HUMAN-BOT CONVERSATIONS
Home » Case Study » HUMAN-BOT CONVERSATIONS
Project Overview:
Objective
The goal of this project is to refine and enhance the interaction between humans and conversational bots. By collecting and annotating diverse conversation datasets, moreover, we aim to develop bots that can understand and respond to human language more accurately and naturally. Additionally, through this process, we intend to foster a deeper level of engagement and improve the overall user experience. Furthermore, by analyzing various conversational patterns and nuances, we can identify areas for improvement and implement targeted enhancements.
Scope
The project entails the advancement of sophisticated NLP (Natural Language Processing) models adept at comprehending and generating human-like responses in conversations across diverse contexts. These include customer service, personal assistance, and informal chats.
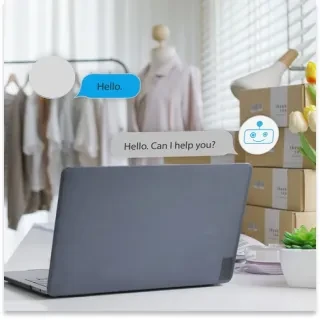
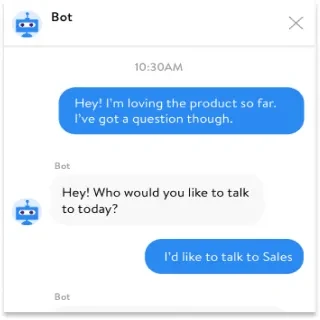
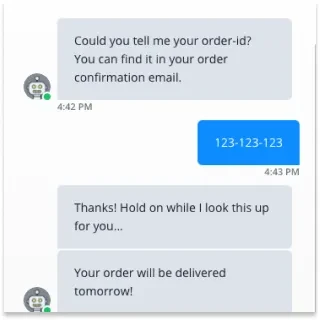
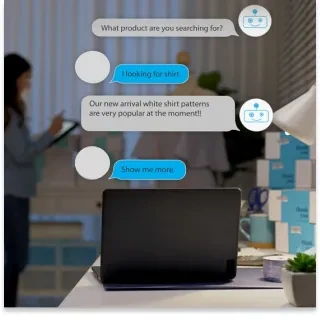
Sources
- Scanned documents (e.g., reports, books, forms).
- Street signs and billboards.
- Handwritten notes.
- Printed receipts and invoices.
- Digital displays and screens.
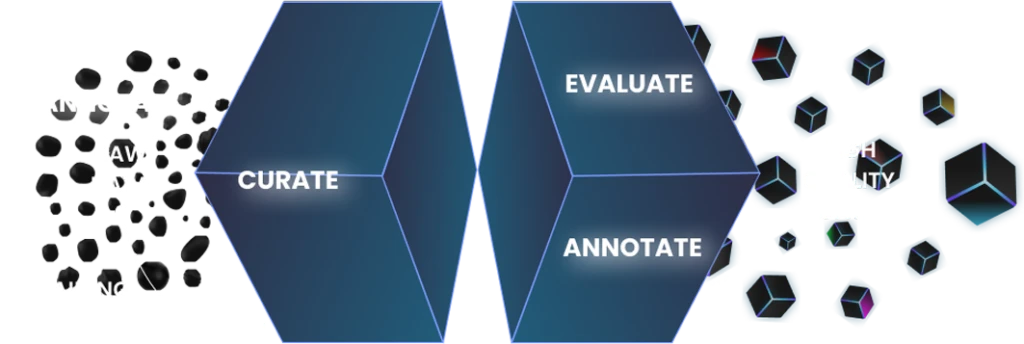
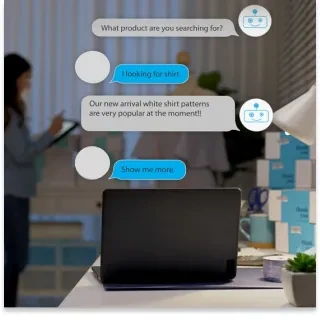
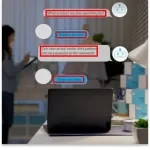
Data Collection Metrics
- Total Data Points: 15000 individual conversation instances were collected.
- Data Diversity: Conversations span a wide array of topics, tones, and complexities, encompassing informal chats, formal dialogues, customer service interactions, and more.
Annotation Process
Stages
- Data Categorization: Classify conversations based on context, sentiment, and intent.
- Language Annotation: Additionally, Language Annotation entails annotating linguistic features such as syntax, semantics, and pragmatics to enhance understanding of human language.
