Sentiment Analysis for Movie Reviews
Home » Case Study » Sentiment Analysis for Movie Reviews
Project Overview:
Objective
The objective of sentiment analysis for movie reviews is to automatically analyze and categorize the sentiments expressed in reviews, providing insights into audience opinions, emotions, and reactions towards films.
Scope
The scope of sentiment analysis for movie reviews encompasses the automated analysis of sentiments expressed in film reviews, serving both the film industry and audiences. It provides filmmakers and studios with valuable insights into audience reactions, aiding in film production and marketing decisions.
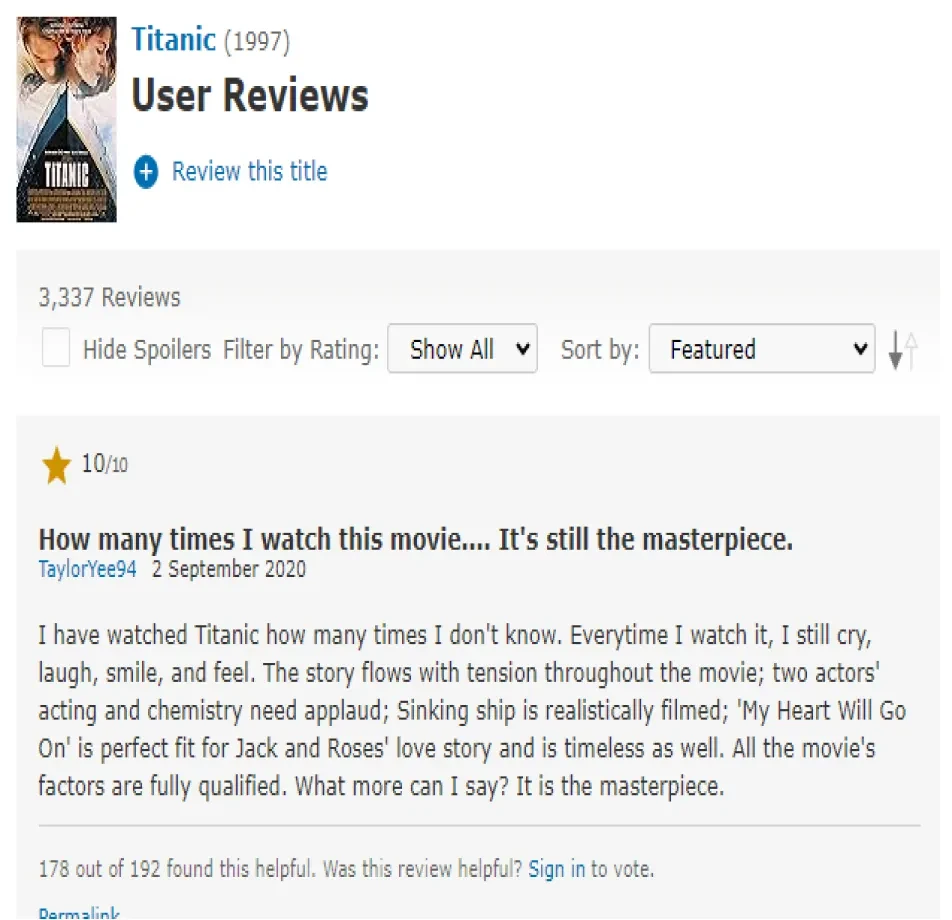
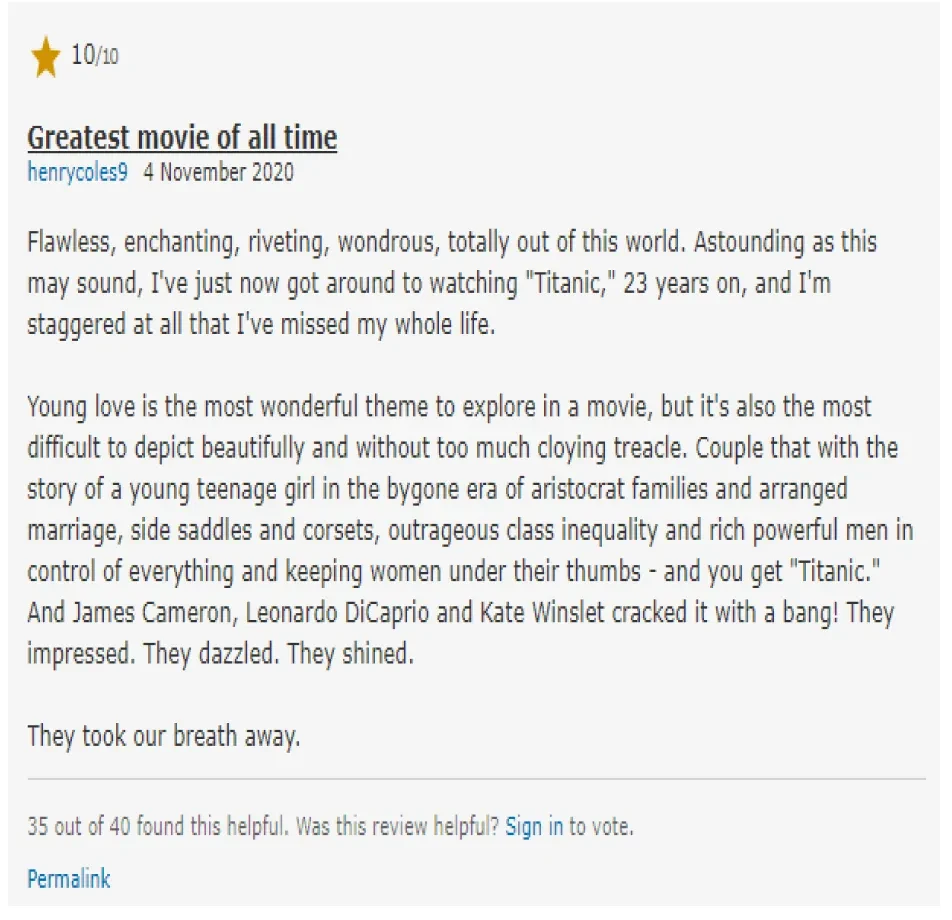
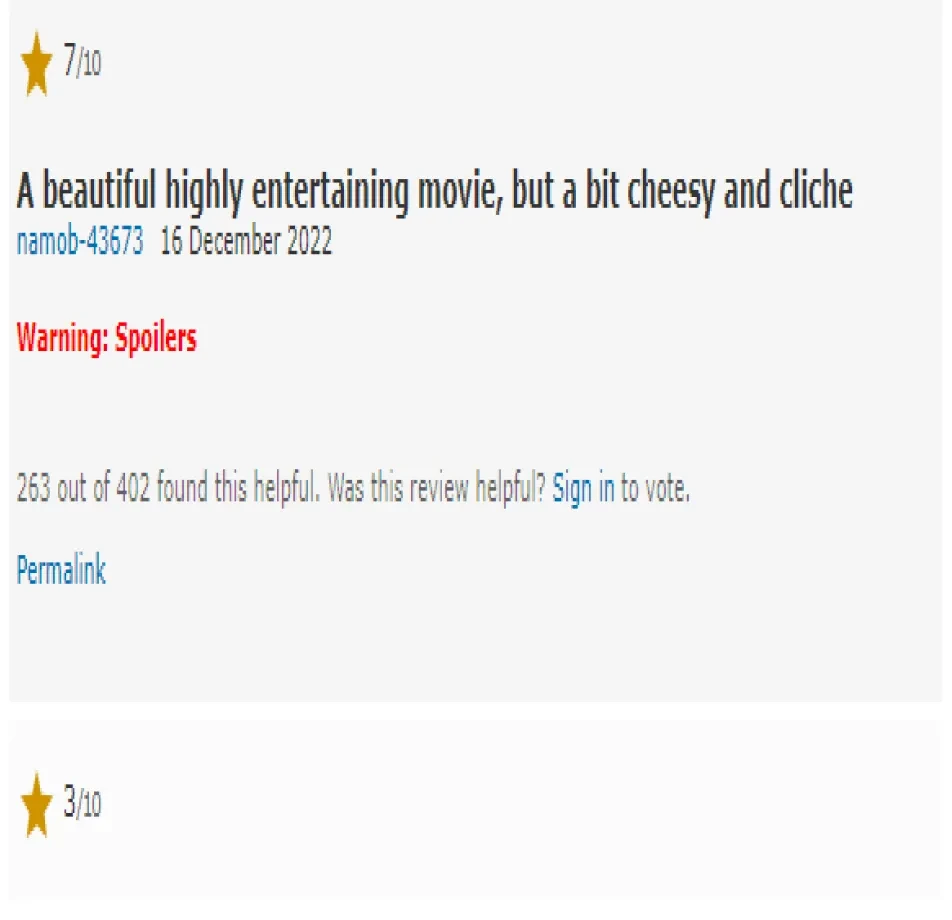
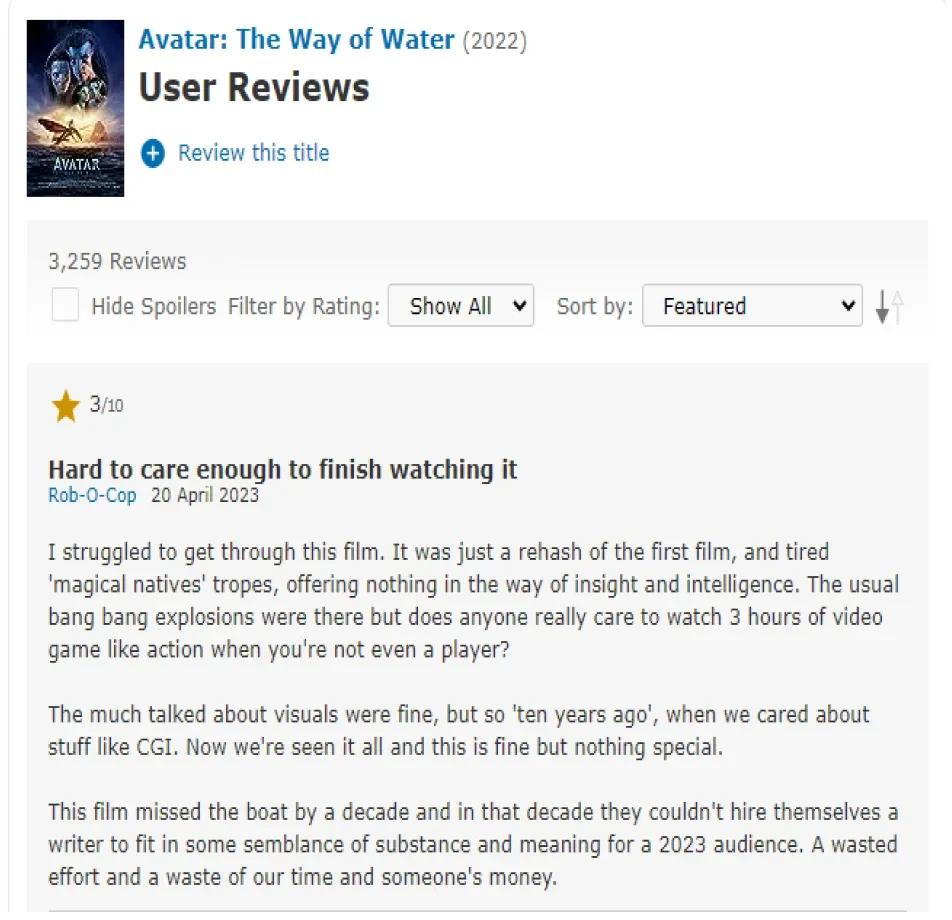
Sources
- Movie Review Websites: Gather movie review data from popular websites like IMDb, Rotten Tomatoes, or Metacritic, where users and critics share their opinions and ratings.
- Social Media Platforms: Extract movie-related sentiments from social media platforms like Twitter, Facebook, and Reddit, where users frequently discuss films and share their views and reactions.
