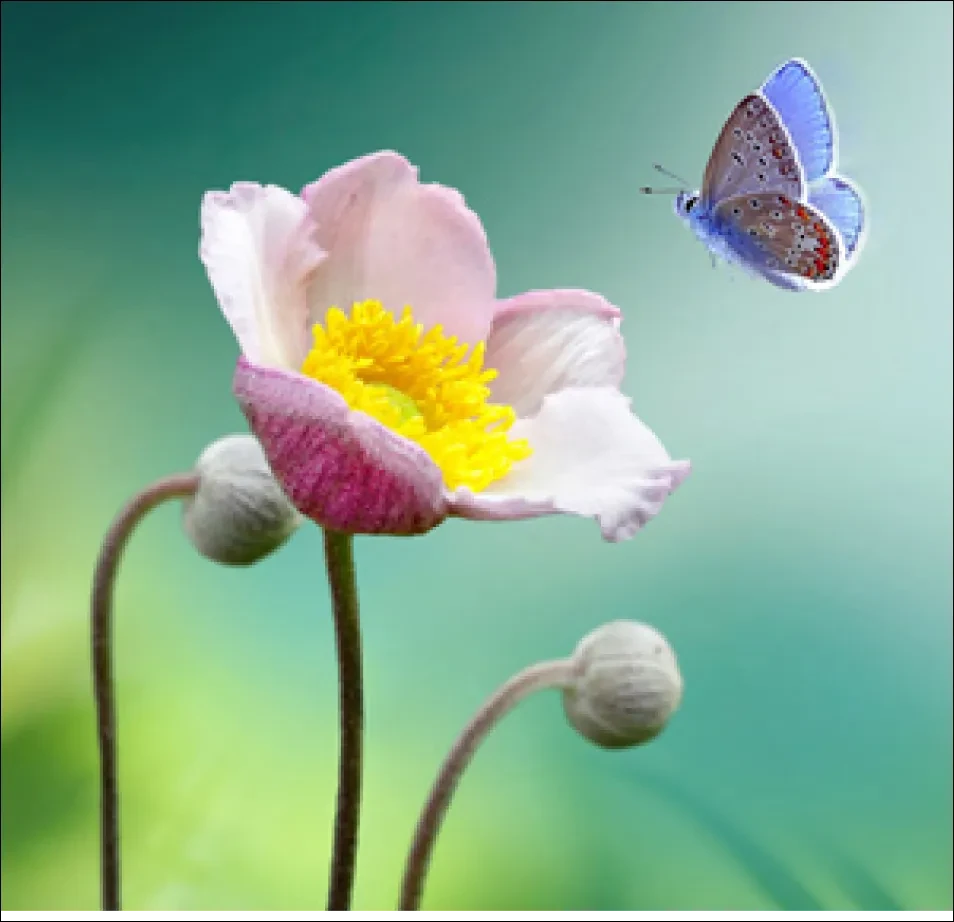
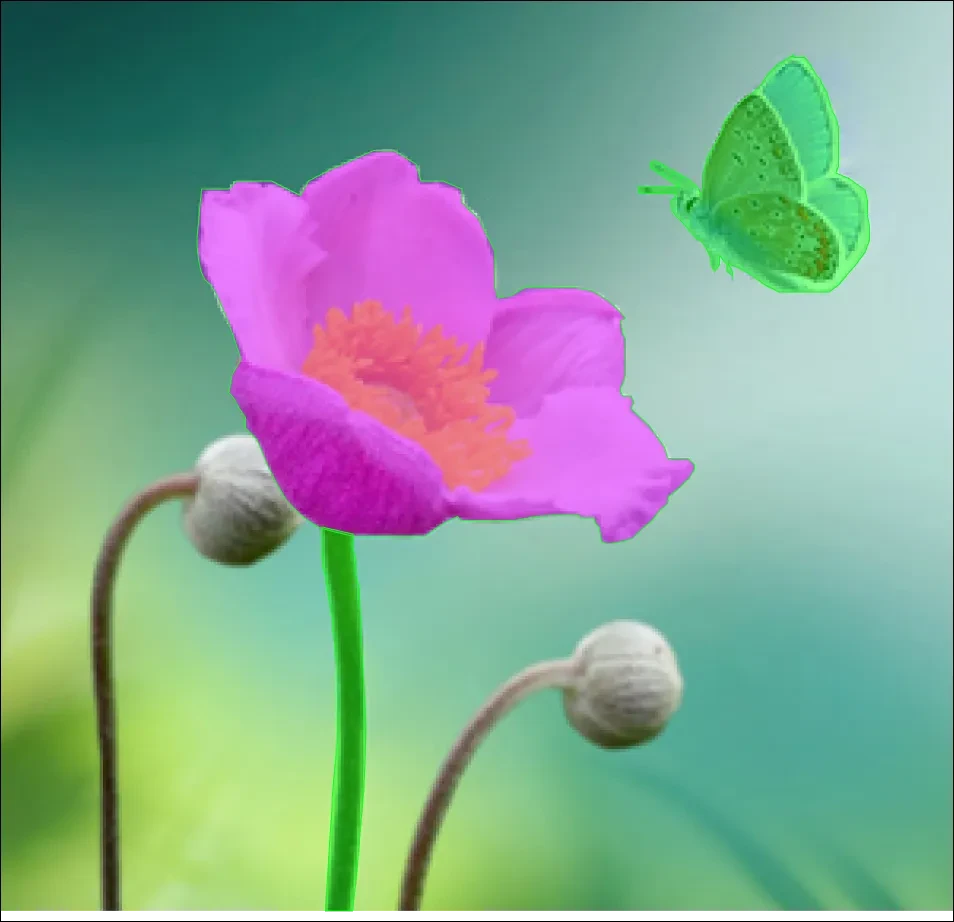
Data Collection Metrics
- Total Images: 22,000
- Public Spaces: 8,000
- Indoor Settings: 7,000
- Transport Hubs: 5,000
- Miscellaneous: 2,000
Annotation Process
Stages
- Image Pre-processing: standardization techniques will be applied to ensure consistent visual quality across the dataset.
