Clothing Segmentation and Fabrics Classification Dataset
Home » Case Study » Clothing Segmentation and Fabrics Classification Dataset
Project Overview:
Objective
We embarked on creating a comprehensive dataset to assist in the segmentation of various clothing items and their classification based on fabric types. Our primary goal was to foster innovation in fashion technology, particularly in the areas of Clothing Segmentation and Fabrics Classification, including retail analytics and virtual fitting rooms.
Scope
Our team diligently compiled a vast array of images, showcasing a wide range of clothing items across different body types and poses. Moreover, each image was meticulously segmented and classified by fabric type, ensuring a rich and diverse dataset.
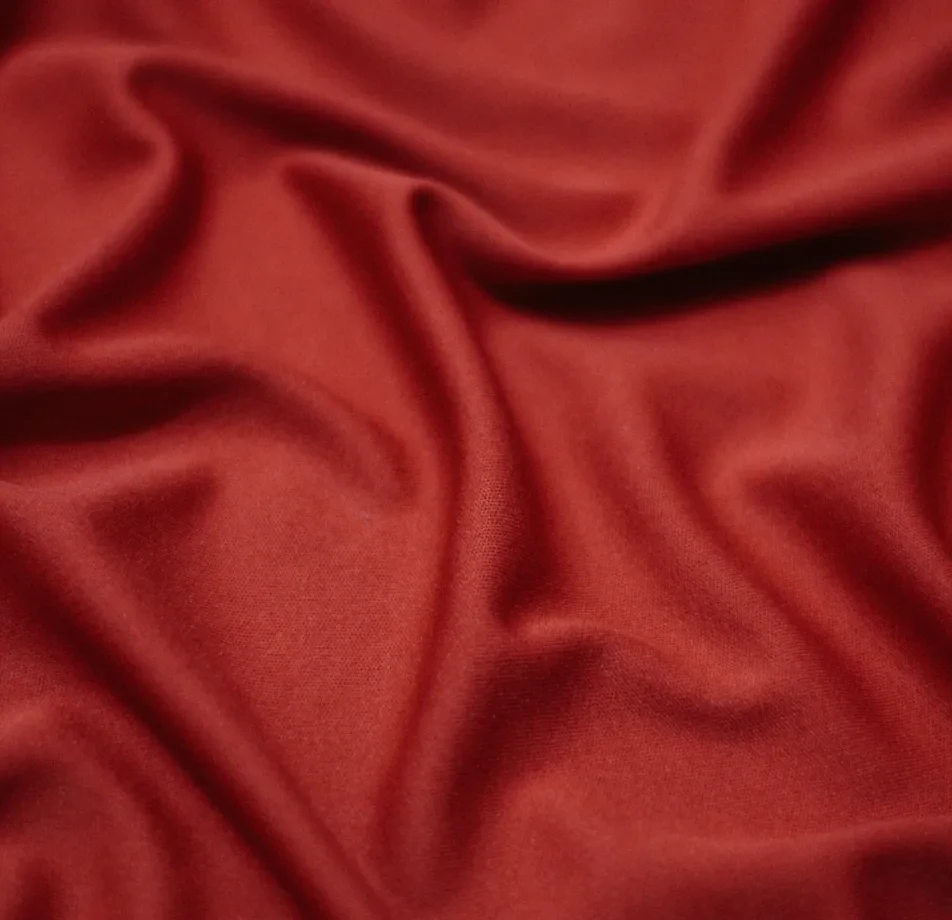
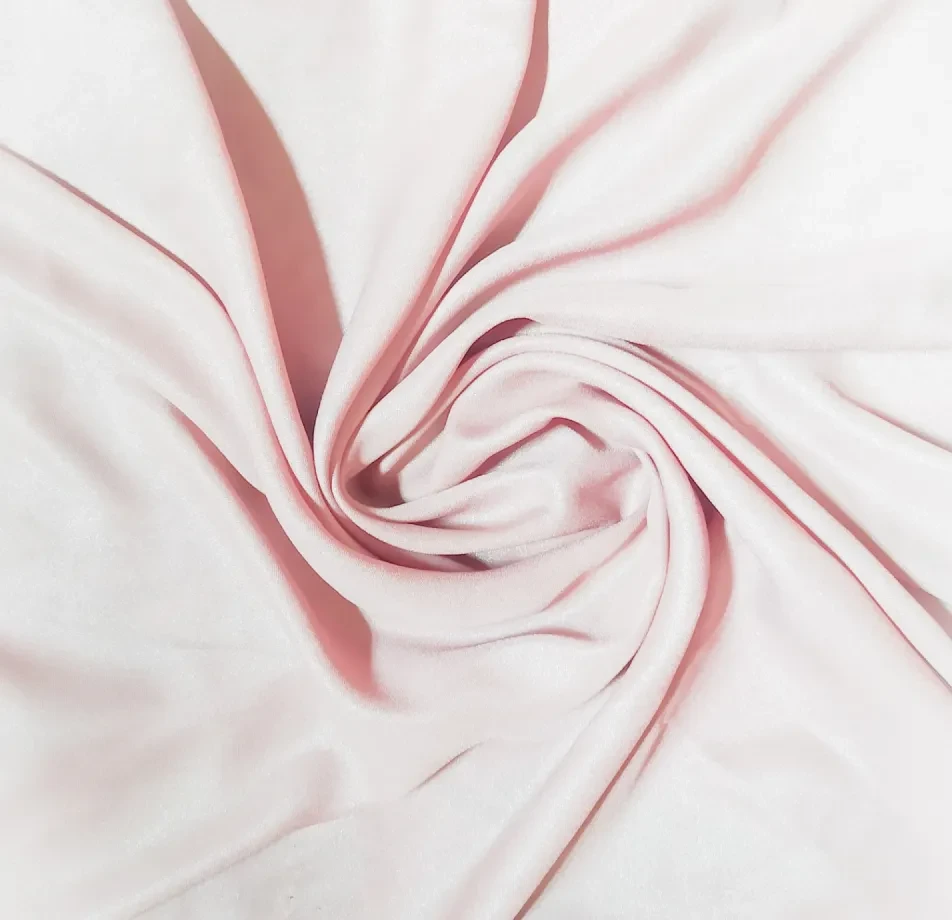
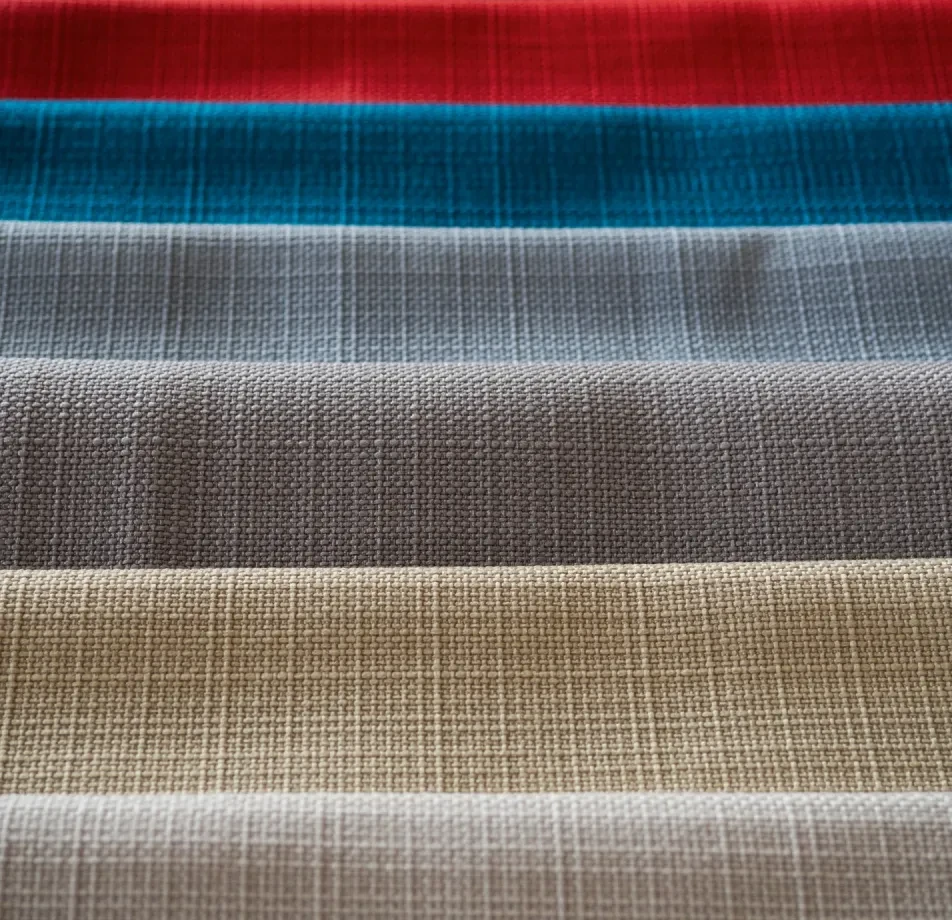
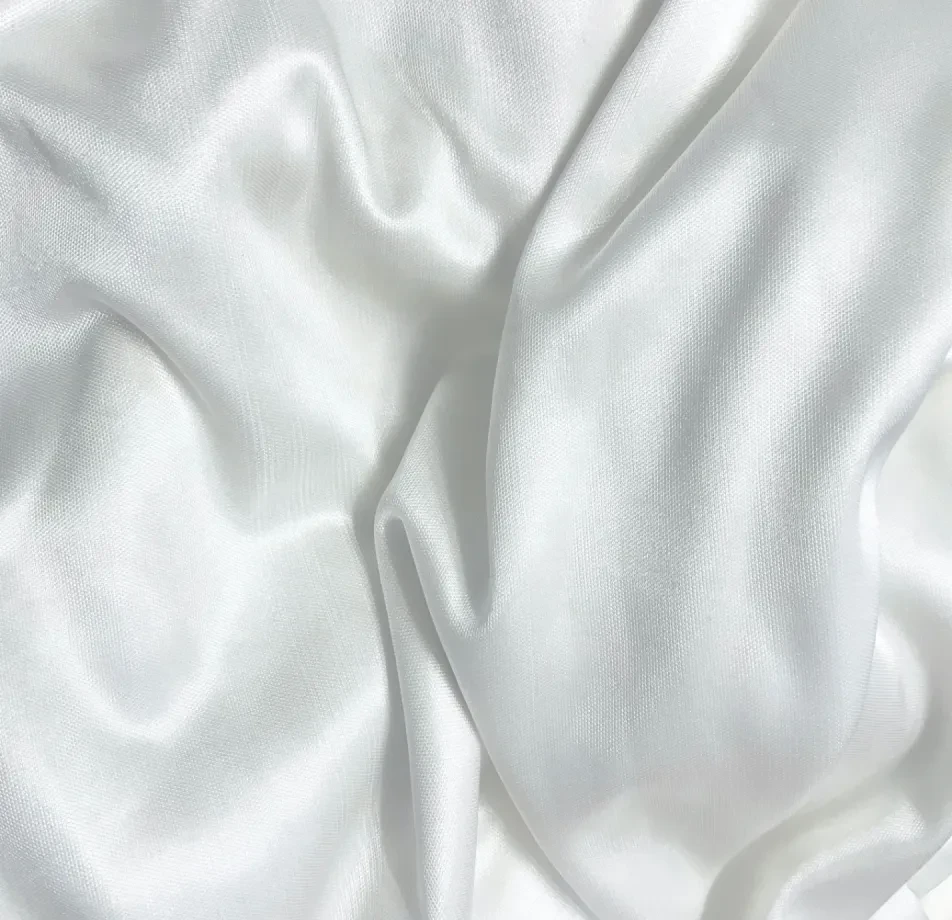
Sources
- Established partnerships with prominent clothing brands and online fashion retailers.
