Automated Data Extraction from Invoices
Home » Case Study » Automated Data Extraction from Invoices
Project Overview:
Objective
The objective of automated data extraction from invoices is to streamline and optimize business processes. By utilizing machine learning and OCR technologies, companies can automatically and accurately capture data from invoices. This reduces manual effort, minimizes errors, and improves overall efficiency in financial and administrative tasks.
Scope
Automated data extraction from invoices encompasses the utilization of technology to streamline the capture and processing of invoice data. This approach reduces manual effort and enhances efficiency in financial and administrative tasks for businesses. Moreover, it facilitates seamless integration with existing systems, thereby optimizing workflow management. Additionally, it enables real-time monitoring and analysis of financial transactions, fostering better decision-making processes. Furthermore, it minimizes the risk of errors and inaccuracies often associated with manual data entry, ensuring greater accuracy and reliability in financial records.
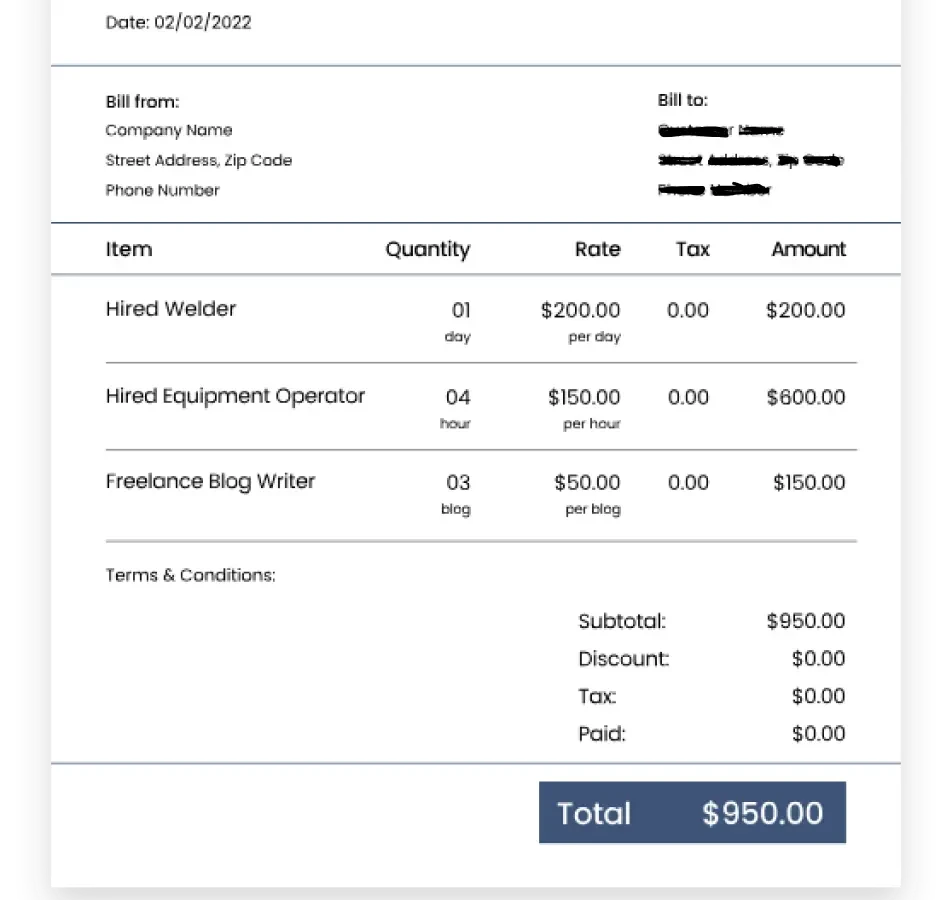
