HaGRID – HAnd Gesture Recognition Image Dataset
Home » Dataset Download » HaGRID – HAnd Gesture Recognition Image Dataset
HaGRID – HAnd Gesture Recognition Image Dataset
Datasets
HaGRID - HAnd Gesture Recognition Image Dataset
File
HaGRID - HAnd Gesture Recognition Image Dataset
Use Case
Gesture Recognition
Description
The HaGRiD (Hand Gesture Recognition Image Dataset) is a collection of images and data designed for the development and evaluation of hand gesture recognition systems. Hand gesture recognition is a technology used in human-computer interaction, sign language recognition, and various other applications.
Description:
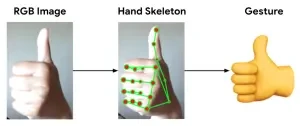
We introduce a large image dataset HaGRID (HAnd Gesture Recognition Image Dataset) for hand gesture recognition (HGR) systems. You can use it for image classification or image detection tasks. Proposed dataset allows to build HGR systems, which can be used in video conferencing services (Zoom, Skype, Discord, Jazz etc.), home automation systems, the automotive sector, etc.
HaGRID size is 716GB and dataset contains 552,992 FullHD (1920 × 1080) RGB images divided into 18 classes of gestures. Also, some images have no_gesture
class if there is a second free hand in the frame. This extra class contains 123,589 samples. The data were split into training 92%, and testing 8% sets by subject user-id, with 509,323 images for train and 43,669 images for test.
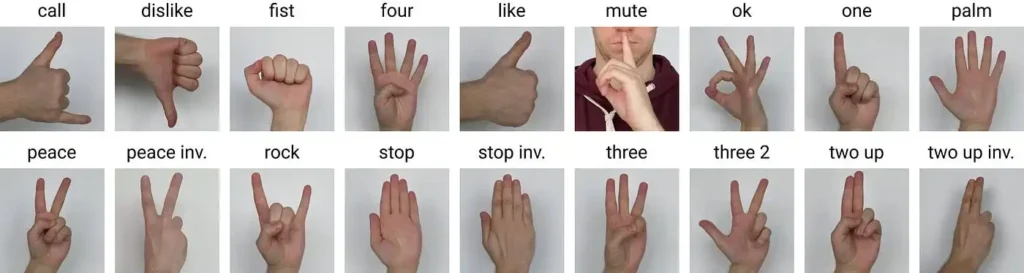
The subjects are people from 18 to 65 years old. The dataset was collected mainly indoors with considerable variation in lighting, including artificial and natural light.
Annotations
[top left X position, top left Y position, width, height]
. Also, annotations have 21 landmarks
in format [x,y]
relative image coordinates, markups of leading hands
(left
of right
for gesture hand) and leading_conf
as confidence for leading_hand
annotation. We provide user_id
field that will allow you to split the train / val dataset yourself.
"0534147c-4548-4ab4-9a8c-f297b43e8ffb": {
"bboxes": [
[0.38038597, 0.74085361, 0.08349486, 0.09142549],
[0.67322755, 0.37933984, 0.06350809, 0.09187757]
],
"landmarks"[
[
[
[0.39917091, 0.74502739],
[0.42500172, 0.74984396],
...
],
[0.70590734, 0.46012364],
[0.69208878, 0.45407018],
...
],
],
"labels": [
"no_gesture",
"one"
],
"leading_hand": "left",
"leading_conf": 1.0,
"user_id": "bb138d5db200f29385f..."
}
Download
Download and unzip our train dataset split into 18 archives by gestures. Get started with the links below
Trainval
GestureSize Gesture Size
call 39.1 GBpeace 38.6 GB
dislike 38.7 GBpeace_inverted 38.6 GB
fist 38.0 GB rock 38.9 GB
four 40.5 GB stop 38.3 GB
like 38.3 GB stop_inverted 40.2 GB
mute 39.5 GB three 39.4 GB
ok 39.0 GB three2 38.5 GB
one 39.9 GB two_up 41.2 GB
palm 39.3 GB two_up_inverted 39.2 GBtrain_val
annotations: ann_train_val
Test
Test Archives Size
images test 60.4 GB
annotations ann_test 27.3 MB
It has 100 items per gesture.
Archives Size
images subsample 2.5 GB
annotations ann_subsample 1.2 МB
Models
We provide some pre-trained classifiers and detectors as the baseline solutions. You can download them via github repo.
Links
Conclusion
Globose Technology Solutions excels in hand gesture recognition, utilizing the HaGRID dataset for precise identification. Our advanced algorithms contribute to innovative applications in human-computer interaction and virtual reality, reflecting our commitment to cutting-edge gesture recognition technology.
Download the dataset now!
Contact Us

Quality Data Creation

Guaranteed TAT

ISO 9001:2015, ISO/IEC 27001:2013 Certified

HIPAA Compliance

GDPR Compliance

Compliance and Security
Let's Discuss your Data collection Requirement With Us
To get a detailed estimation of requirements please reach us.