Portrait Matting Dataset
Home » Case Study » Portrait Matting Dataset
Project Overview:
Objective
The goal of this project is to develop a comprehensive Portrait Matting Dataset to enhance image processing capabilities, particularly in the field of portrait photography. This dataset is designed to enable advanced algorithms to distinguish foreground elements (people) from varying backgrounds accurately, a crucial step in image editing and compositing.
Scope
This project aims to create a dataset of diverse portrait images. Specifically, it focuses on different lighting conditions, backgrounds, and subjects. Moreover, the dataset includes high-resolution images that cover a wide demographic range. This variety ensures that the matting algorithms trained on it will be robust and effective.
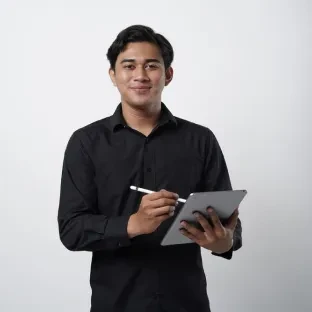
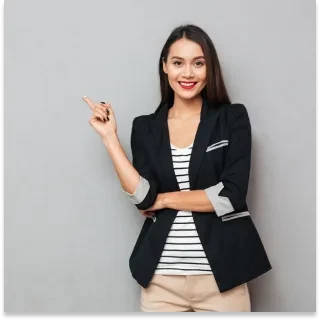
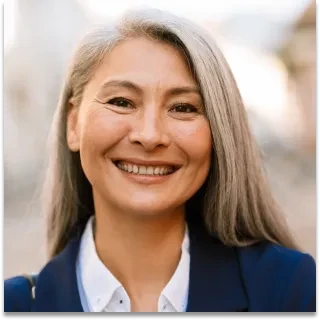
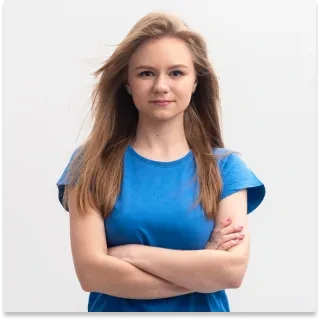
Sources
- High-Resolution Portrait Images: Collecting a diverse range of portrait images from various photographers and studios.
- Public Image Databases: Utilizing publicly available image datasets to supplement the collection.
- User-Generated Content: Incorporating user-submitted portrait images, ensuring variety and real-world applicability.
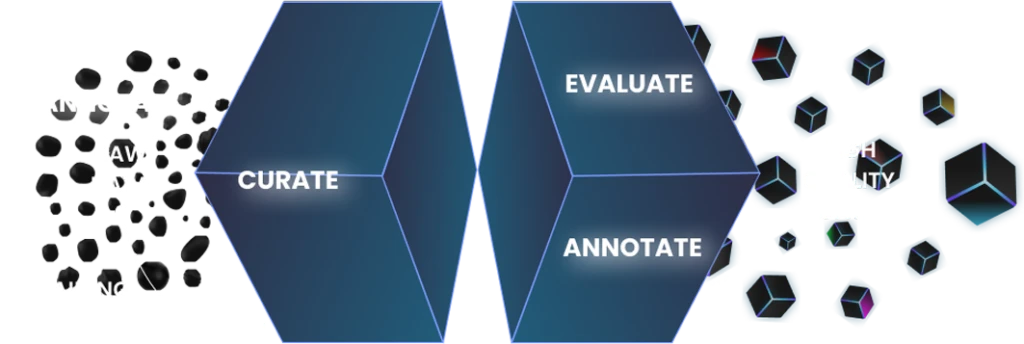
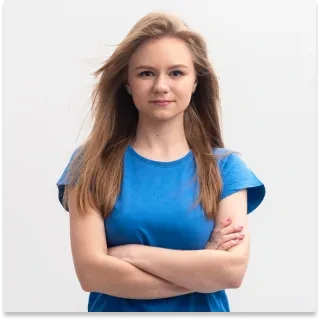
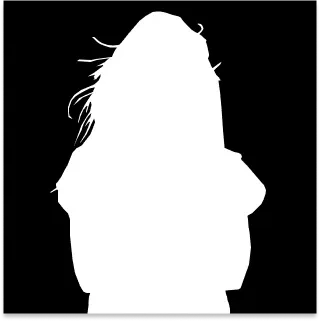
Data Collection Metrics
- Total Data Points: 15000 high-resolution portrait images.
- Data Diversity: Images represent a wide array of ages, ethnicities, lighting conditions, and backgrounds.
Annotation Process
Stages
- Data Segmentation: First, manually separate each portrait image to distinguish the subject from the background.
- Feature Tagging: Next, annotate each image with tags related to lighting, background complexity, subject position, and orientation.
- Matting Algorithms: Finally, use both manual and automated methods to improve the foreground-background separation in each image.
