Named Entity Recognition for Legal Documents
Home » Case Study » Named Entity Recognition for Legal Documents
Project Overview:
Objective
Our latest project involved applying Named Entity Recognition (NER) to legal documents. The goal was to seamlessly identify and categorize key entities such as individual names, organizations, legal terminologies, dates, and clauses within complex legal texts. This project highlights our capability in handling diverse datasets, including text data, vital for machine learning models.
Scope
The scope of Named Entity Recognition (NER) for legal documents covers the detection and classification of specific entities such as party names, legal references, and dates within legal texts
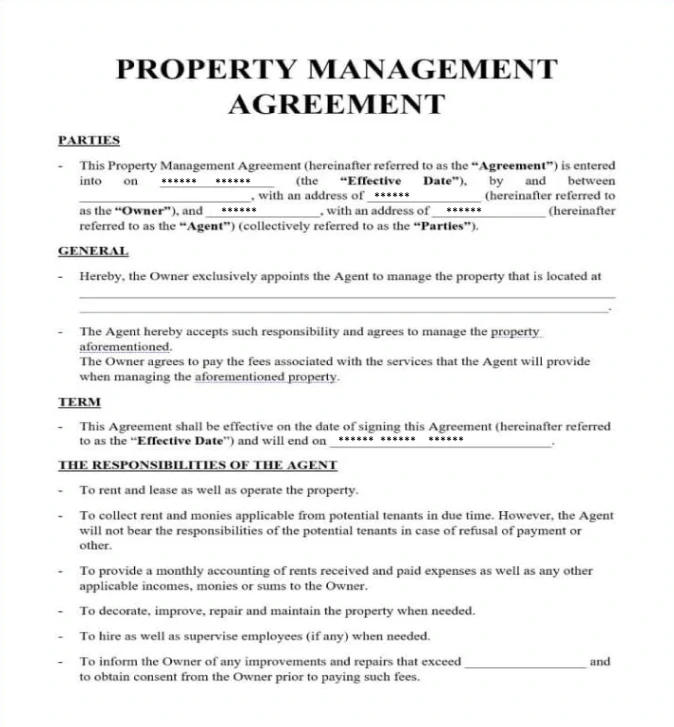
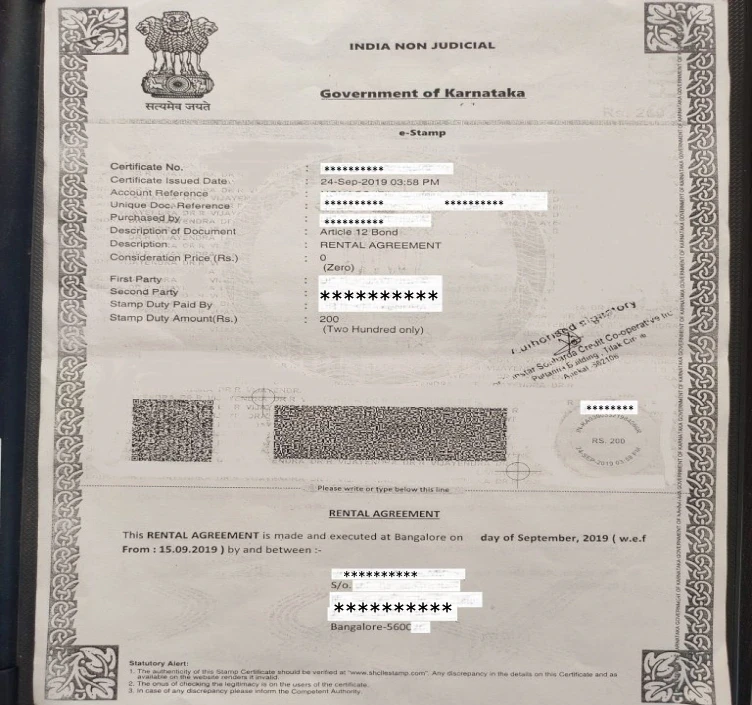
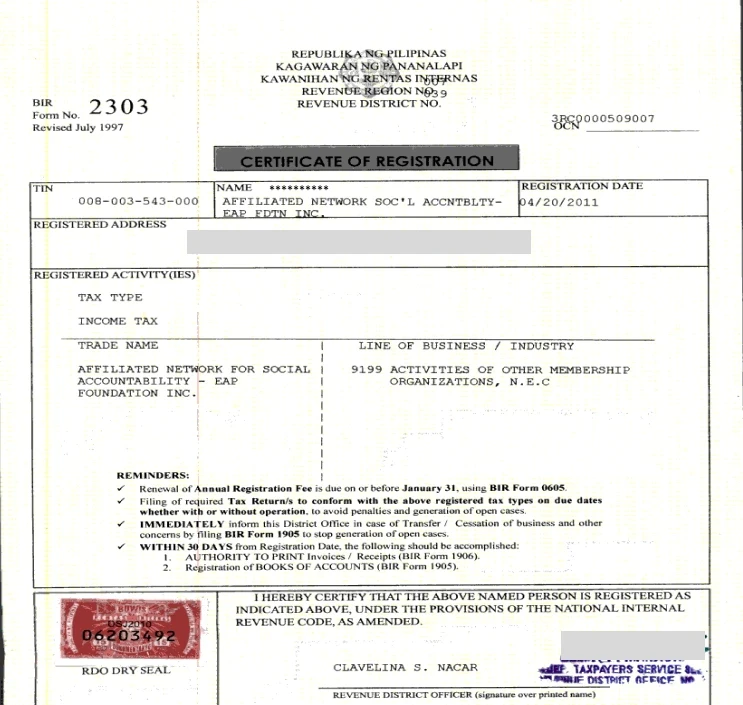
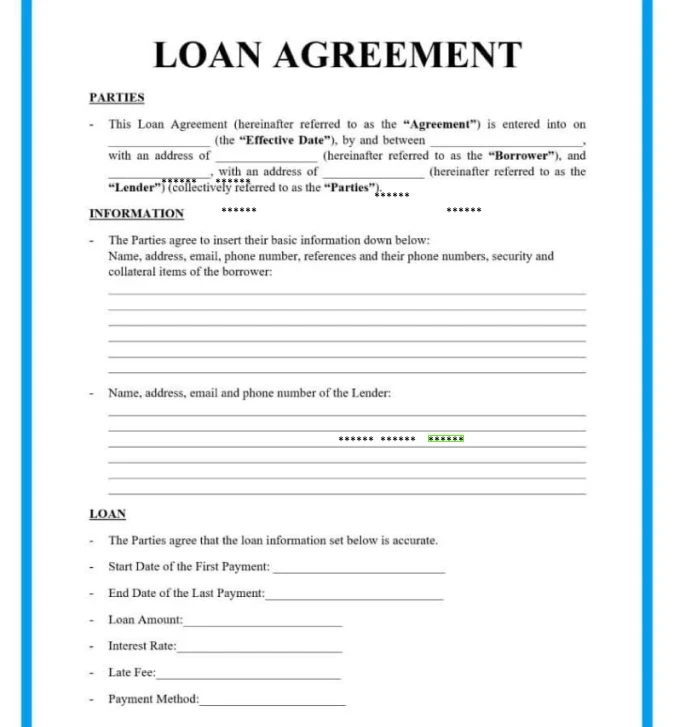
Sources