- Response Formulation: Furthermore, Response Formulation involves developing templates for bot responses that are contextually appropriate and linguistically accurate.
Annotation Metrics
- Conversational Accuracy: Evaluate bots’ ability to respond accurately and contextually in conversations.
- User Engagement Rate: Assess user engagement and satisfaction with bot interactions.
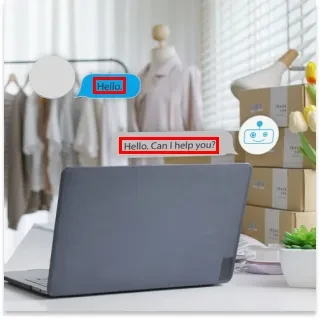
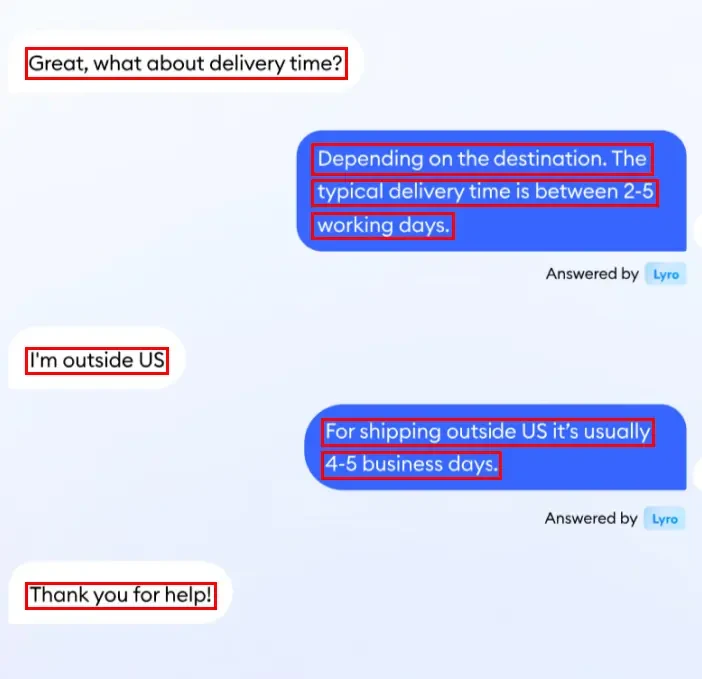
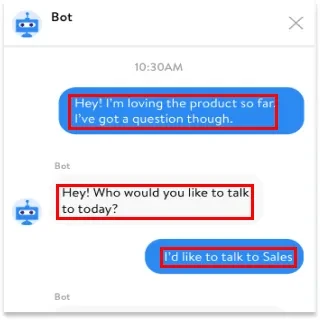
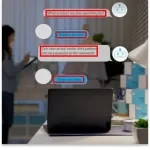
Quality Assurance
Stages
Expert Linguist Review:Â Linguists and conversation analysts review a subset of bot conversations for linguistic and contextual accuracy.
User Feedback Incorporation: Additionally, they incorporate user feedback and adapt models to changing conversational trends.
Â
QA Metrics
- Reviewed Conversation Instances: 15% of all collected conversations are thoroughly reviewed.
- Improvement in Engagement: Track enhancements in user engagement and satisfaction over time. Additionally, monitor shifts in user behavior to gauge improvements. Moreover, assess user feedback regularly to identify areas for enhancement. Furthermore, analyze user interactions with the platform to pinpoint any trends or patterns. Lastly, utilize surveys and metrics to quantify changes in user satisfaction.
Conclusion
The Human-Bot Conversations project is crucial for advancing the field of AI and machine learning. Specifically, by meticulously collecting and annotating conversation data, we are pushing the boundaries of how machines understand and engage in human language. Consequently, this effort leads to more seamless and productive human-bot interactions.

Quality Data Creation

Guaranteed TAT

ISO 9001:2015, ISO/IEC 27001:2013 Certified

HIPAA Compliance

GDPR Compliance

Compliance and Security
Let's Discuss your Data collection Requirement With Us
To get a detailed estimation of requirements please reach us.