Acne Dataset in YOLOv8 Format
Home » Dataset Download » Acne Dataset in YOLOv8 Format
Acne Dataset in YOLOv8 Format
Datasets
Dataset in YOLOv8 Format
File
Dataset in YOLOv8 Format
Use Case
healthcare
Description
The dataset is in YOLOv8 format. The dataset is divided into train, validation and test. Data replication processes were also applied.
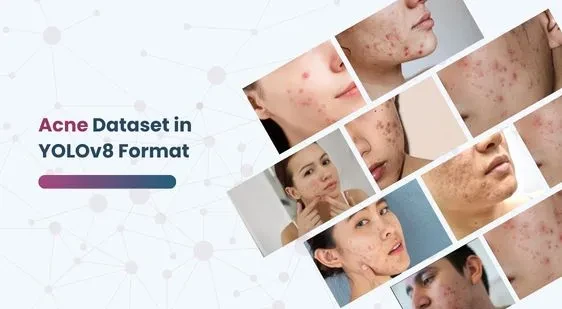
About Dataset
The Dataset includes 927 image files, annotated using Roboflow and structured specifically for YOLOv8, a model designed for advanced computer vision tasks. It is organized into three segments: training, validation, and testing. Each segment has been meticulously prepared to ensure a balanced and comprehensive representation of the data. Consequently, this careful organization facilitates the training process and significantly enhances the model’s performance.
The training set includes a substantial portion of the dataset, thereby providing the necessary variety and volume of images to train the YOLOv8 model effectively. For instance, this set contains numerous examples of different data types, allowing the model to learn and generalize from diverse inputs. Consequently, the training phase benefits from the robust and extensive nature of the data, which is crucial for developing a high-performing model.
Dataset
The validation set plays a critical role in the model development process because it helps fine-tune the model’s parameters and ensures it does not overfit the training data. By evaluating the model’s performance on the validation set, we can make necessary adjustments to improve accuracy and generalization. Consequently, the validation set is an essential component for achieving a well-balanced model.
The testing set plays a crucial role in assessing a model’s performance in real-world scenarios. Notably, this set remains isolated from the training and validation processes, thereby providing an unbiased evaluation of the model’s capabilities. By testing the model on unseen data, we can, therefore, gauge its effectiveness and reliability in practical applications. Additionally, the testing set ensures that the model can handle new and unforeseen data types, thus confirming its robustness. Consequently, this process validates the model’s ability to perform well in diverse and unpredictable situations.
Preprocessing
- Auto-Orient: Applied
- Resize: Stretch to 640×640
Augmentations
- Outputs per training example: 3
- 90° Rotate: Clockwise, Counter-Clockwise
- Saturation: Between -20% and +20%
- Blur: Up to 1.5px
Contact Us

Quality Data Creation

Guaranteed TAT

ISO 9001:2015, ISO/IEC 27001:2013 Certified

HIPAA Compliance

GDPR Compliance

Compliance and Security
Let's Discuss your Data collection Requirement With Us
To get a detailed estimation of requirements please reach us.