OCT Images Dataset
Home » Dataset Download » OCT Images Dataset
OCT Images Dataset
Datasets
OCT Images Dataset
File
OCT Images Dataset
Use Case
OCT Images Dataset
Description
Explore a balanced dataset of retinal OCT images, ideal for training AI models in diagnosing retinal diseases like CNV, DME, AMD, and more.
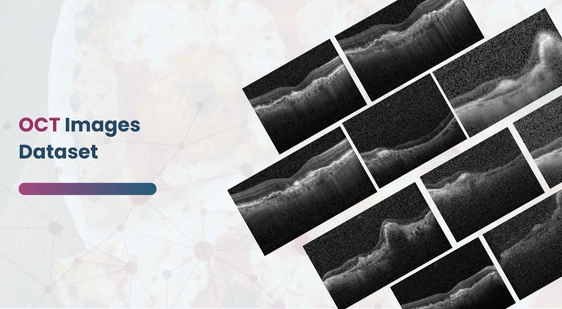
Description:
Retinal Optical Coherence Tomography (OCT):
OCT is a non-invasive imaging technique that generates high-resolution cross-sectional images of the retina. This method is critical for diagnosing and monitoring retinal conditions by allowing clinicians to examine the internal structure of the retina in great detail. According to estimates, around 30 million OCT scans are conducted annually worldwide, underscoring the importance of efficient analysis and interpretation of these images. Traditional OCT image analysis demands significant time and expertise, which is why machine learning models trained on such datasets are increasingly being adopted to assist in the diagnostic process.
Download Dataset
Dataset Composition:
The dataset consists of retinal OCT images divided into four main categories of retinal conditions, enabling researchers to develop balanced models that can classify different eye conditions:
- Choroidal Neovascularization (CNV): This condition involves the formation of new blood vessels in the choroid layer, typically associated with wet age-related macular degeneration (AMD). OCT scans reveal features such as subretinal fluid and the presence of neovascular membranes.
- Image Characteristics: These images highlight areas where fluid has accumulated beneath the retina (subretinal fluid), which appears as dark spaces in the scans. The neovascular membrane can be identify as irregular structures disrupting the retinal layers.
- Diabetic Macular Edema (DME): A complication of diabetes, DME occurs when fluid accumulates in the retina due to leaking blood vessels. Leading to swelling and impaired vision. OCT images of DME typically show increased retinal thickness and the presence of intraretinal fluid.
- Image Characteristics: Retinal-thickening and pockets of intraretinal fluid can be seen as circular dark spaces in the middle layers of the retina, indicating areas affected by edema.
Balanced Version – Importance of Equal Representation:
This balanced version of the dataset ensures that each condition, including normal retinas, is equally represented. By balancing the dataset, researchers can train models that are less biased and can more accurately predict different retinal conditions, thereby improving the generalization ability of AI models.
Applications of the Dataset:
- AI-Assisted Diagnostics: The dataset can be use to develop machine learning algorithms that assist ophthalmologists in diagnosing retinal diseases. Improving both the speed and accuracy of diagnosis.
- Transfer Learning: Given the small size of the dataset, it is an excellent candidate for transfer learning applications. Where pre-train models can be fine-tuned for medical imaging tasks.
- Research in Retinal Disease Progression: The dataset can also be use for studying disease progression by analyzing changes in OCT scans over time. Potentially offering insights into early intervention strategies.
In conclusion, this balance version of the OCT dataset provides a foundational resource for AI-driven medical research, facilitating advancements in the diagnosis, monitoring, and treatment of various retinal diseases. By ensuring equal representation across conditions. The dataset enables more robust model training. Which is essential for developing AI tools that are clinically useful in real-world settings.
This dataset is sourced from Kaggle.
Contact Us

Quality Data Creation

Guaranteed TAT

ISO 9001:2015, ISO/IEC 27001:2013 Certified

HIPAA Compliance

GDPR Compliance

Compliance and Security
Let's Discuss your Data collection Requirement With Us
To get a detailed estimation of requirements please reach us.