Panoptic Scenes Segmentation Dataset
Home » Case Study » Panoptic Scenes Segmentation Dataset
Project Overview:
Objective
In this project, we have meticulously developed a comprehensive dataset tailored for panoptic segmentation. This innovative dataset merges the nuances of both semantic and instance segmentation across diverse scenes. Consequently, it marks a significant leap in fields like urban planning, autonomous driving, robotics, and augmented reality.
Scope
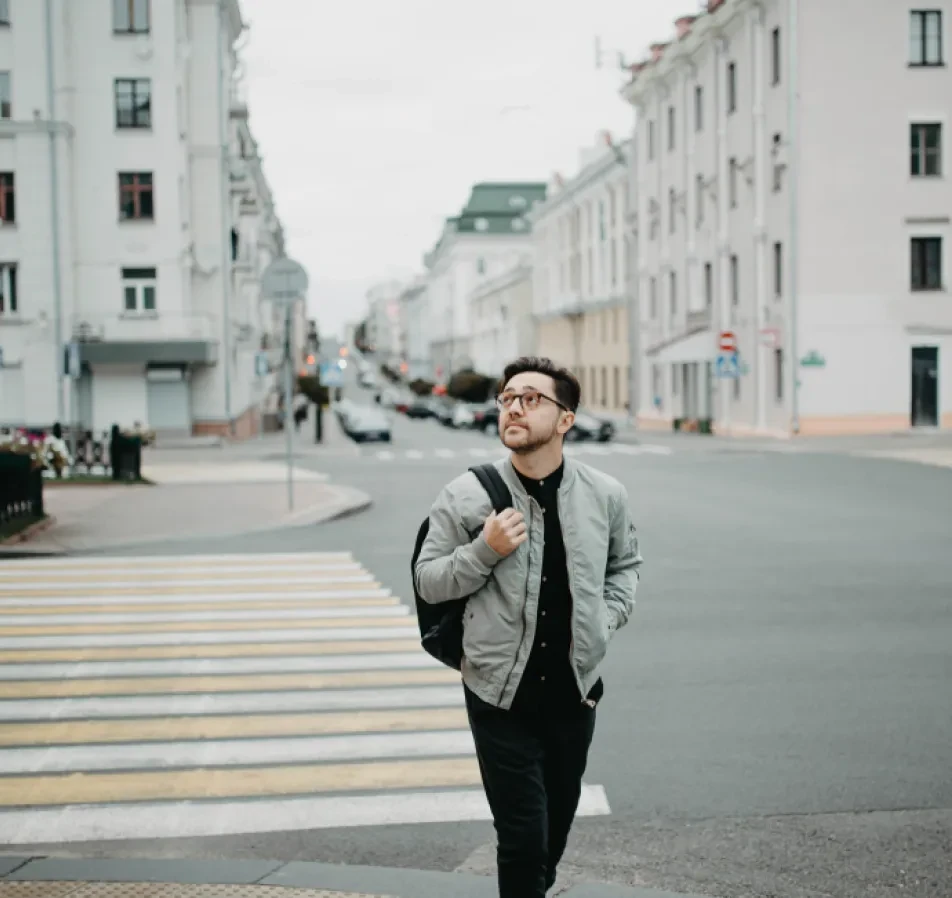
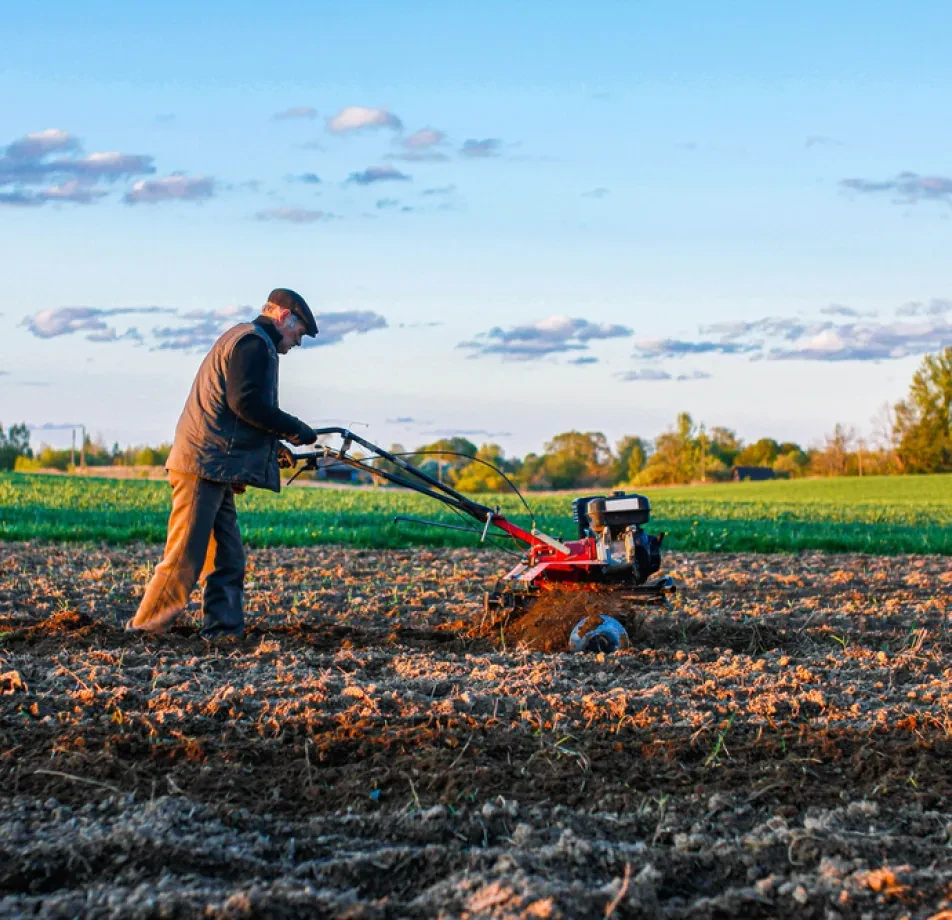
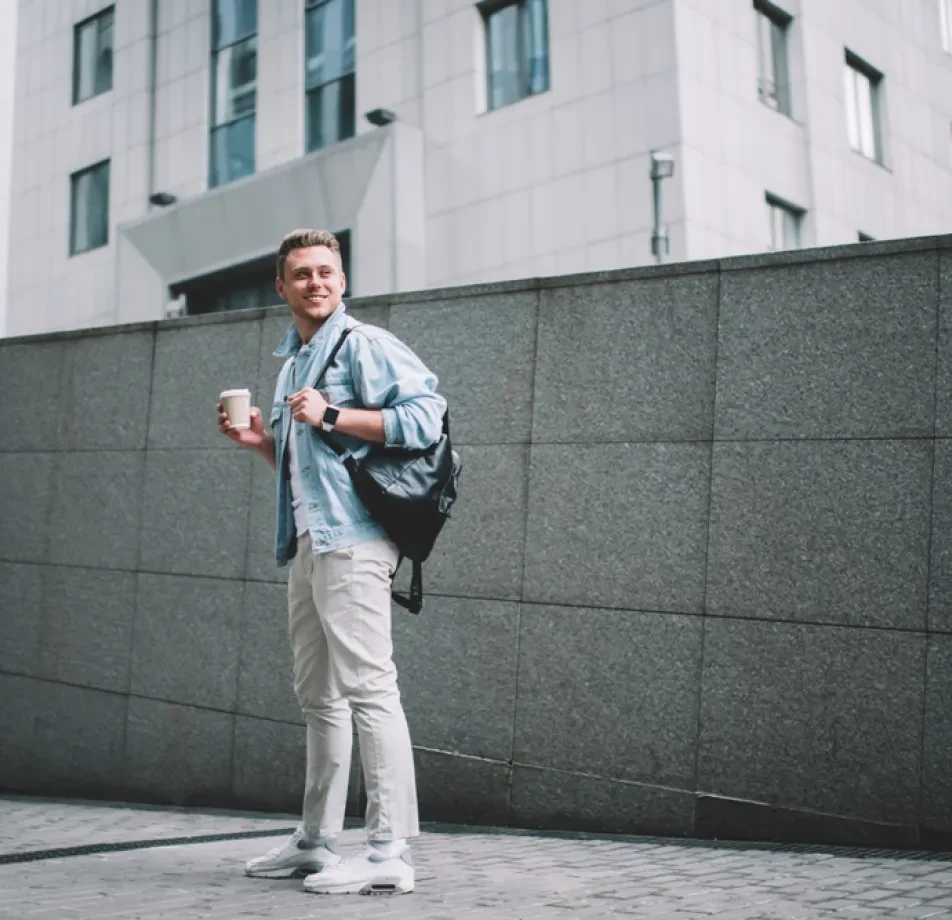
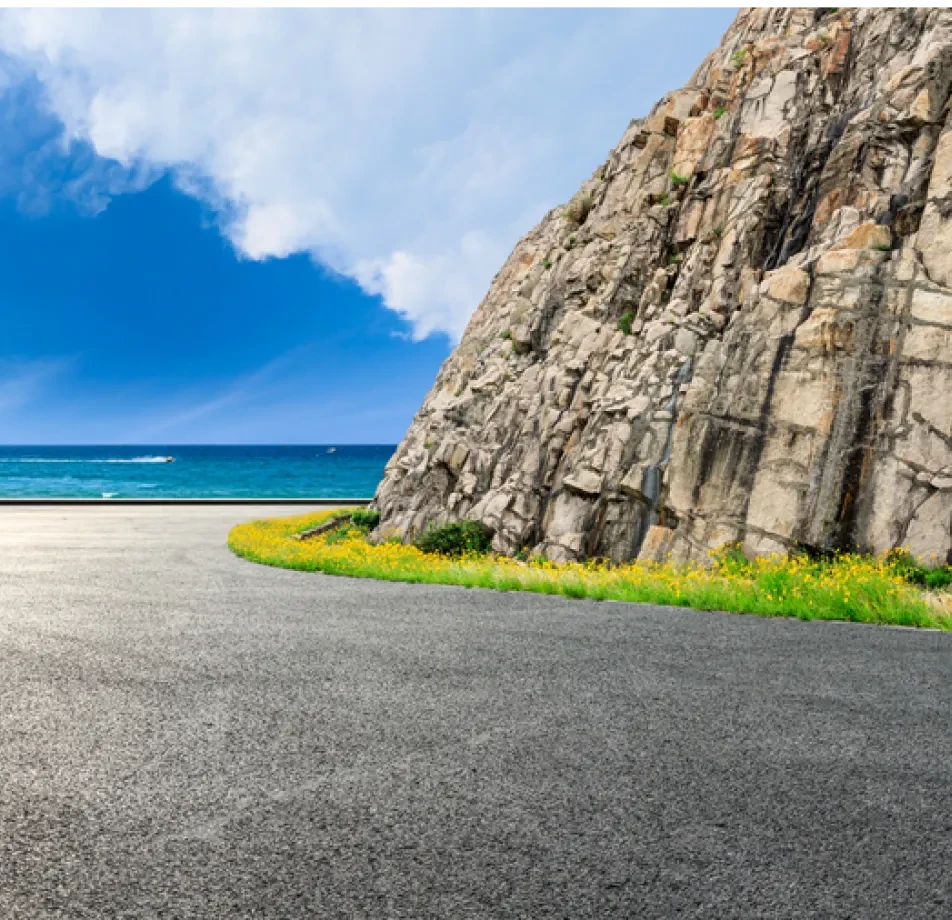
Sources
- Collaborative efforts with city councils for urban and traffic scenes.
- Additionally, forming partnerships with natural reserves and national parks allows us to capture the essence of nature more effectively.
- Furthermore, leveraging open-source imagery platforms will provide us with a wider perspective.
- To increase our reach even more, we encourage crowdsourced contributions through our dedicated applications.