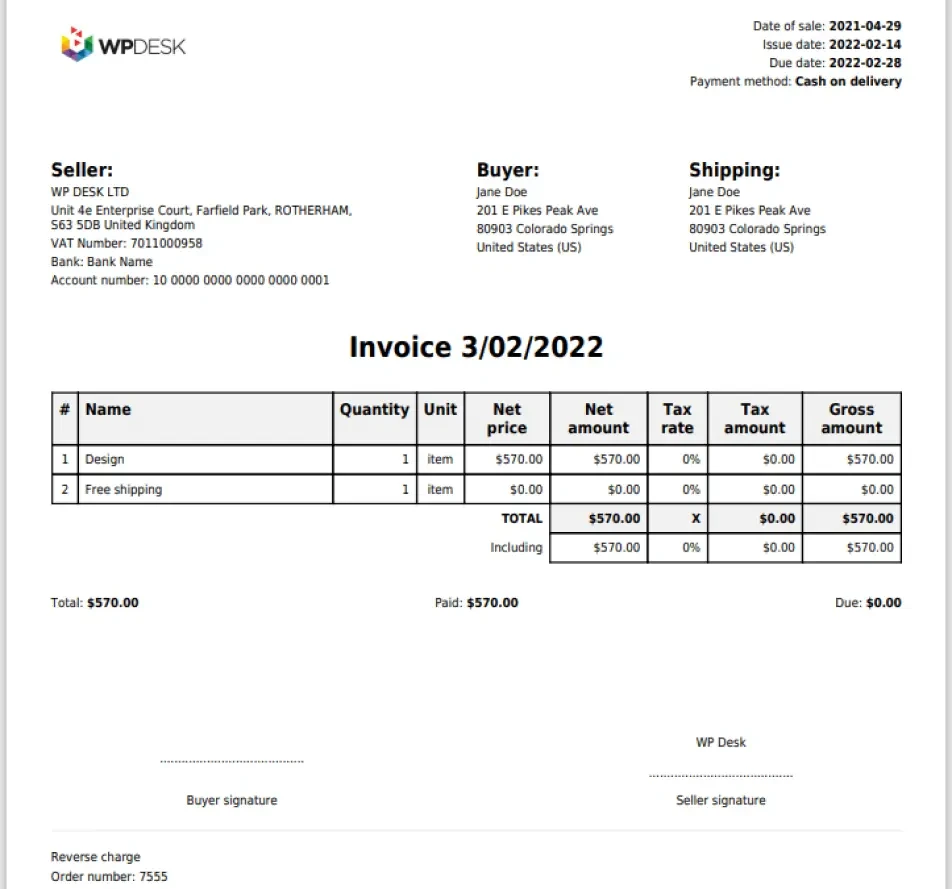
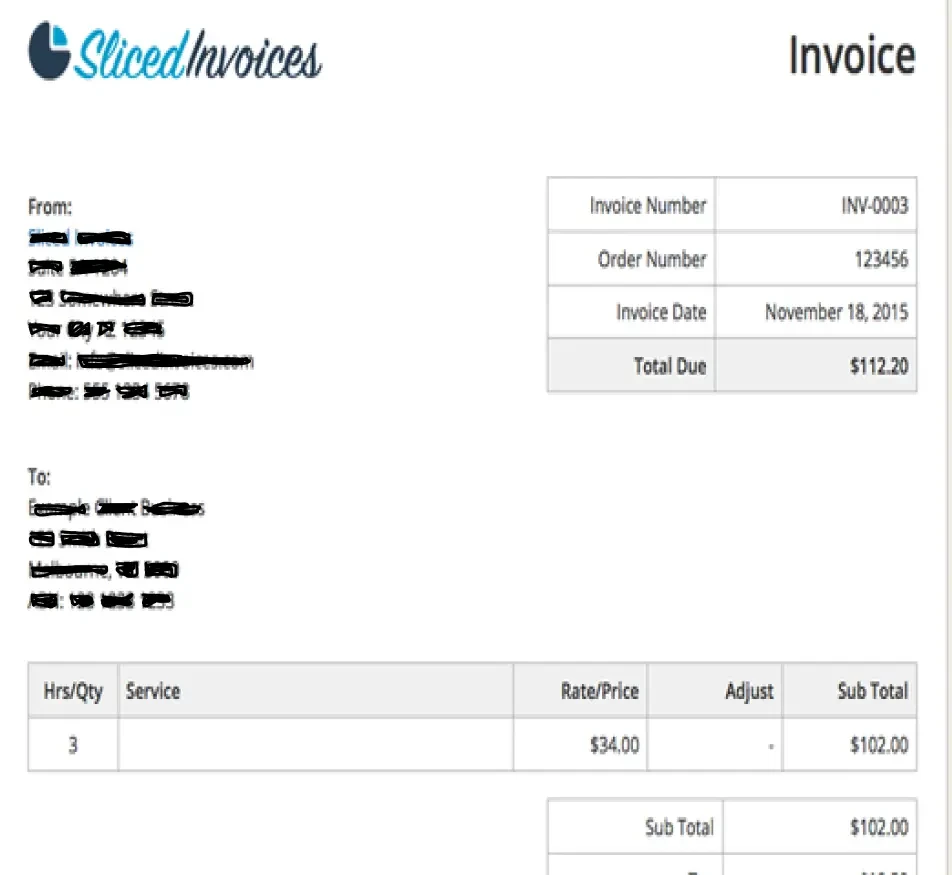
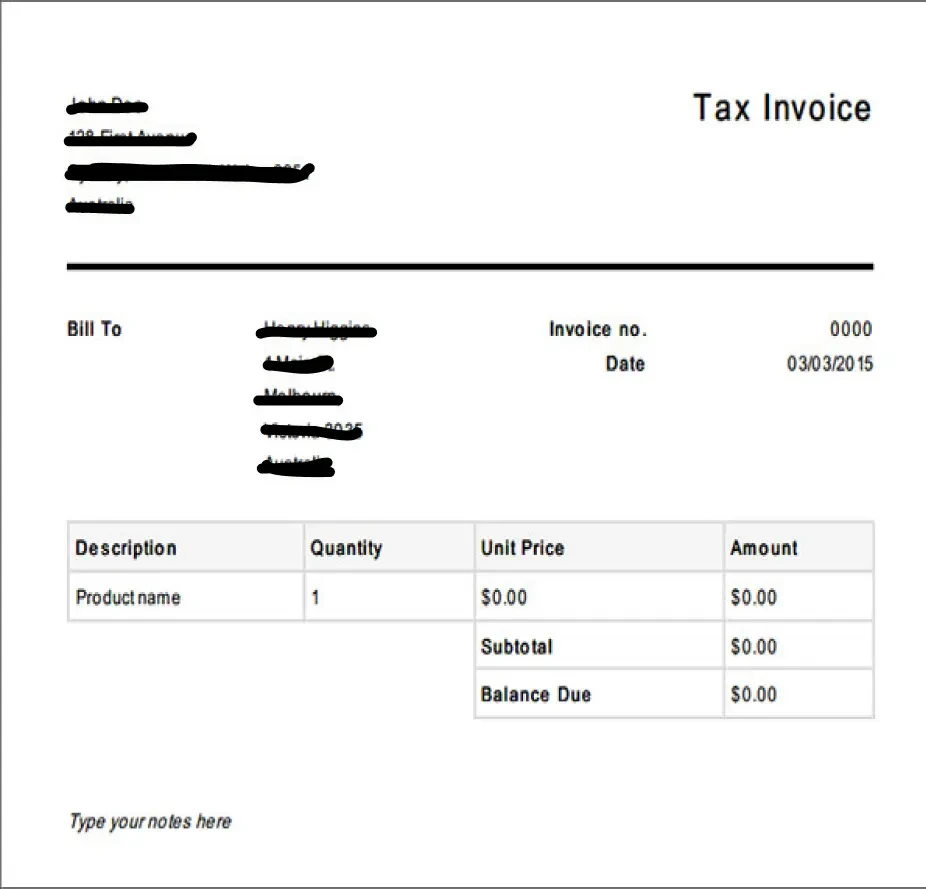
Sources
Industry Reports: Within the financial and technology sectors, reports provide valuable insights into current trends, best practices, and case studies regarding automated data extraction from invoices. These reports highlight emerging technologies and strategies while showcasing successful implementations in various contexts. Moreover, they offer practical guidance for organizations seeking to streamline their invoice processing workflows and improve efficiency.
