Data Annotation for Self-Driving Cars
Home » Case Study » Data Annotation for Self-Driving Cars
Project Overview:
Objective
Data Annotation for Self-Driving Cars:Â facilitates the development of safe and reliable autonomous vehicles by enabling these models to recognize and respond to various real-world driving scenarios, ultimately enhancing road safety and transportation efficiency. The goal is to create a dataset that makes self-driving systems more accurate and efficient by using different types of real driving data.
Scope
Creating extensive datasets that cover various driving conditions, scenarios, and edge cases is crucial for ensuring AI models are robust and adaptable to real-world road conditions. By incorporating diverse and challenging situations, we can ensure that the models are well-equipped to handle the complexities of actual driving environments.
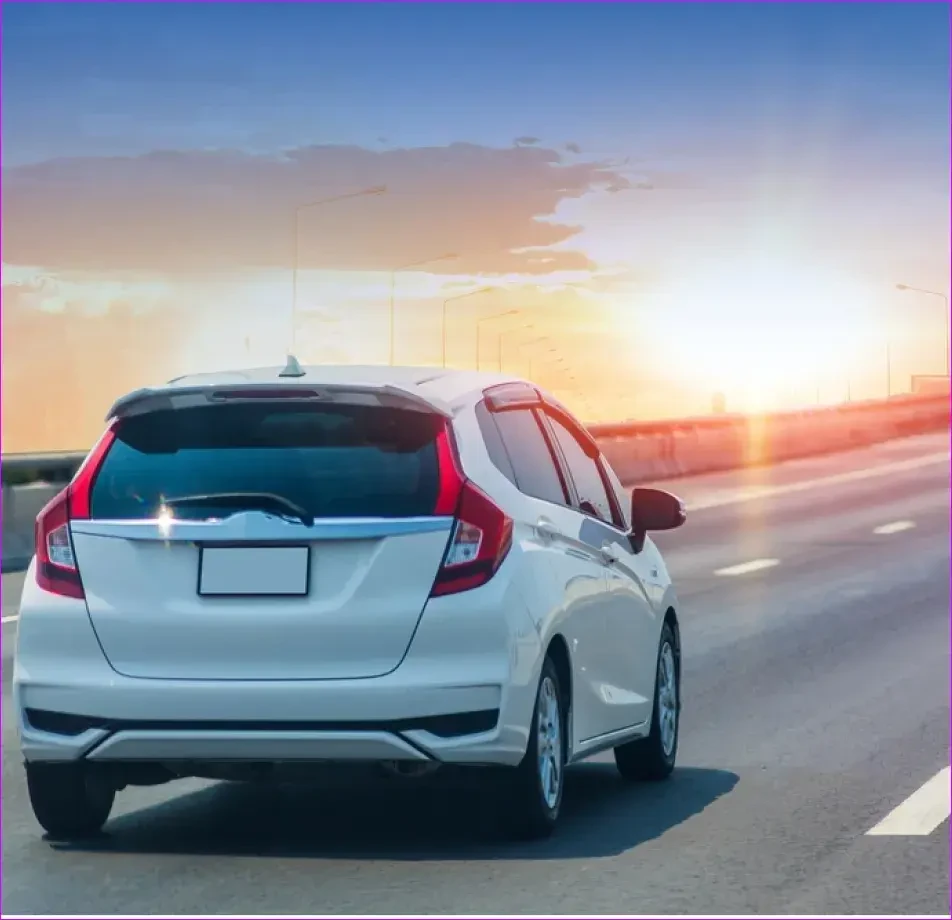
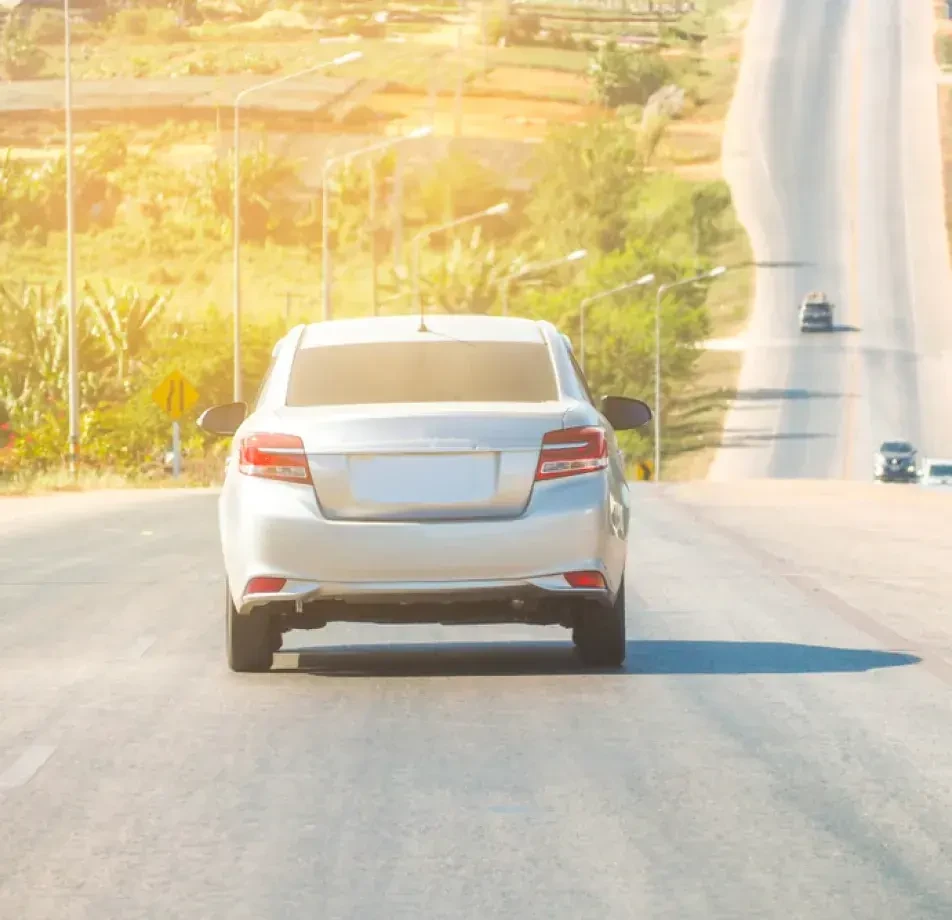
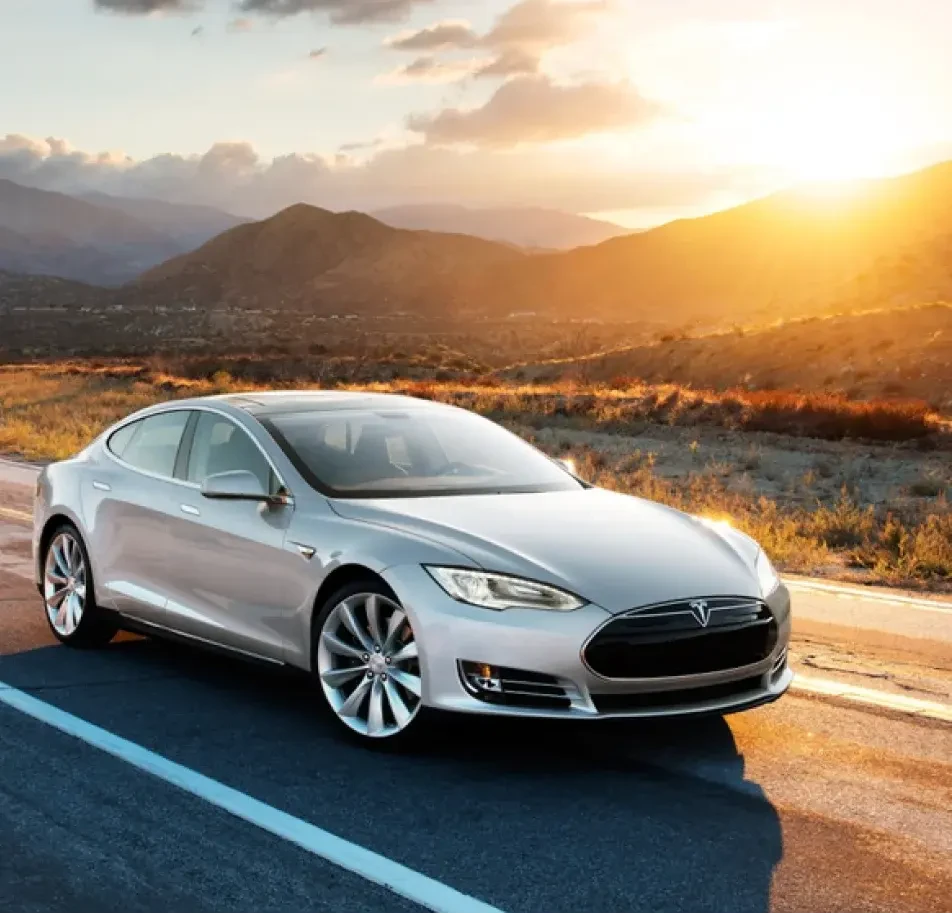
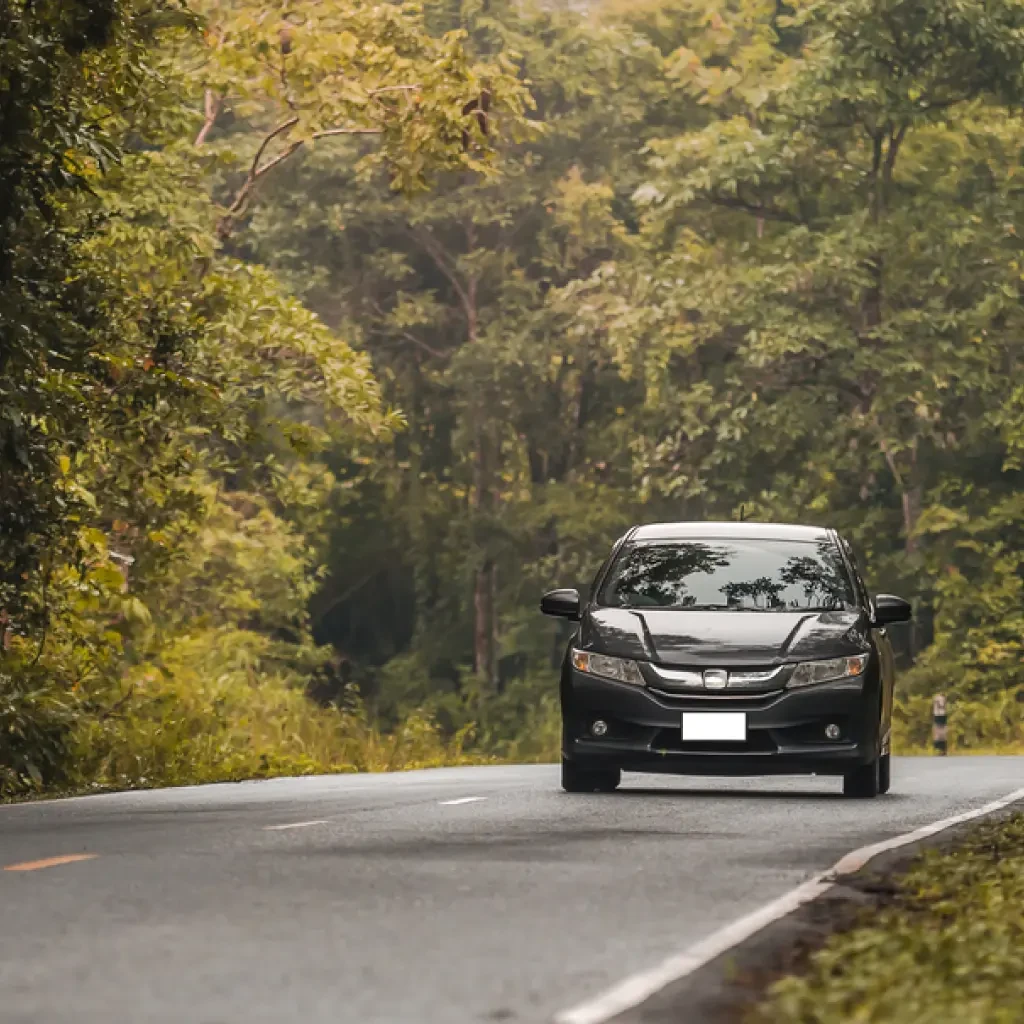
Sources
- Automotive Companies: Industry leaders share insights and methodologies in research papers.
