Text Summarization for Research Papers
Home » Case Study » Text Summarization for Research Papers
Project Overview:
Objective
We’re on a mission to craft a rich dataset that will let AI models smartly condense research papers, making academic discoveries easier to grasp. Our project, “Text Summarization for Research Papers”, is on a mission to create an awesome dataset. This tool will teach machine learning models how to craft succinct yet meaningful summaries of research papers from all academic disciplines – it’s like finding the express lane straight to the key points of any scholarly write-up!
Scope
However, we need to analyze research papers from different fields, summarize the key information accurately, and annotate them to capture the main objectives, methods, results, and conclusions. This project’s all about digging up research papers from various academic fields, pulling out the important bits, and then boiling them down into clear-cut summaries that nail the main goals of the research, how they did it, what they found out, and what it all means.
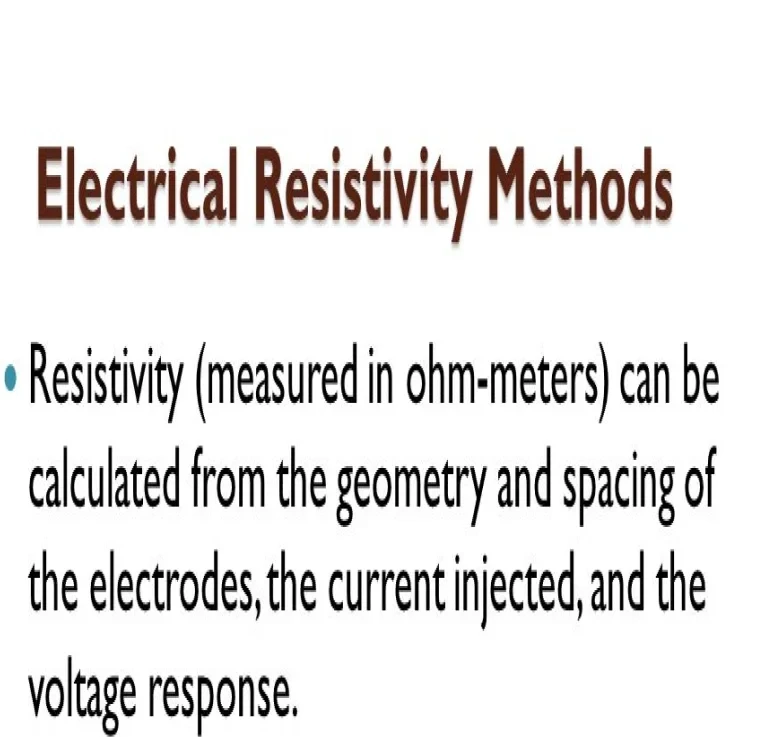
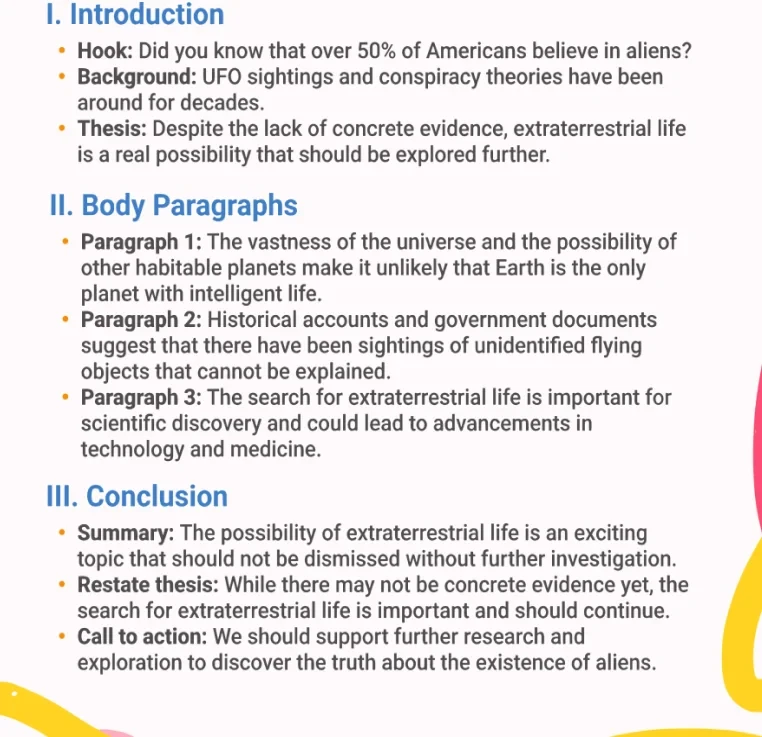
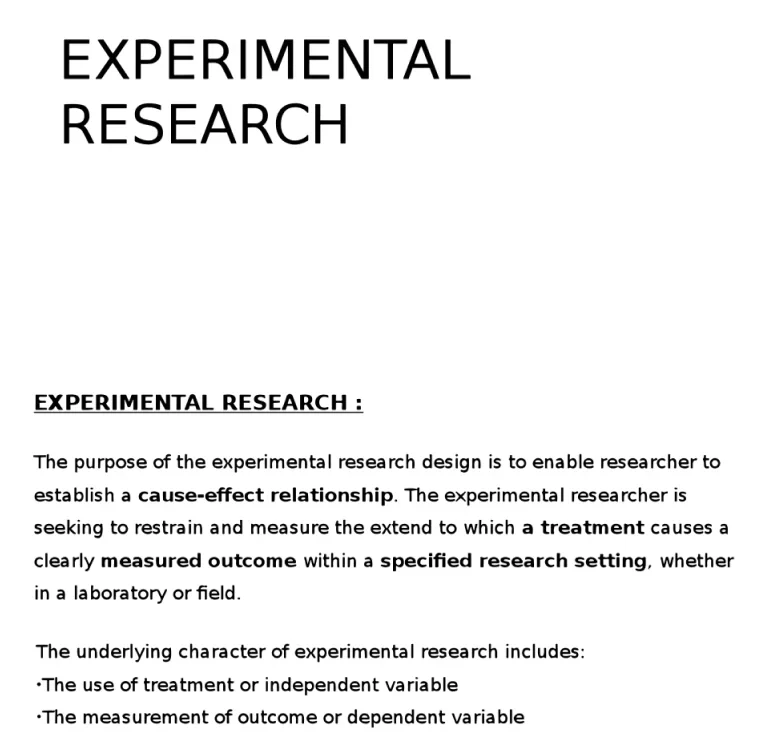
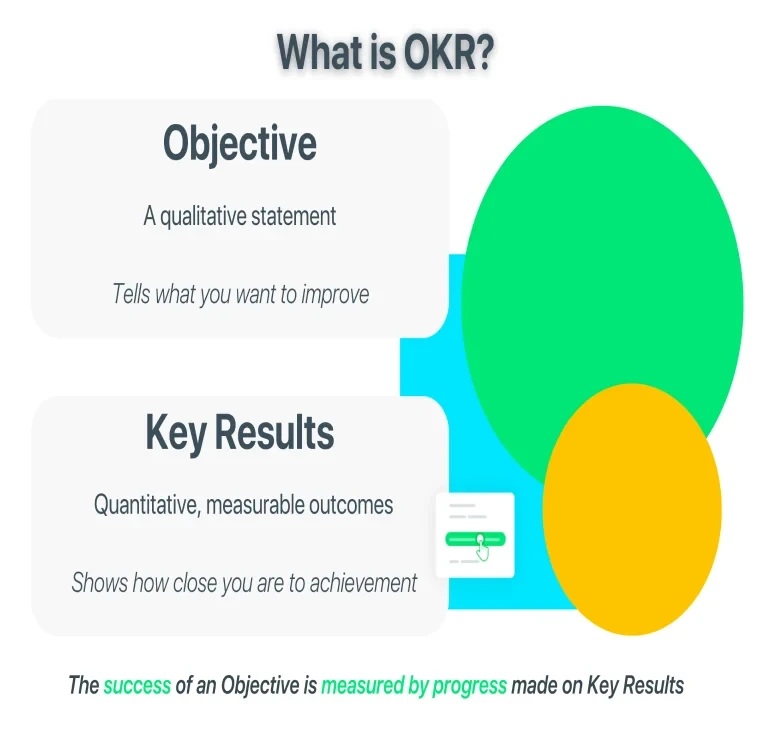
Sources
- Academic Journals: Gather research papers from renowned academic journals spanning a wide range of subjects, including science, engineering, social sciences, and humanities.