Â- Documentation from software vendors specializing in data extraction and OCR technologies not only offers practical guidance but also provides solutions for implementing automated invoice processing. These solutions streamline the process by extracting relevant data from invoices and converting them into digital formats. Additionally, they enable businesses to automate repetitive tasks, reducing manual errors and increasing efficiency. Furthermore, these technologies integrate seamlessly with existing systems, ensuring a smooth transition and minimal disruption to operations. Moreover, they offer customizable features that can be tailored to meet specific business needs, enhancing flexibility and scalability.
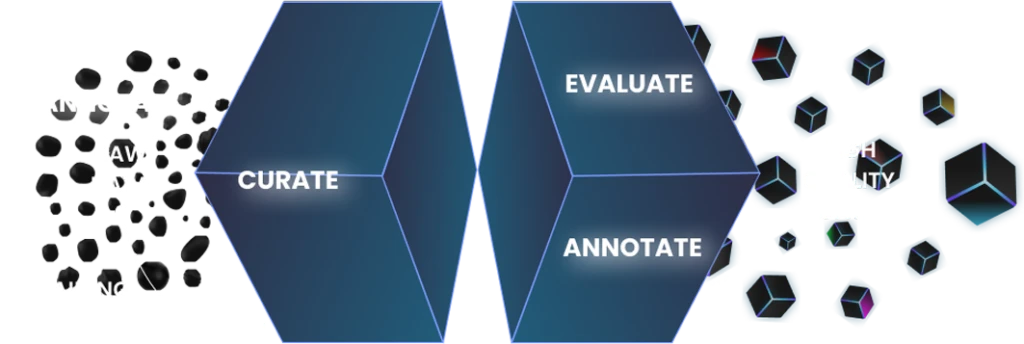
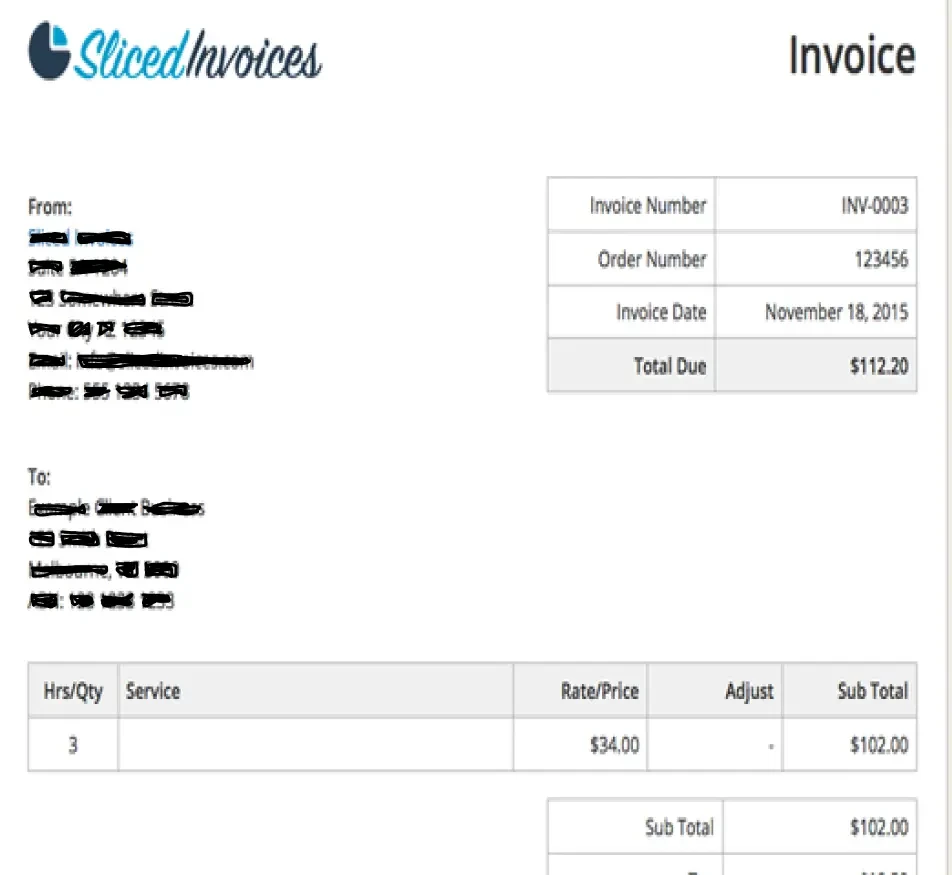
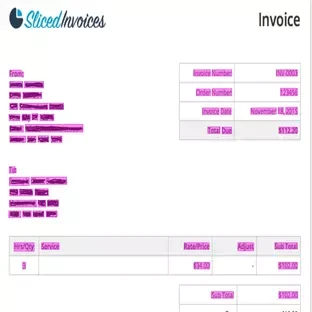
