German Traffic Sign Recognition Dataset
Home » Case Study » German Traffic Sign Recognition Dataset
Project Overview:
Objective
Our main aim was to create the German Traffic Sign Recognition Dataset, which not only assists current systems in recognizing traffic signs but also paves the way for advancements in new self-driving car technologies. We aimed to ensure that these systems can spot and understand road signs with greater accuracy and speed.
Scope
To make this happen, we carefully gathered and marked lots of different traffic signs from various places in Germany, even in different weather and lighting. Our dataset was made to look like real-life situations, so AI models can work well in all sorts of environments.
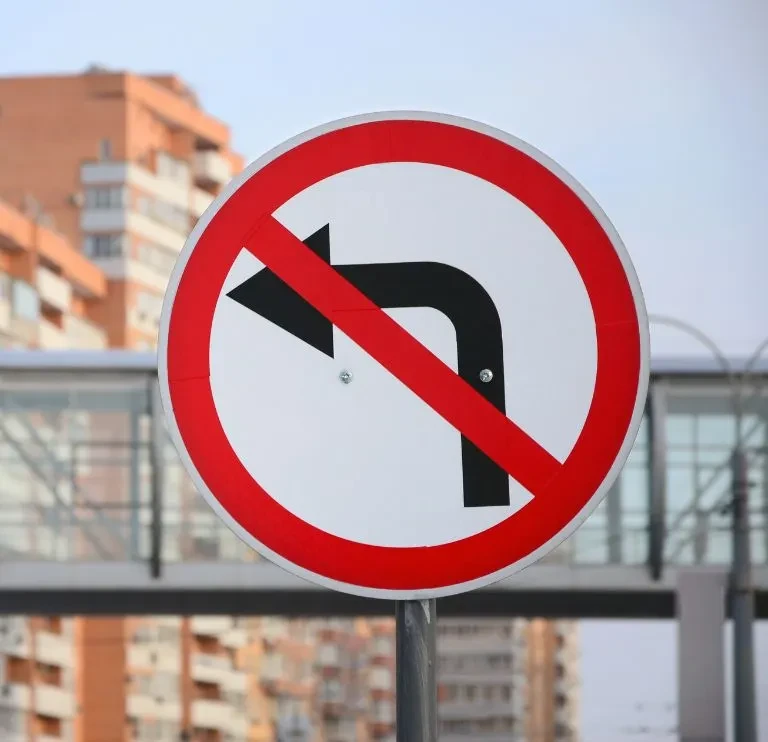
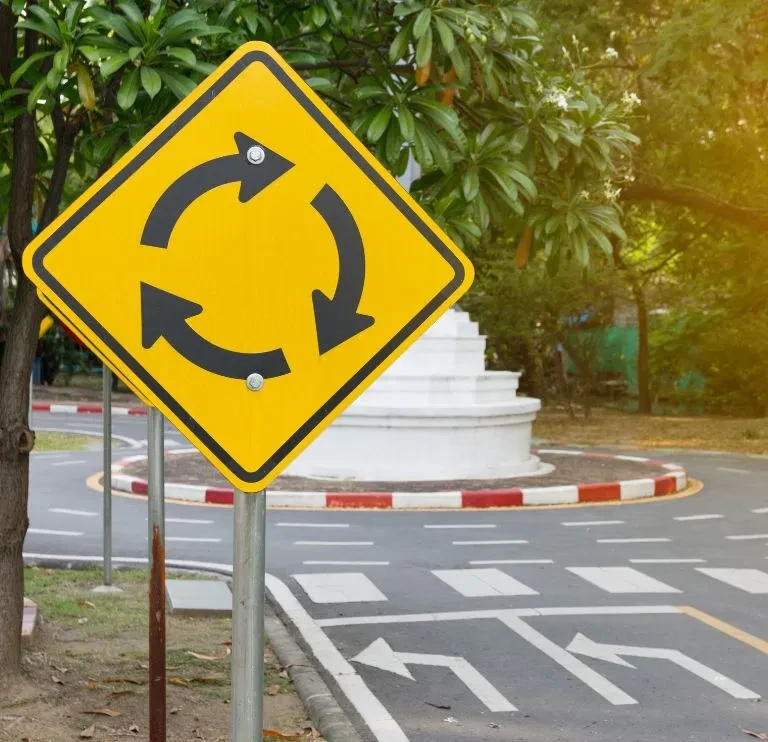
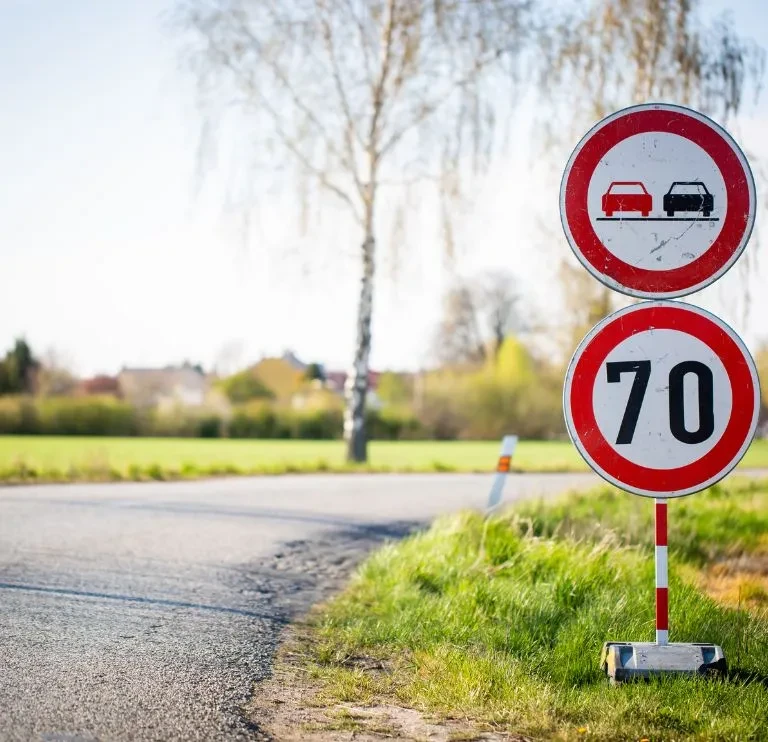
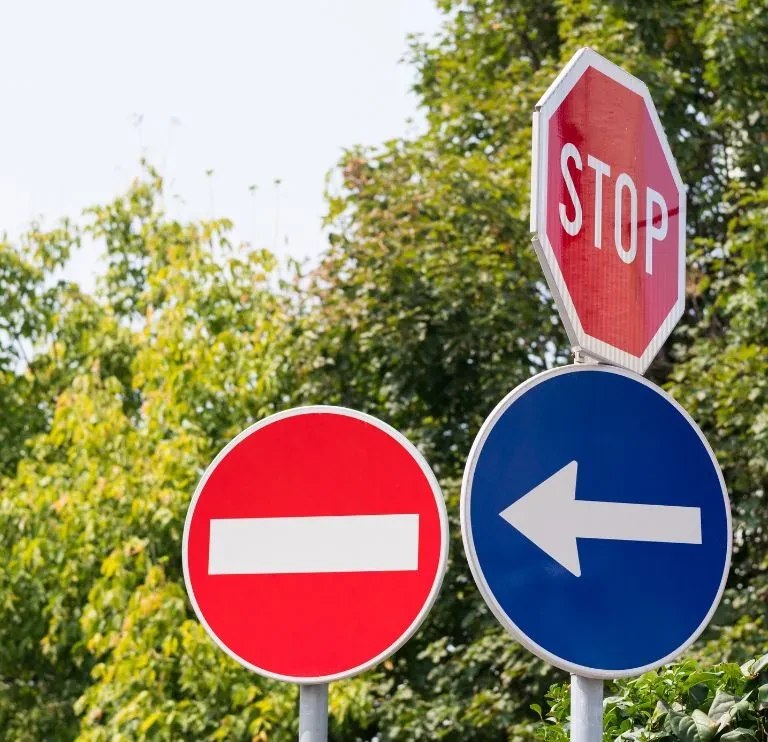
Sources
- On-Site Captures: We sent teams to different places in Germany to take pictures of traffic signs using cameras fixed on vehicles.