Annotation Metrics
- Segmentation Accuracy: Assessing the precision of separating subjects from backgrounds in annotated images. This helps to ensure the clarity and accuracy of the annotations.
- Variety in Annotations: Ensuring that a wide range of image complexities and scenarios are covered. This allows for a more comprehensive evaluation of the model’s performance across different situations.
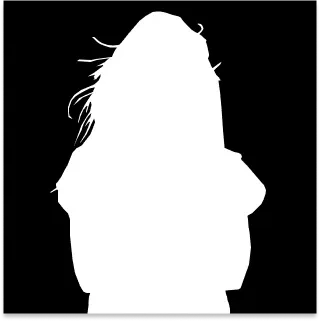
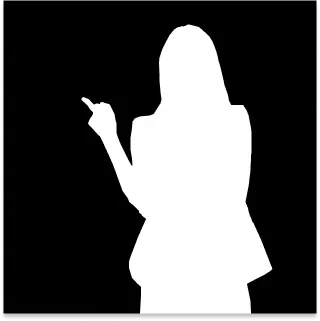
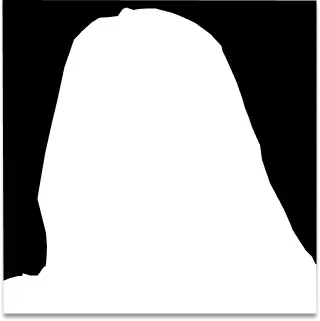
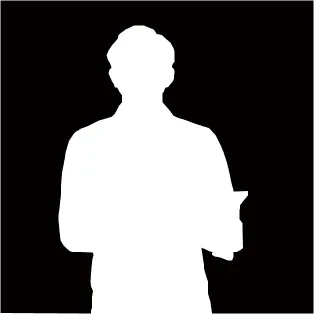
Quality Assurance
Stages
Expert Review: To ensure high-quality annotations, we will collaborate closely with professional photographers and image editors. They will help us validate and refine our annotations.
Algorithm Testing: Additionally, we will regularly test the dataset using advanced matting algorithms. This will help us assess the effectiveness of our dataset.
User Feedback: Furthermore, we will incorporate feedback from our users to continuously improve the quality of our annotations. This iterative process will help us maintain high standards and meet user expectations.
QA Metrics
- Reviewed Annotations: 20% of the dataset subjected to expert review.
- Improvement Rate: Tracking enhancements in algorithm performance using the dataset.
Conclusion
The Portrait Matting Dataset project is crucial for advancing digital image processing, especially in portrait photography. By offering a rich, diverse, and accurately annotated dataset, it sets the stage for developing more sophisticated and precise matting algorithms. This dataset not only aids the image editing industry but also drives research in computer vision and artificial intelligence, leading to more refined and realistic image composites.

Quality Data Creation

Guaranteed TAT

ISO 9001:2015, ISO/IEC 27001:2013 Certified

HIPAA Compliance

GDPR Compliance

Compliance and Security
Let's Discuss your Data collection Requirement With Us
To get a detailed estimation of requirements please reach us.