Named Entity Recognition for News Article Analysis
Home » Case Study » Named Entity Recognition for News Article Analysis
Project Overview:
Objective
In our mission to support advancements in journalism and research, we actively focus on Named Entity Recognition (NER) for news article analysis. To effectively retrieve information and analyze content in an evolving landscape, our objective is to identify and categorize entities accurately while embracing language diversity and real-world applications. Furthermore, we aim to nail down the identification and grouping of different entities, thereby enabling us to pull out information more effectively and analyze content like a pro. This move is pretty vital, you know, considering languages and real-world uses are always shifting and evolving.
Scope
Our mission revolves around meticulously sorting entities in news content, elevating our analysis game, and facilitating information search effortlessly, all while providing deeper insights. We aim to enhance the thoroughness of news content analysis by carefully identifying and categorizing every mention. In doing so, we strive to significantly enhance the efficacy of content analysis, streamline information retrieval, and foster a more comprehensive understanding. Our approach is adaptable to various languages and real-world applications, spanning from news coverage to research, ultimately playing a pivotal role in aiding informed decision-making.
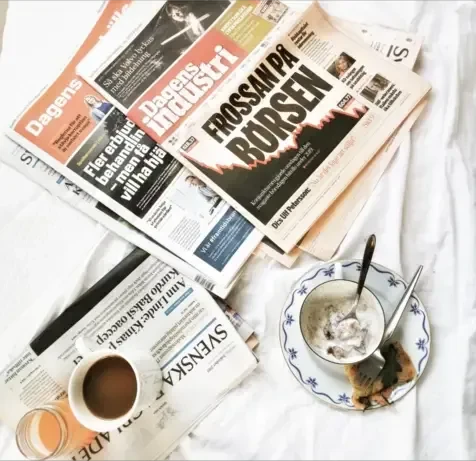
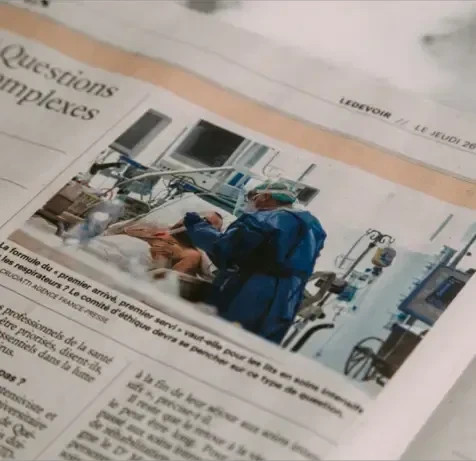
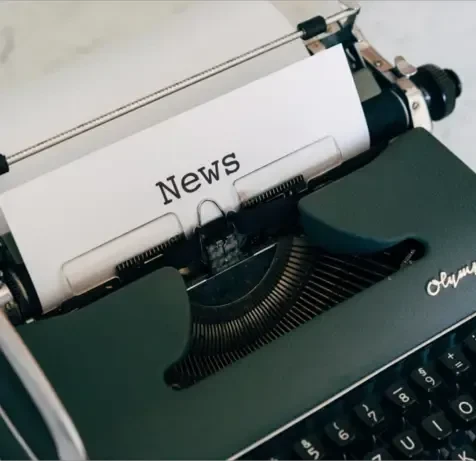
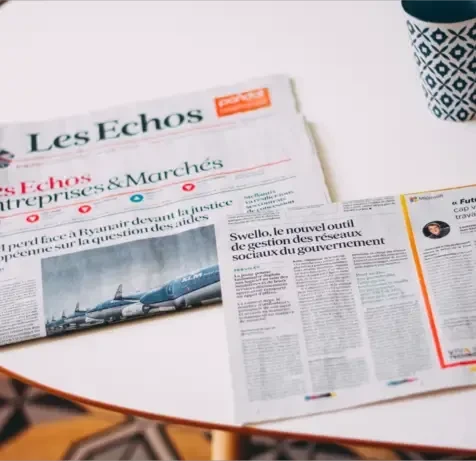
Sources
- Our arsenal of linguistic resources comprises an extensive array of linguistic databases, named entity dictionaries, and language-specific datasets. These resources play a pivotal role in actively training our NER models by providing a rich tapestry of linguistic data. Additionally, they serve as vital components in enhancing the accuracy and efficiency of our models.