- Legal Repositories: Databases containing statutes, case laws, and legal journals.
- Document Archives: Collections of contracts, agreements, and other legal paperwork.
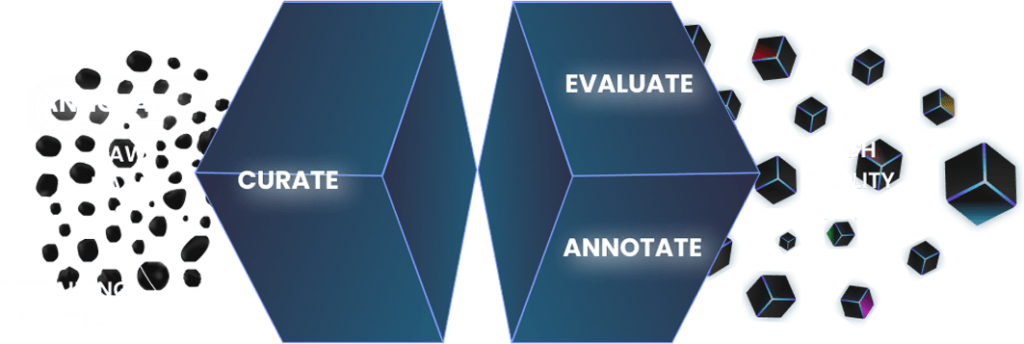
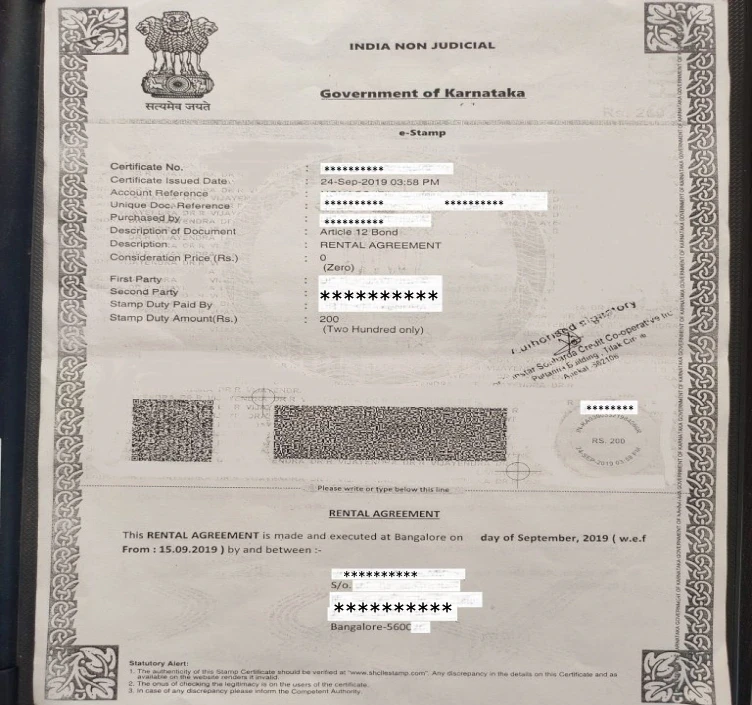
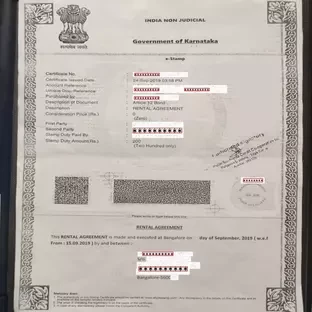
Data Collection Metrics
- Coverage Rate: Percentage of total legal documents from which entities are extracted.
- Entity Accuracy: Proportion of correctly identified and classified entities in the sampled documents.
Annotation Process
Stages
- Preprocessing: Cleaning and standardizing the legal text for analysis.
- Training: Feeding labeled legal data to train the NER models.
- Entity Extraction: Identifying specific entities within the legal documents.
- Entity Classification: Categorizing the extracted entities into predefined classes.
- Validation: Cross-checking the identified entities against a benchmark or labeled dataset.
