Call Center Speech Dataset for Retail & E-commerce
Home » Case Study » Call Center Speech Dataset for Retail & E-commerce
Project Overview:
Objective
The objective is to leverage the Call Center Speech Dataset to significantly enhance the performance of NLU models, thereby enabling more accurate and efficient customer support interactions. This involves not only improving the recognition of various accents, dialects, and languages but also accurately identifying customer intents and sentiments in diverse retail and e-commerce scenarios.
Scope
The dataset comprises a vast collection of recorded call center interactions and simulated dialogues, covering multiple languages and regional accents. Consequently, this extensive scope ensures that the dataset can comprehensively train and test NLU models, accurately reflecting the variety of real-world customer service scenarios.
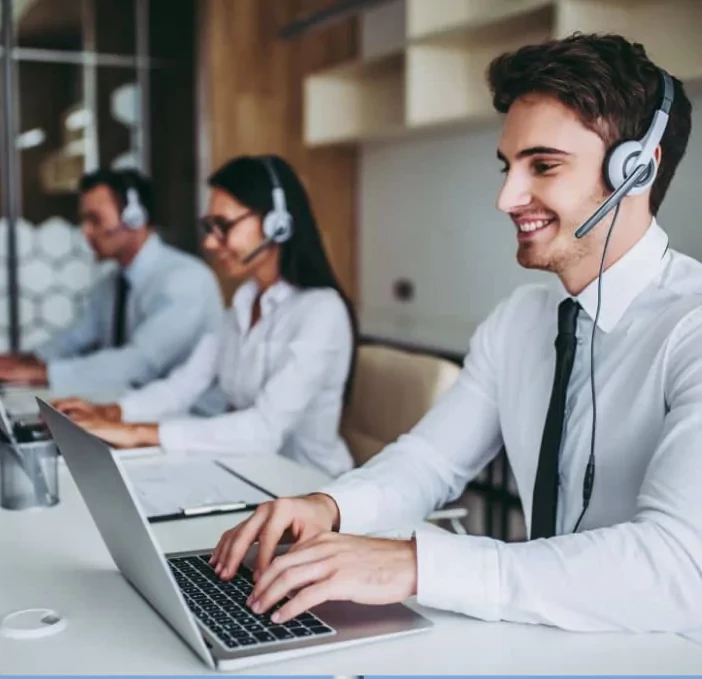
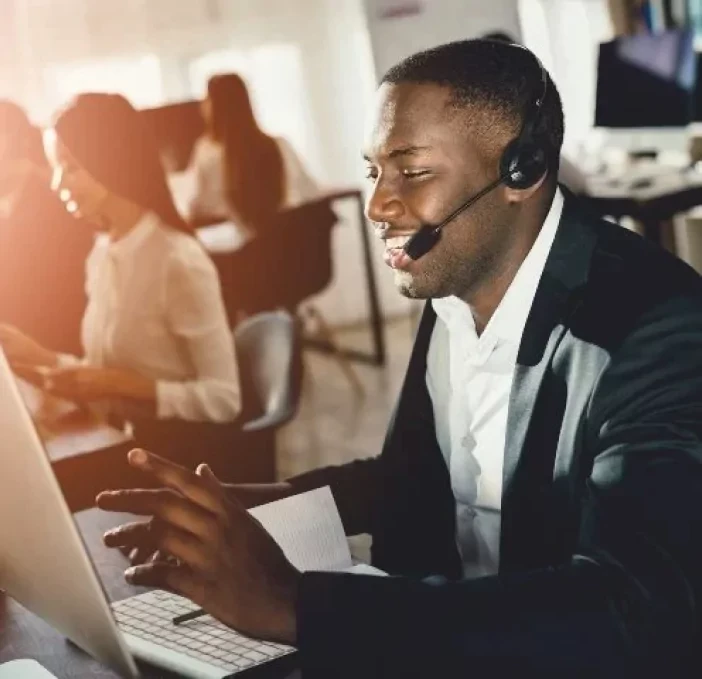
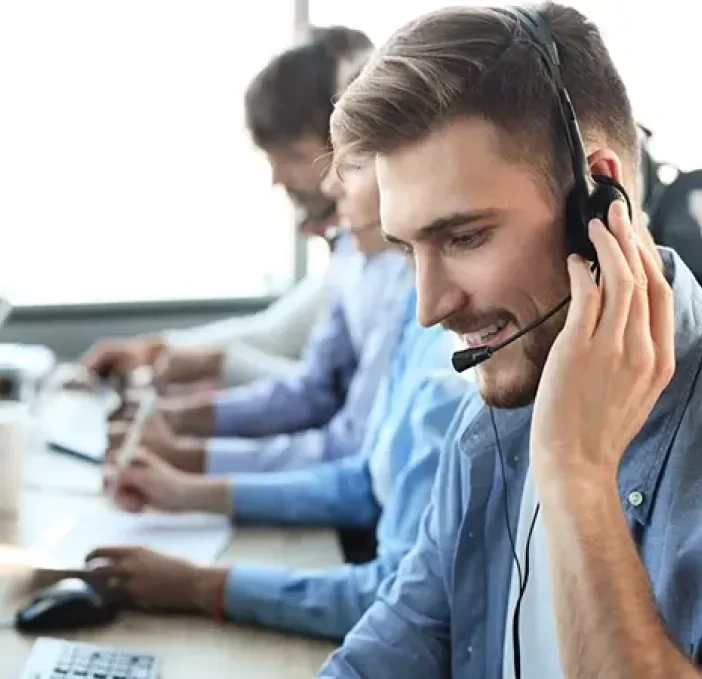
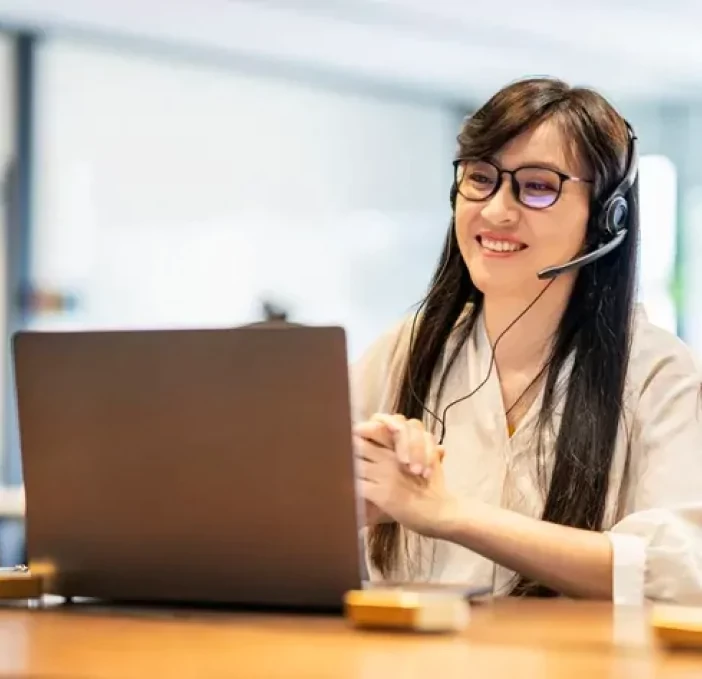
Sources
- Recorded call center interactions from diverse retail and e-commerce platforms are invaluable.
- Additionally, simulated dialogues created by professional actors cover a broader range of scenarios.
