Visual question-answering tasks
Home » Case Study » Visual question-answering tasks
Project Overview:
Objective
Our mission was to assemble a vast dataset specifically designed for visual question-answering (VQA) tasks, which is crucial for the advancement of AI in both computer vision and natural language processing. Consequently, this dataset serves as a testament to our expertise in gathering and annotating various data types. Therefore, it is essential for developing state-of-the-art AI models.
Scope
We began by gathering a large collection of images, each accompanied by well-crafted questions. Furthermore, every element was annotated with precise text-based answers, thus creating a strong VQA dataset.
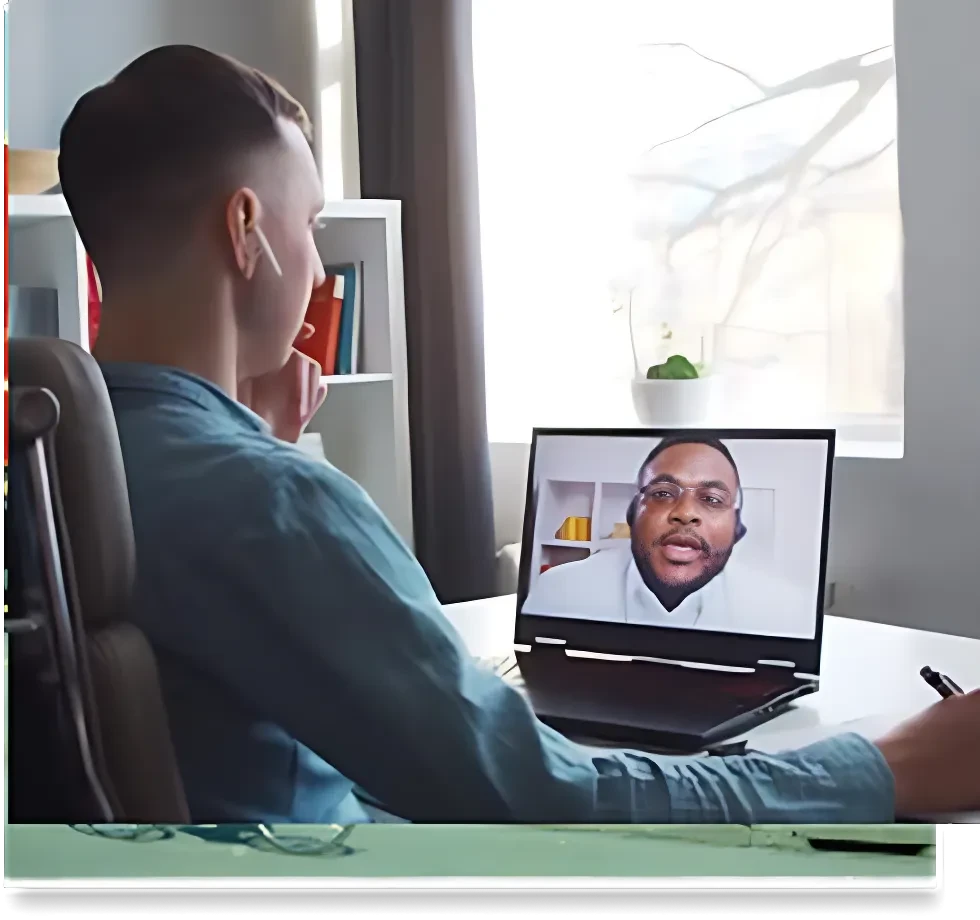
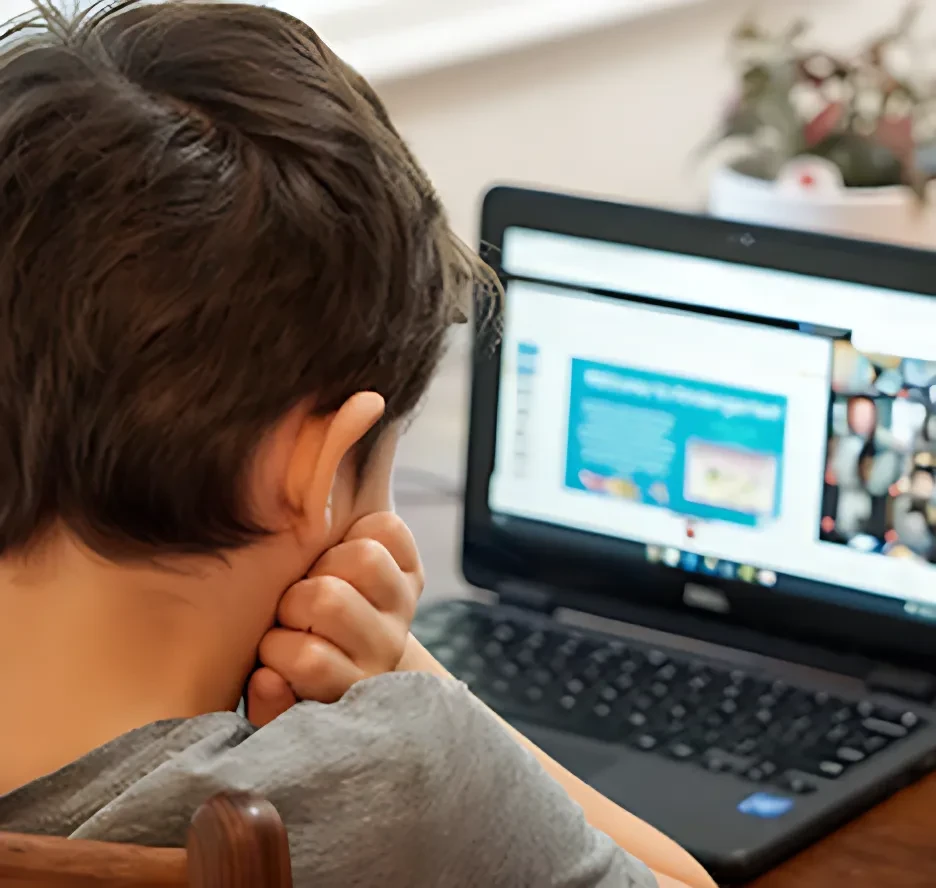
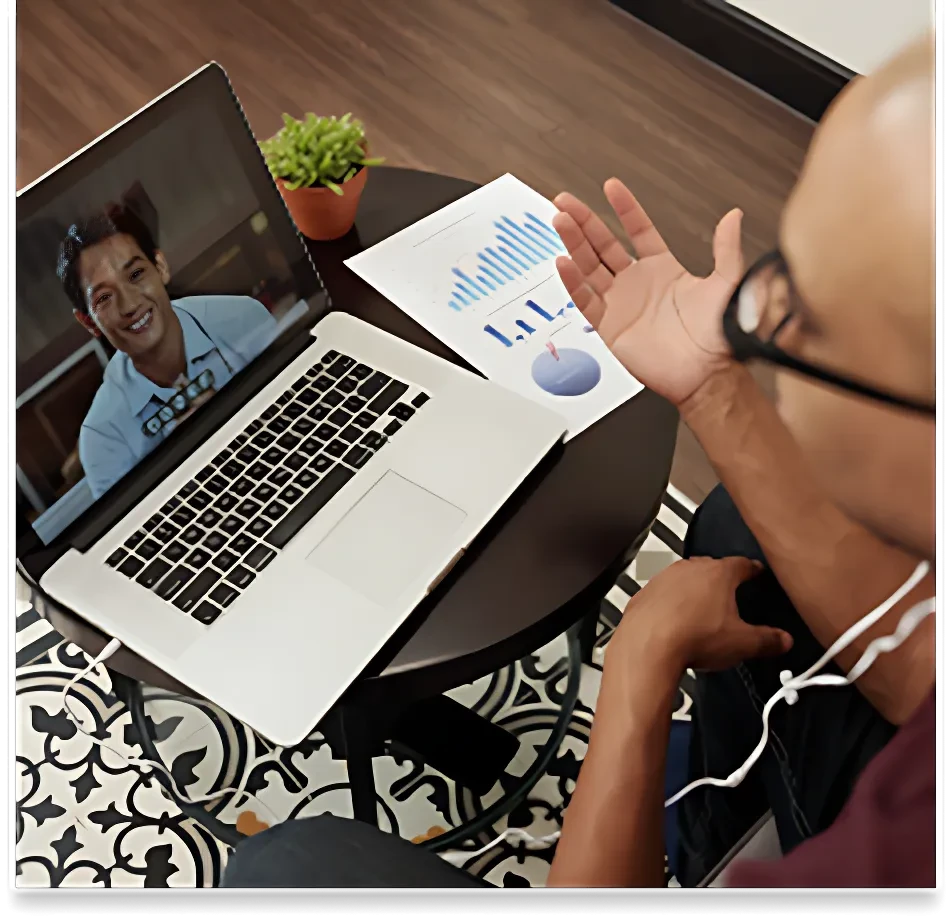
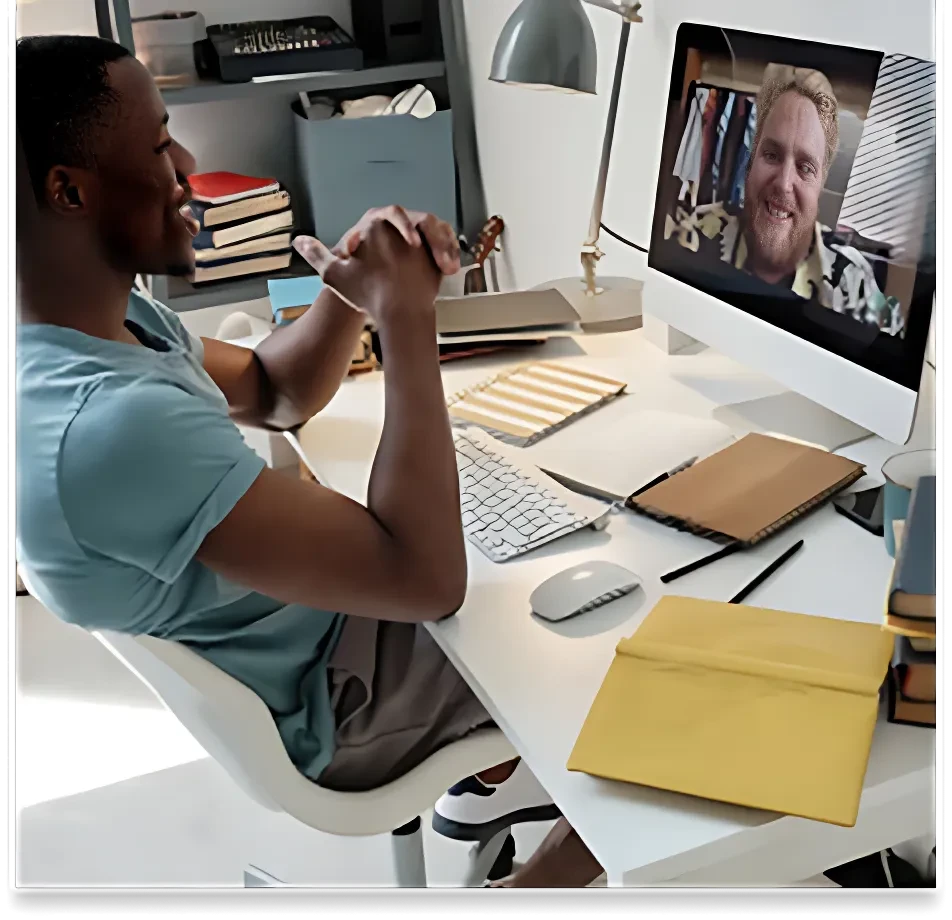
Sources
- Image Databases: We obtained a large collection of images from various sources, such as public image databases and licensed image providers. As a result, we have a diverse set of visual data to work with.
- Question Generation: We created a wide range of questions related to the images. This can be done through crowdsourcing or using automatic question-generation techniques. By using both methods, we ensured a comprehensive and varied set of questions.