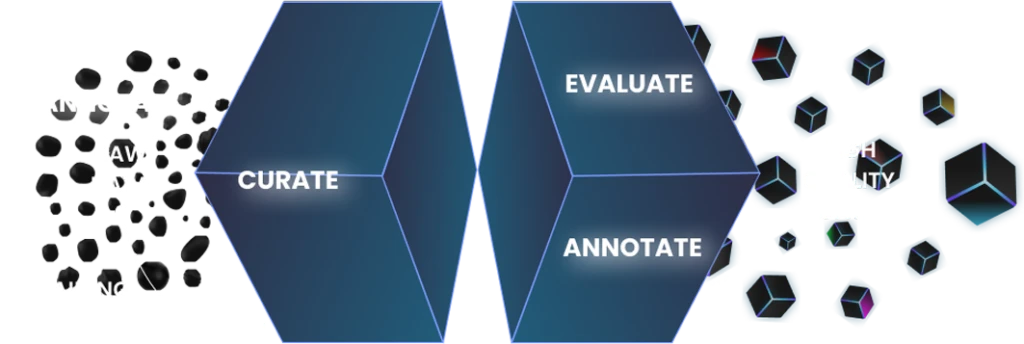
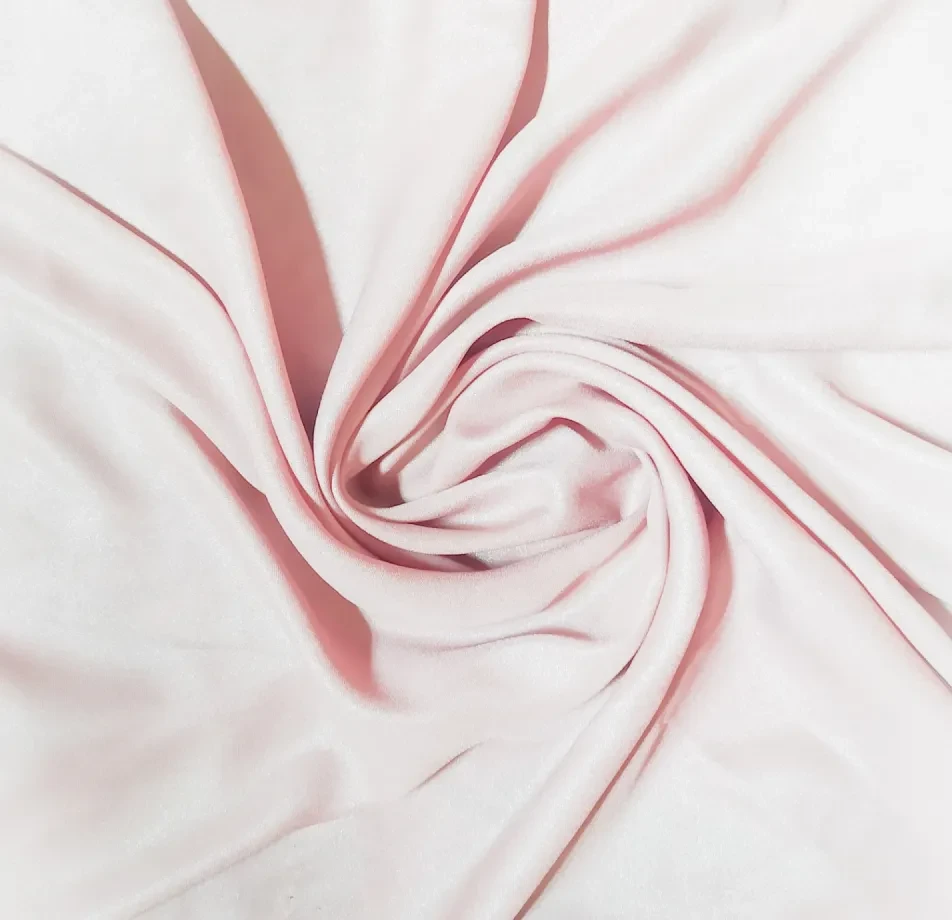
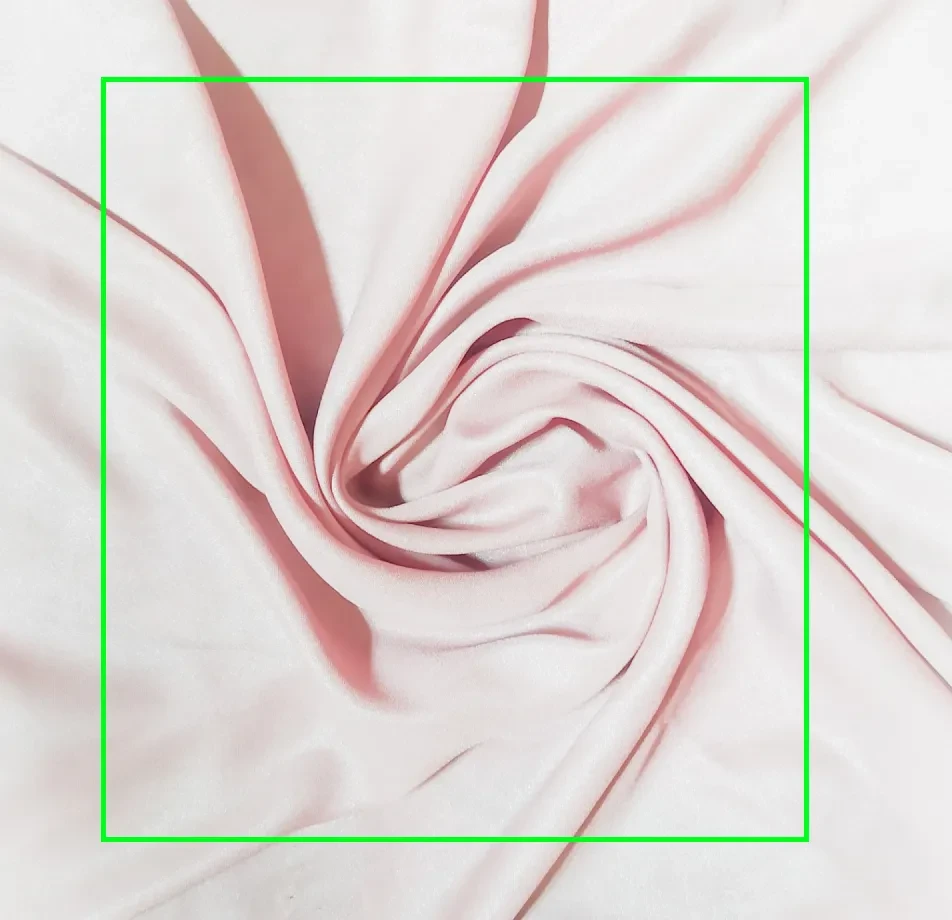
Data Collection Metrics
- Total Images Compiled: 45,000
- Categorized as follows:
- Tops & Shirts: 13,500
- Dresses: 11,000
- Pants & Skirts: 10,500
- Traditional/Ethnic Wear: 10,000
Annotation Process
Stages
- Image Pre-processing: To begin with, we standardized the images for resolution, lighting, and orientation.
- Pattern Annotation: Next, each clothing item was meticulously annotated with details like “stripes,” “floral,” and “geometric.”
- Validation: Finally, fashion industry experts verified the accuracy of these pattern annotations.
