Sentiment and Emotion Detection for Social LLMs
Project Overview:
Objective
The goal was to develop a dataset that improves LLMs’ empathy and response accuracy by annotating social media posts with both sentiment and specific emotions.
Scope
The dataset encompassed a wide range of social media posts from platforms like Twitter, Reddit, and Instagram, representing diverse topics and emotional tones. The posts were annotate to capture both the sentiment (positive, negative, neutral) and specific emotions (e.g., happiness, anger, sadness).
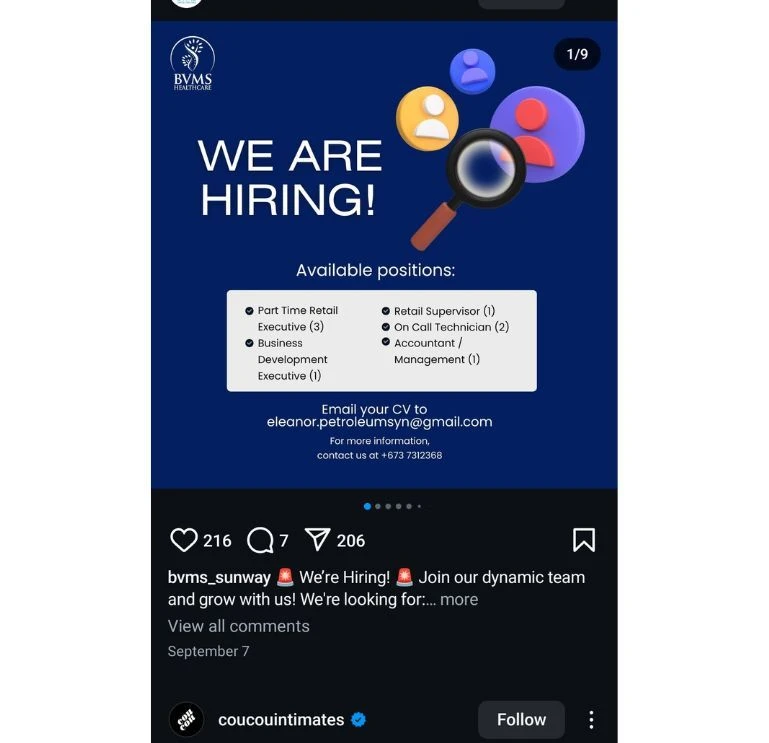
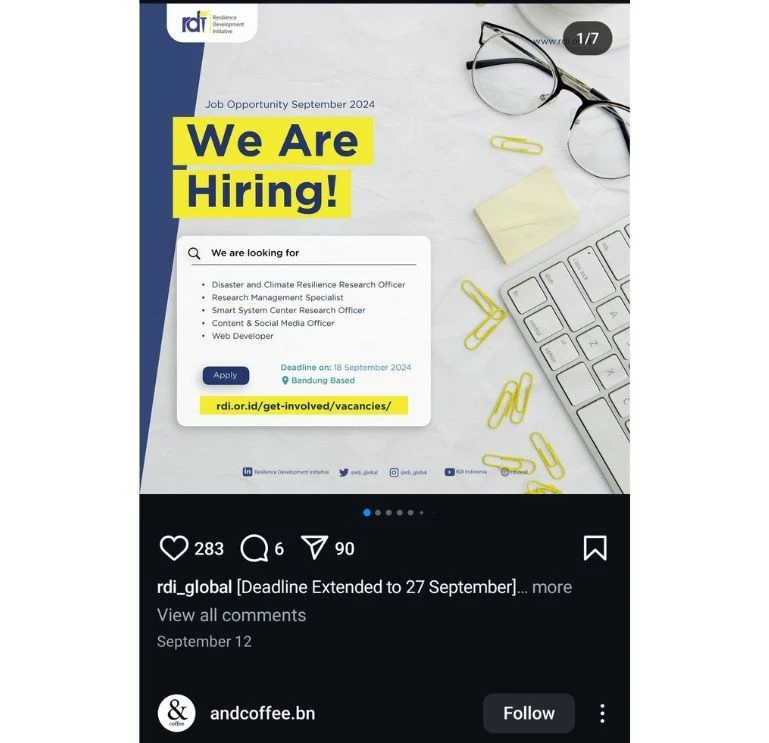
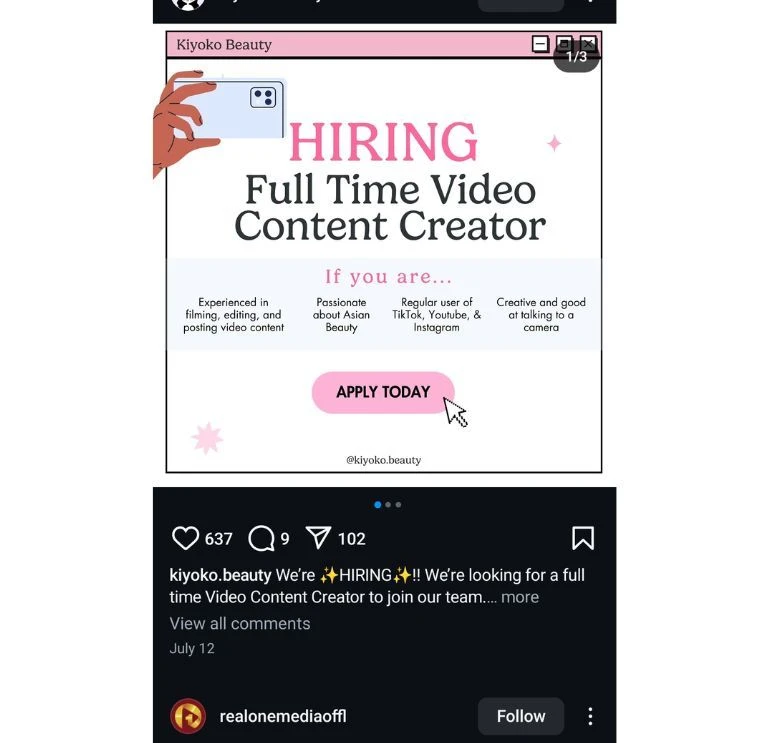
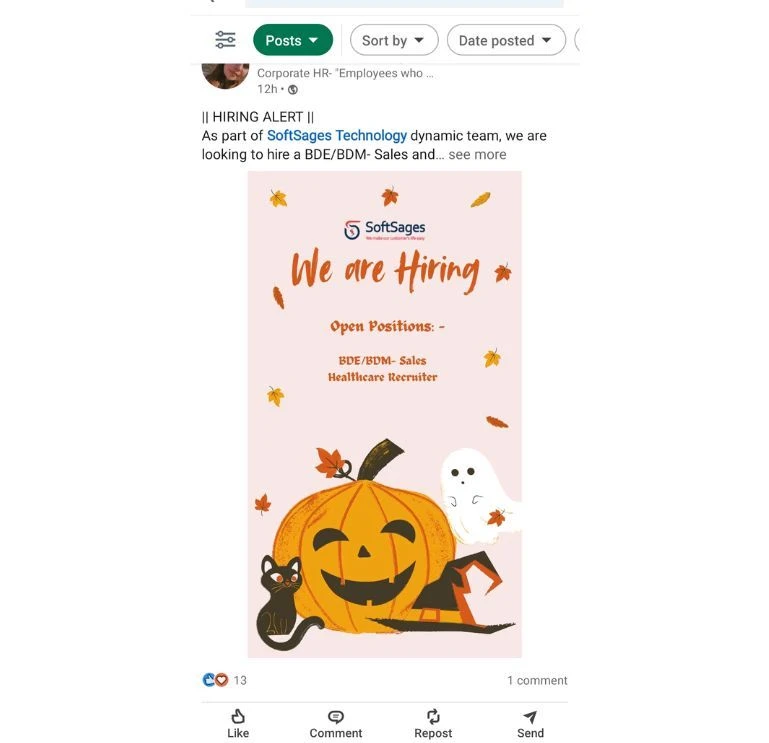
Sources
- Social Media Platforms: 80,000 posts were collected from Twitter, Reddit, and Instagram, ensuring a broad representation of emotions and sentiments across different contexts and user interactions.
