Object Detection – Wildlife Dataset – YOLO Format
Home » Dataset Download » Object Detection – Wildlife Dataset – YOLO Format
Object Detection – Wildlife Dataset – YOLO Format
Datasets
Object Detection – Wildlife Dataset – YOLO Format
File
Object Detection – Wildlife Dataset – YOLO Format
Use Case
Computer Vision
Description
To create a dataset for YOLO-based object detection, we compile 1500 images across four classes: buffalo, elephant, rhino, and zebra, preprocessed for optimal model training.
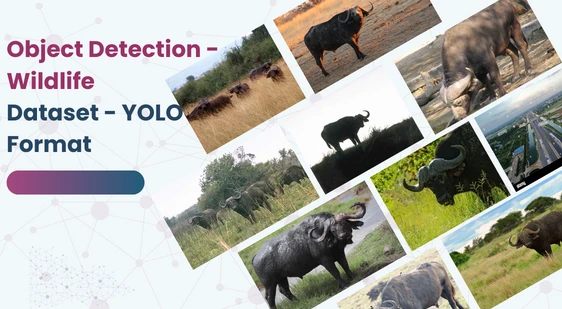
About Dataset
Creating a high-quality dataset for YOLO-based object detection involves compiling 1500 images across four distinct classes: buffalo, elephant, rhino, and zebra. Each image undergoes meticulous preprocessing to ensure optimal conditions for model training. The core of this preparation involves annotating each image with bounding boxes and corresponding class labels in the YOLO format, which includes the class ID and normalized bounding box coordinates.
To facilitate effective model training, validation, and testing, the dataset is strategically divided into three subsets. Specifically, 1200 images are allocated for training, 150 images for validation, and 150 images for testing, maintaining an 8:1:1 ratio. This careful division ensures a balanced and comprehensive dataset, crucial for achieving high performance in object detection tasks.
The training set, comprising the bulk of the images, is essential for the model to learn and generalize the features of each class. By exposing the model to a diverse set of training examples, we ensure it can recognize and accurately detect buffalo, elephant, rhino, and zebra in various conditions and contexts. The validation set plays a critical role during the training process, providing a means to tune the model’s hyperparameters and make necessary adjustments without overfitting. Regular evaluation on the validation set helps monitor the model’s performance and guide the training process.
This dataset is sourced from Kaggle.
Contact Us

Quality Data Creation

Guaranteed TAT

ISO 9001:2015, ISO/IEC 27001:2013 Certified

HIPAA Compliance

GDPR Compliance

Compliance and Security
Let's Discuss your Data collection Requirement With Us
To get a detailed estimation of requirements please reach us.