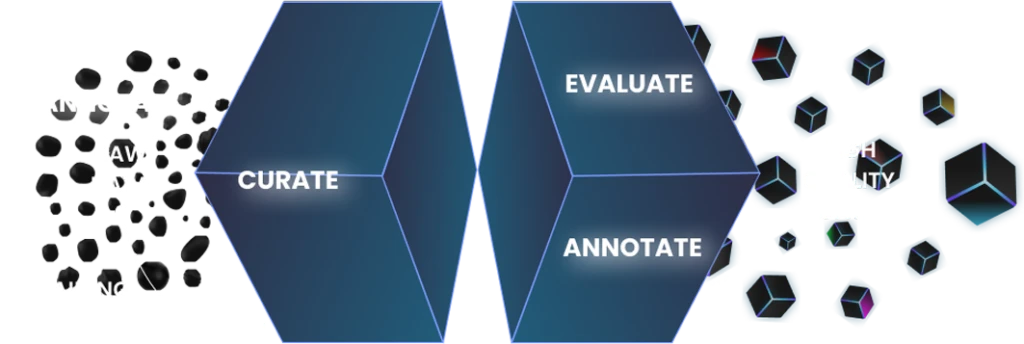
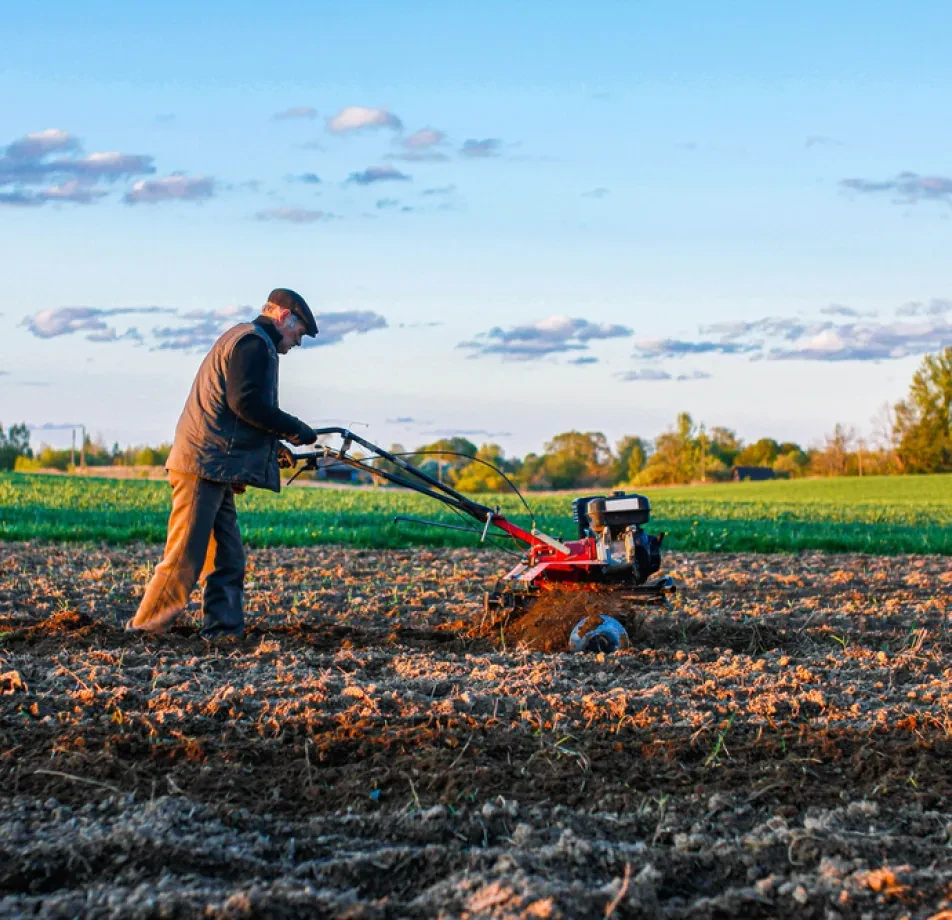
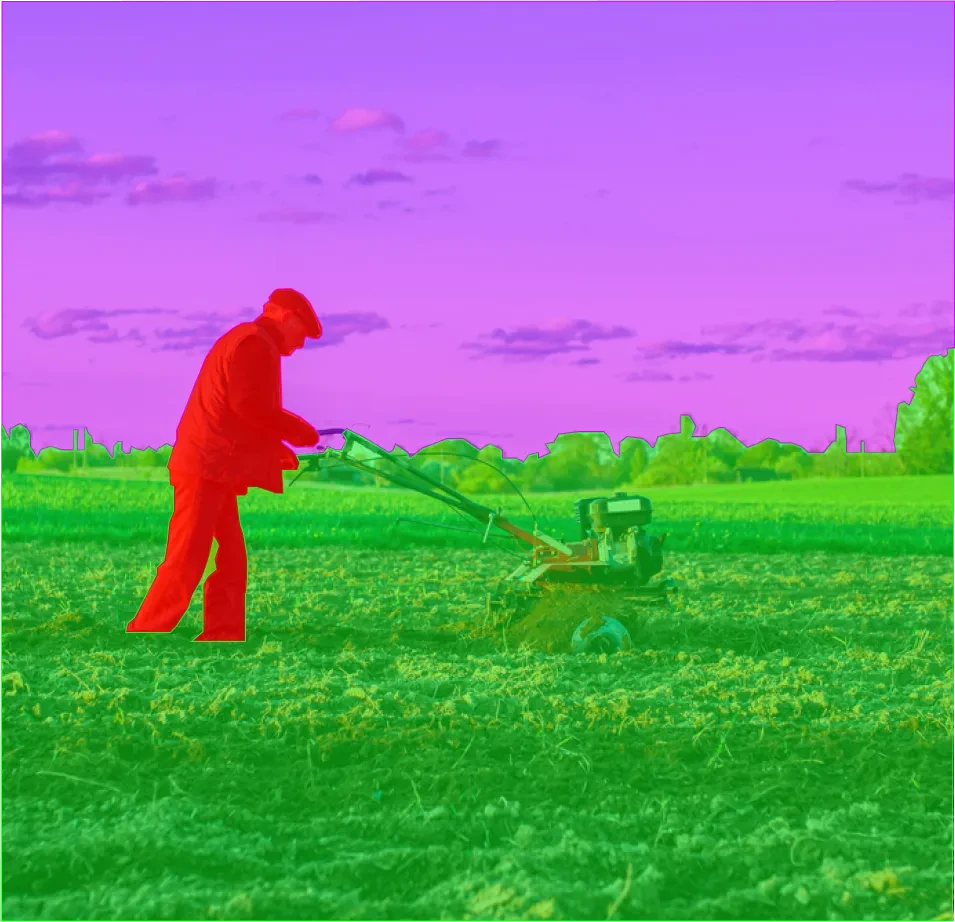
Data Collection Metrics
- Total Scenes Collected: 25,000
- Urban Scenes: 10,000
- Rural Landscapes: 4,000
- Indoor Settings: 6,000
- Natural Environments: 5,000
- Total Scenes Annotated: 20,000
Annotation Process
Stages
- Image Pre-processing: To ensure each image meets our high standards of quality, we perform color balancing, sharpening, and normalization.
- Pixel-wise Segmentation: Equipped with the latest tools, our annotators meticulously label every pixel, thereby creating a comprehensive segment map.
- Validation: A secondary team of experts then reviews the annotations to ensure utmost accuracy.
Annotation Metrics
- Total Pixel-wise Annotations: 20,000
- Average Annotation Time per Scene: 40 minutes, reflecting the intricacy of our work.
