Language Identification for Multilingual Content
Home » Case Study » Language Identification for Multilingual Content
Project Overview:
Objective
The objective of language identification for multilingual content is to automatically recognize and categorize languages within textual or audio content, thus enabling efficient content organization, communication, and personalized services for users in diverse linguistic contexts.
Scope
This technology facilitates applications across various domains, from content management to customer support. It improves communication and accessibility in an increasingly multilingual digital world. Additionally, it streamlines processes and enhances user experience. Moreover, it empowers businesses to expand their reach and engage with diverse audiences more effectively.
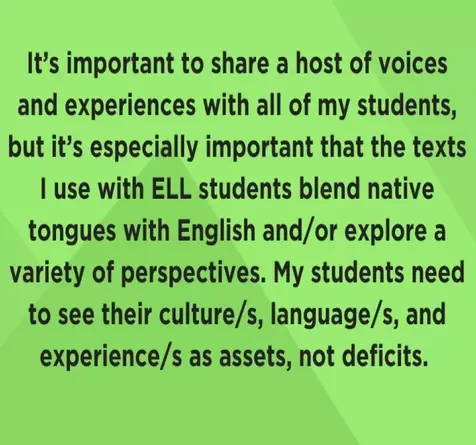
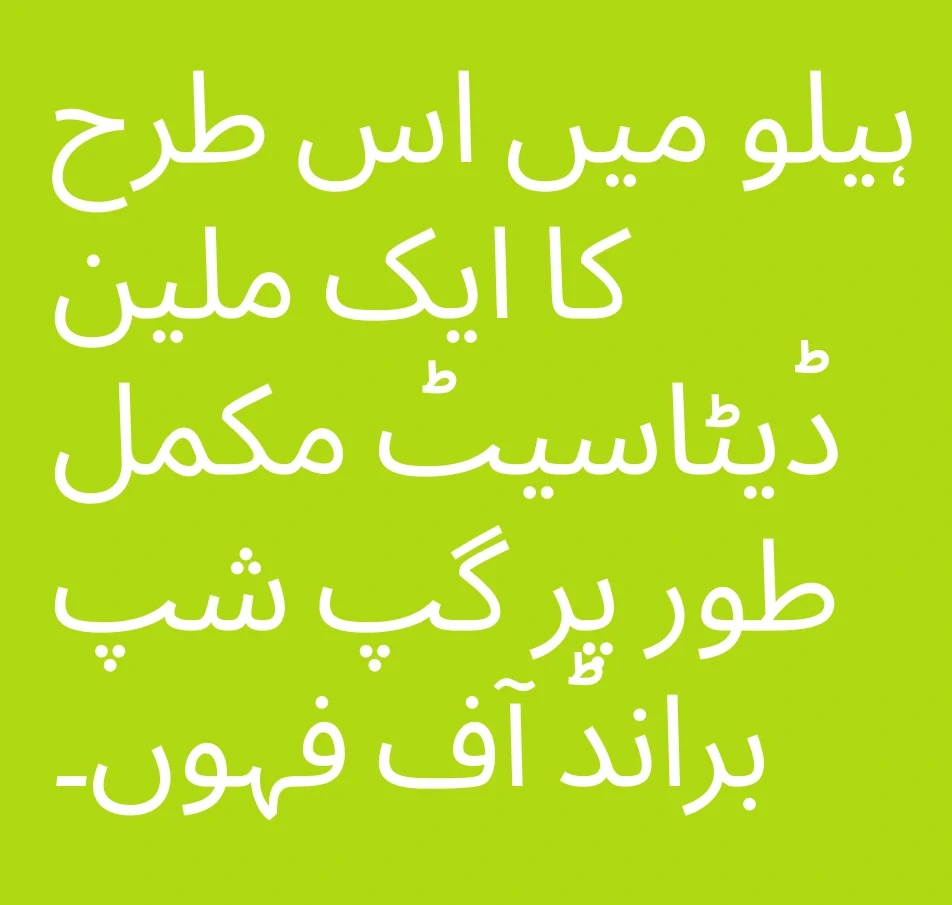
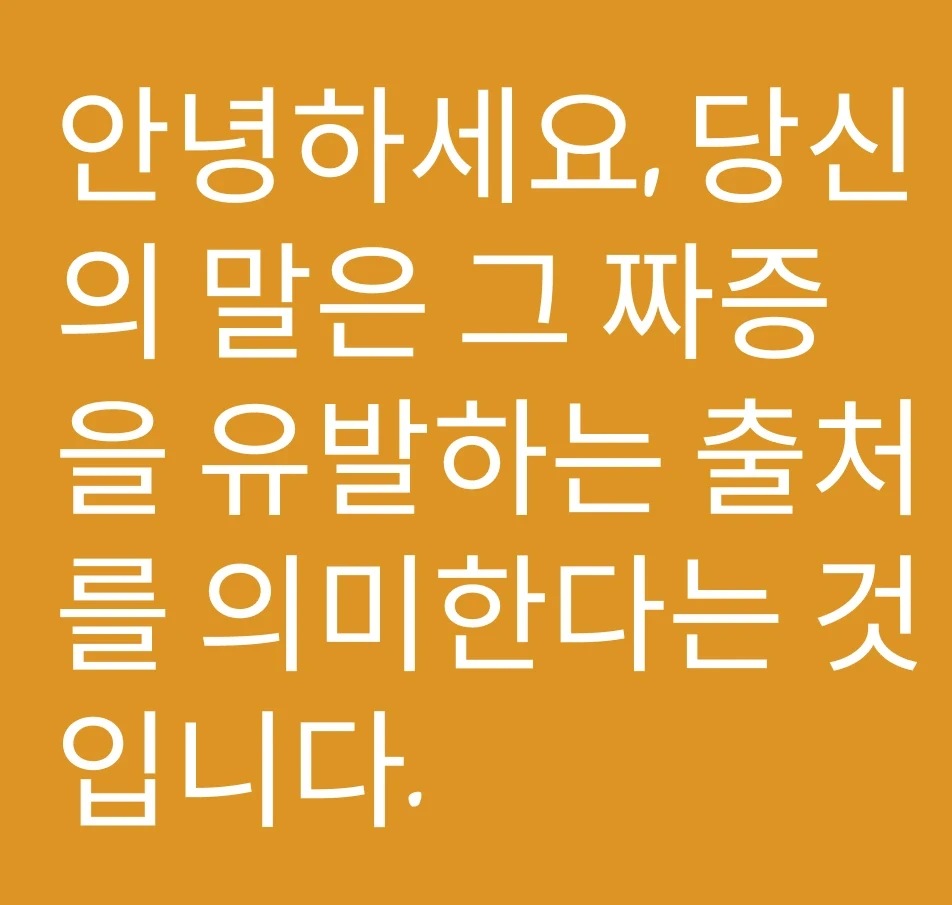
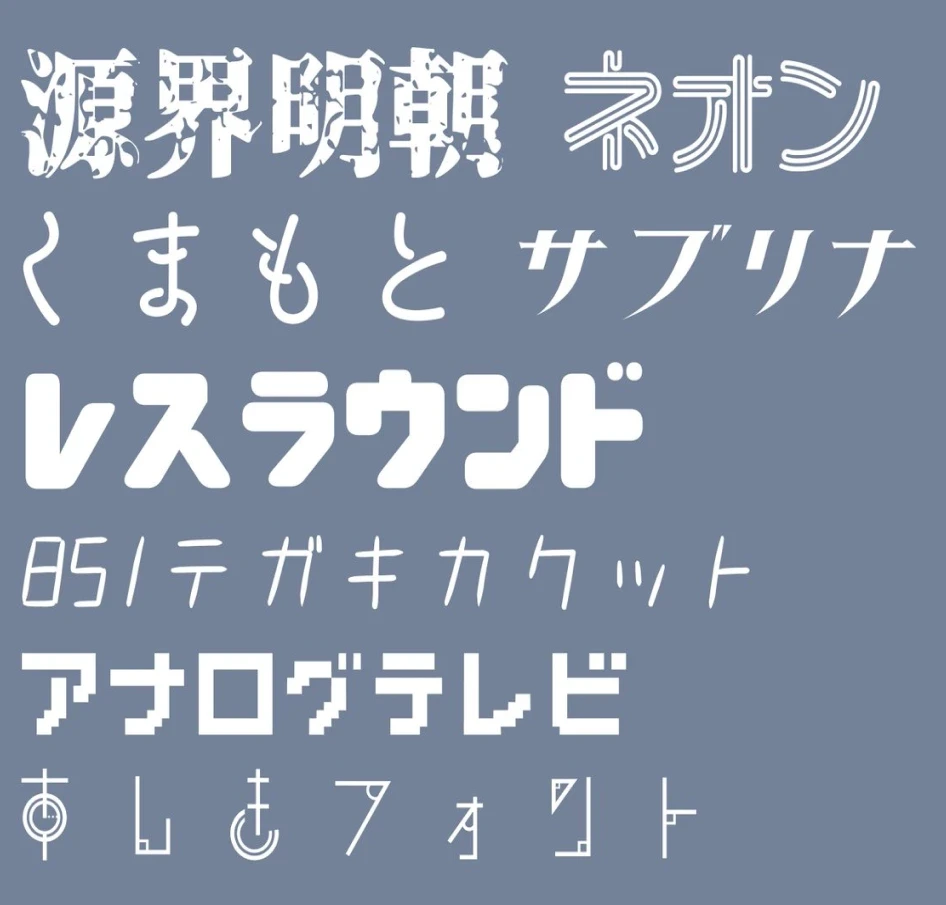
Sources
- Moreover, gain access to publicly available multilingual datasets for training and validation.