- Integration: Incorporating the extracted data into relevant systems or databases.
- Feedback & Refinement: Iteratively improving the model based on performance feedback.
Annotation Metrics
- Annotation Consistency: Degree of agreement among multiple annotators for the same entities.
- Entity Boundary Accuracy: Correctness in determining the start and end points of an entity.
- Entity Type Accuracy: Proportion of entities correctly classified into their respective categories.
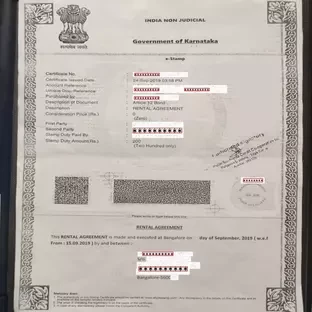
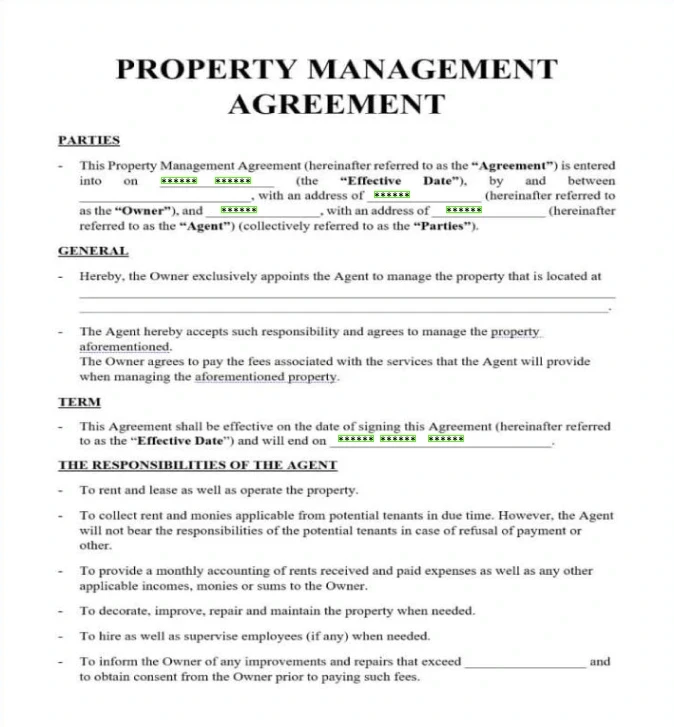
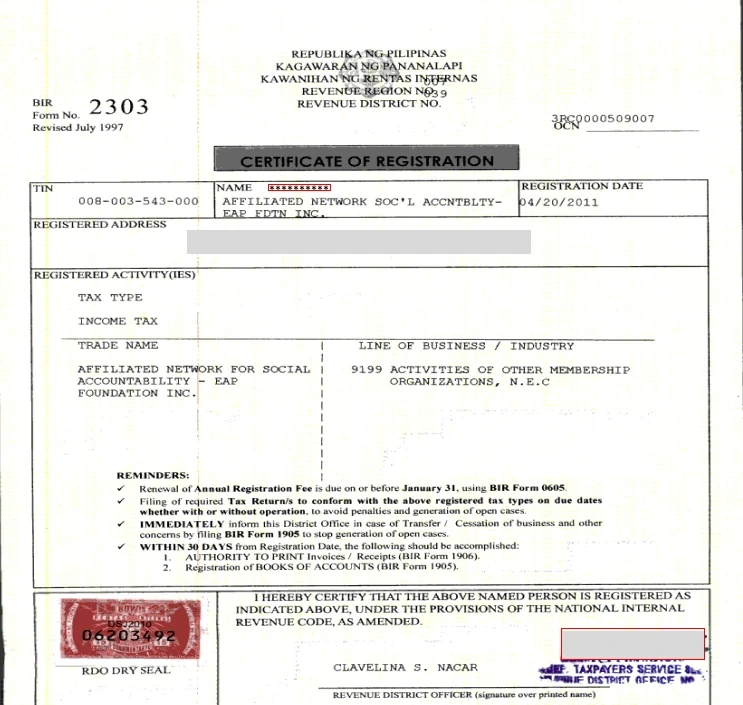
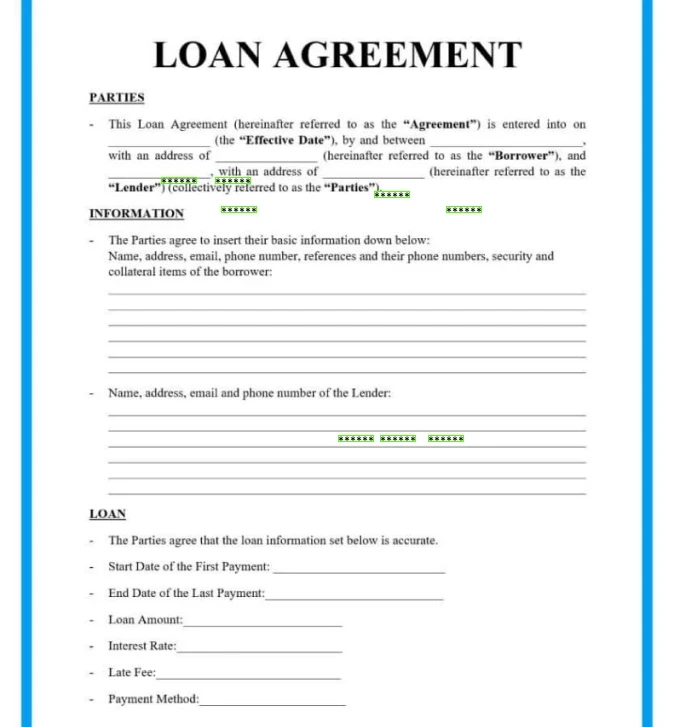
Quality Assurance
Stages
Data Validation: Implementing protocols to ensure the accuracy and relevance of extracted entities.
Anonymization: Removing or obfuscating personal and sensitive data to uphold privacy standards.
Role-based Access: Granting data access only to authorized individuals to prevent misuse and ensure data privacy.
QA Metrics
- Accuracy Rate: Percentage of entity identifications and classifications that are correct.
- False Positive Rate: Proportion of incorrectly identified entities relative to all identified entities.
Conclusion
Named Entity Recognition (NER) for legal documents is a pivotal tool in extracting structured information from vast, intricate legal texts. By identifying and classifying entities such as party names, dates, contract clauses, and legal references, NER enhances the efficiency and accuracy of legal data retrieval.

Quality Data Creation

Guaranteed TAT

ISO 9001:2015, ISO/IEC 27001:2013 Certified

HIPAA Compliance

GDPR Compliance

Compliance and Security
Let's Discuss your Data collection Requirement With Us
To get a detailed estimation of requirements please reach us.