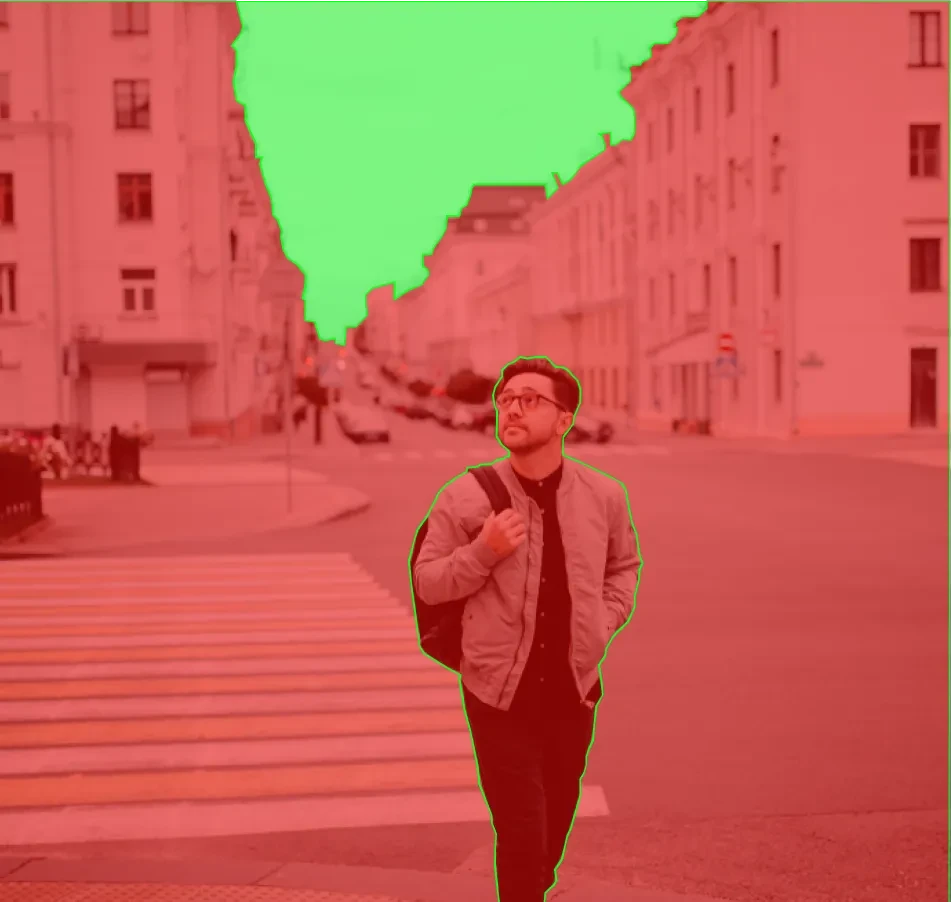
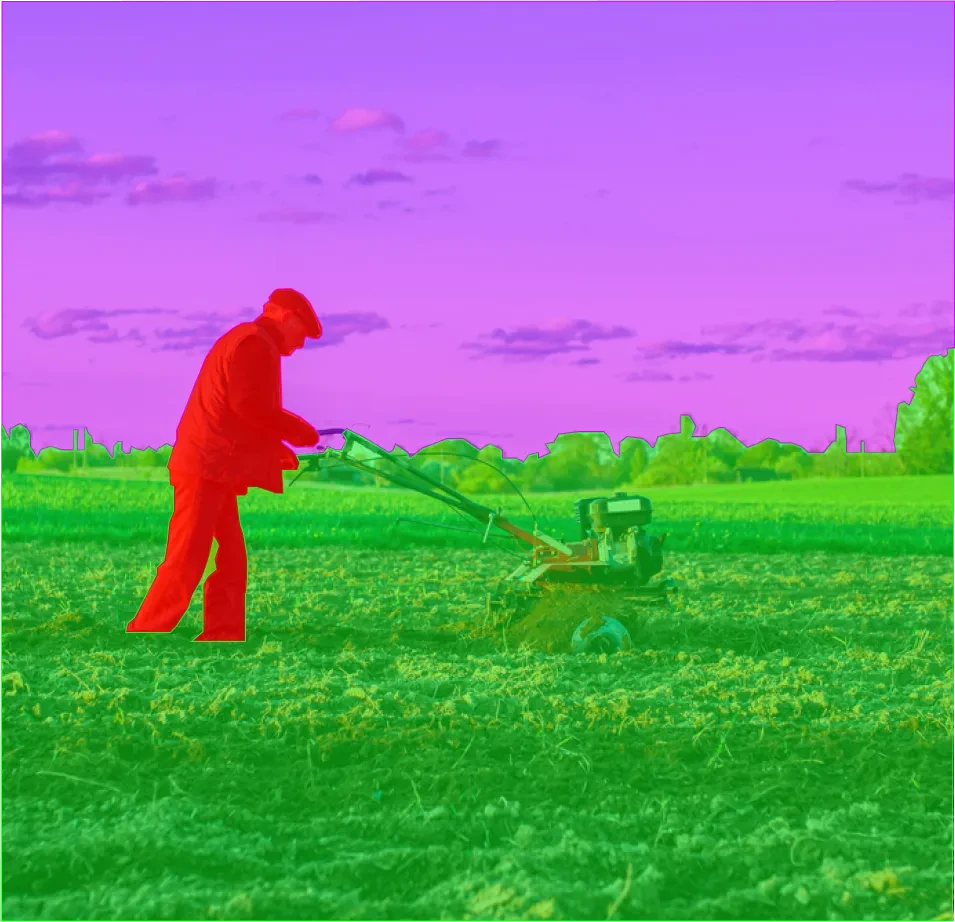
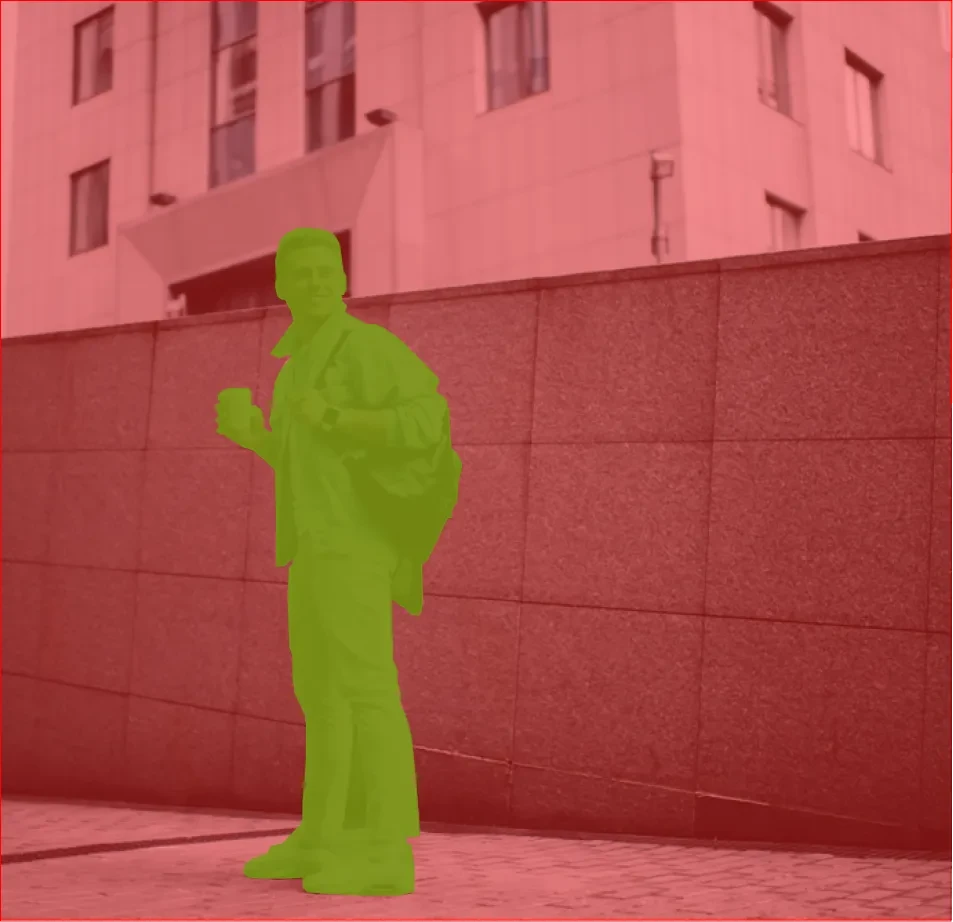
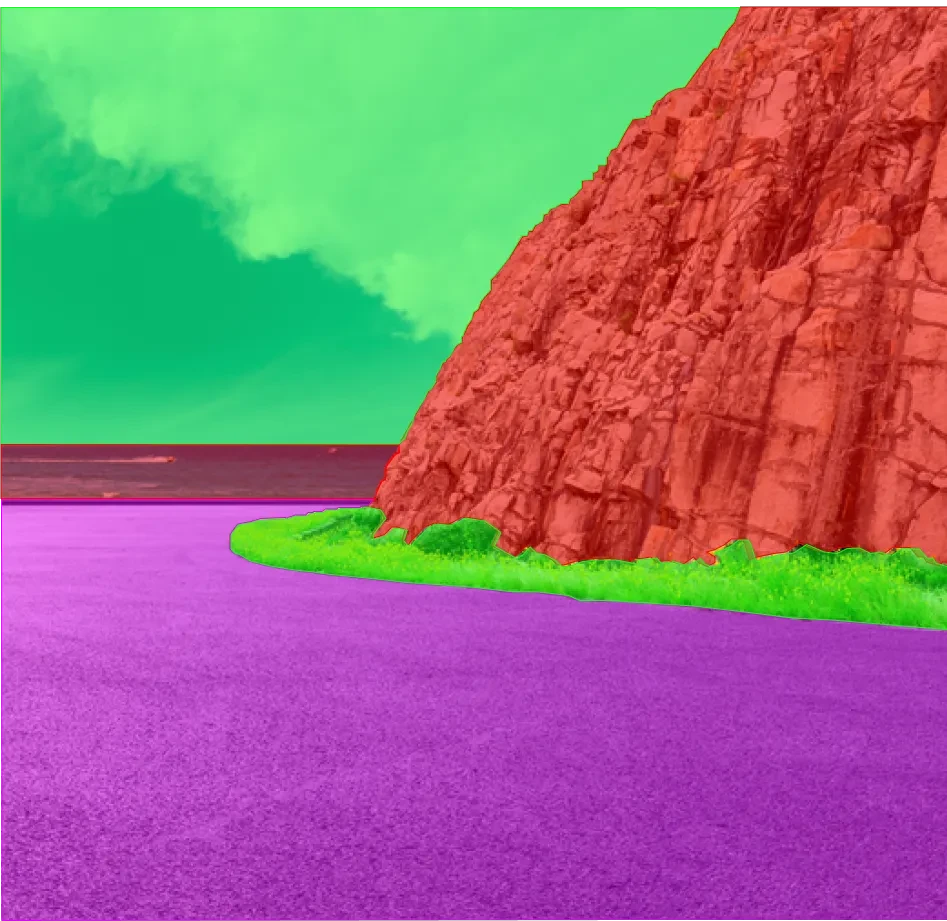
Quality Assurance
Stages
Automated Model Assessment: We employ cutting-edge models to ensure annotation accuracy. Consequently, this allows us to identify and correct any discrepancies efficiently.
Peer Review: Additionally, experienced annotators cross-check a subset of images for consistency. This step further guarantees the reliability of our annotations.
Inter-annotator Agreement: Moreover, we randomly select images for multiple annotators to review. This practice helps us establish a standard of excellence and maintain high-quality annotations.
QA Metrics
- Annotations Verified using Models: 12,500 (50% of total annotated scenes)
- Additionally, we have Peer-reviewed Annotations: 8,000 (40% of total annotated scenes)
- Moreover, we identified Inconsistencies Identified and Rectified: 1,000 (5% of total annotated scenes)
Conclusion
The Panoptic Scenes Segmentation Dataset represents a revolutionary stride in the domain of computer vision. By encompassing a broad spectrum of scenarios, ranging from bustling urban streets to serene natural vistas, this dataset aims to become the cornerstone for next-generation visual recognition systems. Its precision and depth promise to propel forward applications in urban planning, autonomous mobility, and beyond.

Quality Data Creation

Guaranteed TAT

ISO 9001:2015, ISO/IEC 27001:2013 Certified

HIPAA Compliance

GDPR Compliance

Compliance and Security
Let's Discuss your Data collection Requirement With Us
To get a detailed estimation of requirements please reach us.