- Research Publications: Furthermore, keep updated through academic research and conferences in NLP and machine learning.
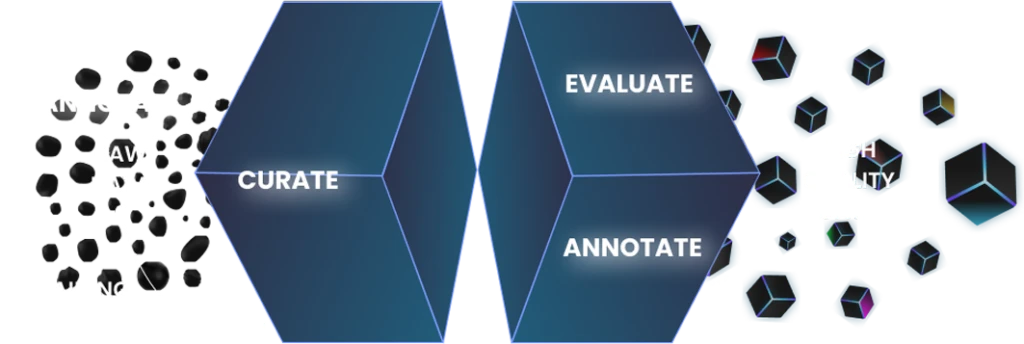
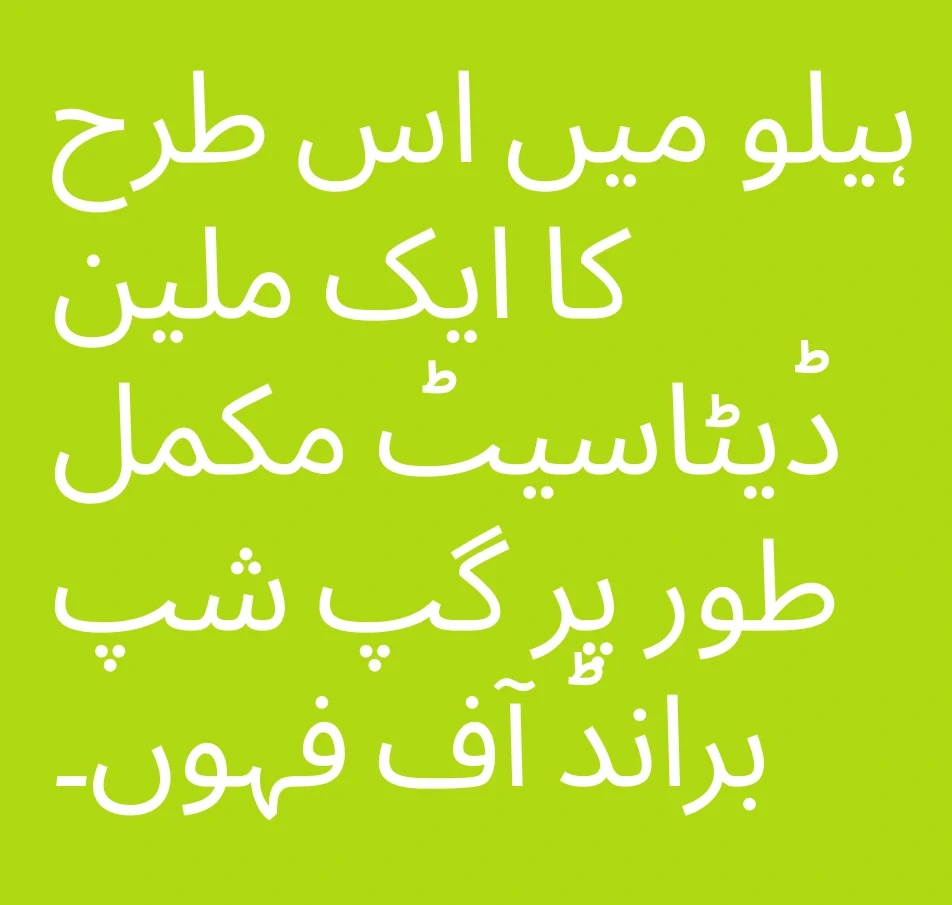
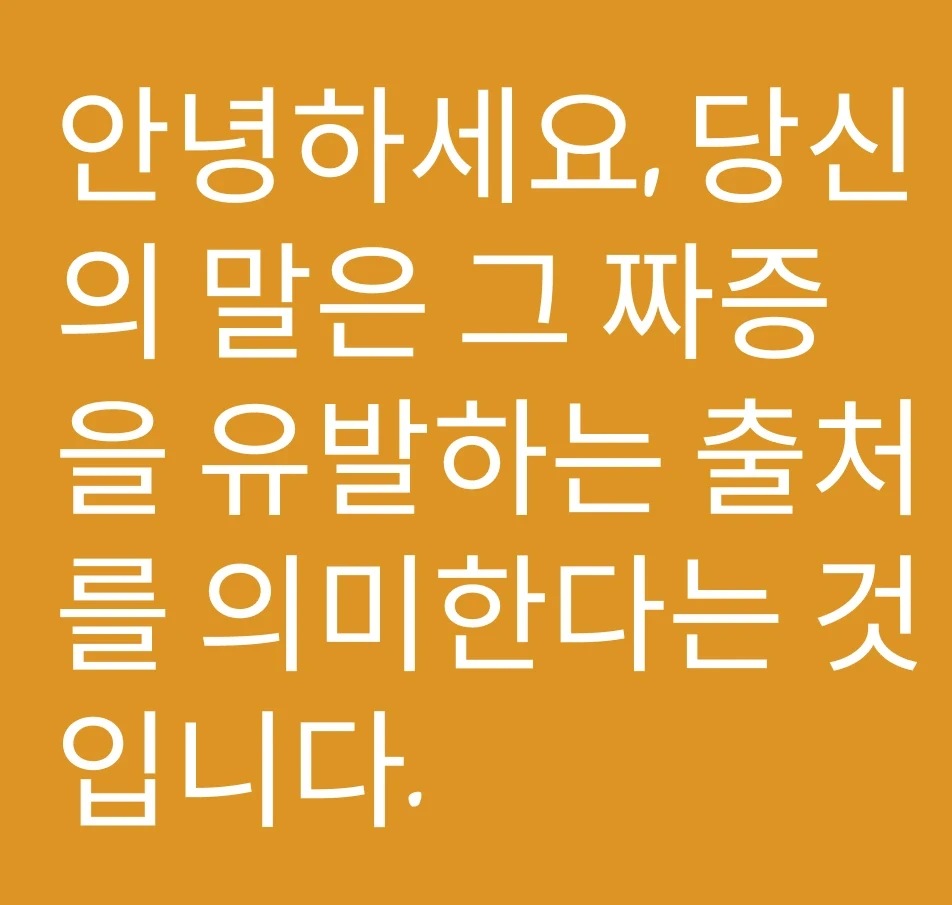
Data Collection Metrics
- Data Volume: Quantity of collected multilingual content.
