Textual Entailment Dataset – Stanford Natural Language Inference
Home » Case Study » Textual Entailment Dataset – Stanford Natural Language Inference
Project Overview:
Objective
The objective is to develop a robust dataset that aids in improving the performance of natural language understanding systems, particularly in the task of textual entailment. This involves determining the logical relationship between pairs of sentences, such as whether one sentence entails, contradicts, or is neutral to the other.
Scope
The dataset includes lots of pairs of sentences that talk about different things in different ways. This helps it capture all the little details of how language works in real life.
Sources
- Crowdsourced Annotations: Data is collected through crowdsourcing platforms, where annotators assess the relationship between sentence pairs based on their semantic content.
- Text Corpora: Existing text corpora are utilized to extract diverse sentence pairs, ensuring a comprehensive coverage of linguistic phenomena and discourse patterns.
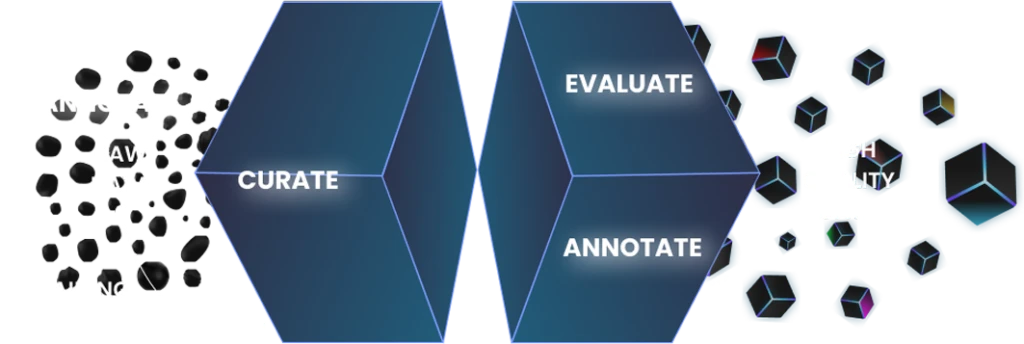
Data Collection Metrics
- Total Data Collected: 500,000 sentence pairs.
- Data Annotated for ML Training: 450,000 sentence pairs with detailed labels for machine learning training and evaluation.
Annotation Process
Stages
- Labeling Scheme: People who annotate the data decide if the sentences in each pair are related in different ways, like if one sentence proves or disproves the other, or if they don’t relate at all.
- Quality Control: We make sure the labeling process is accurate and consistent by regularly checking if different annotators agree on the labels and by holding calibration sessions to keep everyone on the same page.
Annotation Metrics
- Labeling Accuracy: The dataset achieves a high level of accuracy in labeling sentence pairs, with inter-annotator agreement exceeding 90%.
- Diversity of Labels: The dataset includes a diverse range of logical relationships between sentence pairs, capturing various linguistic phenomena and semantic nuances.
Quality Assurance
Stages
Labeling Accuracy: The dataset achieves a high level of accuracy, with inter-annotator agreement exceeding 90%
Diversity of Labels: The dataset encompasses a wide range of logical relationships between sentence pairs, capturing various linguistic phenomena and semantic nuances.
QA Metrics
- Accuracy Testing: Regular evaluations ensure the accuracy and reliability of the dataset labels.
- Consistency Checks: Continuous monitoring guarantees consistency across annotations and minimizes discrepancies.
Conclusion
Creating the Stanford Natural Language Inference dataset is a big step forward in understanding how computers grasp language. It offers a huge collection of sentence pairs that are carefully labeled, making it super useful for teaching and testing machine learning models on tasks like understanding text connections. This dataset helps build smarter systems for tasks like answering questions, summarizing text, and having conversations, making them more accurate and reliable.

Quality Data Creation

Guaranteed TAT

ISO 9001:2015, ISO/IEC 27001:2013 Certified

HIPAA Compliance

GDPR Compliance

Compliance and Security
Let's Discuss your Data collection Requirement With Us
To get a detailed estimation of requirements please reach us.