- Pixel-wise Segmentation:utilizing state-of-the-art annotation tools, annotators will meticulously define every pixel associated with the “obvious objects” present in each scene.
- Validation: Annotation, a secondary team will meticulously cross-check the annotations to confirm both correctness and thoroughness.
Annotation Metrics
- Total Pixel-wise Annotations: 22,000 (one for each image)
- Moreover, the average annotation time per image: 25 minutes (given the variance of objects and their environments)
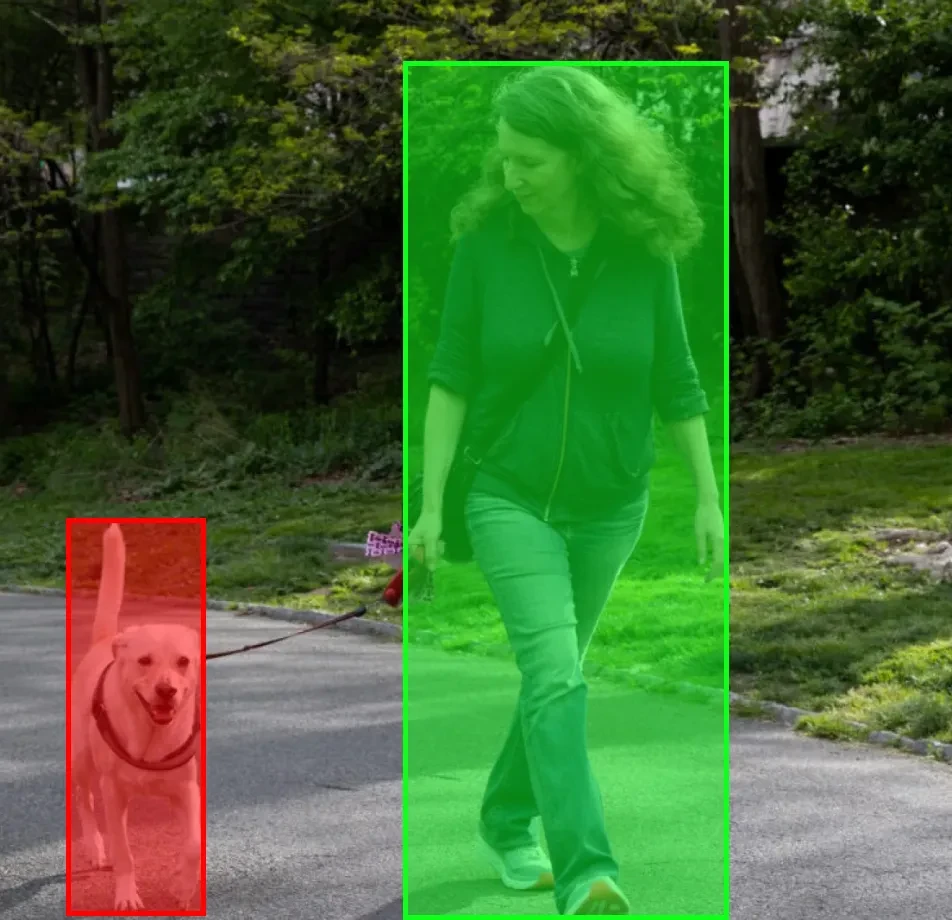
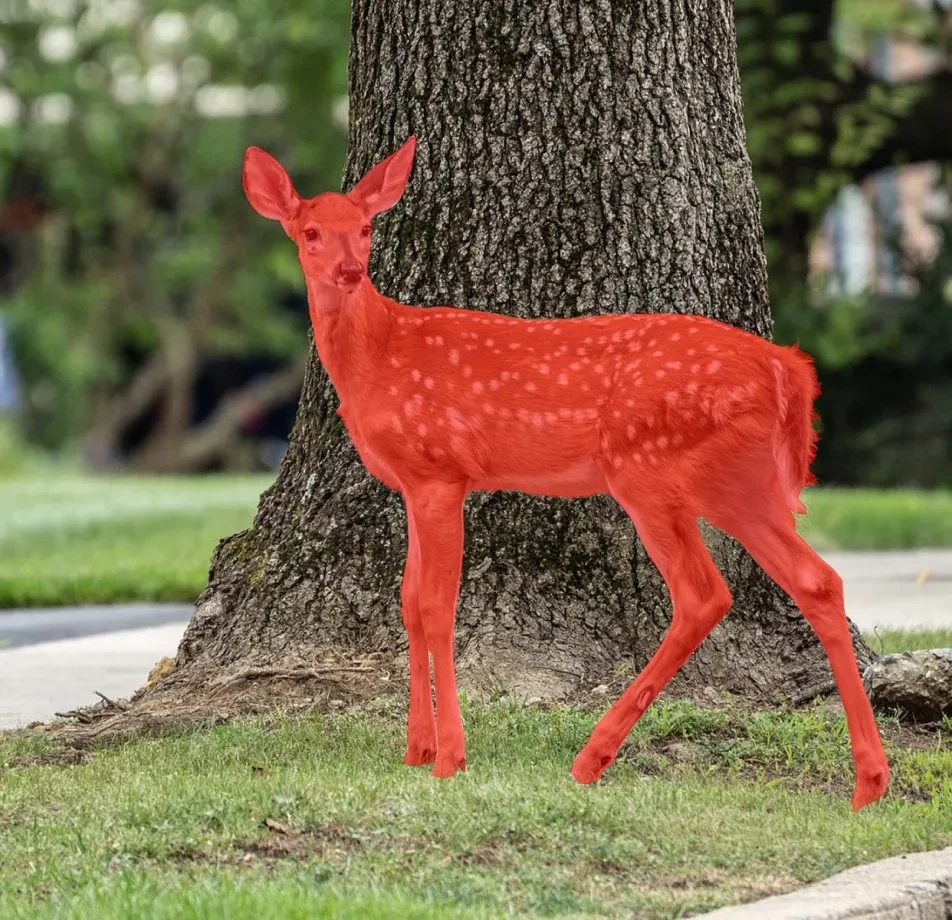
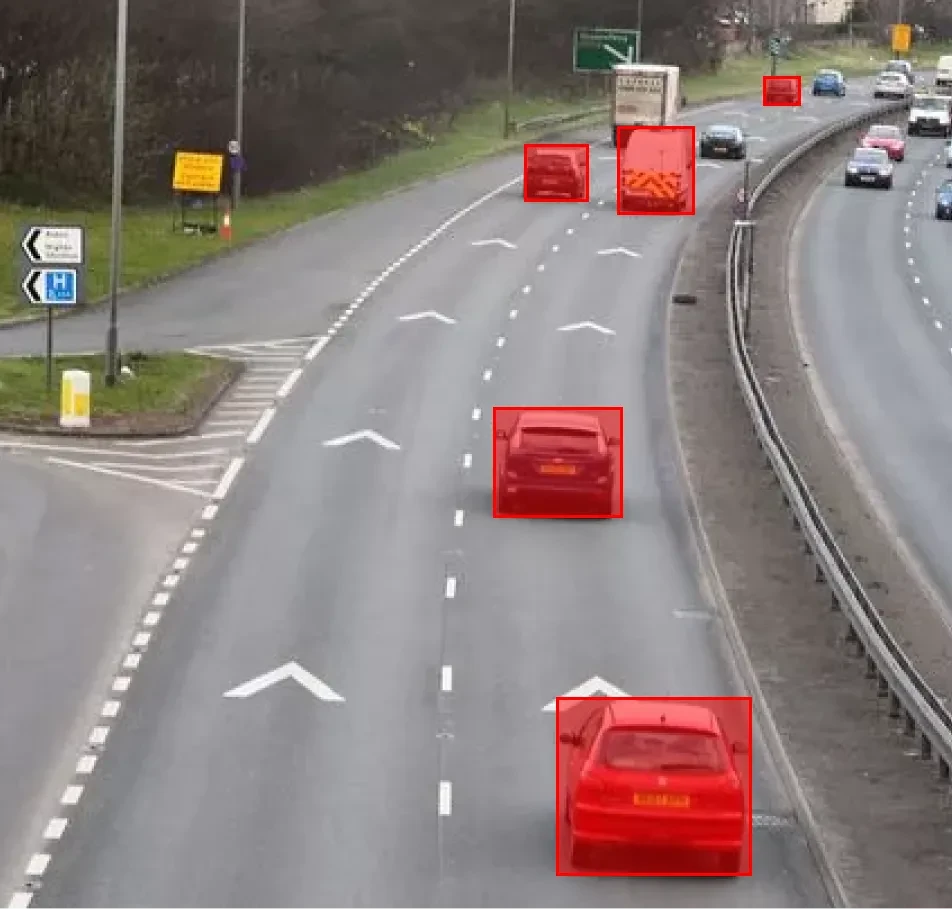
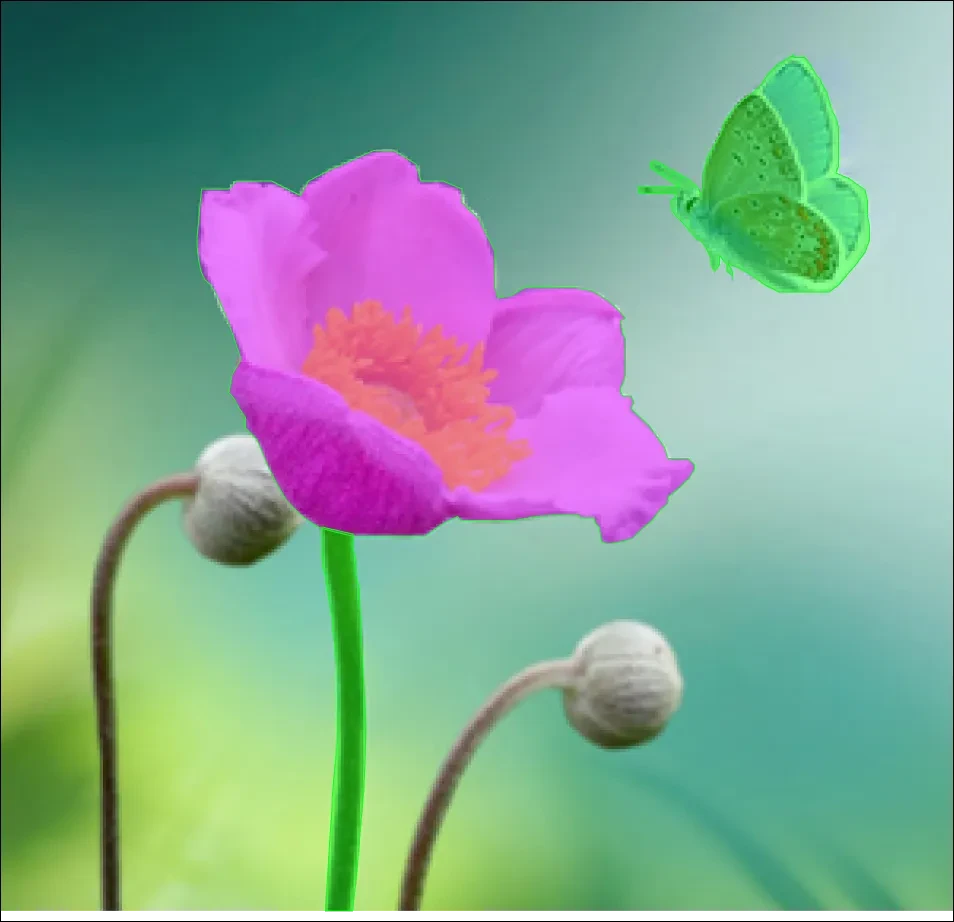
Quality Assurance
Stages
Automated Model Review: Furthermore, early-stage segmentation models are used to verify the integrity of annotations.
Peer Review: Additionally, selected images undergo rigorous scrutiny by senior annotators to ensure a gold standard of quality.
Inter-annotator Agreement: Moreover, a few images are set aside to be annotated by multiple experts, ensuring the uniformity of interpretation regarding what qualifies as an “obvious object”.
QA Metrics
- Annotations Verified using Models: 11,000 (representing 50% of total images)
- Additionally, peer-reviewed annotations account for 6,600 images (30% of total images)
- Furthermore, inconsistencies identified and resolved stand at 440 (2% of total images)
Conclusion
The Obvious Objects Segmentation Dataset marks a crucial step, in enhancing machine comprehension of everyday environments. By highlighting and understanding those objects, that naturally draw human attention, this dataset will undoubtedly drive innovations, in safety, accessibility, and real-time image interpretation. It’s a testament to the symbiotic relationship, between human intuition and machine learning.

Quality Data Creation

Guaranteed TAT

ISO 9001:2015, ISO/IEC 27001:2013 Certified

HIPAA Compliance

GDPR Compliance

Compliance and Security
Let's Discuss your Data collection Requirement With Us
To get a detailed estimation of requirements please reach us.