- Data Diversity: Variety of languages and contexts in the dataset.
Annotation Process
Stages
- Data Collection: First, gather a diverse dataset containing text or audio samples in various languages.
- Data Preprocessing: Next, clean and standardize the collected data, including text normalization and audio cleaning.
- Model Training: Then, utilize machine learning algorithms to train the language identification model.
- Validation and Testing: After that, assess the model’s accuracy and performance on separate datasets to ensure robustness.
- Integration: Implement the trained model into the desired applications or systems.
- Ongoing Monitoring and Updates: Continuously monitor and update the model to adapt to evolving linguistic patterns and new languages.
Annotation Metrics
- Inter-Annotator Agreement (IAA): To ensure consistency in annotations, we measure the level of agreement among human annotators when labeling languages in the dataset. Additionally, we employ transition words to enhance the flow of the content and ensure clarity.
- Annotation Accuracy: To evaluate the precision and correctness of language annotations, we calculate the percentage of correctly labeled instances. Additionally, we incorporate transition words such as furthermore or moreover to enhance coherence.
- Annotation Efficiency: Evaluating the speed and cost-effectiveness of the annotation process ensures scalability for large datasets and projects. Firstly, by meticulously assessing the speed of annotation, we can gauge the efficiency of the process. Secondly, considering the cost-effectiveness of annotation allows us to allocate resources optimally. Furthermore, analyzing scalability ensures that the annotation process can seamlessly handle large datasets and projects without compromising quality.
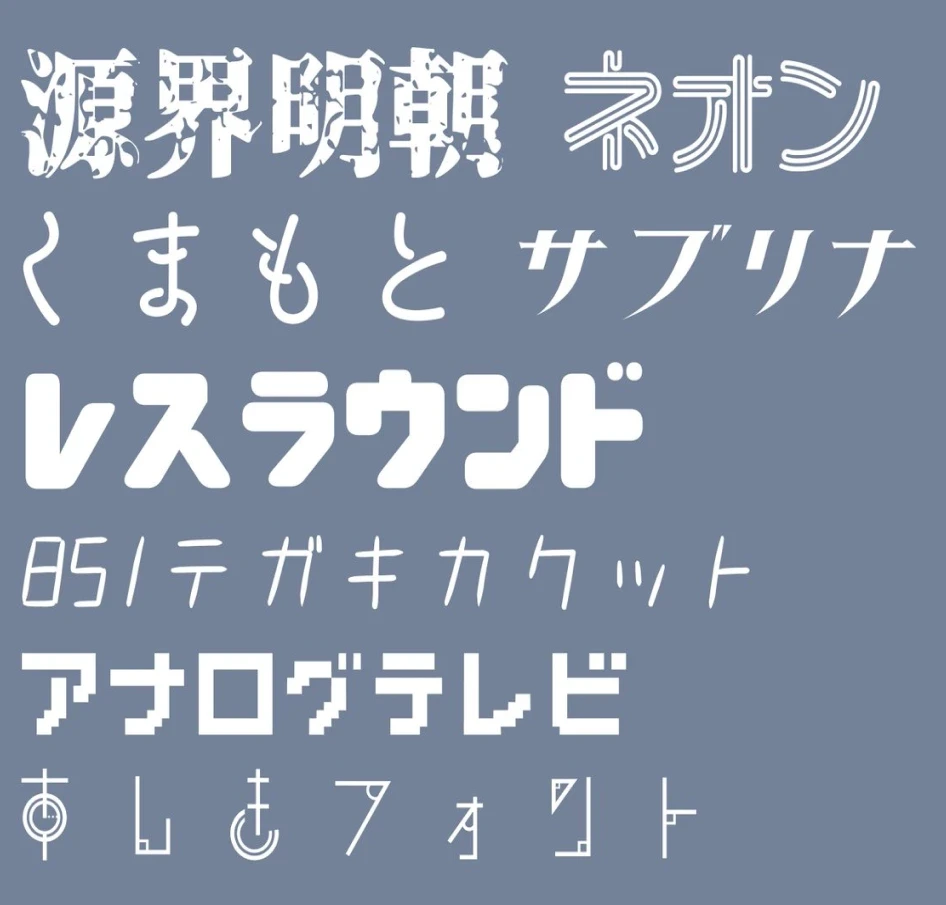
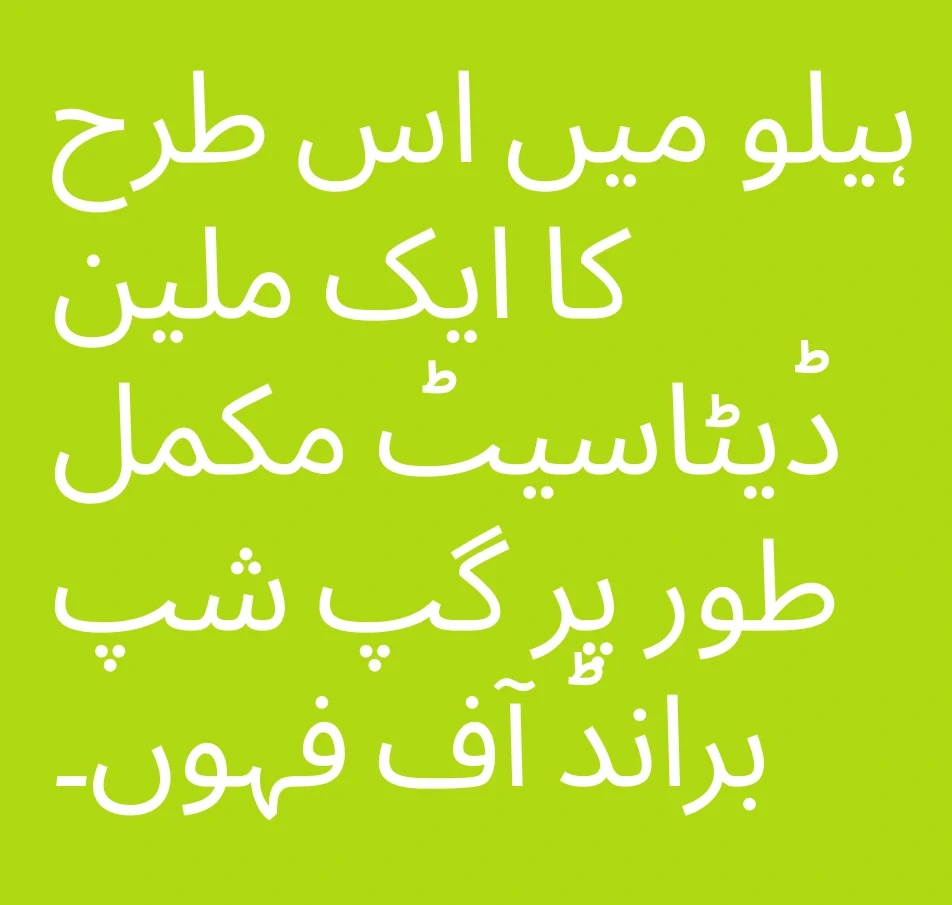
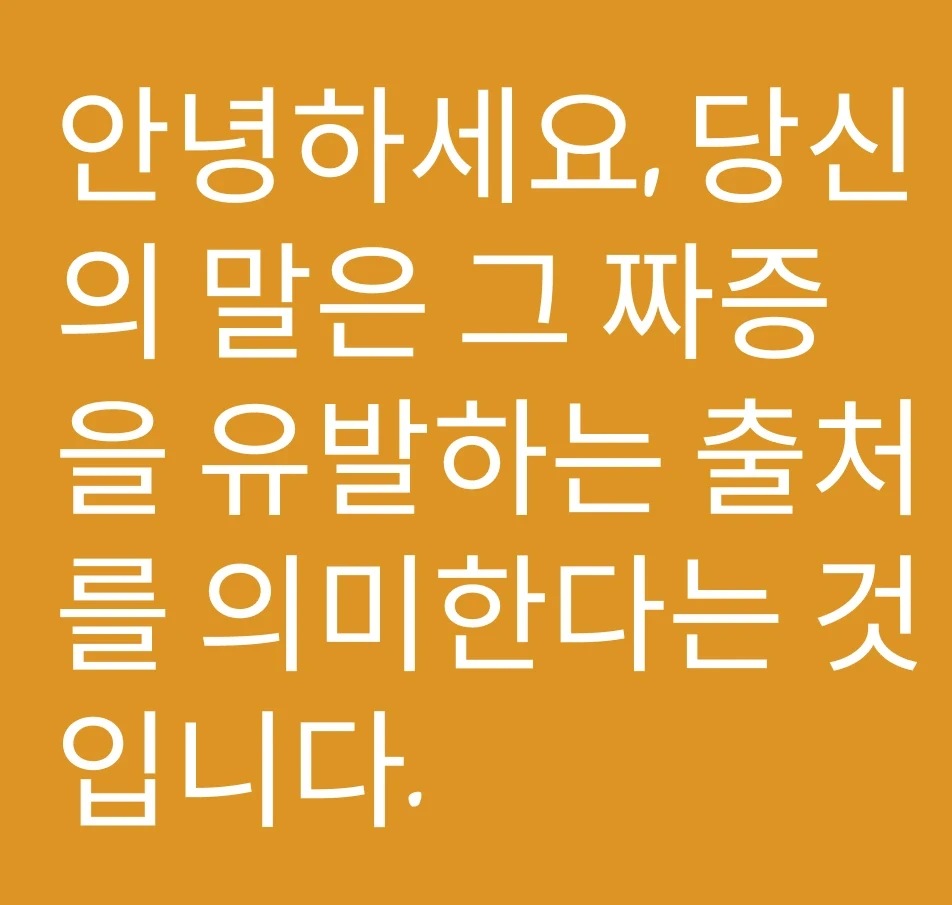
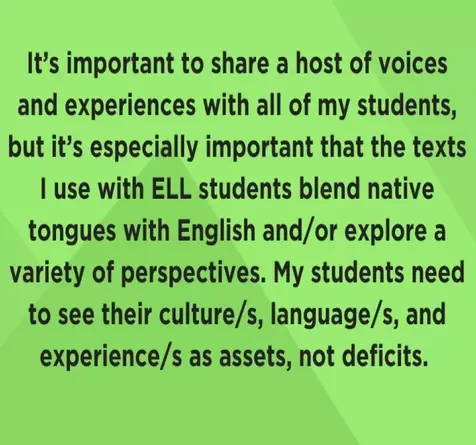
Quality Assurance
Stages
Data Privacy: Safeguard user data and privacy during language identification.
Bias Evaluation: Ensure fairness and accuracy across linguistic groups.
User Consent: Communicate data usage and obtain user consent transparently.
QA Metrics
- Accuracy: Measures language identification precision.
- Efficiency: Evaluates speed and resource usage.
Conclusion
Language identification for multilingual content plays a pivotal role in bridging linguistic barriers and enhancing user experience across a wide range of applications. By automatically recognizing and categorizing languages within textual or audio content, this technology enables efficient content organization, targeted communication, and effective language-specific services.

Quality Data Creation

Guaranteed TAT

ISO 9001:2015, ISO/IEC 27001:2013 Certified

HIPAA Compliance

GDPR Compliance

Compliance and Security
Let's Discuss your Data collection Requirement With Us
To get a detailed estimation of requirements please reach us.