- Conferences: Access papers from prestigious conferences in various fields, such as computer science, medicine, and economics.
- Open Access Repositories: Utilize publicly available research papers from open-access repositories and institutional websites.
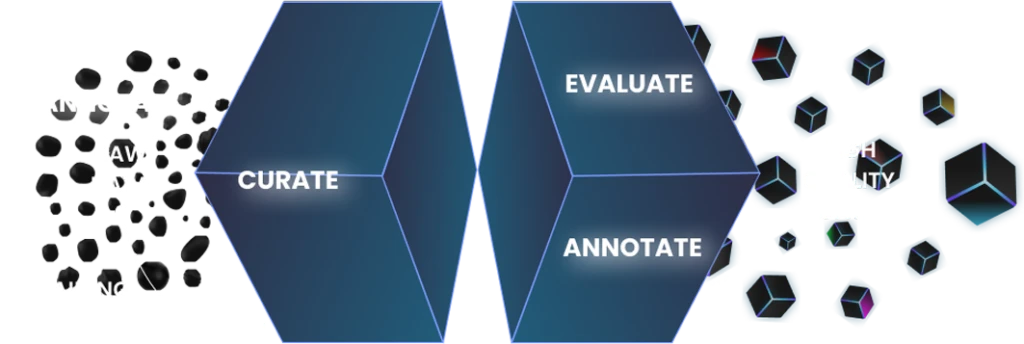
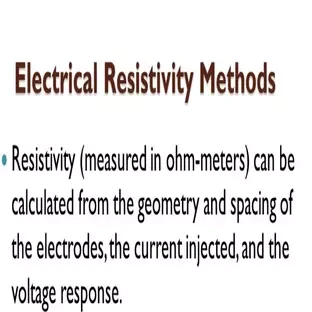
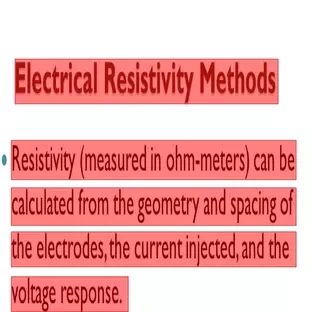
Data Collection Metrics
- Total Research Papers for Summarization: 20,000 papers
- Academic Journals: 10,000
- Conferences: 5,000
- Open Access Repositories: 5,000
Annotation Process
Stages
- Text Summarization: Annotate each research paper with a concise summary that distills the main research objectives, methods, findings, and conclusions.
- Metadata Logging: Log metadata, including paper title, authors, publication date, keywords, and citation information.