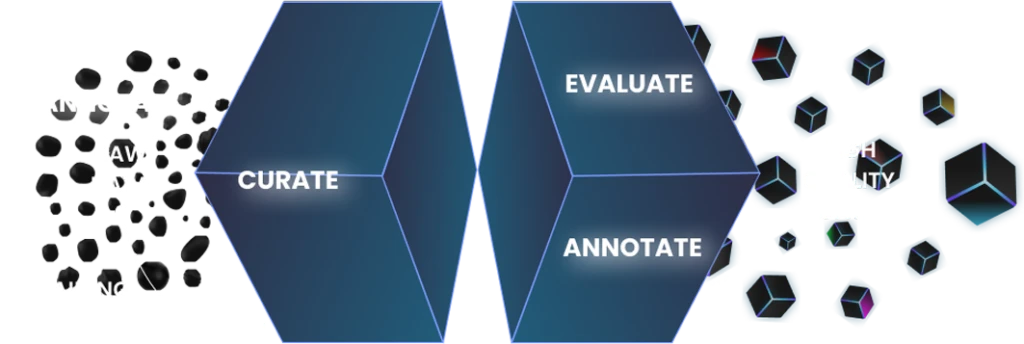
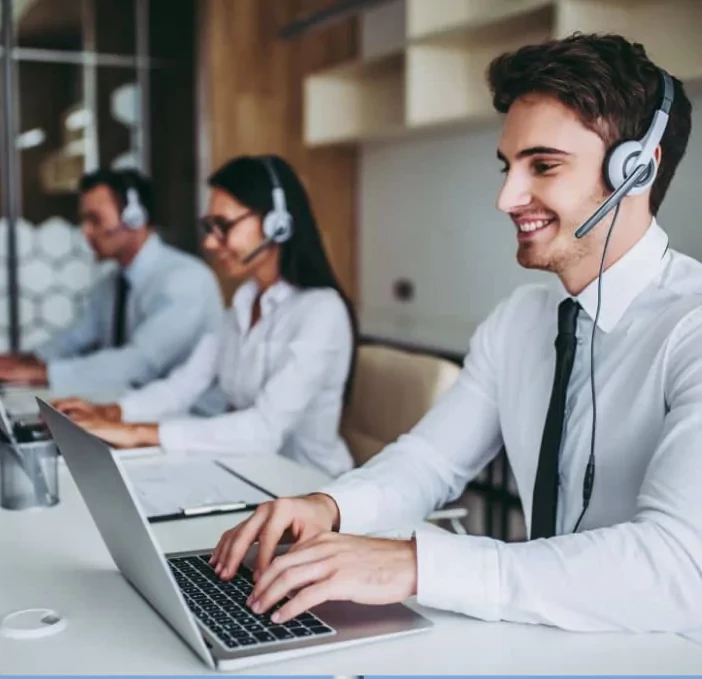
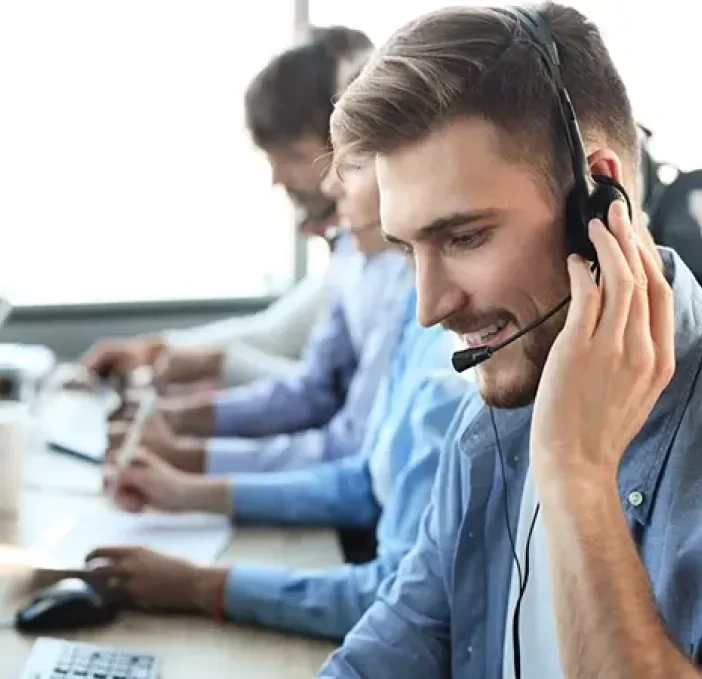
Data Collection Metrics
- Total Conversations Recorded: 15,000
- Duration of Recordings: 1,200 hours
- Languages Covered: English, Mandarin, Spanish, and French
- Dialects and Accents: 30+ regional variants
Annotation Process
Stages
- Transcription: This involves converting speech to text.
- Categorization: This step focuses on classifying conversations based on topics like complaints, inquiries, and more.
- Sentiment Analysis: Here, we tag the emotional tone of the conversation – whether it is positive, negative, or neutral.
- Intent Recognition: This process is about identifying the customer’s intent in each interaction segment.