- Diverse Topics and Emotional Tones: The dataset included posts on various topics, ensuring that the LLMs could understand and respond to a wide range of emotional cues.
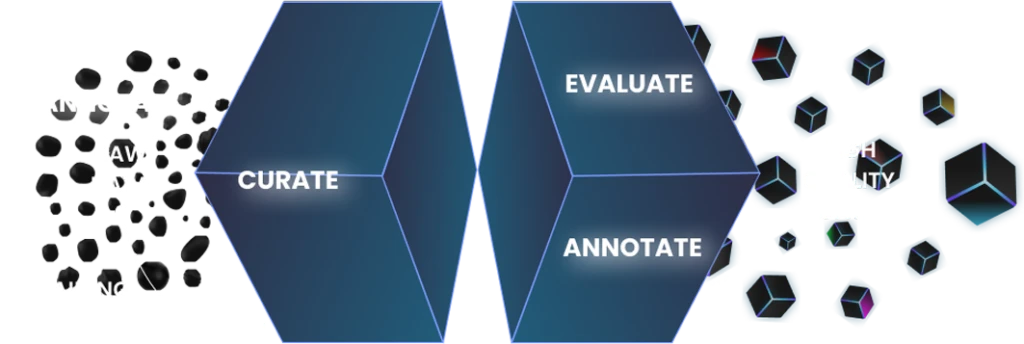
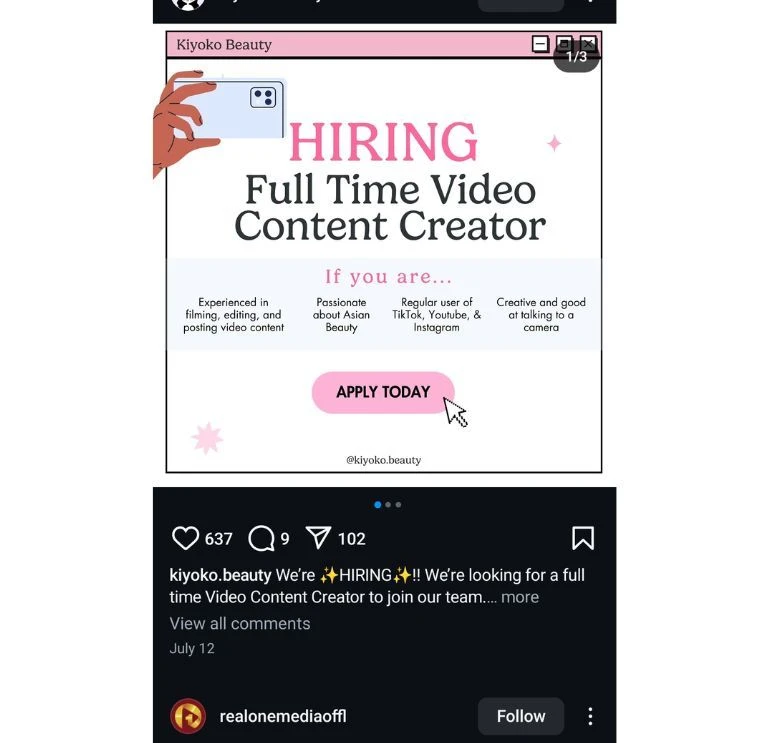
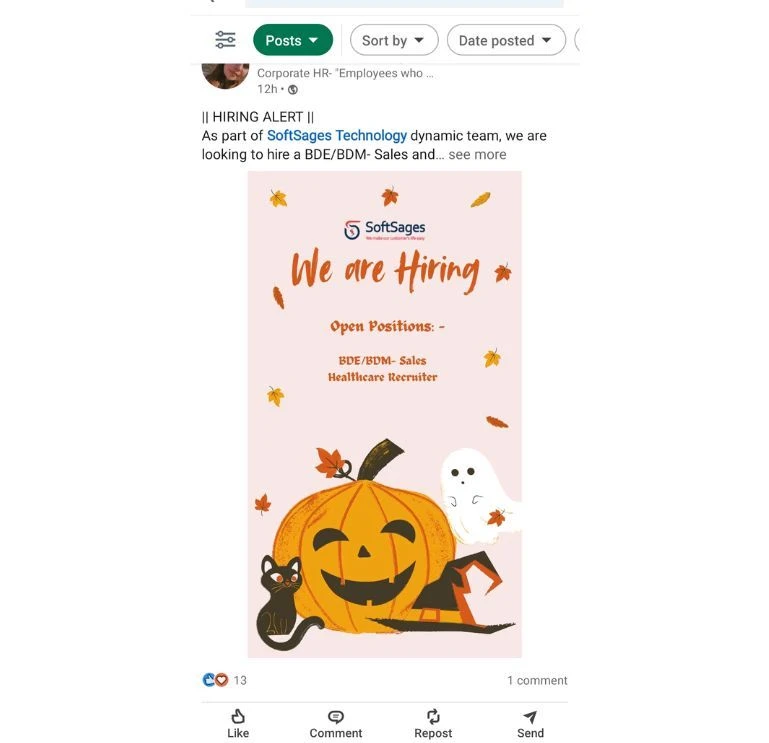
Data Collection Metrics
- Total Posts Collected: 80,000 social media posts.
- Emotion Tags Added: 240,000 emotion tags (3 emotions per post), encompassing a wide spectrum of emotional states.
Annotation Process
Stages
- Sentiment Analysis: Annotators identify and label each post with the underlying sentiment (positive, negative, neutral).