Data Collection Metrics
- Invoice Volume: Total number of processed invoices.
- Data Accuracy: Measurement of correctness and completeness of extracted data.
Annotation Process
Stages
- Data Capture: Capture invoice data from various sources, including scanned documents and digital formats.
- Preprocessing: Prepare the data for extraction by cleaning, enhancing, and standardizing it.
- OCR and Extraction: Employ OCR and data extraction algorithms to identify and extract relevant information.
- Data Validation: Verify the accuracy of extracted data and perform validation checks.
- Integration: Integrate the extracted data into financial and administrative systems for further processing.
- Feedback and Improvement: Continuously monitor and improve the extraction process based on feedback and evolving invoice formats.
Annotation Metrics
- Accuracy Rate: Measures correctness compared to a reference or gold standard.
- Inter-annotator Agreement: Evaluates consistency among different annotators when performing the same annotation tasks.
- Annotation Speed: Tracks the time taken for each annotation task.
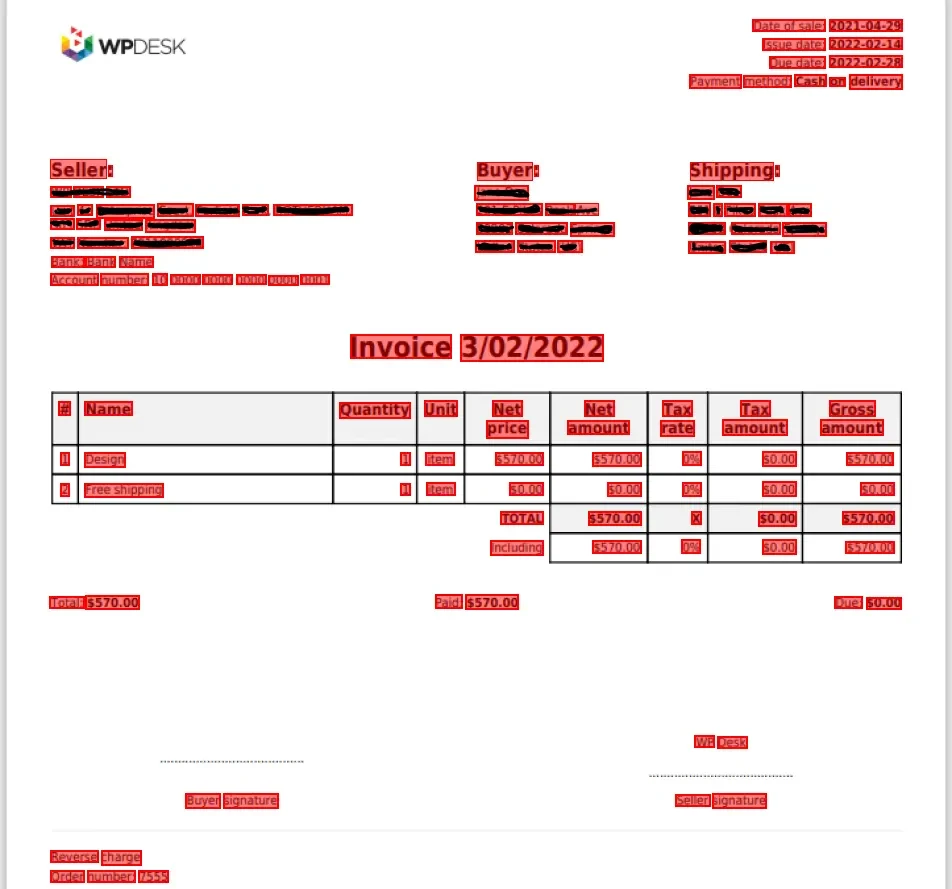
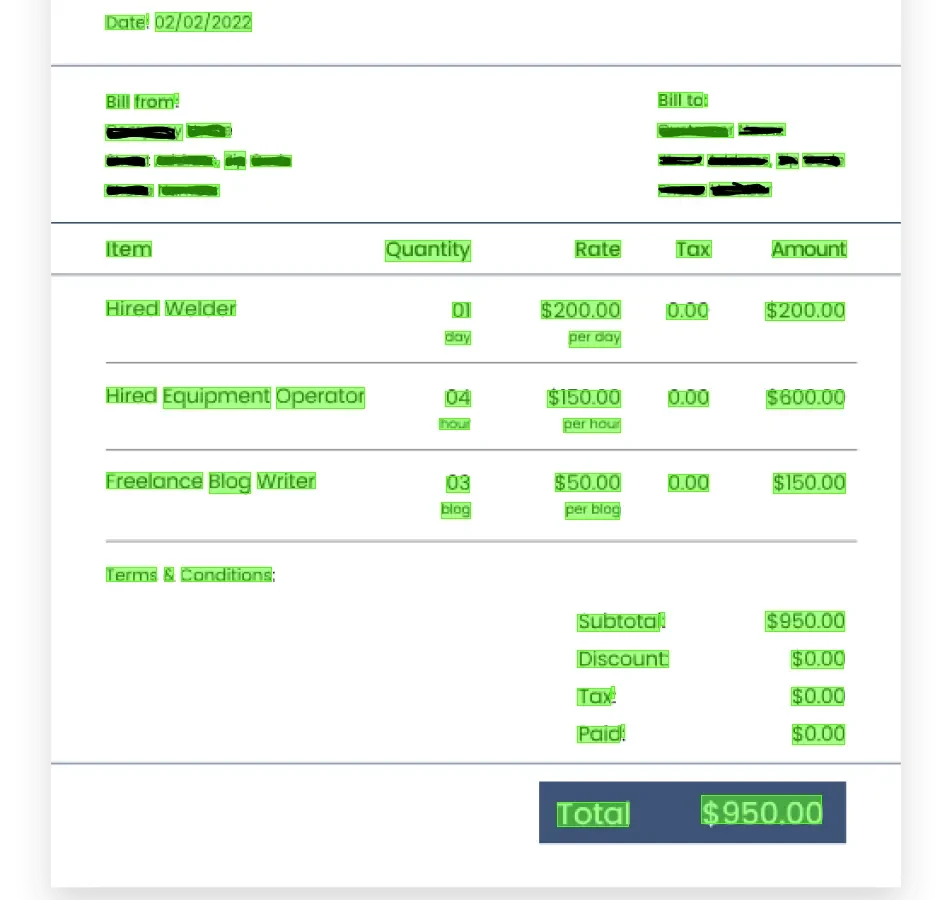
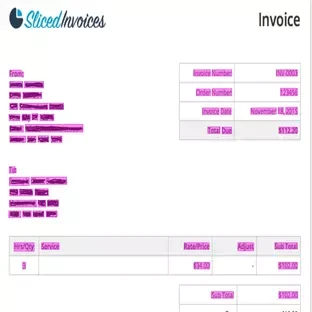
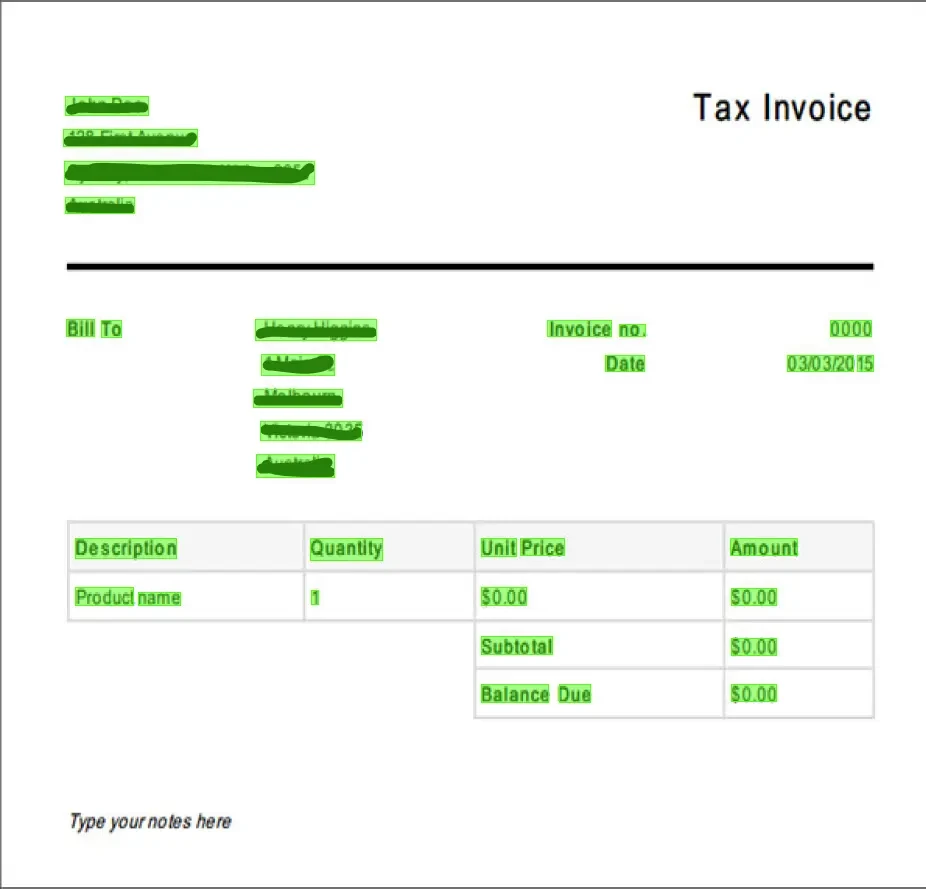
Quality Assurance
Stages
Data Accuracy Testing: Implement rigorous quality checks to ensure accurate data extraction from invoices.
Data Security: Safeguard sensitive financial data by adhering to data security protocols and privacy regulations.
User Consent and Transparency: Ensure transparency in data handling and obtain user consent when necessary to maintain privacy and compliance.
QA Metrics
- Defect Density: Measures the number of defects per unit, indicating the quality of data extraction.
- Accuracy Testing: Evaluates the accuracy of extracted data through rigorous quality checks.
Conclusion
Automated data extraction from invoices represents a transformative leap in streamlining business processes and improving efficiency. By leveraging machine learning and optical character recognition (OCR) technologies, organizations can significantly reduce manual data entry, errors, and processing times.

Quality Data Creation

Guaranteed TAT

ISO 9001:2015, ISO/IEC 27001:2013 Certified

HIPAA Compliance

GDPR Compliance

Compliance and Security
Let's Discuss your Data collection Requirement With Us
To get a detailed estimation of requirements please reach us.