Butterfly Image Classification
Home » Dataset Download » Butterfly Image Classification
Butterfly Image Classification
Datasets
Butterfly Image Classification
File
Image Classification Dataset
Use Case
Image Classification
Description
The dataset features 75 different classes of Butterflies. The dataset contains about 1000+ labelled images including the validation images.
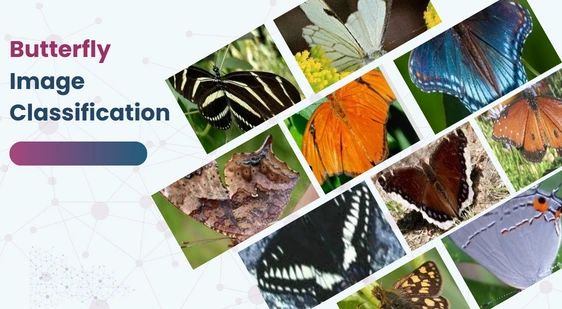
About Dataset
The dataset features 75 different classes of Butterflies. The dataset contains about 1000+ labelled images including the validation images. Each image belongs to only one butterfly category.
The label of each image are saved in Training_set.csv.
The Testing_set.csv contains names of image in test folder, which you need to predict the label and submit to Data Sprint 107 –
Techniques and Algorithms Of Butterfly Image Classification
Convolutional Neural Networks (CNNs): Foundation of Image Recognition
Transfer Learning: Leveraging Pre-trained Models
Transfer learning plays a pivotal role in Butterfly Image Classification by leveraging pre-trained CNN models like ResNet, VGG, and Inception.Â
Data Augmentation: Enhancing Model Robustness
 By artificially expanding the training dataset, these techniques mitigate overfitting and improve the generalization capability of the AI model across diverse butterfly species and image conditions.
Applications of Butterfly Image Classification
Conservation Biology: Monitoring Species Populations
In conservation biology, data aids in monitoring changes in butterfly populations and habitats. By automating species identification from camera trap images or field photographs, conservationists can track population trends, assess biodiversity hotspots, and prioritize conservation efforts effectively.
Citizen Science Initiatives: Engaging the Public
Citizen science projects data utilize to engage the public in monitoring and documenting butterfly diversity.Â
Educational Tools: Inspiring Future Generations
Educational institutions and nature centers utilize Butterfly Image Classification as an educational tool to inspire curiosity about biodiversity and technology among students. By showcasing the intersection of AI and ecology, these tools encourage STEM education and foster a deeper appreciation for the natural world.
Challenges and Future Directions
Data Quality and Quantity
One of the primary challenges in Butterfly Image Classification is the availability of high-quality, well-annotated datasets encompassing diverse butterfly species and geographical regions. Addressing this challenge requires collaborative efforts to collect, curate, and share open-access datasets to support research and development in AI.
Ethical Considerations
As AI technologies advance, ethical considerations surrounding data privacy, bias in algorithms, and responsible use of AI in ecological research become increasingly important. Implementing transparent and ethical practices ensures that Butterfly Image Classification contributes positively to conservation efforts without compromising privacy or perpetuating biases.
Conclusion
 By harnessing the power of machine learning algorithms, researchers and conservationists can streamline species identification processes, engage the public in scientific endeavors, and pave the way for innovative solutions to biodiversity challenges.
This dataset is sourced from Kaggle.
Contact Us

Quality Data Creation

Guaranteed TAT

ISO 9001:2015, ISO/IEC 27001:2013 Certified

HIPAA Compliance

GDPR Compliance

Compliance and Security
Let's Discuss your Data collection Requirement With Us
To get a detailed estimation of requirements please reach us.