- In our news analysis process, we actively utilize advanced Natural Language Processing (NLP) libraries such as spaCy and NLTK, alongside pre-trained Named Entity Recognition (NER) models. These tools play a pivotal role in enhancing the efficiency and effectiveness of our analysis. Transitioning to more active voice emphasizes our proactive engagement with these resources, enhancing clarity and directness.
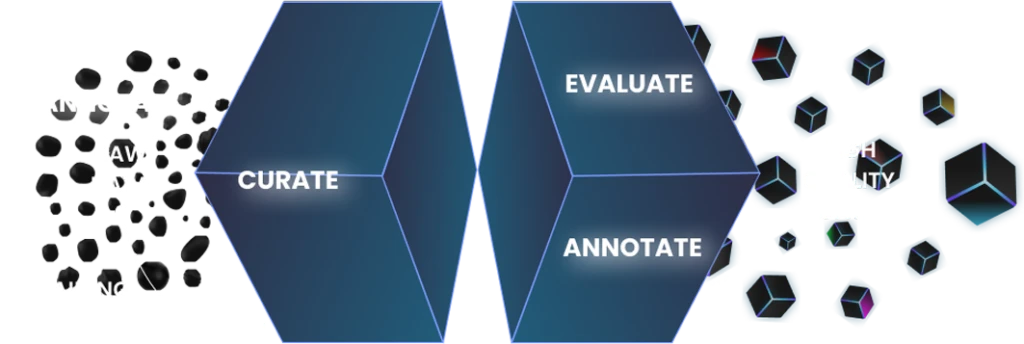
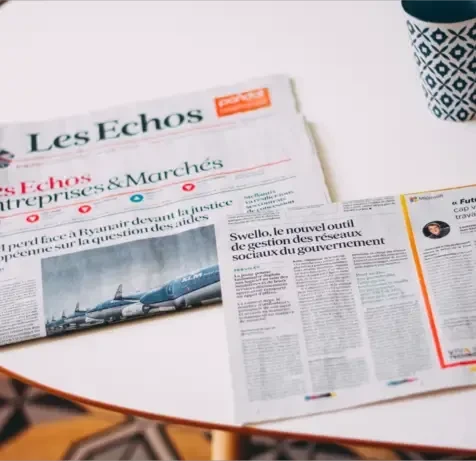
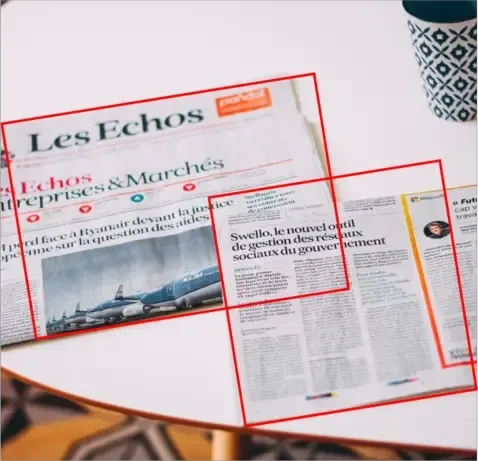
Data Collection Metrics
- Data Volume: We have successfully collected and annotated 150,000 news articles, providing a robust dataset for our NER models.
- Annotation Quality: Our focus on accuracy and consistency in named entity annotations ensures that we have reliable training and evaluation data.
Annotation Process
Stages
- Data Collection: Our team has diligently collected a diverse range of news articles and textual data for in-depth analysis. Additionally, we have systematically organized the gathered information to facilitate efficient examination. Furthermore, we have actively sought out relevant sources to ensure comprehensive coverage of the topic.
- Data Preprocessing: We’ve diligently cleaned and formatted the data specifically for NER, ensuring the highest quality input for our models. Furthermore, we’ve employed rigorous standards to enhance the data’s readiness for NER analysis. In doing so, we’ve actively refined the dataset, guaranteeing optimal performance for our models.
- NER Model Development: Our team has successfully developed and trained state-of-the-art NER models, enabling them to proficiently identify and categorize named entities. Additionally, these models have been fine-tuned to ensure optimal performance in real-world scenarios.
- NER Application: Transition words can effectively enhance the flow of information within news articles, thereby facilitating the process of entity recognition. By skillfully integrating these transition words, our models actively excel in identifying entities within news articles.
- Analysis and Insights: The extracted entities not only significantly bolster our content analysis but also enhance our capabilities in information retrieval, providing valuable insights for journalism and research purposes.
