Street-Level House Numbers Dataset – Street View House Numbers
Home » Case Study » Street-Level House Numbers Dataset – Street View House Numbers
Project Overview:
Objective
The Street-Level House Numbers Dataset serves as a crucial resource for developing advanced AI tools aimed at assisting farmers in early pest detection and effective management. In creating the Pest Detection Dataset, our goal was to forge a comprehensive resource for the identification and classification of agricultural pests and diseases. This dataset is meticulously designed to be the cornerstone for developing AI tools that enhance crop health and yield by aiding farmers in early pest detection and efficient management practices.
Scope
The dataset encompasses a wide range of urban and suburban landscapes, thereby capturing diverse environmental conditions. Furthermore, it includes various lighting variations and architectural styles. These factors ensure robust model performance across different scenarios.
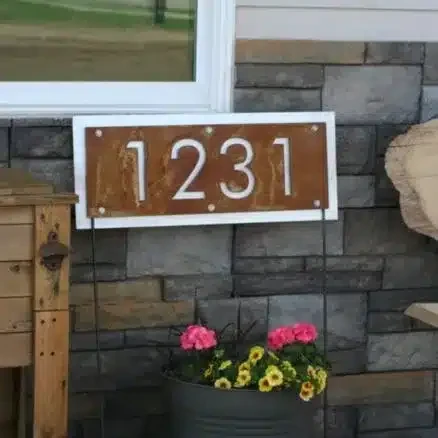
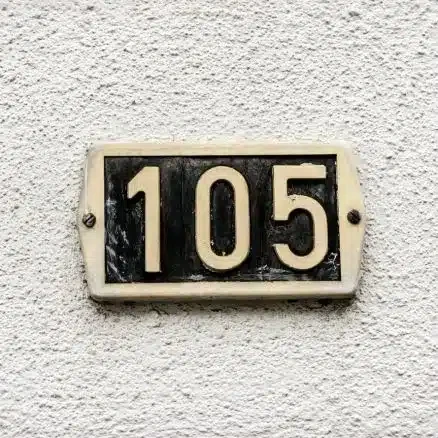
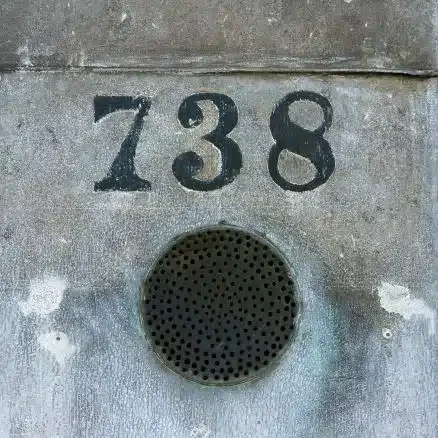
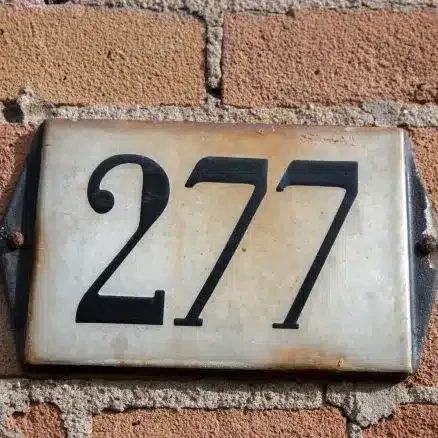
Sources
- Street View Images: We collected a large volume of images containing street-level house numbers from different locations across India utilizing Google Street View and other street imaging platforms.
- Manual Photography: Furthermore, in addition to automated data sources, we manually captured images of house numbers in various neighborhoods and cities to ensure a comprehensive dataset representation.
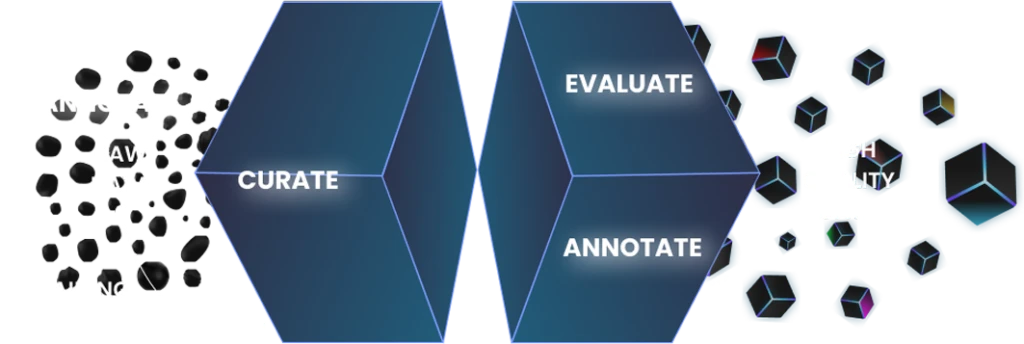
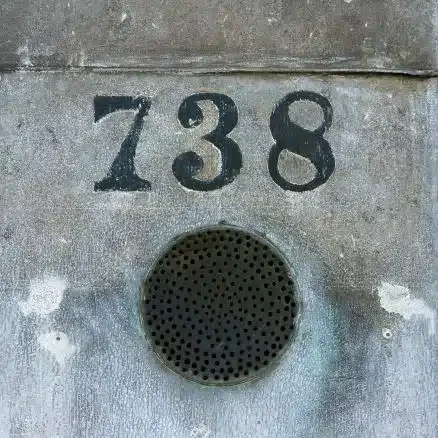
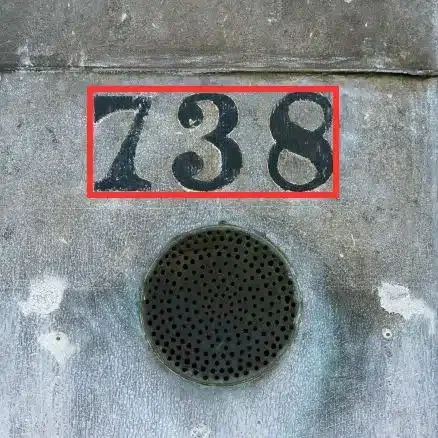
Data Collection Metrics
- Total Data Collected: 50,000 high-resolution images containing street-level house numbers.
- Data Annotated for ML Training: 60,000 images with meticulously labeled house numbers for machine learning training purposes.
Annotation Process
Stages
House Number Extraction: The annotation team carefully analyzed each image to extract and label individual house numbers, ensuring accurate identification and localization through this meticulous process.
Annotation Metrics
