Road Lane Instance Segmentation
Home » Dataset Download » Road Lane Instance Segmentation
Road Lane Instance Segmentation
Datasets
Road Lane Instance Segmentation
File
Road Lane Instance Segmentation
Use Case
Road Lane Instance Segmentation
Description
Explore a comprehensive Road Lane Instance Segmentation dataset featuring high-resolution dashcam images with annotated lane markings like solid lines, divider lines, and more.
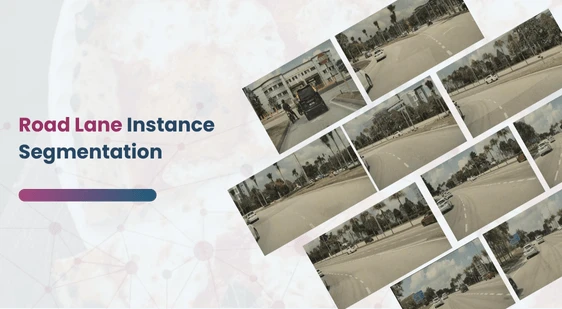
Description:
The Road Lane Instance Segmentation dataset consists of a collection of images captured from vehicle dashcams, providing detailed segmentation labels for various types of road lane markings. This dataset is specifically designed to support the development of advanced driver assistance systems (ADAS), autonomous driving research, and lane detection algorithms. It provides high-quality, annotated data for training machine learning models capable of accurately recognizing and distinguishing between different types of lane markings on diverse road surfaces.
Dataset Composition:
Each image in the dataset contains comprehensive instance segmentation labels for different road lane markings, allowing precise identification of lane boundaries and other road-related lines. The annotations are pixel-perfect, ensuring high accuracy for tasks like semantic segmentation, lane boundary detection, and instance-level classification.
Download Dataset
Classes:
The dataset categorizes road lane markings into the following classes:
- Divider Line: Solid lines used to separate traffic moving in opposite directions, typically found in the center of the road.
- Dotted Line: Broken lines used for lane division or indicating areas where lane changes are permitted.
- Double Line: A pair of solid lines, often indicating areas where overtaking is prohibited.
- Random Line: Miscellaneous markings such as temporary construction lines, which may not follow standard lane marking patterns.
- Road Sign Line: Specialized markings indicating directions, such as turn arrows or crosswalks.
- Solid Line: Continuous lines used to define the edge of a lane or boundary where lane changes are not allowed.
Data Annotations:
- Instance Segmentation: Each lane marking is segmented with a unique instance mask, allowing algorithms to distinguish between individual lanes and classify them accordingly.
- Bounding Boxes: In addition to instance segmentation masks, the dataset also provides bounding box annotations for lane markings.
- Lane Curvature and Width: Some annotations include additional metadata relate to the curvature and width of lanes, useful for training models on highways, city streets, and rural roads.
Applications:
This dataset is suitable for a wide range of computer vision tasks, including:
- Autonomous Driving: The dataset is ideal for developing systems capable of lane detection and navigation in autonomous vehicles, enabling safe lane-keeping and transition maneuvers.
- Advanced Driver Assistance Systems (ADAS): Useful for real-time lane detection and warning systems in smart vehicles.
- Road Condition Monitoring: Can be use by transportation authorities to identify worn-out or missing lane markings that need maintenance.
- Traffic Management Systems: Enables smart city infrastructure by integrating road lane data for efficient traffic flow management.
Dataset Features:
- Diverse Road Conditions: The images cover a wide variety of road conditions, including urban, suburban, and highway environments, with varying lighting conditions (day/night) and weather situations (rain, snow, fog).
- Resolution and Quality: All images are high-resolution, ensuring clear visualization of lane details, even in complex or challenging scenes.
- Scalable and Customizable: The dataset is expandable with additional road classes and can be customize according to specific user requirements or research goals.
- Platform Compatibility: The dataset can be seamlessly integrate into machine learning platforms like TensorFlow, PyTorch, and others, with annotations provide in commonly use formats such as COCO and Pascal VOC.
This dataset is sourced from Kaggle.
Contact Us

Quality Data Creation

Guaranteed TAT

ISO 9001:2015, ISO/IEC 27001:2013 Certified

HIPAA Compliance

GDPR Compliance

Compliance and Security
Let's Discuss your Data collection Requirement With Us
To get a detailed estimation of requirements please reach us.