- Academic Research: Meanwhile, academic researchers actively contribute valuable techniques and innovations through their publications.
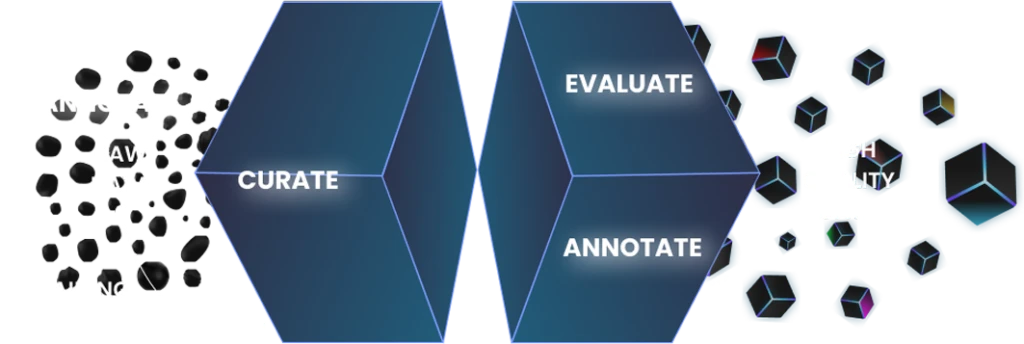
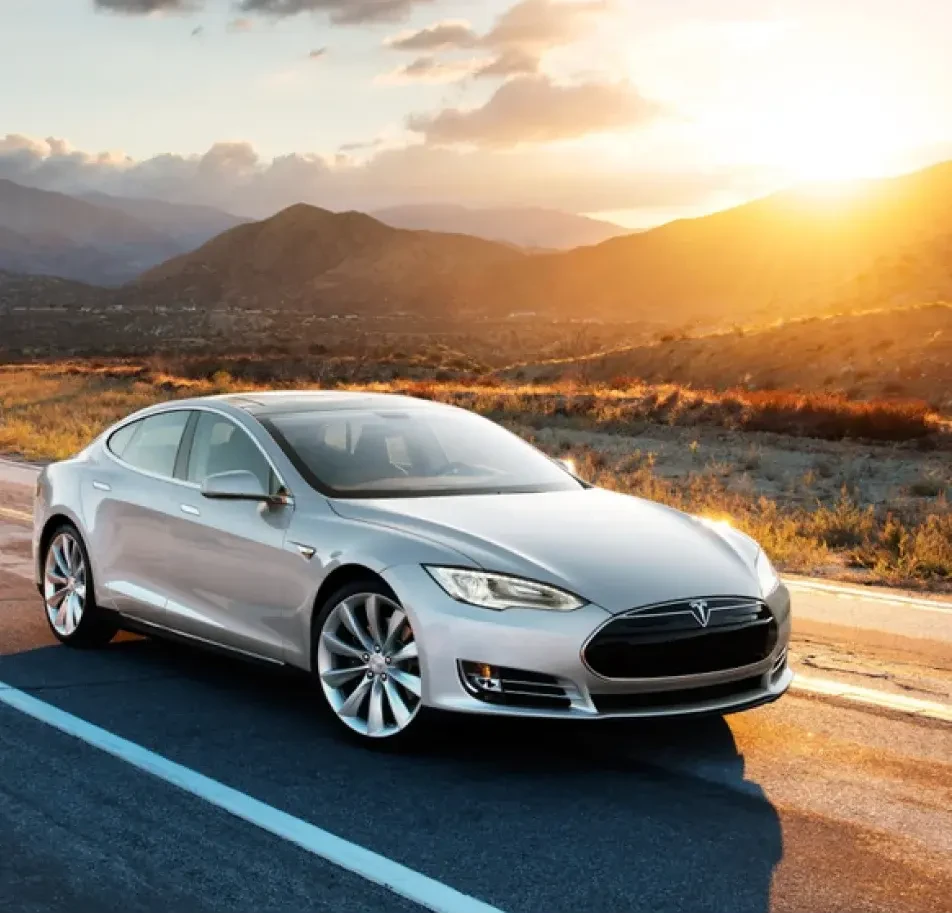
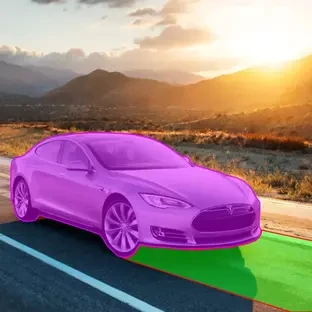
Data Collection Metrics
- Volume: The total amount of data collected.
