Machine Part Defects Segmentation Dataset
Home » Case Study » Machine Part Defects Segmentation Dataset
Project Overview:
Objective
As a leading data collection and annotation firm, we recently completed a significant project aimed at enhancing automated quality control and maintenance in manufacturing. Our objective was to construct a dataset that segments defects in machine parts within images, aiding in the development of cutting-edge machine learning models for industrial applications.
Scope
Our team successfully built an extensive image repository, which includes a variety of machine parts. Each image features meticulously annotated segmentation, highlighting defects and anomalies, vital for machine learning applications in quality control, maintenance, and manufacturing processes.
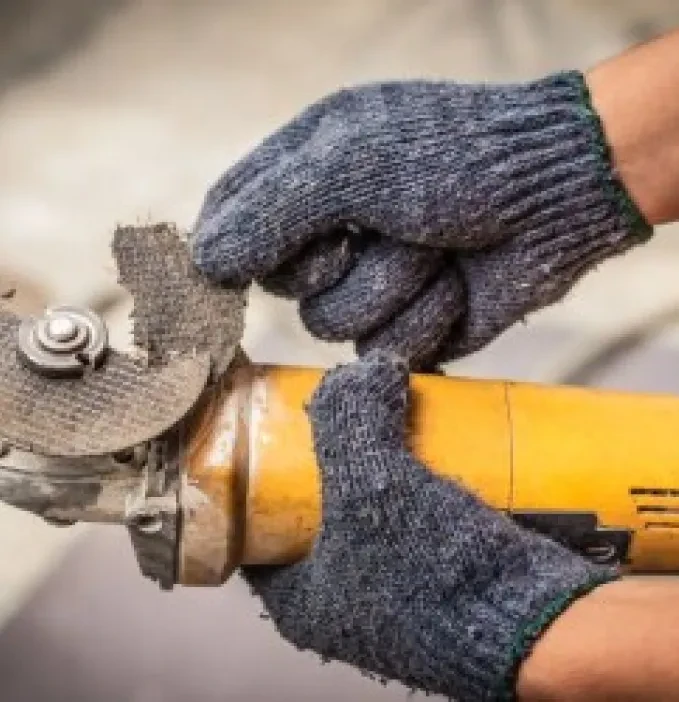
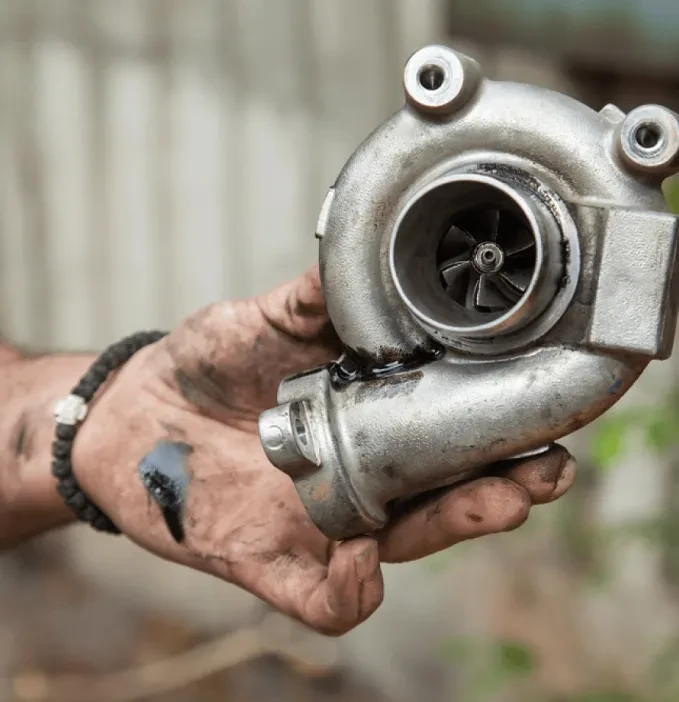
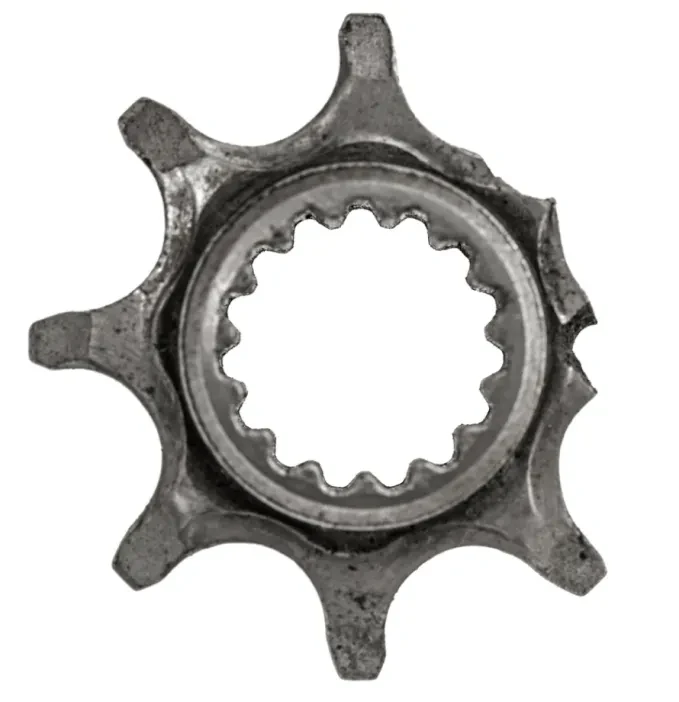
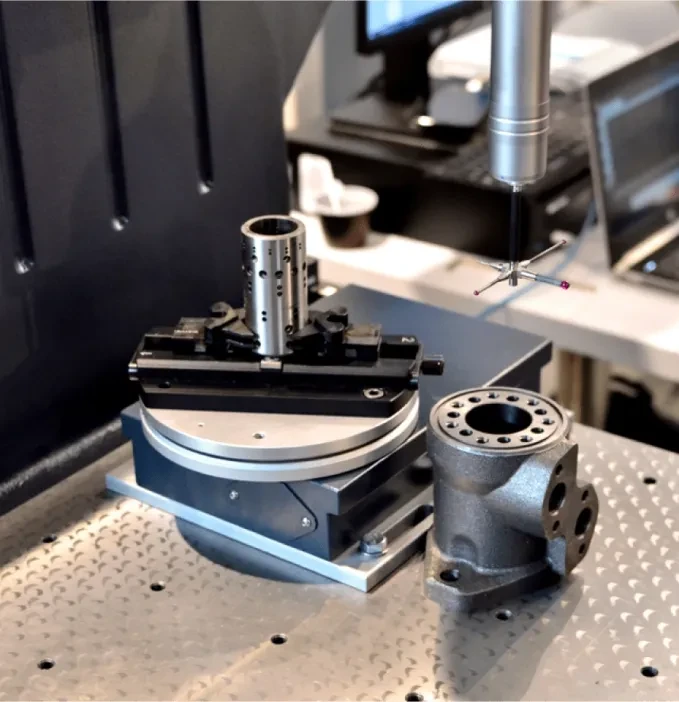
Sources
- Manufacturing Facilities: Collaborate with industrial manufacturing plants to obtain images captured during quality control inspections.
- Quality Control Records: Extract images from historical quality control records, which often contain documentation of defective parts.
- Public Submissions: Develop a platform for individuals to contribute images of machine parts with known defects, promoting diversity in defect types and machine parts.