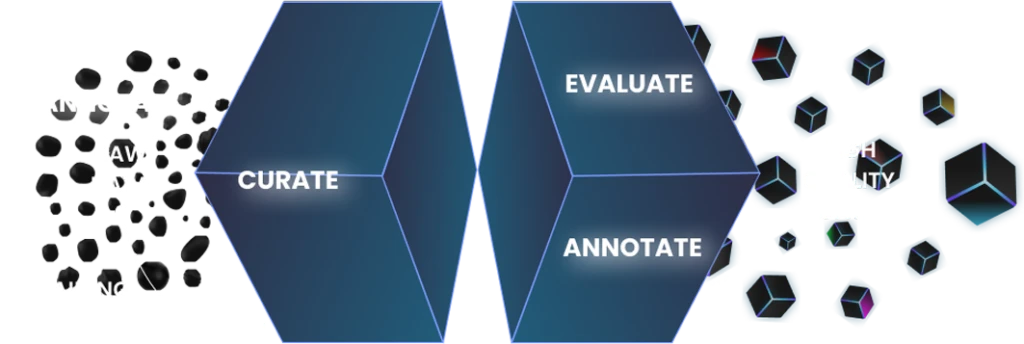
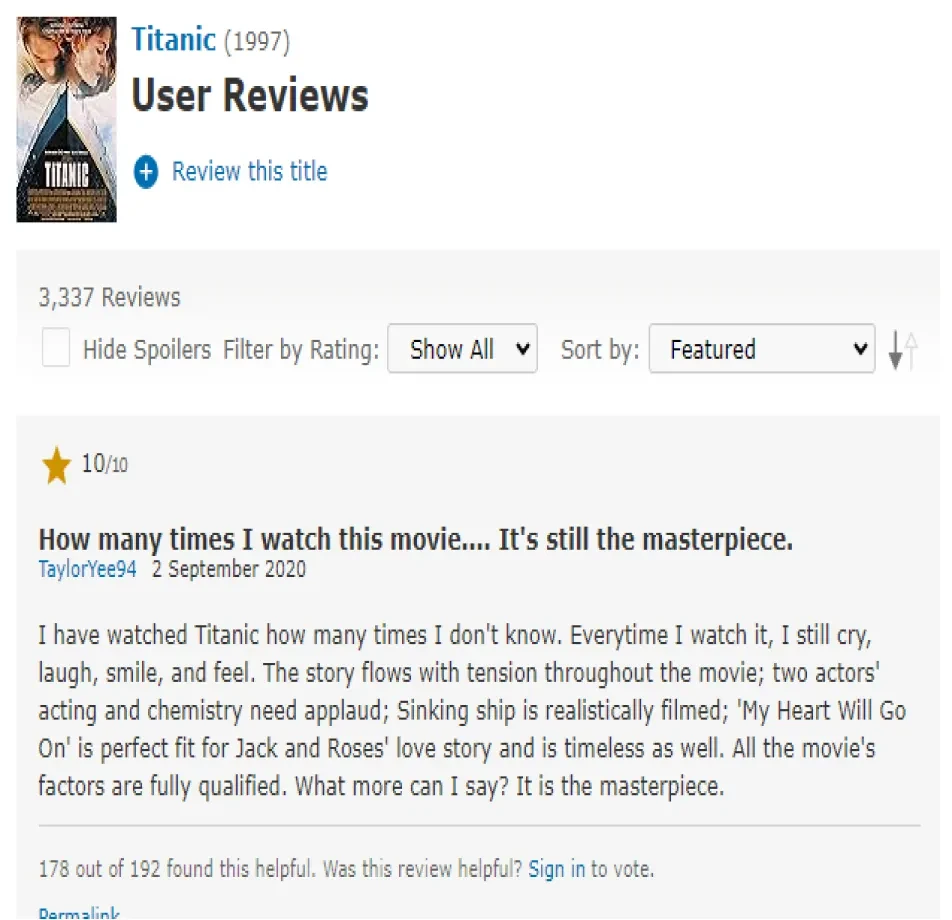
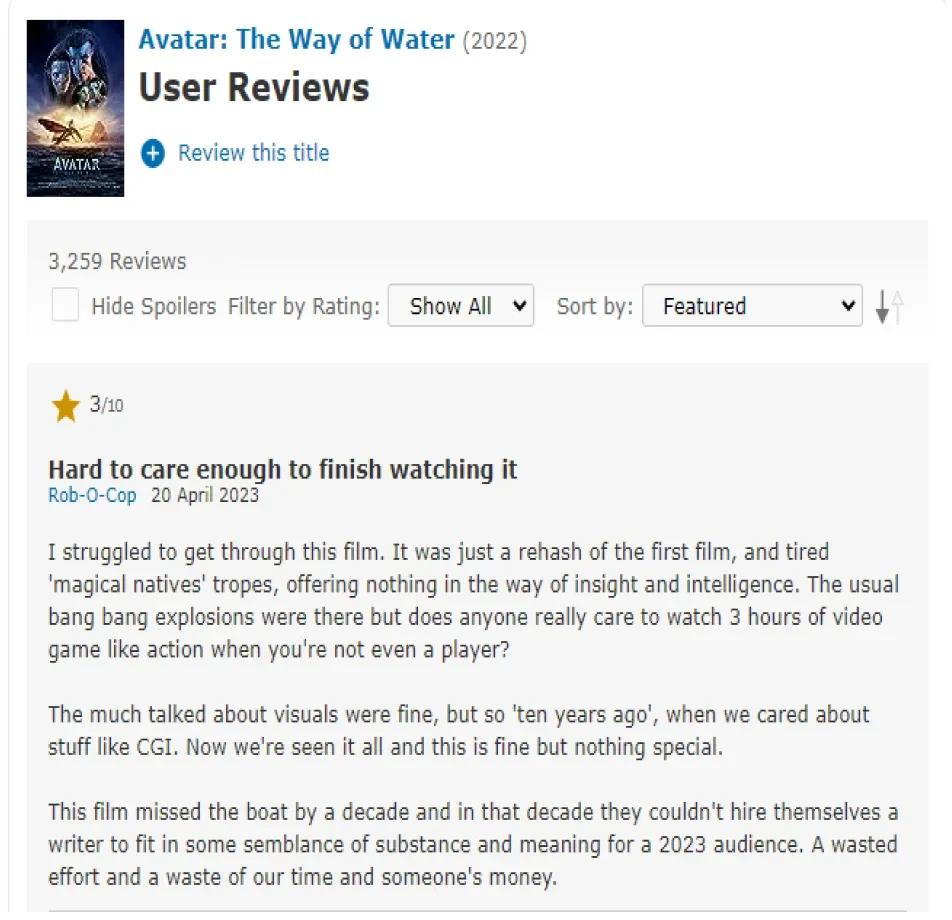
Data Collection Metrics
- Data Volume: Quantity of collected movie reviews.
- Data Diversity: Variety of review sources and types.
Annotation Process
Stages
- Data Collection: Gather movie reviews from various sources, including websites, social media, and review platforms.
- Data Preprocessing: Clean and standardize the collected text data, including text normalization and removal of irrelevant information.
- Sentiment Labeling: Annotate the reviews with sentiment labels (positive, negative, neutral) or a more fine-grained sentiment scale.
- Feature Extraction: Extract relevant features from the text data, such as keywords, sentiment words, or embeddings.
- Model Training: Utilize machine learning algorithms or deep learning models to train sentiment analysis classifiers.
- Sentiment Analysis: Apply the trained models to analyze and categorize sentiments in movie reviews.
Annotation Metrics
- Inter-Annotator Agreement (IAA): Measure the level of agreement among human annotators when labeling sentiments in movie reviews, ensuring consistency and reliability in annotations.
- Annotation Accuracy: Evaluate the precision of sentiment annotations by assessing the percentage of correctly labeled sentiments within the dataset.
- Annotation Efficiency: Assess the speed and cost-effectiveness of the annotation process, ensuring that it is efficient and scalable for large movie review datasets.
