Road Anomaly Dataset
Home » Dataset Download » Road Anomaly Dataset
Road Anomaly Dataset
Datasets
Road Anomaly Dataset
File
Road Anomaly Dataset
Use Case
Road Anomaly Dataset
Description
Explore the Road Anomaly Dataset, featuring annotated footage of accidents, car fires, violent altercations, and armed robberies.
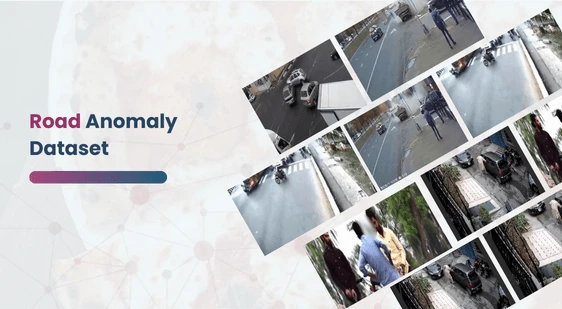
Description:
The Road Anomaly Dataset (RAD) is meticulously designed to tackle the urgent need for real-time detection and response to dangerous and potentially life-threatening road anomalies through surveillance footage. This dataset captures a wide spectrum of hazardous road-related activities, including but not limited to vehicular accidents, car fires, violent altercations, and armed thefts. The RAD dataset serves as a vital resource for improving public safety by equipping machine learning models to detect such anomalies with precision and speed, ensuring a faster and more effective emergency response.
Motivation
With the proliferation of Closed-Circuit Television (CCTV) cameras in urban and rural areas, there is an increasing demand for intelligent surveillance systems capable of detecting abnormal activities in real-world environments. Detecting road anomalies in real-time is particularly crucial, as it can significantly reduce response times to dangerous situations, preventing injuries, fatalities, and property damage. Traditional surveillance systems, however, often fail to recognize these anomalies quickly enough, leading to delayed emergency responses. RAD aims to address this challenge by providing a comprehensive dataset that can train advanced machine learning models to identify, classify, and respond to road anomalies effectively.
Download Dataset
Dataset Description
The RAD dataset comprises an extensive collection of high-quality images and videos, systematically annotated to reflect a variety of road anomalies. The dataset focuses on four primary categories, each representing a critical risk to human safety and property:
- Accidents: Includes footage of car crashes, multi-vehicle collisions, and other traffic-related incidents that pose immediate dangers.
- Car Fires: Captures instances of vehicles engulfed in flames, emphasizing the importance of quick detection to prevent further casualties and property damage.
- Fighting: Contains videos of physical altercations occurring on streets or roads, which could escalate into severe violence or endanger bystanders.
- Armed Robberies (Snatching at Gunpoint): Features videos of robberies and thefts involving firearms, where immediate response is essential for protecting lives and reducing the impact of criminal activities.
Each category in the dataset is annotated with detailed labels to ensure the accurate identification and classification of these anomalies. The annotations include temporal and spatial metadata, allowing for precise localization of the anomalies within the footage. This dataset is primarily aimed at training and testing machine learning models, particularly those using Convolutional Neural Networks (CNNs) and other deep learning architectures for high-accuracy anomaly detection.
Additional Categories and Data Enrichment
To further enhance the dataset’s utility, additional categories of road anomalies are being considered, including:
- Illegal Parking: Captures instances of vehicles parked in unauthorized or hazardous locations, potentially obstructing traffic or emergency services.
- Obstructions on Roadways: Identifies obstacles such as fallen trees, debris, or stalled vehicles, which can disrupt traffic flow and pose risks to motorists.
- Pedestrian Accidents: Includes footage of collisions involving pedestrians, which are often more severe due to the vulnerability of those involved.
Moreover, the RAD dataset has been enriched with contextually relevant metadata, such as environmental conditions (e.g., weather, lighting) and road types (e.g., urban streets, highways), further refining the data for more nuanced training applications.
Applications
The RAD dataset is ideal for developers and researchers working in public safety, smart cities, and autonomous vehicle technologies. Its primary applications include:
- Surveillance Systems: To improve the accuracy and responsiveness of AI-powered surveillance systems by detecting hazardous road activities.
- Traffic Management: To help authorities monitor and manage traffic flow more efficiently, identifying potential threats in real-time.
- Autonomous Vehicles: For the training of autonomous vehicle systems, equipping them to recognize and respond to road anomalies during navigation.
Contact Us

Quality Data Creation

Guaranteed TAT

ISO 9001:2015, ISO/IEC 27001:2013 Certified

HIPAA Compliance

GDPR Compliance

Compliance and Security
Let's Discuss your Data collection Requirement With Us
To get a detailed estimation of requirements please reach us.