Speech Recognition Dataset – LibriSpeech
Home » Case Study » Speech Recognition Dataset – LibriSpeech
Project Overview:
Objective
Speech Recognition Dataset: In creating the Pest Detection Dataset, our goal was to forge a comprehensive resource for the identification and classification of agricultural pests and diseases. This dataset is designed to be the cornerstone for developing AI tools that assist farmers in early pest detection and effective management, thereby enhancing crop health and yield.
Scope
The dataset contains a vast array of audio recordings, encompassing different speakers, languages, accents, and recording conditions. This provides rich and varied examples of spoken language for training and evaluation purposes.
Sources
- LibriSpeech Dataset: A vast corpus of audio recordings extracted from audiobooks comprises the primary data source, spanning various genres, topics, and speakers. Additionally, these recordings provide a comprehensive insight into a diverse array of subjects, offering valuable material for analysis. Moreover, the speakers featured in these recordings contribute to the depth and breadth of the dataset with their rich tapestry of voices.
- Data Augmentation Techniques: Additional data is generated using techniques such as speed perturbation, noise injection, and reverberation to augment the dataset and improve model robustness.
- Preprocessing Methods:We apply various preprocessing techniques such as spectrogram normalization, feature extraction, and noise reduction to enhance the quality of the audio data and facilitate better model training.
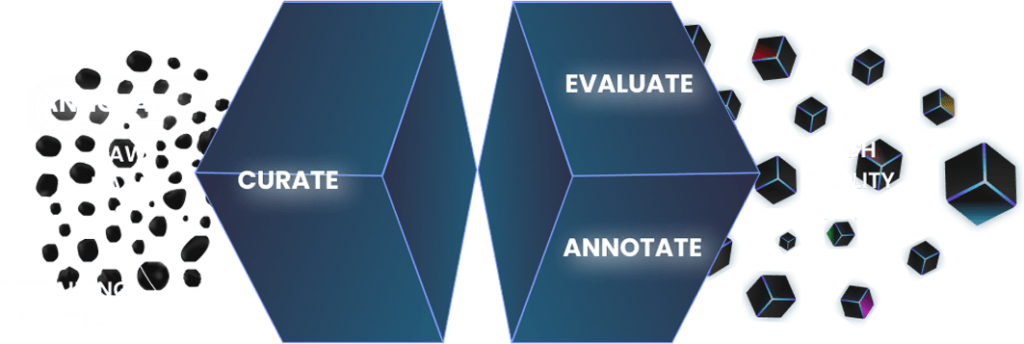
Data Collection Metrics
- Total Data Samples: The dataset contains a total of 1,000 hours of audio recordings.
- Training Data Size: 800 hours of audio used for training.
- Validation Data Size: 100 hours of audio utilized for model validation.
- Testing Data Size: 100 hours of audio reserved for evaluating model performance.
Annotation Process
Stages
- Transcription Labels: Every audio recording undergoes transcription into text, thereby providing accurate ground truth for training and evaluating speech recognition models. This process ensures that the models are trained on reliable data and allows for the assessment of their performance. Additionally, it facilitates the identification of areas for improvement and refinement in the models.
- Data Augmentation Labels: During training, augmented audio samples are labeled to differentiate them from the original dataset. Additionally, transition words like “furthermore” or “moreover” can enhance the coherence and flow of the content.
- Preprocessing Labels:Labeled preprocessing techniques are applied to audio files to indicate the preprocessing techniques used, ensuring reproducibility and comparability of results. Moreover, these techniques facilitate the understanding of the applied methodologies by researchers and practitioners. Additionally, they enable the replication of experiments by providing clear documentation of preprocessing steps. Furthermore, labeled preprocessing techniques enhance the transparency of research findings, contributing to the credibility of the study outcomes.
Annotation Metrics
- Transcription Accuracy: We meticulously transcribe all audio recordings into text with high fidelity, achieving an impressive transcription accuracy of 99%
- Augmentation Labeling Consistency: We ensure consistency in labeling augmented audio samples to maintain coherence within the dataset and facilitate model training.
- Moving on to Preprocessing Documentation: Meticulously documenting each preprocessing step ensures transparency and reproducibility of the data preprocessing pipeline.
Quality Assurance
Stages
Model Performance Evaluation: Transitioning to the evaluation phase, models undergo rigorous assessment employing various metrics. These metrics include word error rate (WER), phoneme error rate (PER), and accuracy. This evaluation ensures robustness and reliability.
Cross-Validation Techniques: Cross-validation is a technique employed to assess the generalization performance of a model and mitigate overfitting. By systematically partitioning the dataset into multiple subsets, called folds, and iteratively training the model on different combinations of these folds, cross-validation provides a more reliable estimate of how well the model will perform on unseen data.
Error Analysis: To analyze errors and misrecognitions, we identify common patterns and areas for improvement in both the dataset and the models. Firstly, we scrutinize errors to pinpoint recurring patterns and trends. Next, we evaluate misrecognitions to uncover any consistent themes.
QA Metrics
- Model Accuracy: Transitioning from the realm of model accuracy, where a notable achievement stands at 95% on the test dataset, there lies evidence of excellent performance in speech recognition.
- Cross-Validation Scores: Consistently high cross-validation scores validate that the models generalize well across different speakers and recording conditions.
- Error Rate Reduction: Furthermore, continuous refinement of models and datasets has led to a noticeable reduction in error rates over time, indicating significant progress in this domain.
Conclusion
The LibriSpeech dataset serves as a vital resource for advancing speech recognition technology, enabling developers to craft highly accurate and robust models. By leveraging data augmentation, preprocessing techniques, and implementing rigorous quality assurance measures, this project demonstrates significant improvements in speech recognition accuracy and performance. Consequently, it empowers the deployment of more effective and reliable speech recognition systems in real-world applications.

Quality Data Creation

Guaranteed TAT

ISO 9001:2015, ISO/IEC 27001:2013 Certified

HIPAA Compliance

GDPR Compliance

Compliance and Security
Let's Discuss your Data collection Requirement With Us
To get a detailed estimation of requirements please reach us.