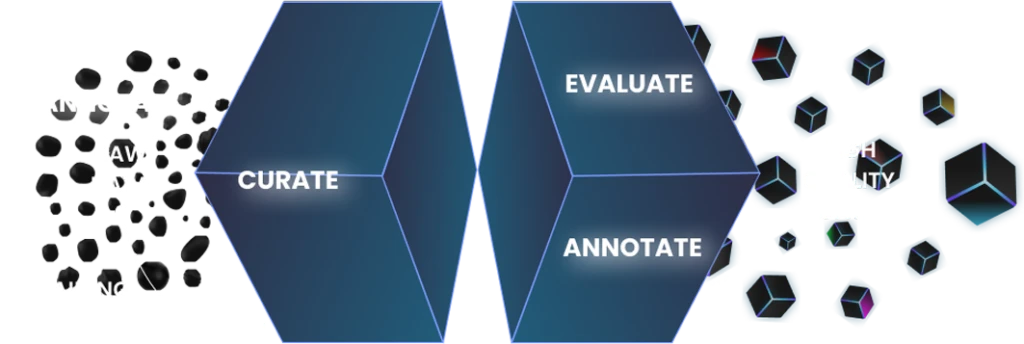
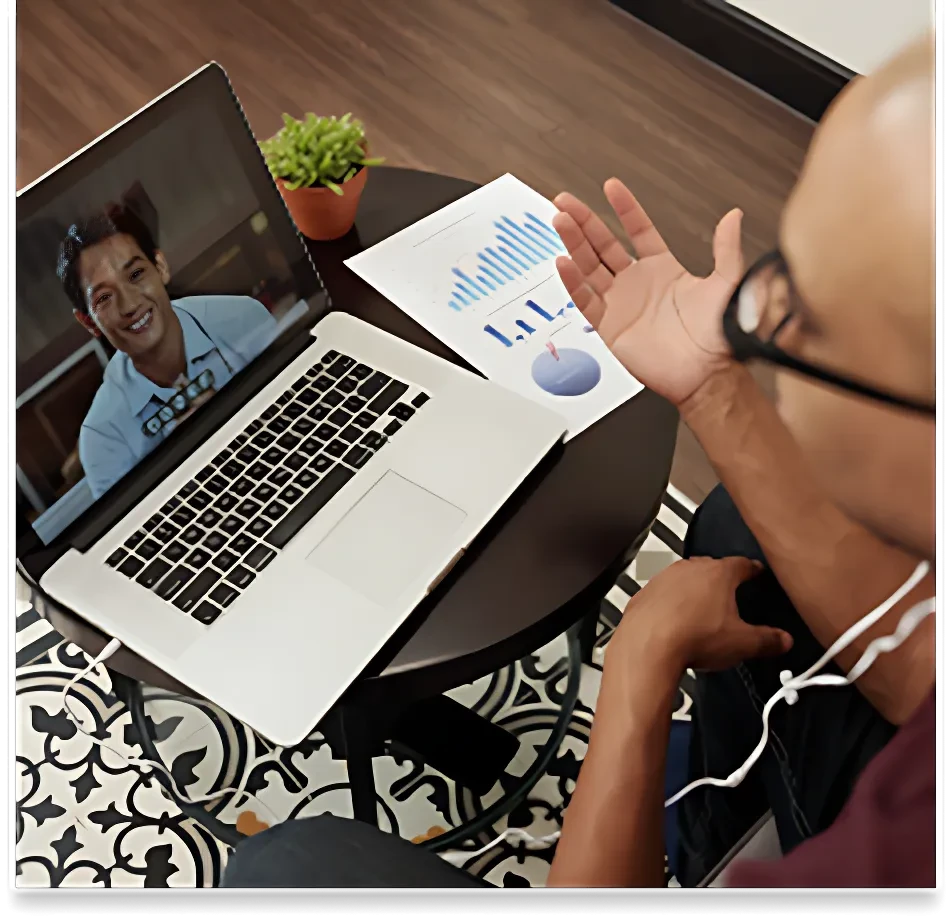
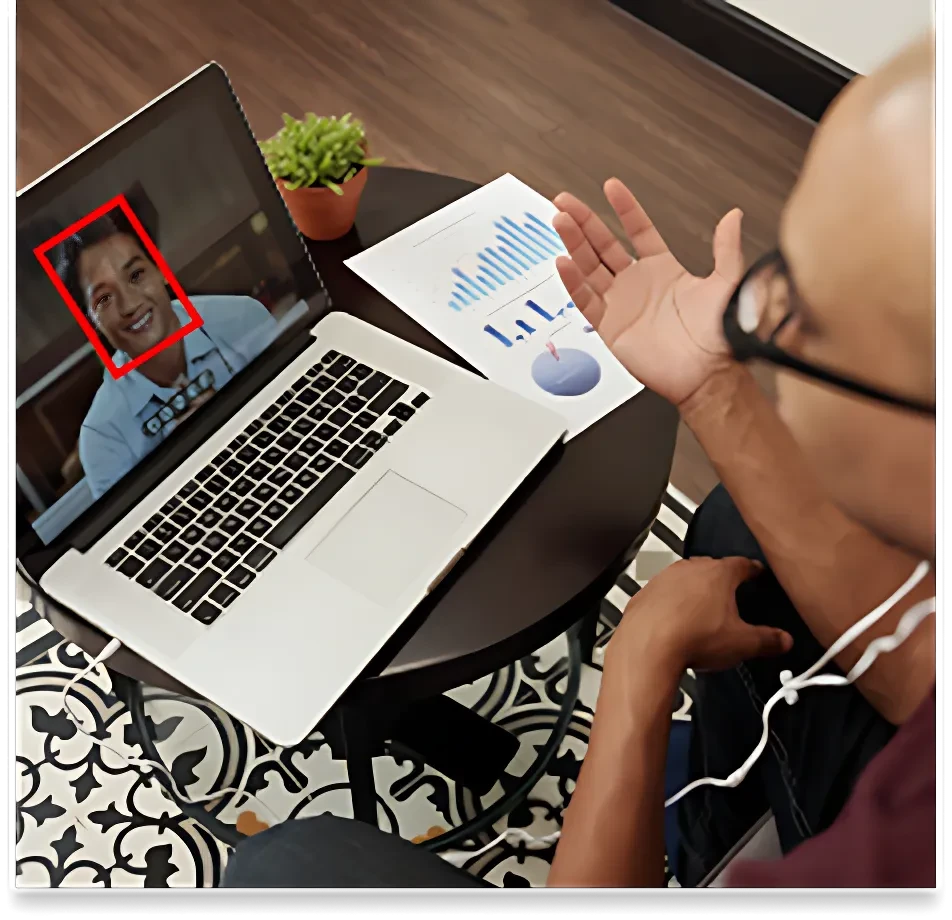
Data Collection Metrics
- Total Images Collected: 120,000
- Questions Generated: 360,000
- Data Annotation:Â Every question is matched with a relevant, annotated answer.
Annotation Process
Stages
- Question-Image Pairing: Aligning each question with a corresponding image involves ensuring that each visual representation accurately reflects the subject of the question. This alignment process covers a wide array of topics and complexities. By doing so, we ensure that the visual context enhances the understanding of the question.
- Answer Annotation: Providing detailed, text-based answers for each question is essential. These annotations must be suitable for various VQA applications. Additionally, they should be clear, concise, and accurate to support diverse users effectively.
- Diversity Control: Ensuring a rich mix of image contents, question types, and answer formats is crucial. By including a variety of topics and visual contexts, we can cater to a broader audience and use case scenarios.
- Quality Control: Upholding high standards in question relevance and answer accuracy is paramount. Thus, each question and answer pair should be meticulously reviewed to maintain consistency and reliability.