- Drones: Drones with cameras were used to take pictures of areas that are difficult to access from the ground.
- Public and Government Contributions: We worked with local authorities to get old pictures and videos of traffic signs, making our dataset more diverse.
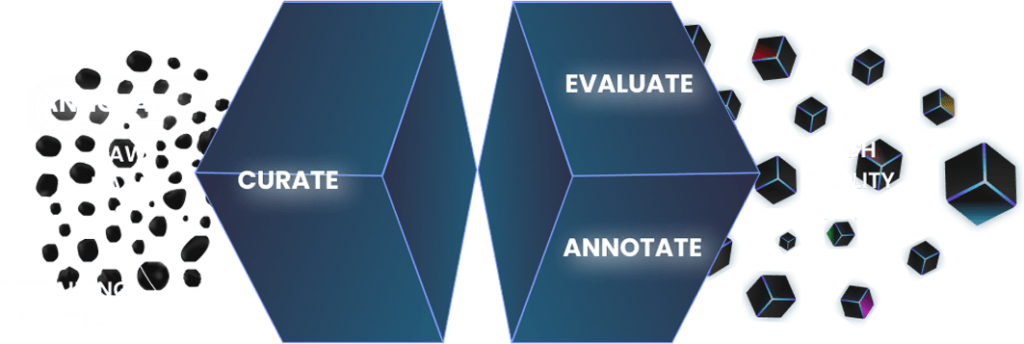
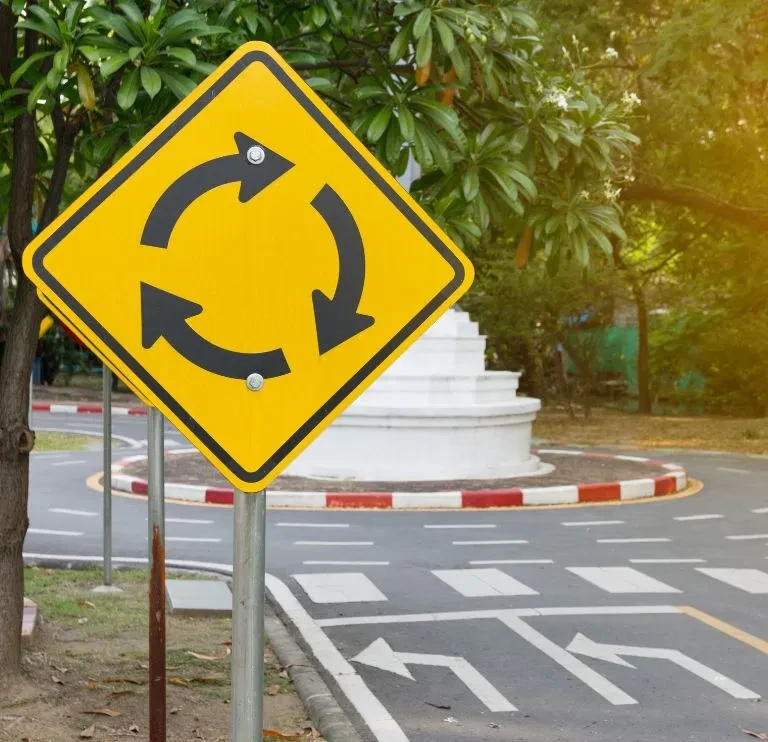
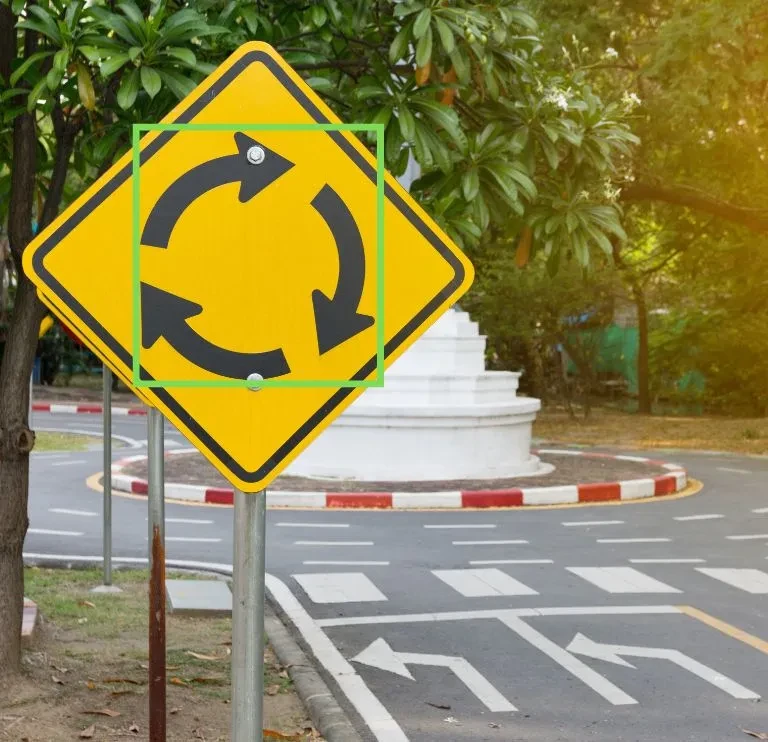
Data Collection Metrics
- Images Captured: We collected 50,000 pictures of different traffic signs.
- High-Resolution Variants: Among these, 15,000 images were high-resolution, allowing for detailed analysis of features.
Annotation Process
Stages
- Manual Tagging: Skilled annotators carefully tagged each picture with precise details like the type of sign, where it was seen (e.g., urban or rural), and extra information like the time of day and weather.
- Automated Tools: We also used semi-automatic tools to initially label images, which were then checked by humans to ensure accuracy.
Data Annotated for ML Training:
- Total Annotations: We added details to 75,000 parts of the images.
- Quality Assurance: Every annotation went through several checks to make sure it was correct, with extra attention given to the specific features of German traffic signs.
Annotation Metrics
- Categorization Labels: We added 75,000 labels, which included over 100 different types of traffic signs.
- Contextual Tags: We provided 50,000 tags describing the surroundings of each sign.
- Accuracy Checks: We double-checked all annotations against the official specifications for German traffic signs.