Annotation Metrics
- Research Papers with Summaries: 20,000
- Metadata Logging: 20,000
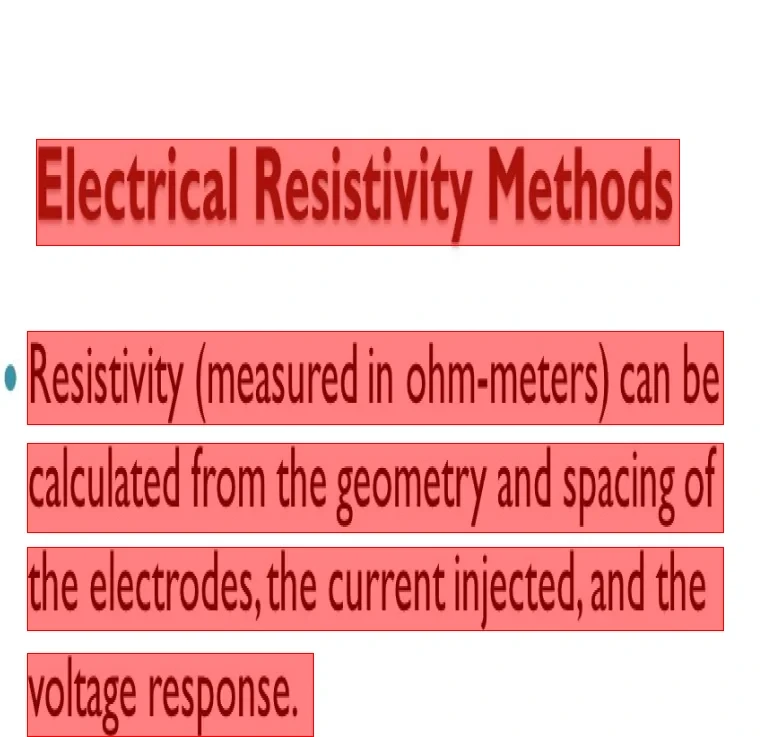
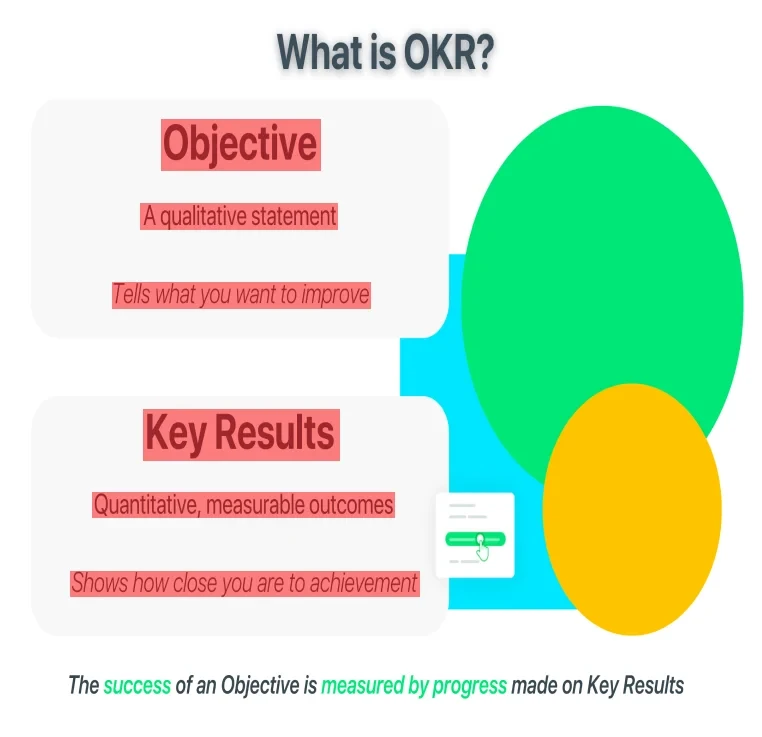
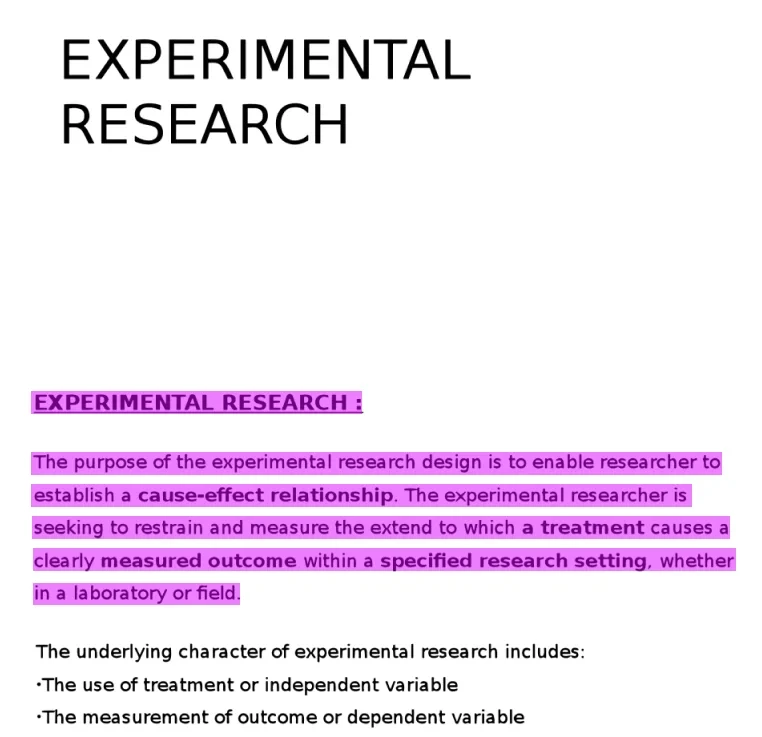
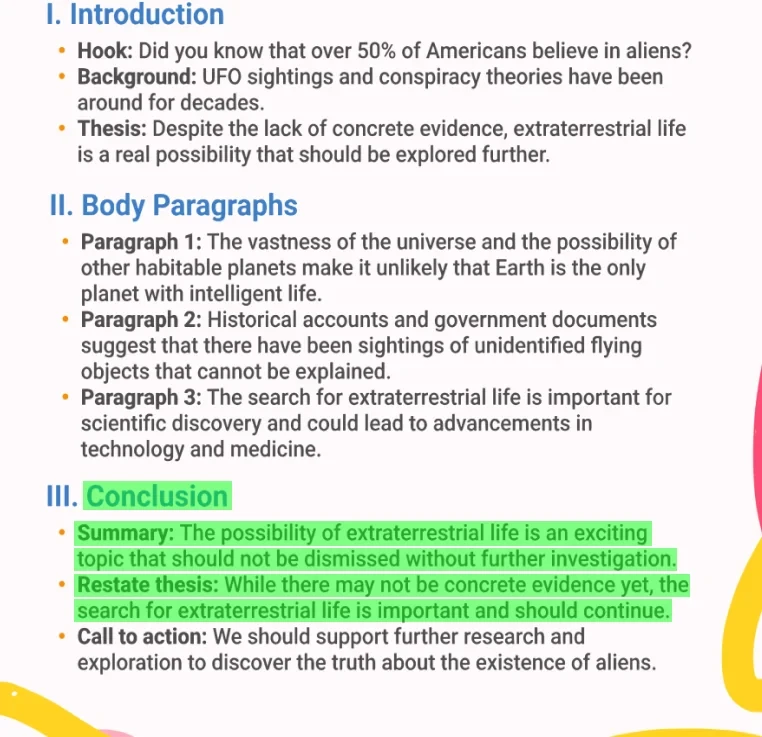
Quality Assurance
Stages
Annotation Verification: Implement a validation process involving subject matter experts to review and verify the accuracy and comprehensiveness of the research paper summaries.
Data Quality Control: Ensure the removal of papers with poor quality content, irrelevant information, or those that fall outside the project’s scope.
Data Security: Protect sensitive research data and adhere to copyright and licensing regulations.
QA Metrics
- Annotation Validation Cases: 2,000 (10% of total)
- Data Cleansing: Remove poor-quality or irrelevant research papers
Conclusion
The “Text Summarization for Research Papers” dataset is a crucial resource for researchers, students, and professionals across various academic disciplines. This dataset, with its meticulously labeled research papers and in-depth metadata, sparks the creation of cutting-edge text summary tools that not only simplify our study methods but also boost how we share knowledge from a broad spectrum of academic work. This dataset is like a goldmine for building kick-ass text summarization tools, making research work smoother and boosting literature reviews. It’s a game-changer in opening doors to key findings from tons of academic papers, pushing scholarly progress forward, and encouraging cross-field teamwork.

Quality Data Creation

Guaranteed TAT

ISO 9001:2015, ISO/IEC 27001:2013 Certified

HIPAA Compliance

GDPR Compliance

Compliance and Security
Let's Discuss your Data collection Requirement With Us
To get a detailed estimation of requirements please reach us.