- Sampling Rate: The frequency at which data is sampled or recorded over time.
Annotation Process
Stages
- Planning: Define objectives, sources, and methods.
- Data Collection: Gather information as per the plan.
- Validation: Verify data accuracy and consistency.
- Cleaning: Address errors and inconsistencies.
- Analysis: Interpret and draw insights from the data.
- Reporting: Communicate findings and outcomes.
Annotation Metrics
- Accuracy Rate: This metric measures the correctness of annotations compared to a reference or gold standard. Therefore, it provides a clear indication of how closely the annotations align with the expected results.
- Inter-annotator Agreement: This metric evaluates the consistency among different annotators when they perform the same annotation tasks. Consequently, it reflects the level of agreement and reliability across multiple annotators.
- Annotation Speed:
This metric tracks the time taken to complete individual annotation tasks. As a result, it helps assess the efficiency and pace of the annotation process.
Â
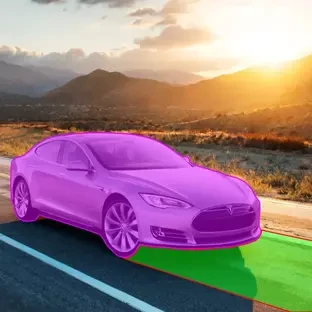
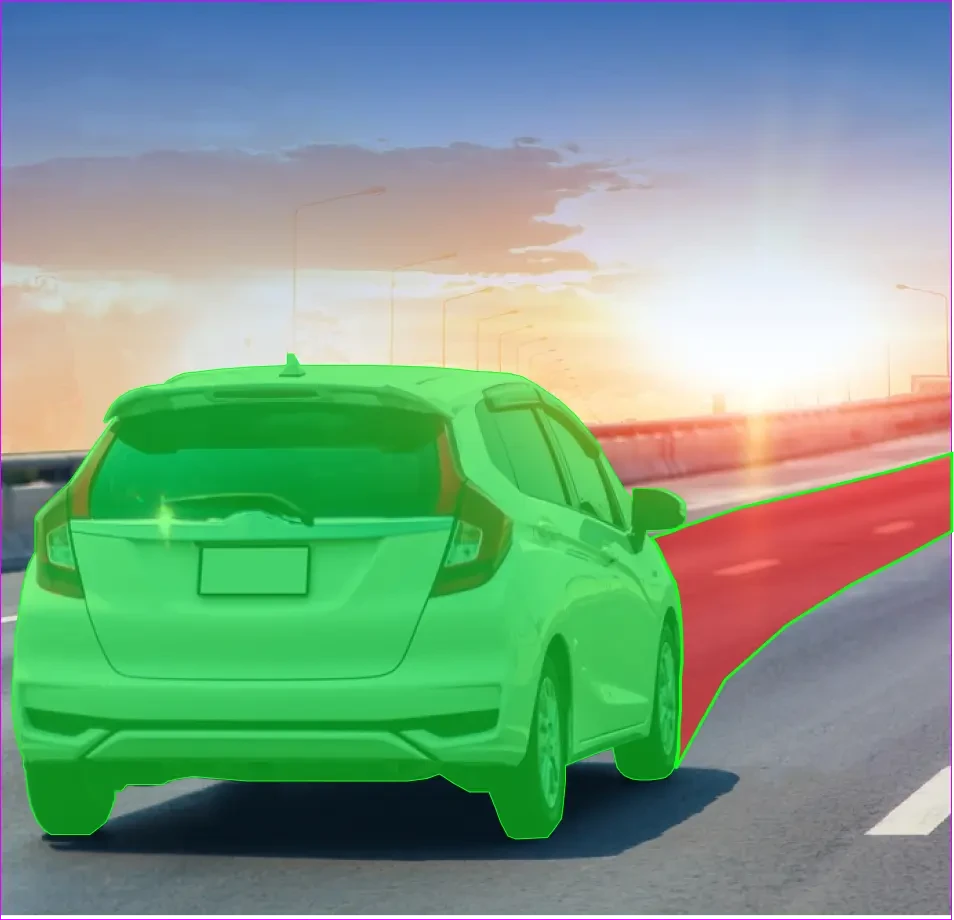
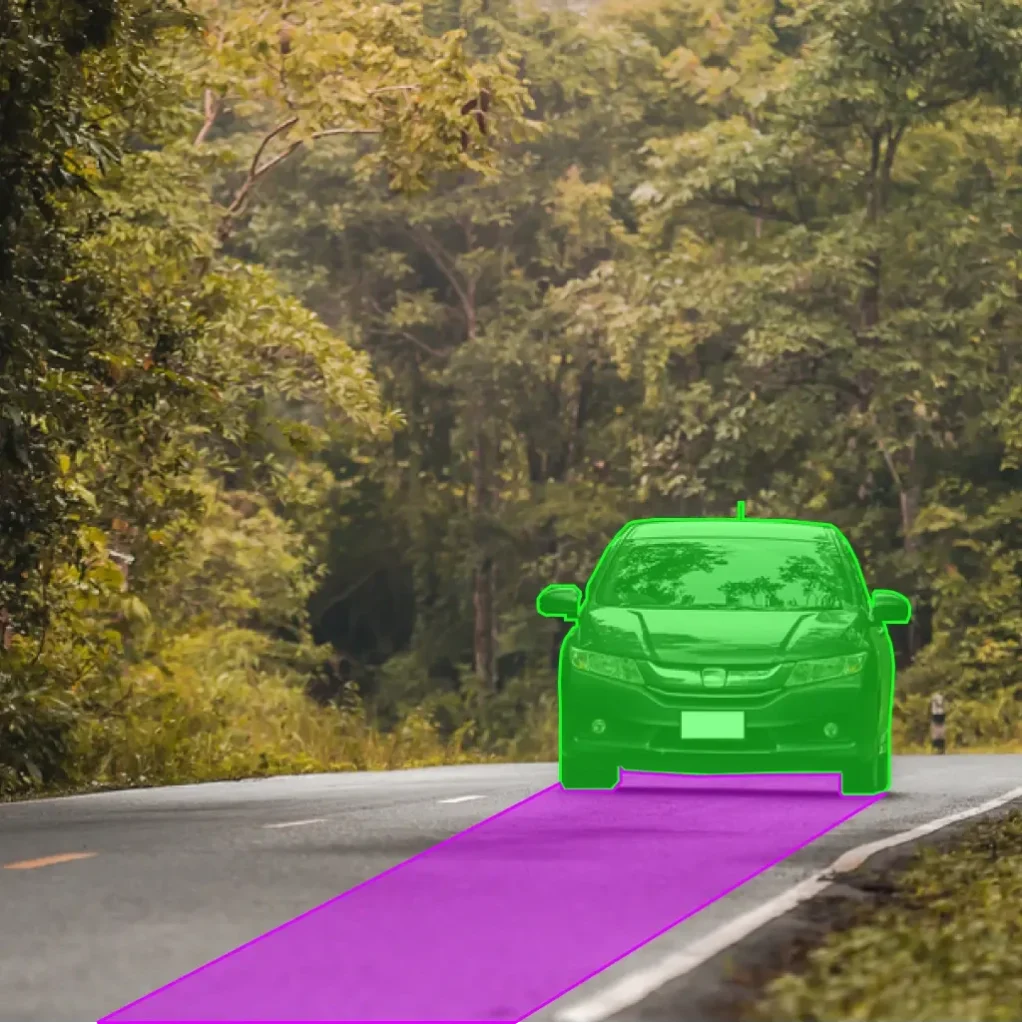
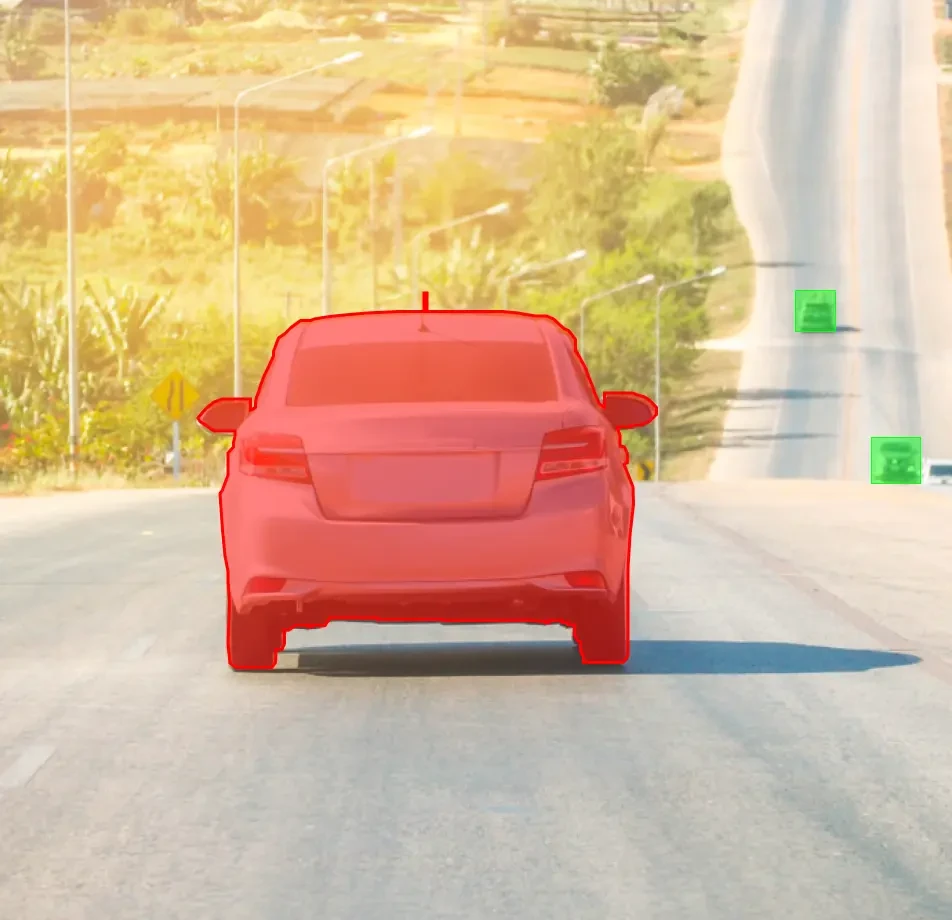
Quality Assurance
Stages
Data Quality: Ensuring data quality means making sure data is accurate, complete, consistent, reliable, and timely. Consequently, this makes the data fit for its intended use and analysis.
Privacy Protection:Privacy protection safeguards personal data from unauthorized access, use, or disclosure. Therefore, it preserves individual rights in the digital era.
Data Security: Data security protects data from unauthorized access and breaches. As a result, it ensures confidentiality and integrity in the digital realm.
QA Metrics
- Defect Density: Measures the number of defects per unit, indicating software quality.
- Additionally, Test Coverage: Evaluates the extent to which testing exercises the application or code, ensuring comprehensive quality assessment.
Conclusion
Data annotation serves as a critical component in the development of self-driving cars, as it enables machine learning algorithms to ensure safety. Despite being labor-intensive, innovations such as crowd-sourcing and privacy measures actively drive progress toward efficient autonomous vehicles. Additionally, by employing crowd-sourcing techniques, developers can tap into a wider pool of annotators, thereby accelerating the annotation process. Moreover, implementing stringent privacy measures not only safeguards sensitive data but also fosters trust among users, further advancing the development of self-driving technology.

Quality Data Creation

Guaranteed TAT

ISO 9001:2015, ISO/IEC 27001:2013 Certified

HIPAA Compliance

GDPR Compliance

Compliance and Security
Let's Discuss your Data collection Requirement With Us
To get a detailed estimation of requirements please reach us.