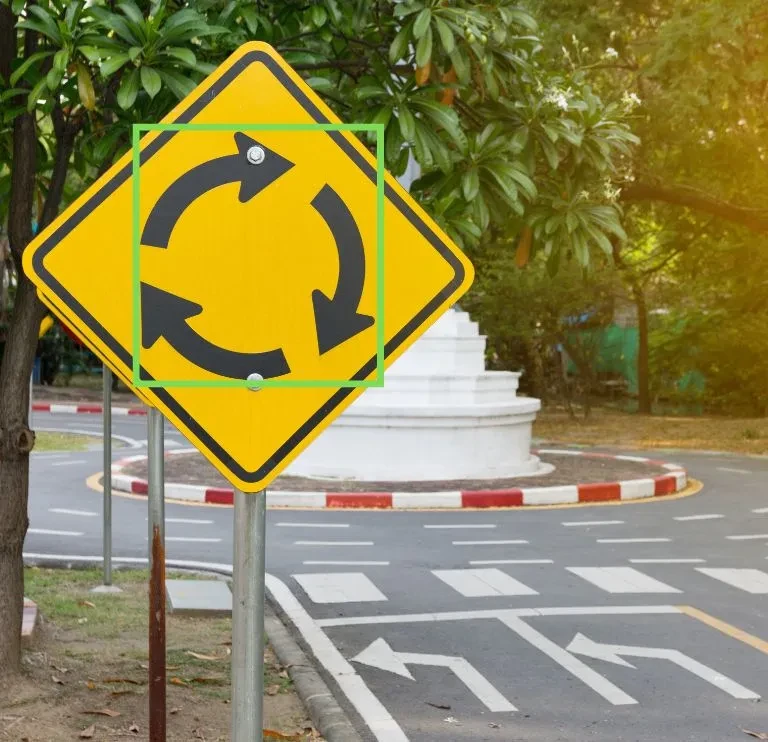
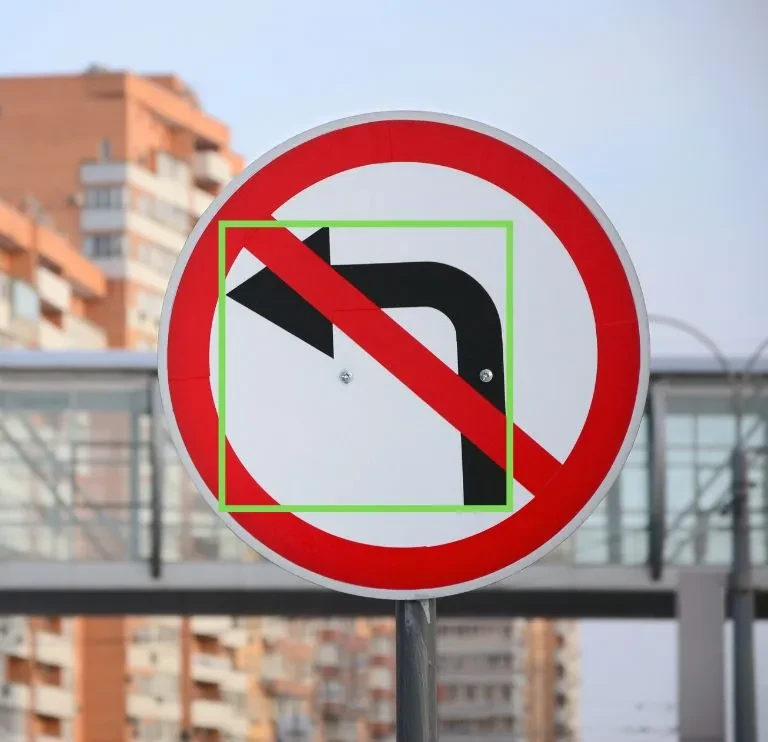
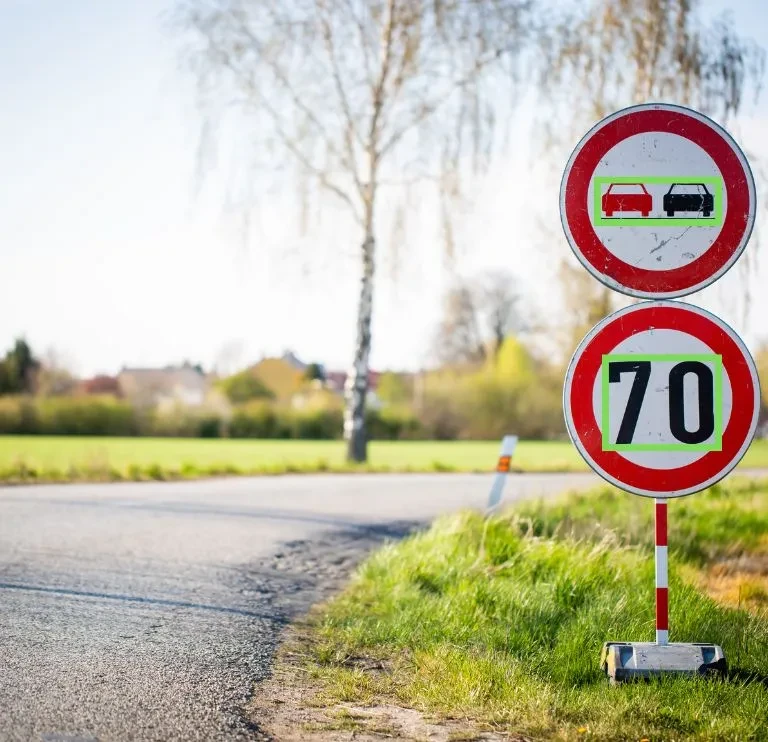
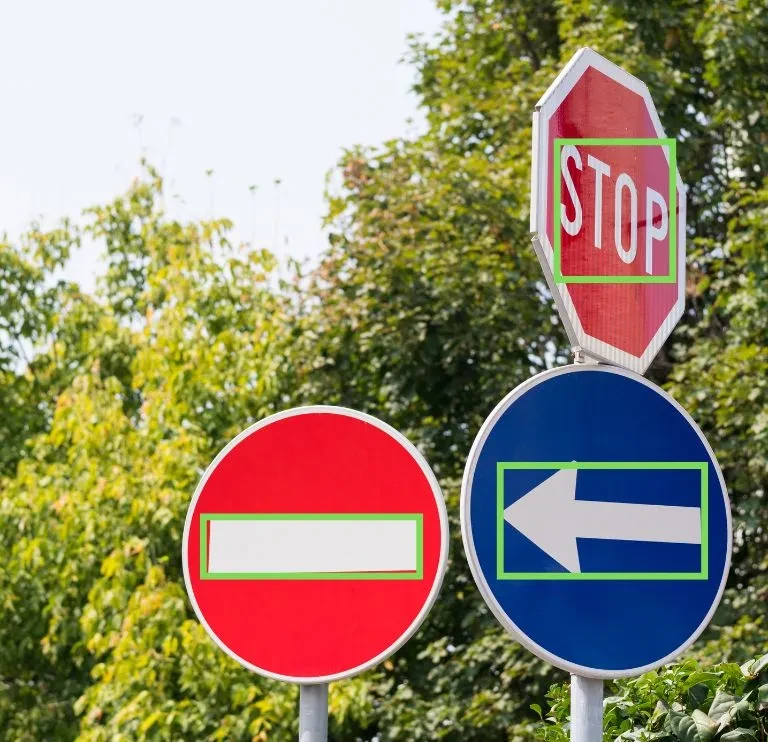
Quality Assurance
Stages
Model Testing: We continuously test our early machine learning models to make sure they can recognize signs accurately.
Privacy and Legal Compliance: We strictly follow the EU GDPR laws when dealing with and using photo data, to protect people’s privacy and obey the law.
QA Metrics
- Recognition Accuracy: We reached a starting accuracy level of 95% in recognizing traffic signs, which is better than before.
- Impact: Our dataset has played a key role in making traffic sign recognition systems for self-driving cars in Germany more dependable and effective.
Conclusion
The Traffic Sign Recognition Dataset – German Traffic Sign Recognition Benchmark is a big leap forward in using computers to help cars drive themselves. With this dataset, which is very accurate and flexible, we’re helping make self-driving cars smarter and safer.

Quality Data Creation

Guaranteed TAT

ISO 9001:2015, ISO/IEC 27001:2013 Certified

HIPAA Compliance

GDPR Compliance

Compliance and Security
Let's Discuss your Data collection Requirement With Us
To get a detailed estimation of requirements please reach us.