Annotation Metrics
- Total Pattern Annotations: 45,000
- Average Annotation Time per Image: 3 minutes
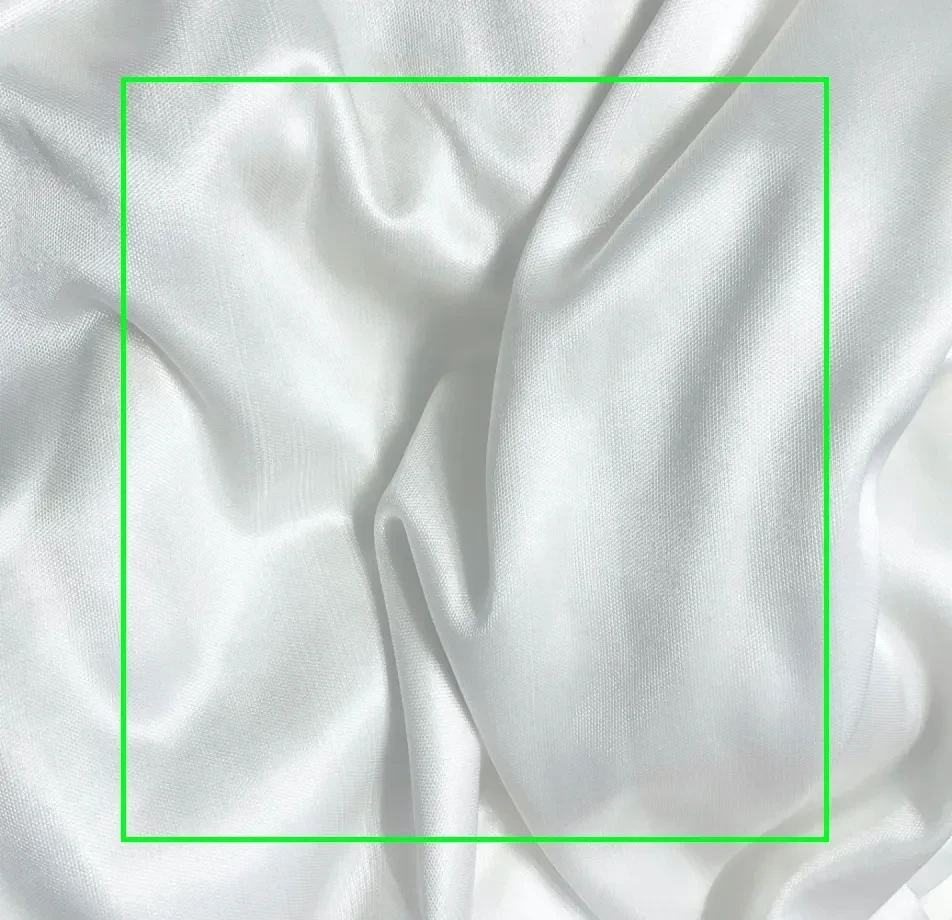
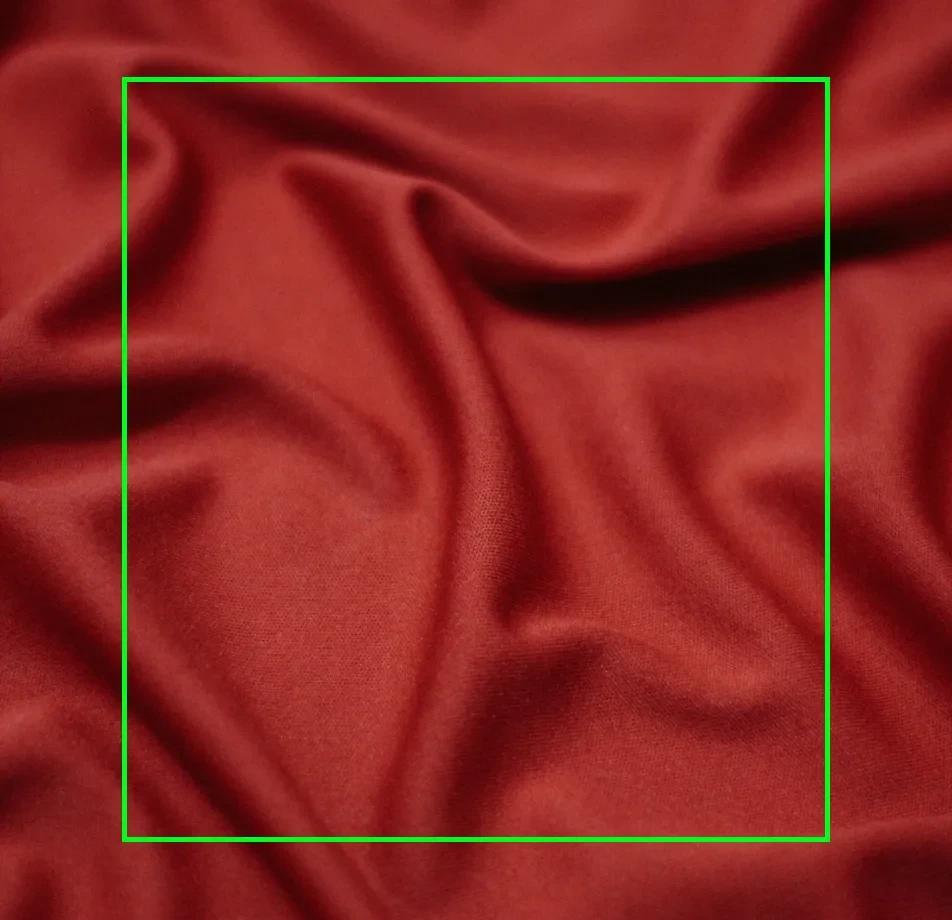
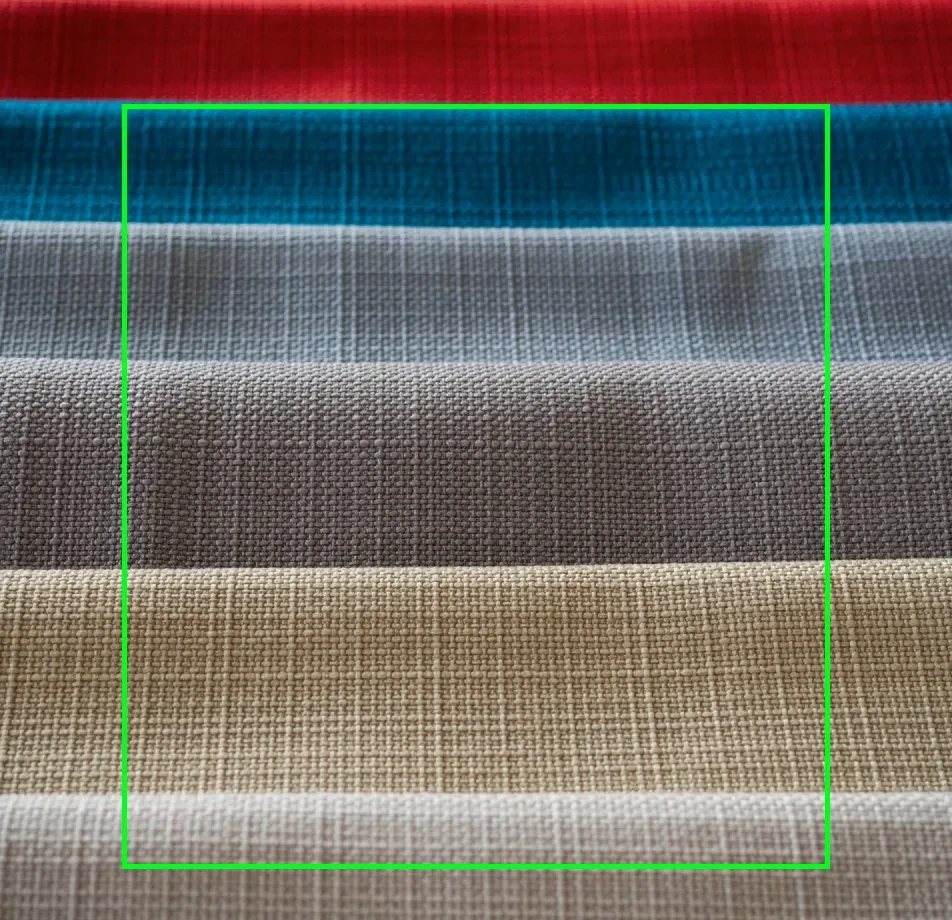
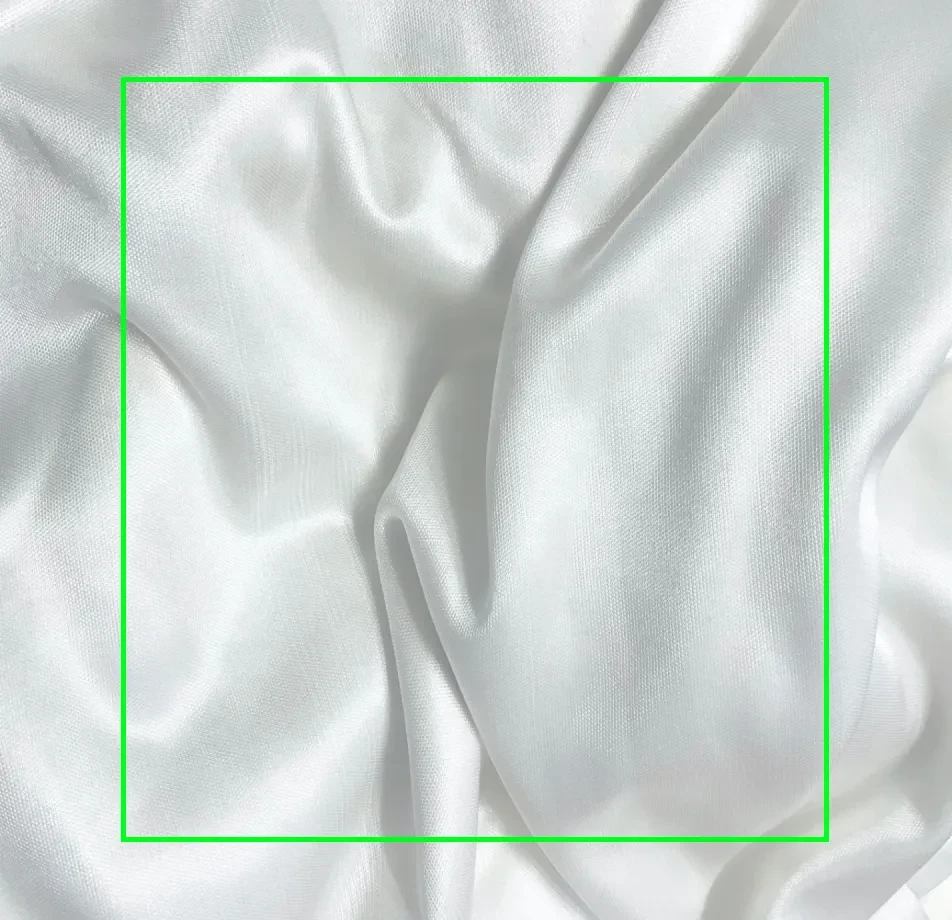
Quality Assurance
Stages
- Automated Verification: We used early-stage pattern classification models to cross-verify results with human annotations. Additionally, we employed these models to ensure consistency and accuracy.
- Peer Review: To enhance reliability, selected images underwent a secondary evaluation by different experts. This step was crucial to validate the initial findings.
- Inter-annotator Agreement: For complex patterns, multiple annotators reviewed the images to achieve consensus. Consequently, this collaborative effort improved the overall annotation quality.
QA Metrics
- Patterns Validated through Automated Checks: 22,500 (50% of total images)
- Peer-reviewed Annotations: 13,500 (30% of total images)
- Inconsistencies Detected and Rectified: 675 (1.5% of total images)
Conclusion
The Clothing Segmentation and Fabrics Classification Dataset is poised to revolutionize the nexus between AI and the fashion industry. By providing an in-depth understanding of clothing items and their fabrics, it lays the groundwork for advanced virtual fitting experiences, intelligent inventory management, and nuanced consumer insights in fashion retail.

Quality Data Creation

Guaranteed TAT

ISO 9001:2015, ISO/IEC 27001:2013 Certified

HIPAA Compliance

GDPR Compliance

Compliance and Security
Let's Discuss your Data collection Requirement With Us
To get a detailed estimation of requirements please reach us.