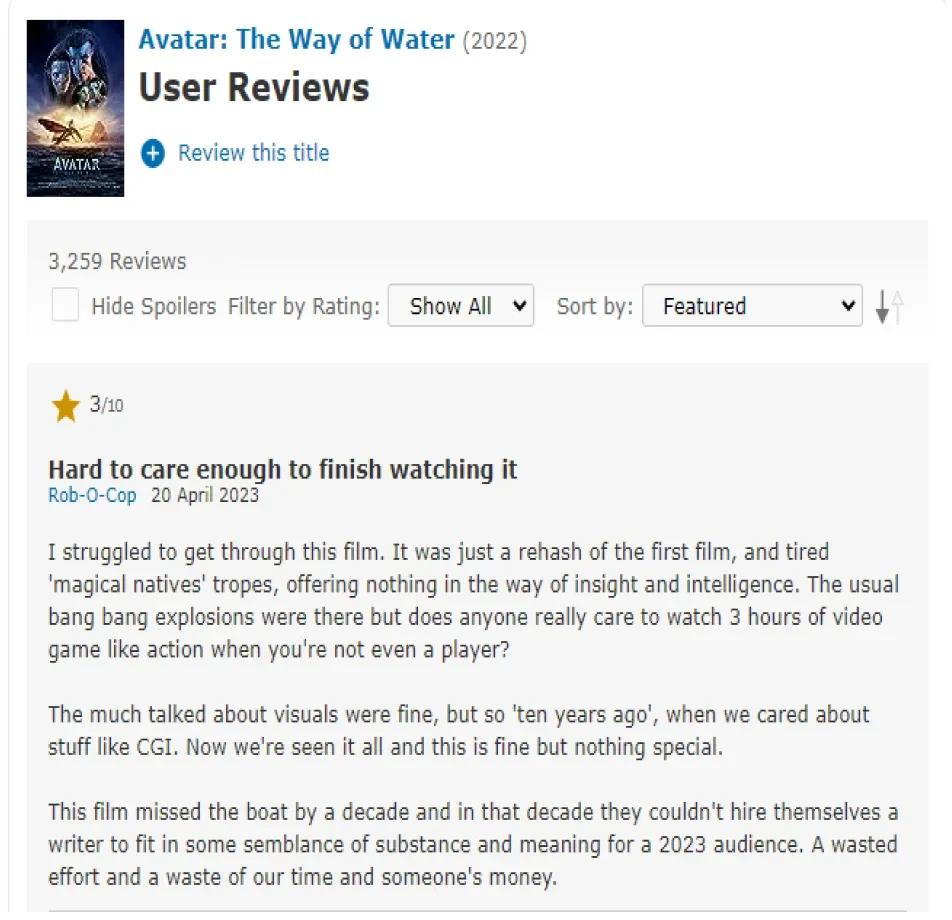
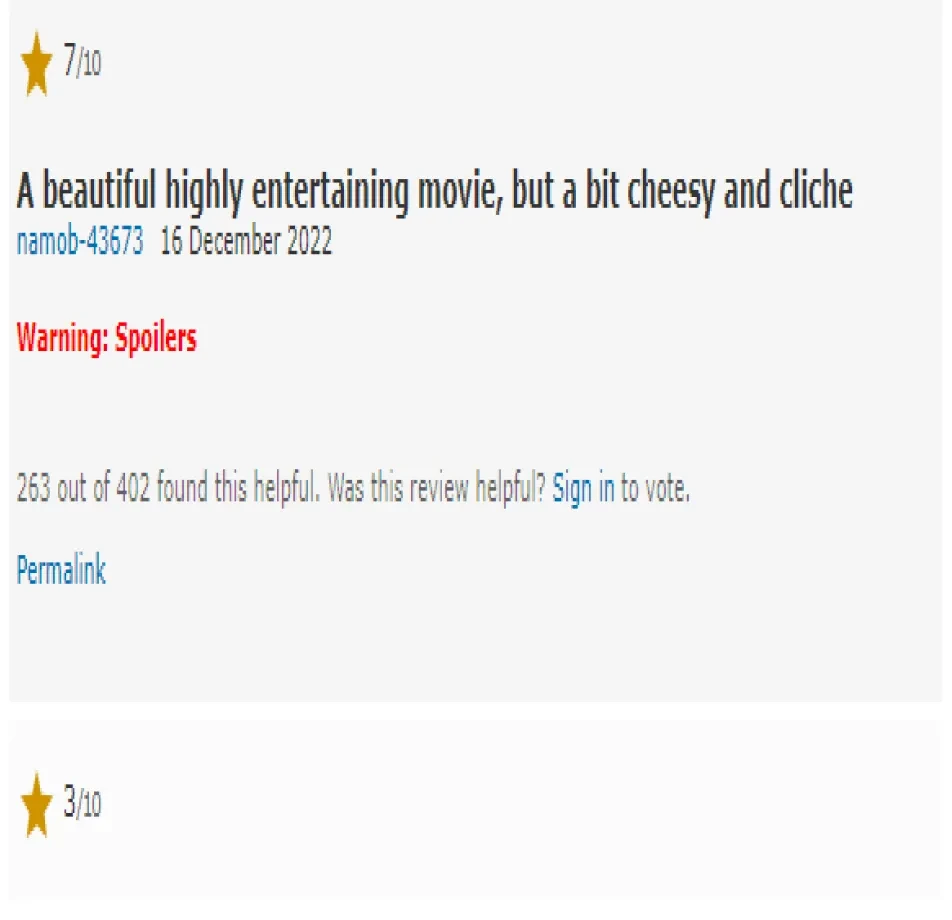
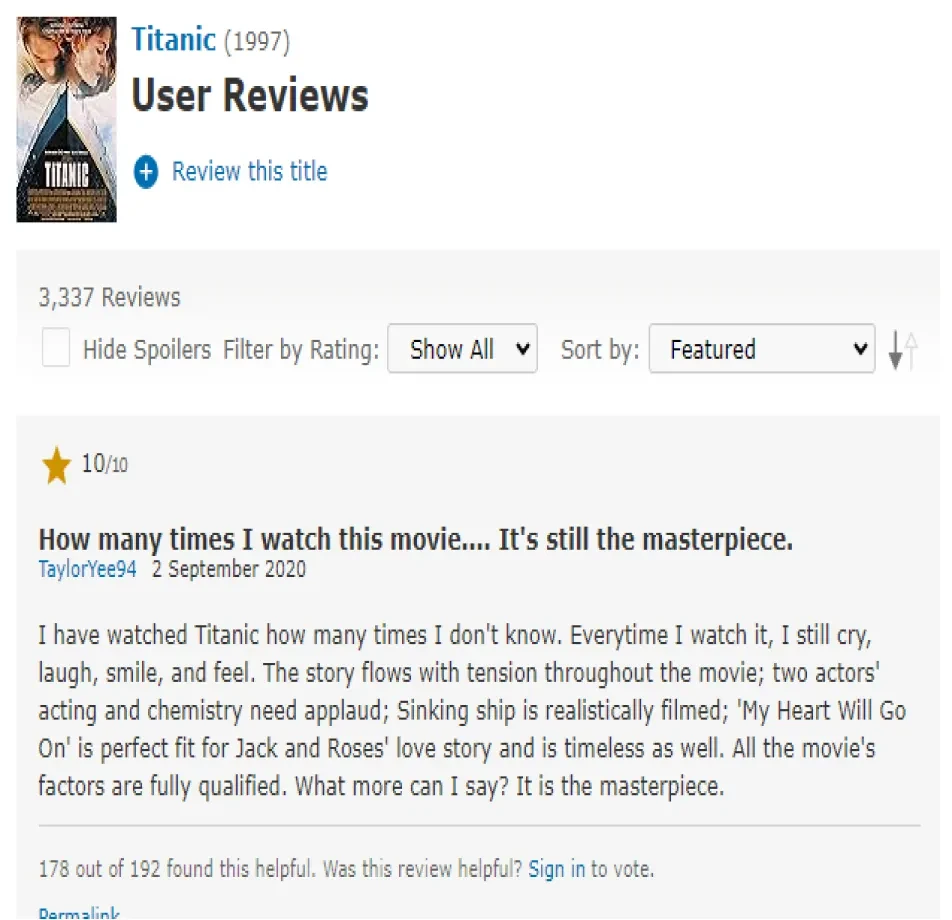
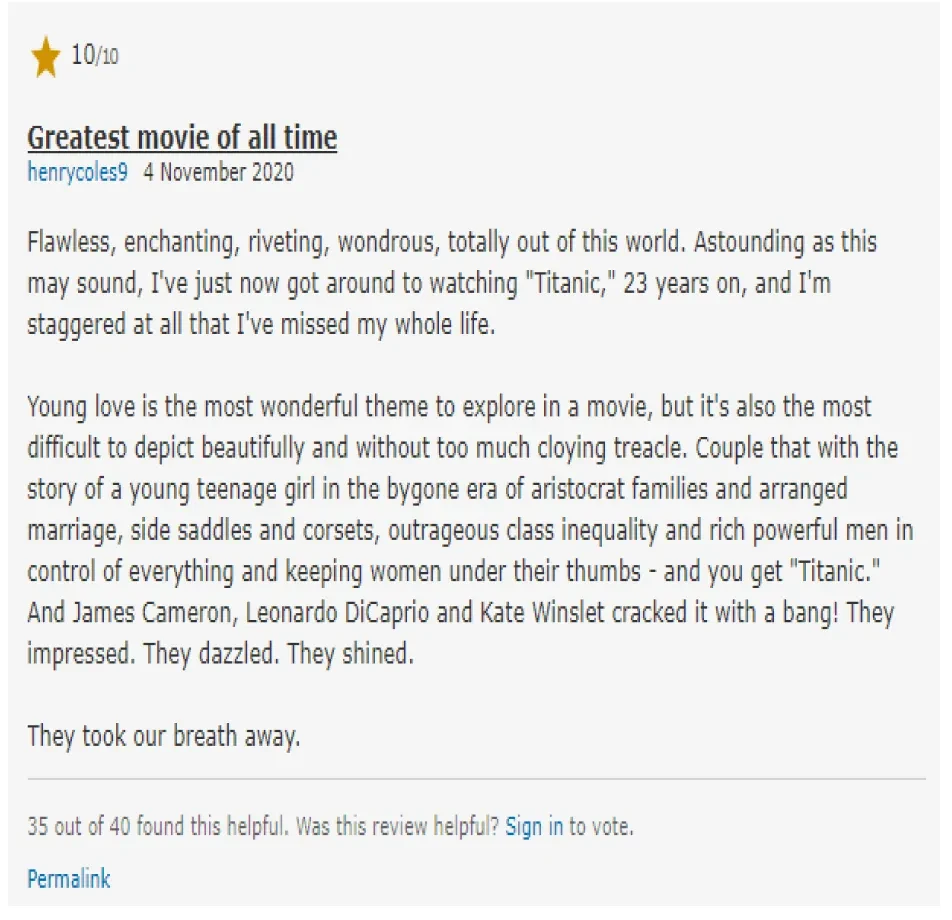
Quality Assurance
Stages
Data Privacy: Secure user data and comply with privacy regulations.
Quality Control: Maintain accuracy and reliability.
Ethical Practices: Adhere to ethical guidelines in data handling.
QA Metrics
- Accuracy: Evaluate alignment with actual sentiments.
- User Satisfaction: Assess user feedback and system usefulness.
Conclusion
Sentiment analysis for movie reviews is a valuable tool that unlocks insights into audience opinions and emotions, aiding both filmmakers and viewers. By automatically assessing sentiments expressed in reviews, this technology enables filmmakers to gauge audience reactions, refine storytelling, and enhance their craft.

Quality Data Creation

Guaranteed TAT

ISO 9001:2015, ISO/IEC 27001:2013 Certified

HIPAA Compliance

GDPR Compliance

Compliance and Security
Let's Discuss your Data collection Requirement With Us
To get a detailed estimation of requirements please reach us.