Annotation Metrics
- Total Annotations: 450,000
- Average Annotations per Conversation: 30
- Unique Annotation Tags: 200+
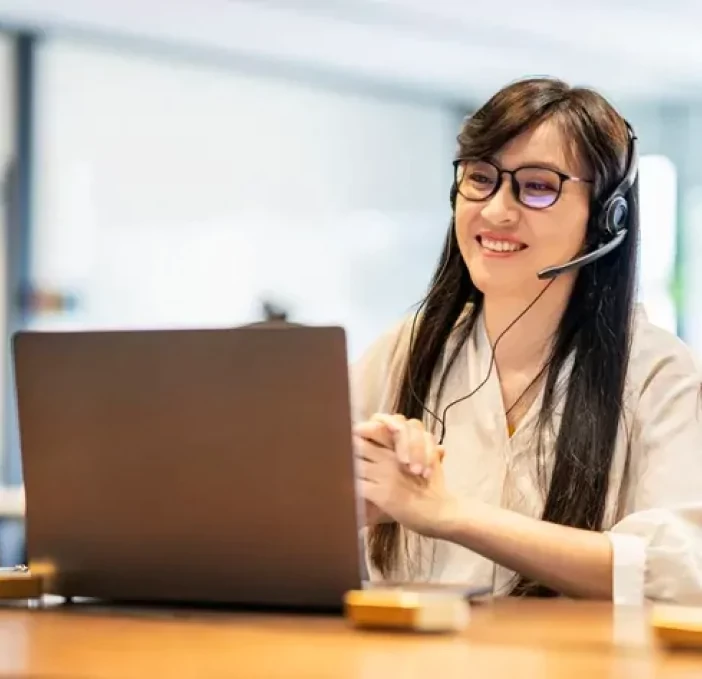
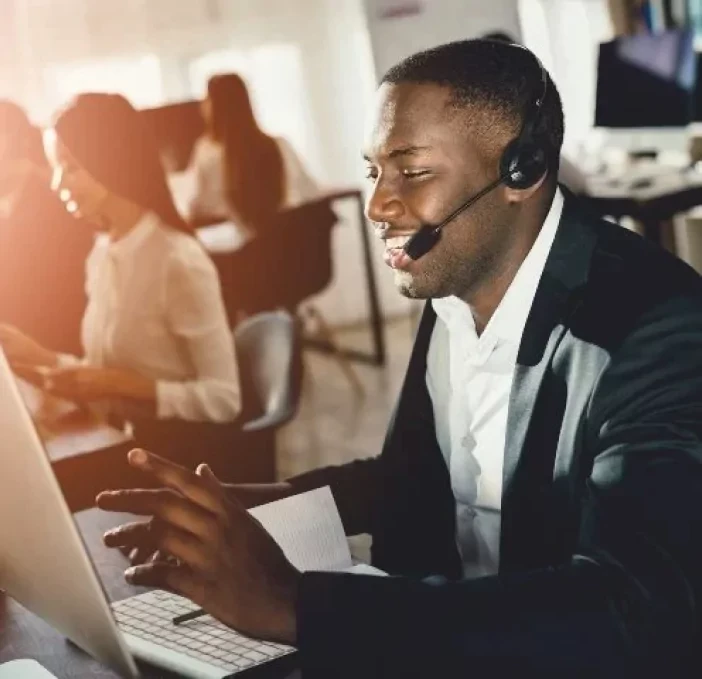
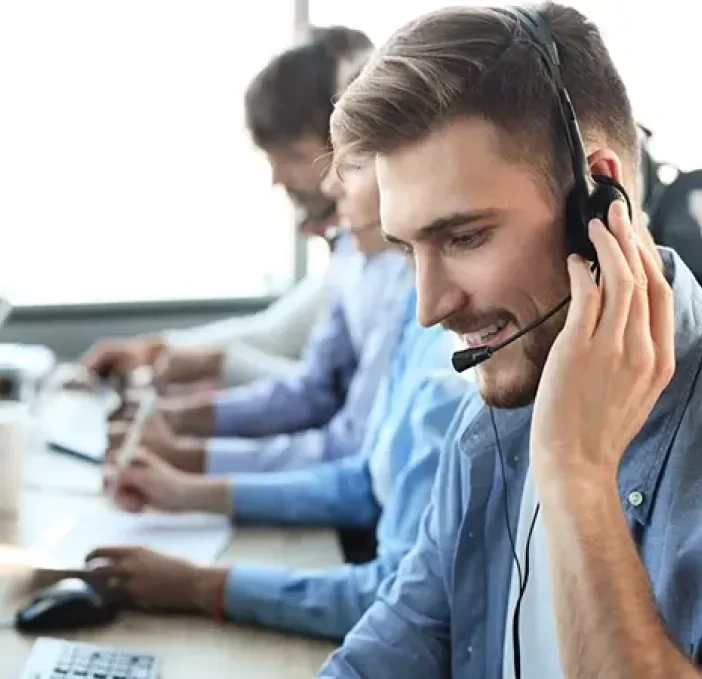
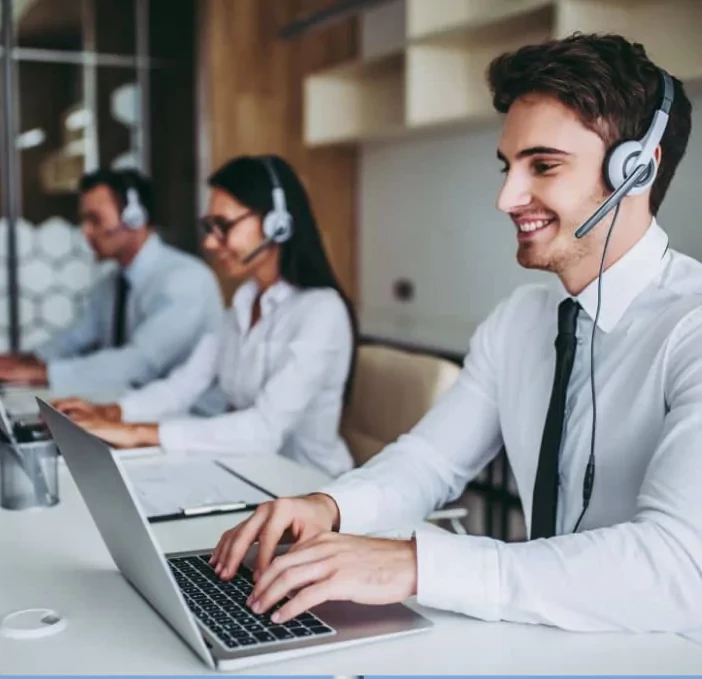
Quality Assurance
Stages
- Model Performance Evaluation: Evaluation: To ensure robustness and reliability, it is essential to evaluate models using metrics such as accuracy, precision, recall, and F1-score. These metrics provide a comprehensive view of the model’s performance from multiple perspectives.
- Cross-Validation Techniques: Moreover, employing cross-validation techniques is crucial for assessing a model’s generalization performance. This approach helps mitigate overfitting by ensuring that the model performs well on unseen data.
- Error Analysis: Furthermore, analyzing errors and misclassifications is vital for identifying common patterns and areas for improvement. This analysis can highlight issues in both the dataset and the models, guiding refinements and enhancing overall performance.
QA Metrics
- Accuracy Rate of Annotation: 98%
- Regular Audits: Weekly checks by senior linguists and AI experts.
- Cross-Validation: Random cross-checking of annotated data by an independent team.
Conclusion
The Call Center Speech Dataset for Retail & E-Commerce is a pioneering endeavor, as it provides invaluable resources for enhancing NLU in AI systems. By encompassing a wide range of interactions, dialects, and scenarios, this dataset is poised to significantly elevate the performance of AI in understanding and responding to customer needs in the retail sector. Moreover, the meticulous annotation process and stringent quality assurance measures ensure the dataset’s reliability and effectiveness in real-world applications.

Quality Data Creation

Guaranteed TAT

ISO 9001:2015, ISO/IEC 27001:2013 Certified

HIPAA Compliance

GDPR Compliance

Compliance and Security
Let's Discuss your Data collection Requirement With Us
To get a detailed estimation of requirements please reach us.