Annotation Metrics
- Question-Image Pairs: 150,000 pairs
- Text-Based Answers: 150,000 answers
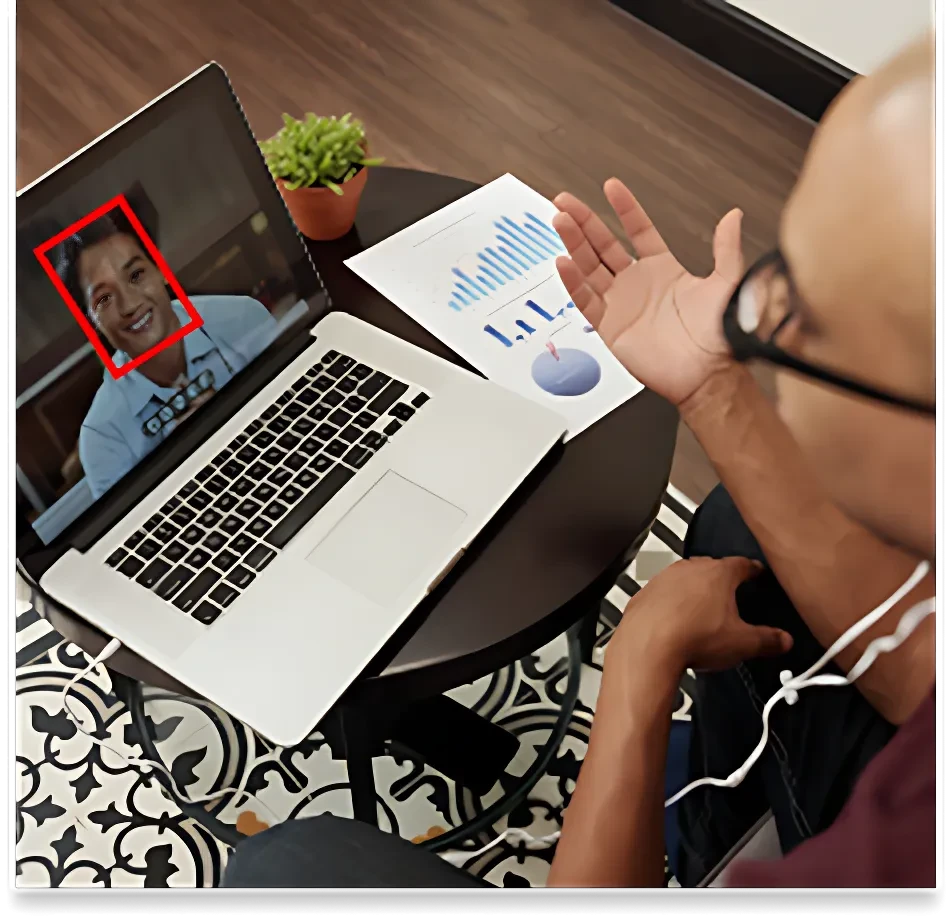
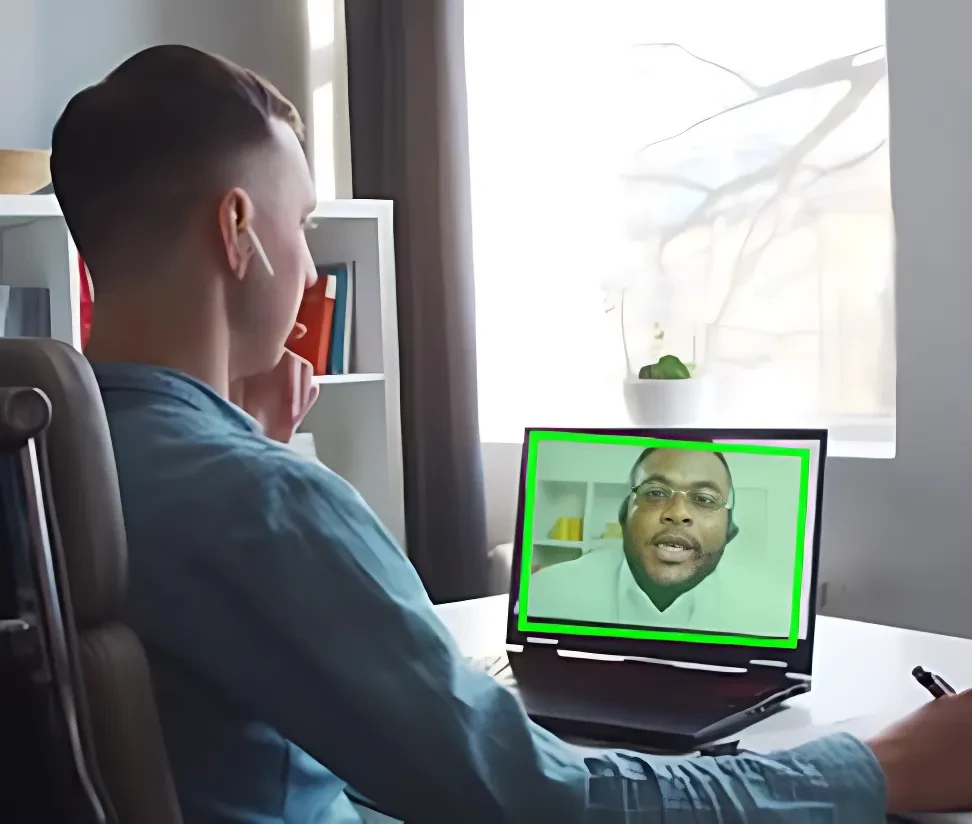
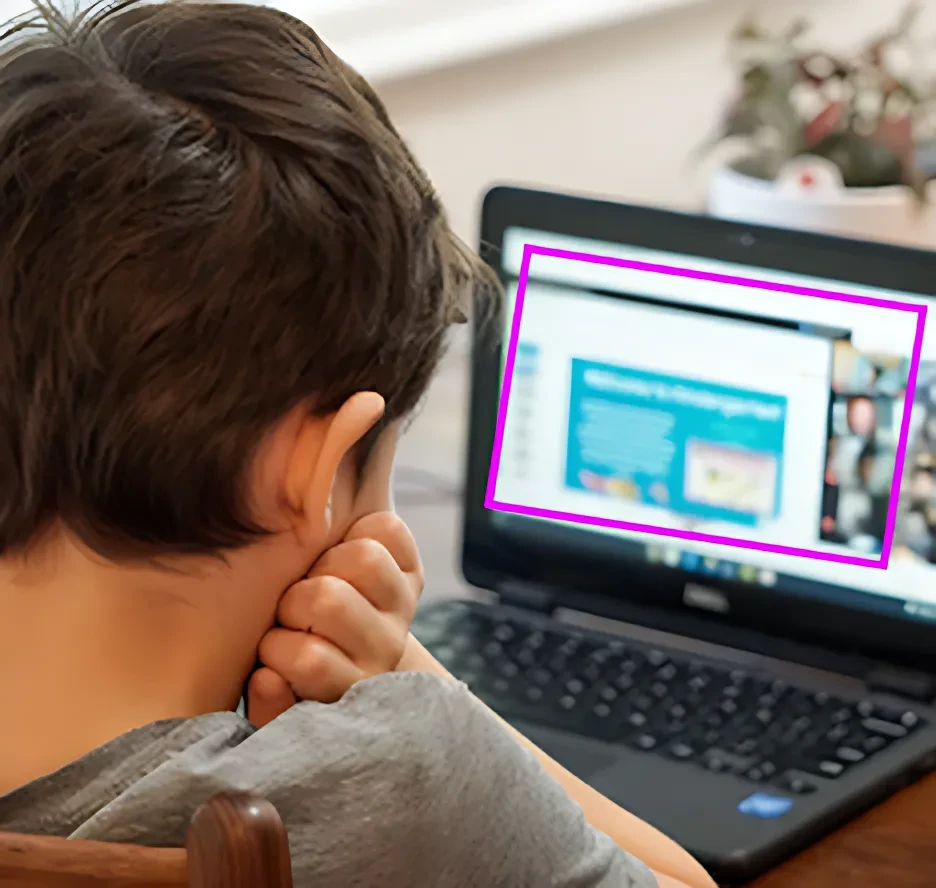
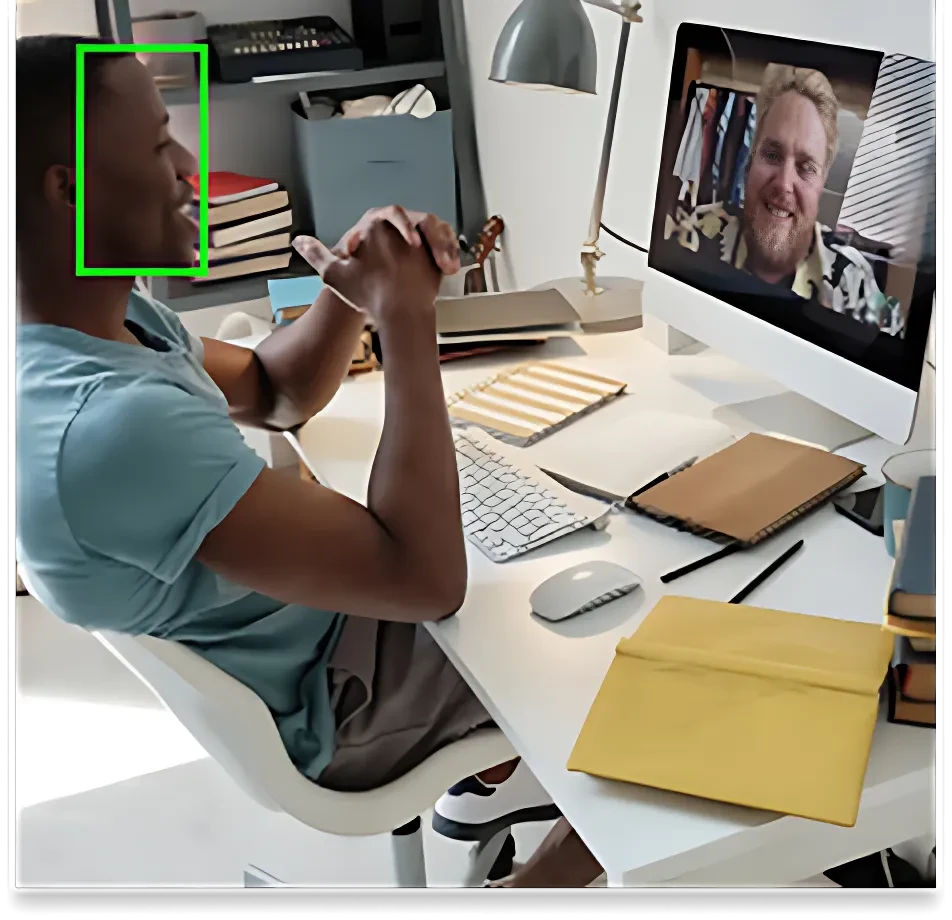
Quality Assurance
Stages
- Annotation Verification: We use strict human review processes to check the relevance of questions and answers. As a result, we ensure accuracy.
- Privacy Compliance: We follow strict privacy laws when handling image content and sensitive data. Thus, we respect and protect individual privacy rights.
- Data Security: We use strong measures to keep personal and sensitive information safe. Consequently, we safeguard your data effectively.
QA Metrics
- Annotation Validation Cases: 10,000 (randomly selected from the dataset)
- Privacy Audits: Ongoing to ensure compliance
Conclusion
This project demonstrates our skill not only in collecting but also in adding useful annotations to datasets. Furthermore, our varied datasets, which include images, videos, texts, and speech, are carefully curated to train advanced AI models. Additionally, we take pride in our human-centered approach, making sure that each dataset we produce is not only large but also high in quality and relevance.

Quality Data Creation

Guaranteed TAT

ISO 9001:2015, ISO/IEC 27001:2013 Certified

HIPAA Compliance

GDPR Compliance

Compliance and Security
Let's Discuss your Data collection Requirement With Us
To get a detailed estimation of requirements please reach us.