- Emotion Detection: Specific emotions such as happiness, anger, sadness, and others were identified and label, providing nuanced emotional context for each post.
Annotation Metrics
- Sentiments Annotated: All 80,000 posts were label with their corresponding sentiment.
- Emotions Tagged: 240,000 emotion tags were applied across the dataset, capturing the complex emotional content in the posts.
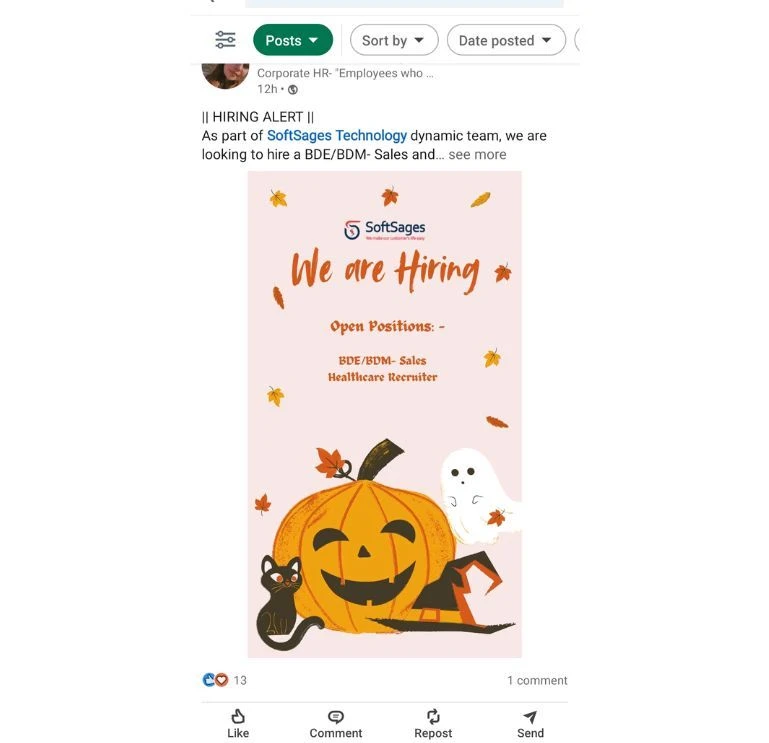
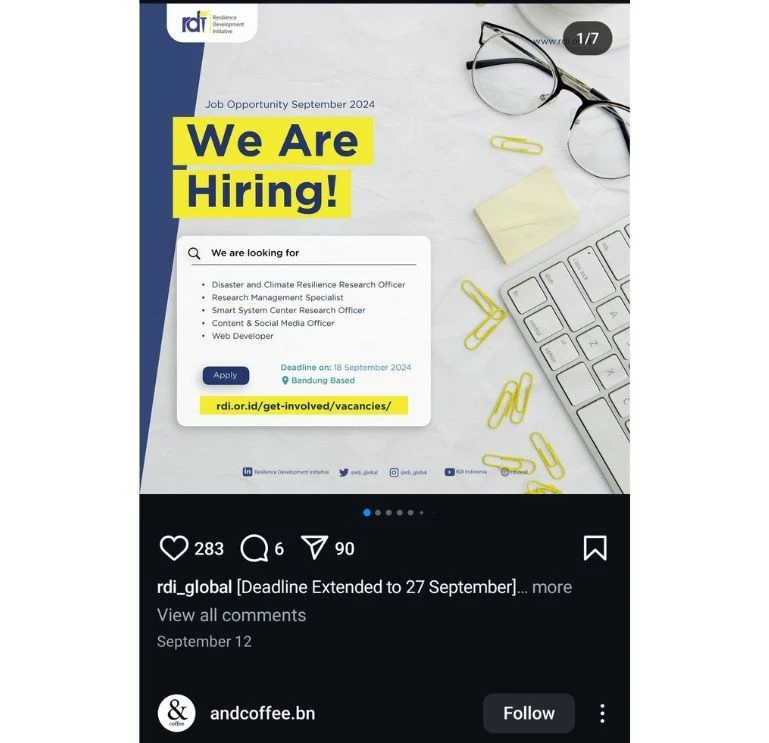
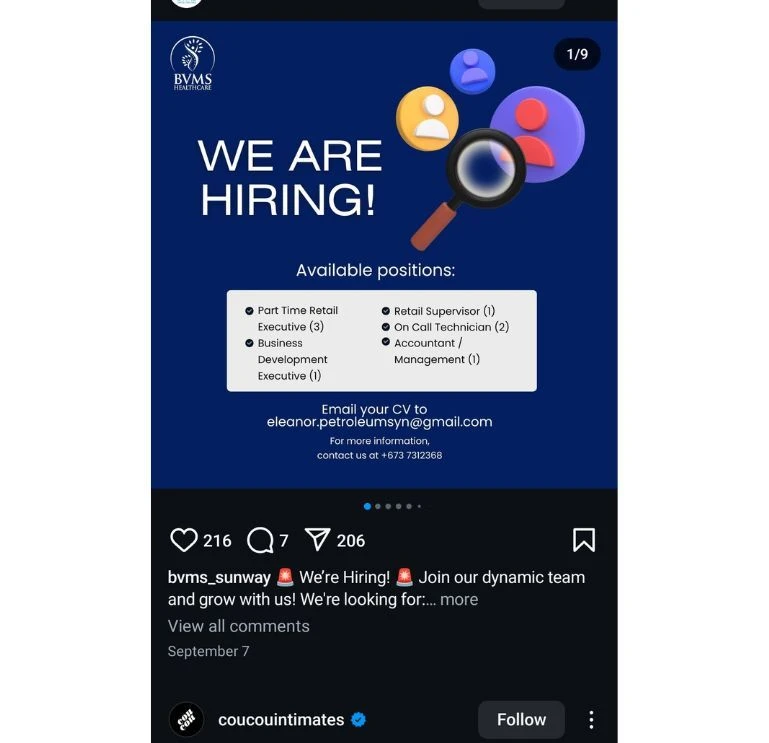
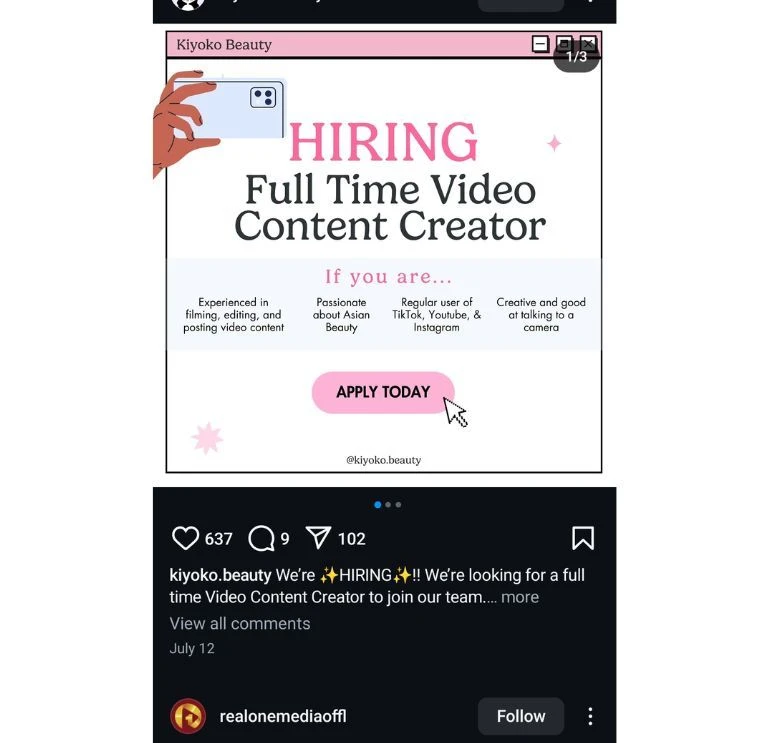
Quality Assurance
Stages
- Continuous Model Testing: The dataset was very meticulously validate as to if it is really contributing to the model’s emotional sensing and response improvement.
- Annotator Expertise: A team of 45 annotators with backgrounds in psychology and social media analysis ensured high-quality and consistent annotations.
- Improvement Process: Feedback loops were established to refine the annotation process and improve the quality of the dataset over time.
QA Metrics
- Emotion Detection Accuracy: The dataset significantly improve the LLM’s ability to detect emotions with high accuracy, resulting in more empathetic and appropriate responses in customer support applications.
- Sentiment Analysis Accuracy: The LLMs trained on this dataset achieved. A high accuracy rate in identifying and responding to different sentiments in social media posts.
- Annotation Consistency: The annotation process maintain a high level of consistency across. The 80,000 posts, ensuring reliable training data for the LLMs.
Conclusion
The creation of this sentiment and emotion detection dataset represents. A significant advancement in improving LLMs’ ability to understand and respond to emotional content. This dataset enhances the LLMs’ empathy and contextual understanding. Making them more effective in applications such as customer support and social media interactions.

Quality Data Creation

Guaranteed TAT

ISO 9001:2015, ISO/IEC 27001:2013 Certified

HIPAA Compliance

GDPR Compliance

Compliance and Security
Let's Discuss your Data collection Requirement With Us
To get a detailed estimation of requirements please reach us.