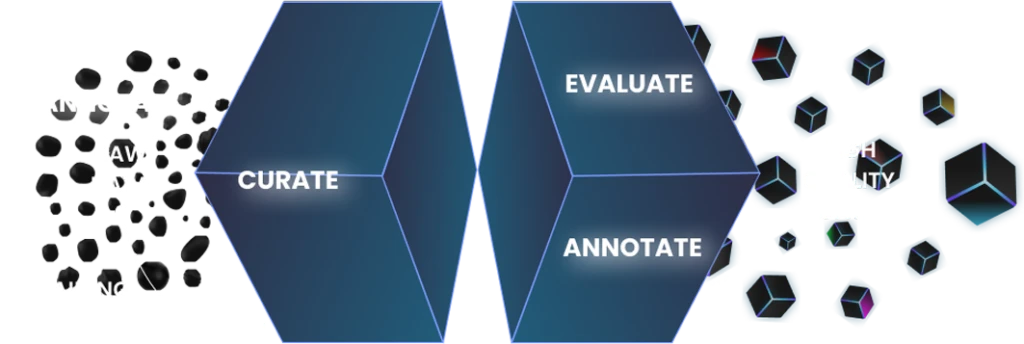
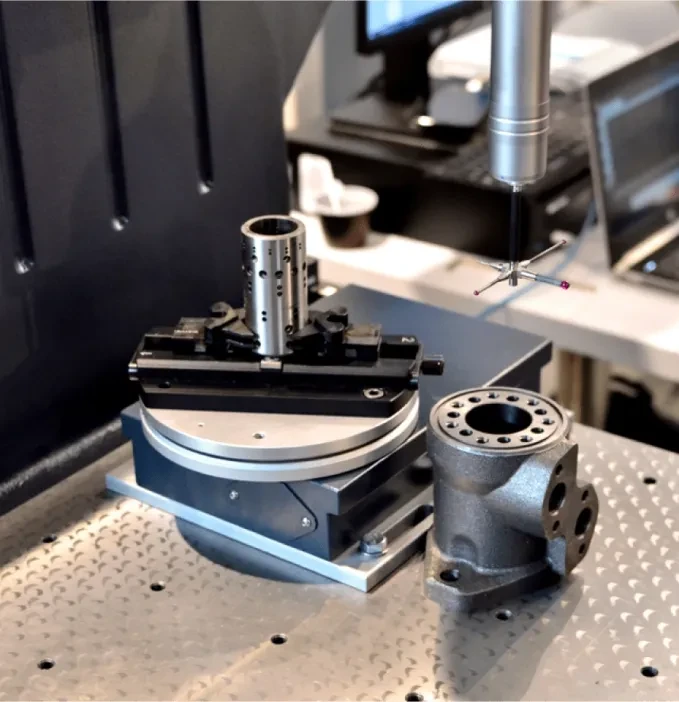
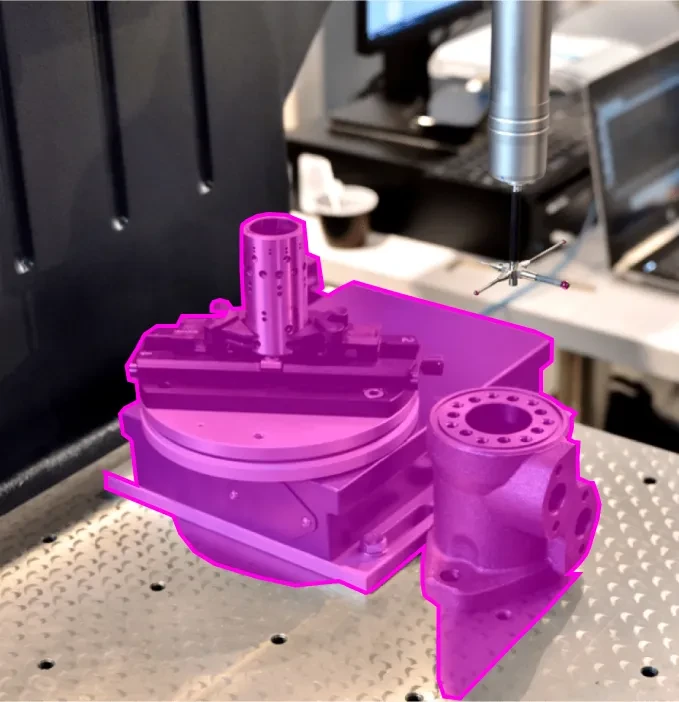
Data Collection Metrics
- Total Images Collected and Annotated: 175,000
- Manufacturing Facility Contributions: 95,000
- Quality Control Records Extracts: 45,000
- Public Submissions: 35,000
Annotation Process
Stages
- Defect Segmentation: We annotated each image to precisely define the defect boundaries.
