HDSNE Chest X-ray Dataset
Home » Dataset Download » HDSNE Chest X-ray Dataset
HDSNE Chest X-ray Dataset
Datasets
HDSNE Chest X-ray Dataset
File
HDSNE Chest X-ray Dataset
Use Case
HDSNE Chest X-ray Dataset
Description
Explore the HDSNE Chest X-ray dataset, designed to eliminate duplication and optimize medical image aggregation for accurate diagnosis of lung infections like pneumonia and COVID-19.
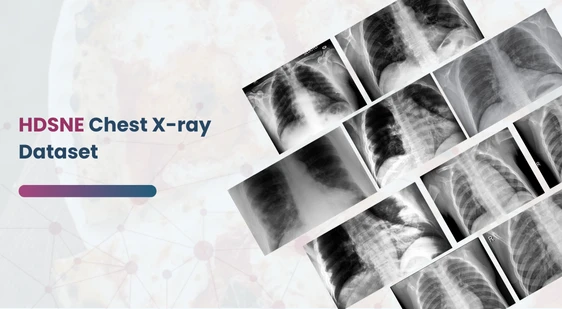
Description:
The continuous release of medical image databases, often featuring overlapping or identical categories, poses a significant challenge for the development of autonomous Computer-Aided Diagnostics (CAD) systems. These systems are essential for creating truly comprehensive medical diagnostics. However, one of the main obstacles lies in the frequent bulk release of datasets, which commonly suffer from two critical issues: image duplication and data corruption.
The Problem of Dataset Redundancy
Repeated releases of the same categories often fail to integrate or deduplicate similar images across databases, which can severely impact the effectiveness of machine learning models. Data duplication not only reduces the efficiency of learning models but also leads to overfitting, wastes computational resources, and increases the carbon footprint due to the energy required for training complex models.
Download Dataset
Proposed Solution: Global Data Aggregation Model
In response to these challenges, we introduce a global data aggregation model that intelligently combines data from six distinct and reputable medical imaging databases. Each database was carefully curated to ensure the elimination of redundancies while preserving data diversity. Two robust algorithms were employed:
- Hash MD5 Algorithm: This algorithm generates unique hash values for each image, helping in the effective detection and elimination of duplicate images.
- t-SNE Algorithm: This technique is used for dimensionality reduction, with a tunable perplexity parameter to ensure accurate representation of high-dimensional data.
Dataset Categories
The final dataset includes an equal number of samples from three key categories of chest X-ray images:
- Normal
- Pneumonia
- COVID-19
This uniform distribution ensures that the dataset is balanced, avoiding class imbalance—a common issue that can skew results in medical image analysis.
Dataset Application & Model Evaluation
The dataset was applied to the Inception V3 pre-trained model, a leading convolutional neural network (CNN) architecture known for its excellence in image classification tasks. The evaluation was conduct using the following performance metrics:
- Accuracy: An exceptional accuracy rate of 98.48% was achieve.
- Precision, Recall, and F1-score: The dataset showed strong performance across these metrics, reducing both false positives and false negatives.
- Statistical Validation: A t-test was conduct to validate the results, and the t-values and p-values confirm the statistical significance of the model’s performance.
Conclusion
The HDSNE Chest X-ray Dataset offers a novel and effective approach to data aggregation, tackling the issues of redundancy and data duplication that have long plagued the field of medical imaging. By maintaining a balance class distribution and eliminating unnecessary data, this dataset provides a cleaner and more efficient resource for training machine learning models.
This dataset is sourced from Kaggle.
Contact Us

Quality Data Creation

Guaranteed TAT

ISO 9001:2015, ISO/IEC 27001:2013 Certified

HIPAA Compliance

GDPR Compliance

Compliance and Security
Let's Discuss your Data collection Requirement With Us
To get a detailed estimation of requirements please reach us.