- Number of Annotations: The annotation team achieved success by annotating 60,000 images, encompassing 120,000 house numbers, marking a significant milestone. This accomplishment also showcases the team’s proficiency and dedication.
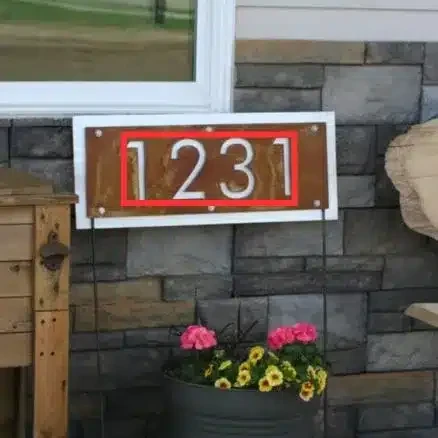
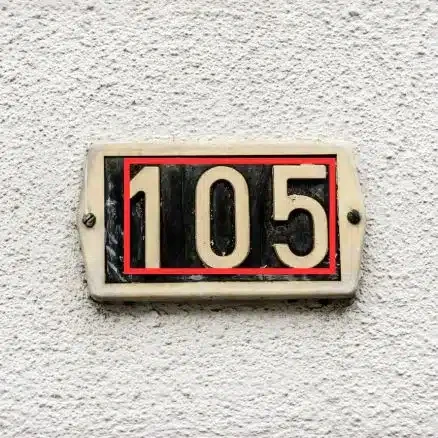
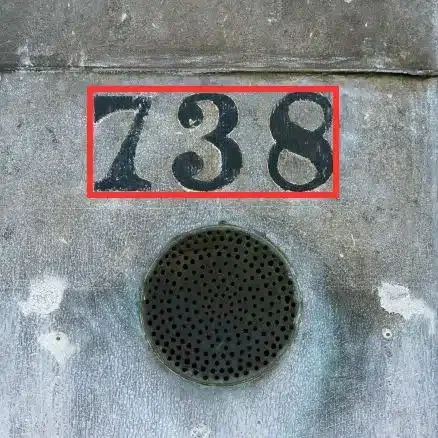
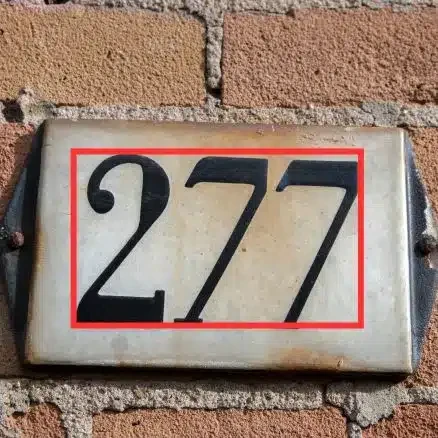
Quality Assurance
Stages
Dataset Validation: To begin with, we conducted thorough validation procedures to confirm the accuracy and consistency of annotations throughout the dataset. Subsequently, ensuring its reliability and quality, we meticulously scrutinized each annotation. Moreover, we implemented stringent checks to validate the integrity of the dataset, thus bolstering its credibility.
Privacy Protection: Secondly, Stringent measures were adopted to anonymize sensitive data, prioritizing compliance with relevant data protection regulations and safeguarding individuals’ privacy.
Improvement Process: Lastly, Feedback mechanisms were established to continuously refine the annotation process, aiming to enhance the overall quality and effectiveness of the dataset.
QA Metrics
- Annotation Accuracy: Achieved a high annotation accuracy rate of 98% for house number identification and localization.
- Privacy Compliance: we also maintained full compliance with privacy regulations, ensuring the protection of individuals’ privacy rights.
Conclusion
The Street View House Numbers (SVHN) dataset signifies a noteworthy progression in computer vision research. Additionally, it provides a comprehensive and diverse collection suitable for training and assessing machine learning models designed for street-level house number recognition tasks. Consequently, this dataset is poised to play an instrumental role in improving the precision and dependability of automated systems utilized in urban navigation, postal services, and various related applications+

Quality Data Creation

Guaranteed TAT

ISO 9001:2015, ISO/IEC 27001:2013 Certified

HIPAA Compliance

GDPR Compliance

Compliance and Security
Let's Discuss your Data collection Requirement With Us
To get a detailed estimation of requirements please reach us.