- Machine Part Identification: Each image was labeled with detailed information about the machine part.
- Defect Type Categorization: We categorized the defects into various types, such as cracks and corrosion.
- Severity Level Assignment: A severity level was tagged to each defect, indicating its impact on machine performance.
Annotation Metrics
- Images with Defect Segmentations: 175,000
- Machine Part Identifications: 175,000
- Defect Type Labels: 175,000
- Severity Level Tags: 175,000
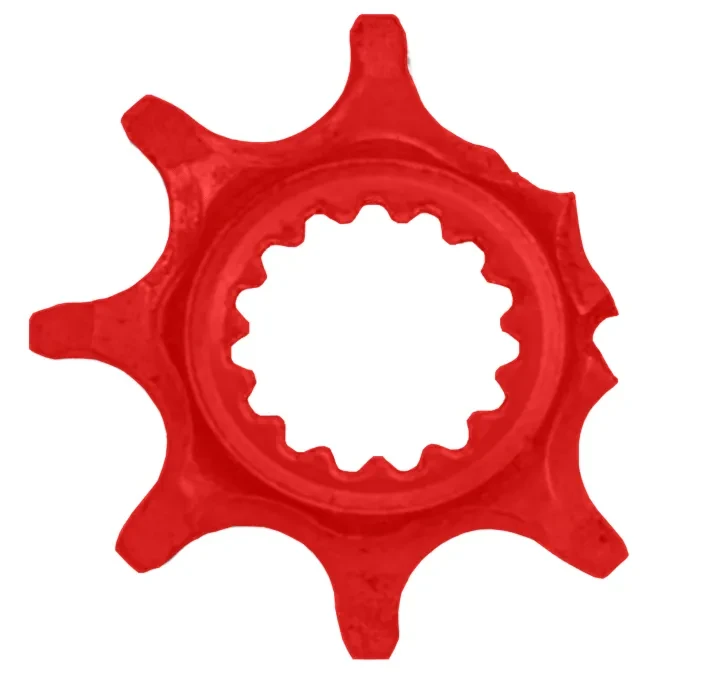
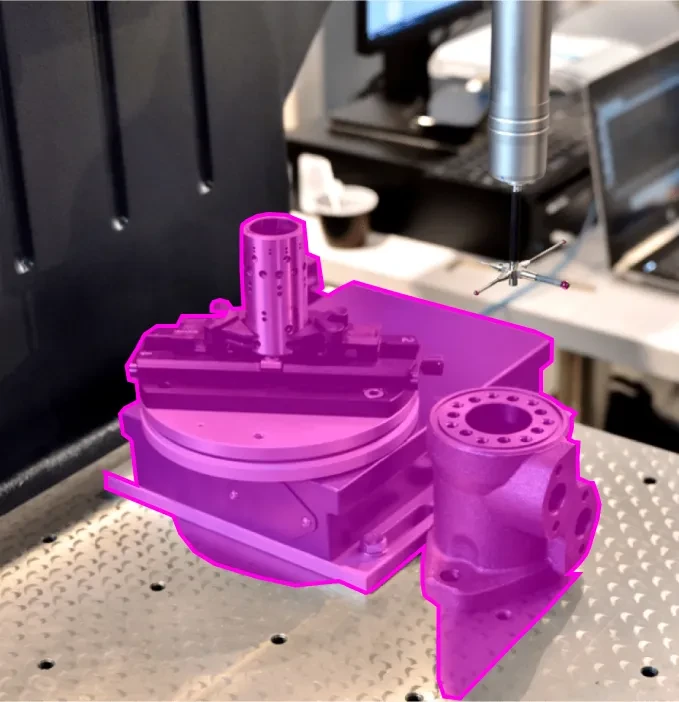
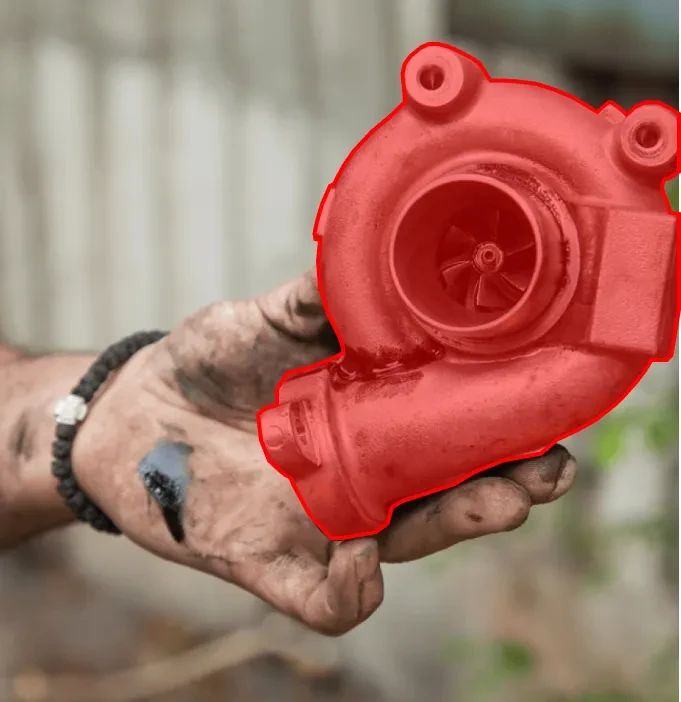
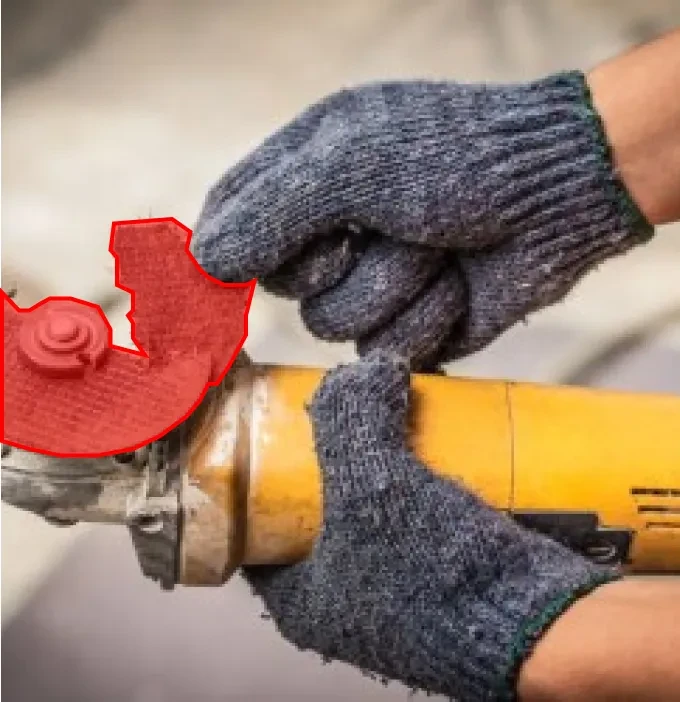
Quality Assurance
Stages
Segmentation Accuracy Checks:Â We used advanced computer vision algorithms, supported by domain experts, to ensure segmentation accuracy.
Metadata Validation:Â Quality control specialists and industrial engineers verified the accuracy of machine part identification and defect categorization.
Privacy Safeguards:Â We implemented strict controls to protect the privacy of publicly contributed images, ensuring no sensitive or proprietary information was included.
QA Metrics
- Segmentation Validation Cases: 17,500
- Metadata Authentication Reviews: 26,250
- Privacy Audits: 35,000
Conclusion
Through the Machine Part Defects Segmentation Dataset Initiative, our company has established a vital resource for the industrial sector. Our comprehensive and accurately annotated dataset is instrumental in developing machine-learning models that streamline defect detection and enhance manufacturing efficiency.

Quality Data Creation

Guaranteed TAT

ISO 9001:2015, ISO/IEC 27001:2013 Certified

HIPAA Compliance

GDPR Compliance

Compliance and Security
Let's Discuss your Data collection Requirement With Us
To get a detailed estimation of requirements please reach us.