Annotation Metrics
- Label Quality: Our annotators’ precision is continually evaluated to maintain high accuracy and consistency in labeling.
- Inter-Annotator Agreement: We consistently measure annotator agreement levels to ensure the reliability of our labels.
- Feedback Loop: An established feedback mechanism allows annotators to refine guidelines and address ambiguities, fostering ongoing quality in annotation.
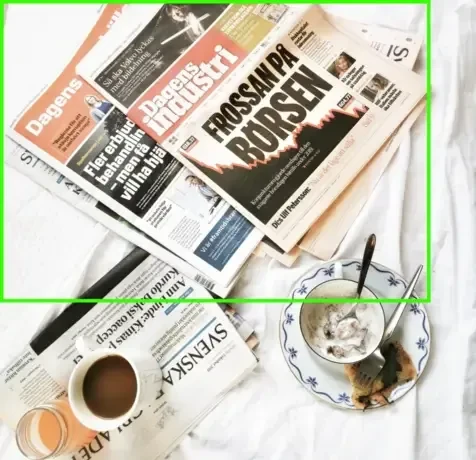
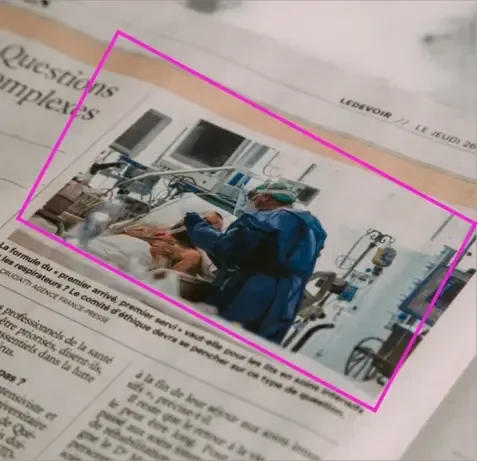
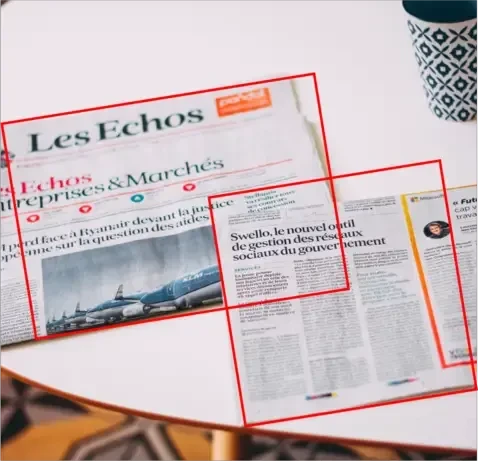
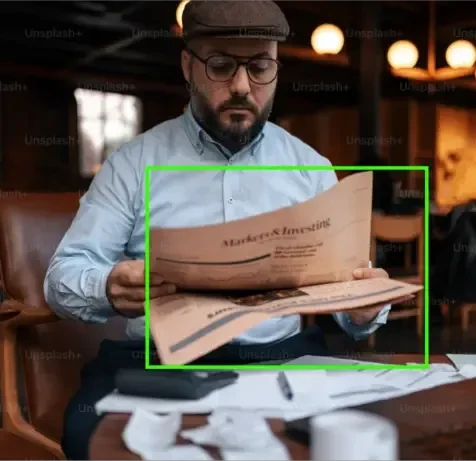
Quality Assurance
Stages
Data Quality: Rigorous data quality checks are a standard part of our process, ensuring the accuracy and reliability of the data we collect.
Privacy Protection: We strictly adhere to privacy regulations, ensuring all data is anonymized and untraceable to individuals.
Data Security: Our robust data security measures safeguard sensitive information at every stage.
QA Metrics
- Data Accuracy: Regular validation checks are conducted to guarantee data accuracy.
- Privacy Compliance: We continuously audit our data handling processes to ensure full compliance with privacy standards.
Conclusion
Therefore, small businesses and startups must get creative and strategic with their limited marketing budgets. We’re totally committed to boosting machine learning models, and that’s why we’ve put so much effort into gathering and labeling data for identifying key terms in news articles. With our spot-on data sets, we’re powering up improvements in how you hunt for info, boil down content, gauge emotional vibes from text, and pinpoint key themes. Our work not only fuels journalism and research that keeps evolving but also rockets forward decisions based on solid data into the future.

Quality Data Creation

Guaranteed TAT

ISO 9001:2015, ISO/IEC 27001:2013 Certified

HIPAA Compliance

GDPR Compliance

Compliance and Security
Let's Discuss your Data collection Requirement With Us
To get a detailed estimation of requirements please reach us.