Lacuna Malaria Detection Challenge Dataset
Home » Dataset Download » Lacuna Malaria Detection Challenge Dataset
Lacuna Malaria Detection Challenge Dataset
Datasets
Lacuna Malaria Detection Challenge Dataset
File
Lacuna Malaria Detection Challenge Dataset
Use Case
Lacuna Malaria Detection Challenge Dataset
Description
Explore the Blood Slide Image Dataset for Malaria Diagnosis, featuring high-resolution images captured with smartphones for rapid malaria detection in low-resource settings
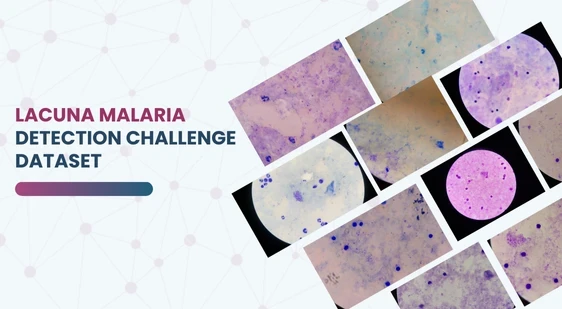
Description:
This dataset is designed to enhance malaria diagnosis in low-resource settings using Computer Vision and Machine Learning. It consists of high-resolution blood slide images captured with a smartphone positioned over a microscope. Each image is accompanied by detailed metadata, including microscope settings like the objective lens and stage micrometer readings, ensuring accurate analysis.
Dataset Overview
- Total Images: Up to 40 images per blood slide, capturing a diverse range of data.
- Image Capture: Smartphone images taken by placing the phone over the microscope to capture the Field of View (FOV) of the blood slide.
- Microscope Data: Each image includes critical information, such as the objective lens and stage micrometer readings, for proper analysis.
- Source: This dataset complements existing malaria microscopy datasets and focuses on low-resource settings like Uganda.
Key Features:
- High-Quality Images: Smartphone-captured images offering detailed views suitable for AI-powered malaria detection.
- Comprehensive Annotations: Images come with important metadata, including microscope settings, for better context.
- Designed for Low-Resource Settings: Specifically intended for regions with limited access to advanced medical equipment, making it easier to apply machine learning models in such environments.
- Generalizable Data: While focused on Uganda, the dataset aims to improve the generalization of machine learning models for other regions facing similar challenges.
Applications:
- Malaria Diagnosis: Perfect for training Computer Vision models to quickly and accurately identify malaria from blood slide images.
- AI Model Development: Assists researchers in developing machine learning models that work in diverse regions with varying data conditions.
- Public Health Research: Supports studies aiming to advance malaria detection and improve public health technologies for low-resource environments.
Data Processing:
- Field of View (FOV): Images are captured by positioning the smartphone camera directly over the microscope, ensuring precise and consistent FOV images.
- Metadata: Alongside each image, the stage micrometer readings and objective lens settings are included to provide valuable context.
- Consistency: A maximum of 40 images per blood slide ensures a diverse dataset for training accurate machine learning models, reducing model biases.
This dataset is sourced from Kaggle.
Contact Us

Quality Data Creation

Guaranteed TAT

ISO 9001:2015, ISO/IEC 27001:2013 Certified

HIPAA Compliance

GDPR Compliance

Compliance and Security
Let's Discuss your Data collection Requirement With Us
To get a detailed estimation of requirements please reach us.