OCR Data Collection
Home » Case Study » OCR Data Collection
Project Overview:
Objective
To acquire and annotate a diverse dataset suitable for OCR applications, it is essential to enhance text recognition in various contexts. Firstly, a wide range of text samples should be collected from different sources, such as printed documents, handwritten notes, and digital text, as part of the OCR Data Collection process. Furthermore, these samples should include various languages, fonts, and styles to ensure comprehensive coverage.
Scope
To begin with, we start by collecting a large number of textual images from various domains. Subsequently, we proceed to perform detailed annotation in order to achieve accurate character recognition.
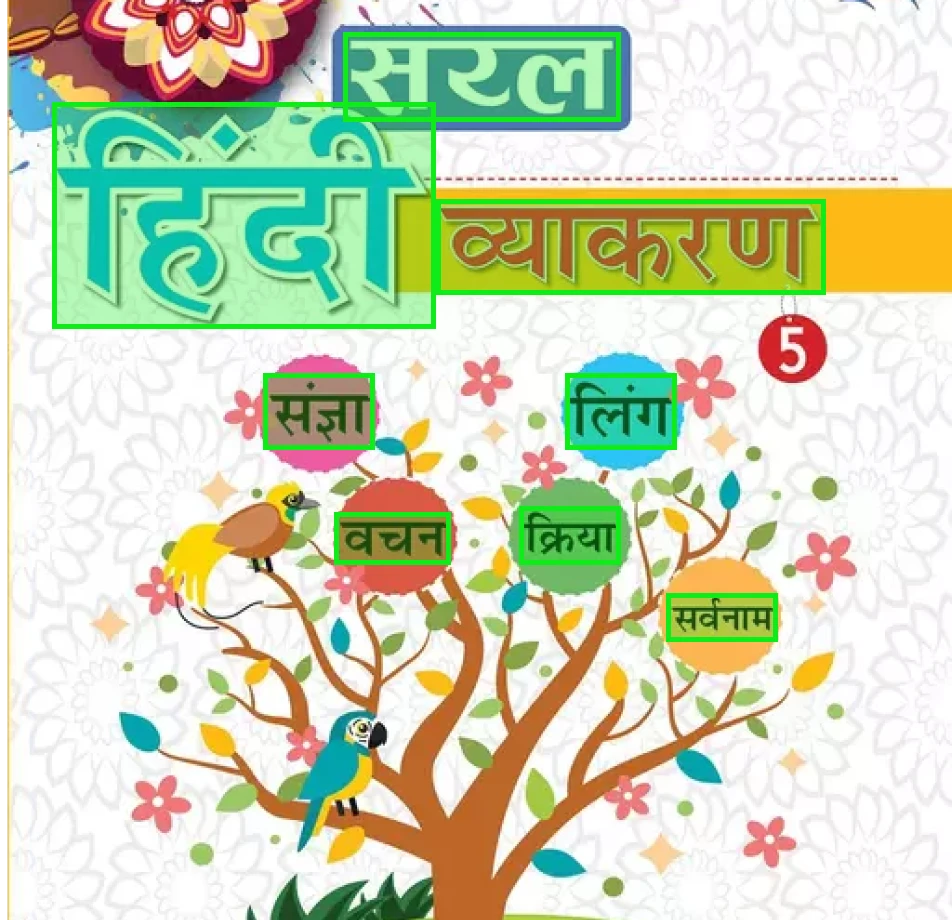
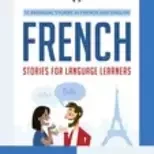
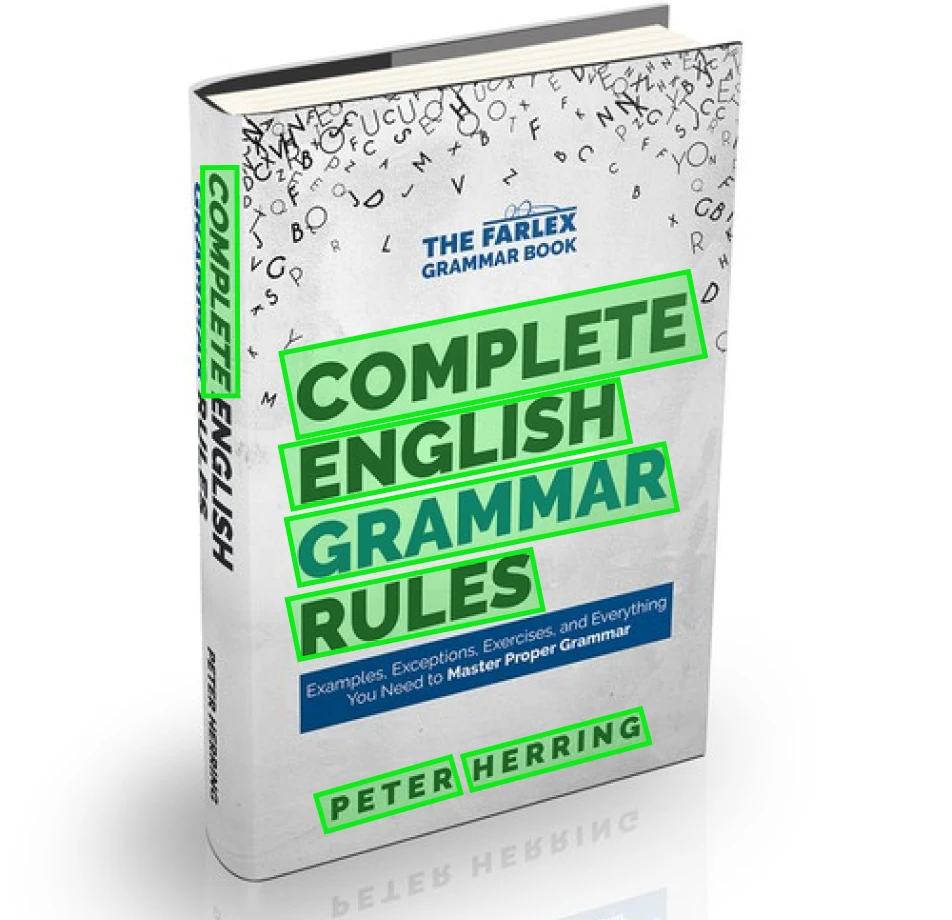
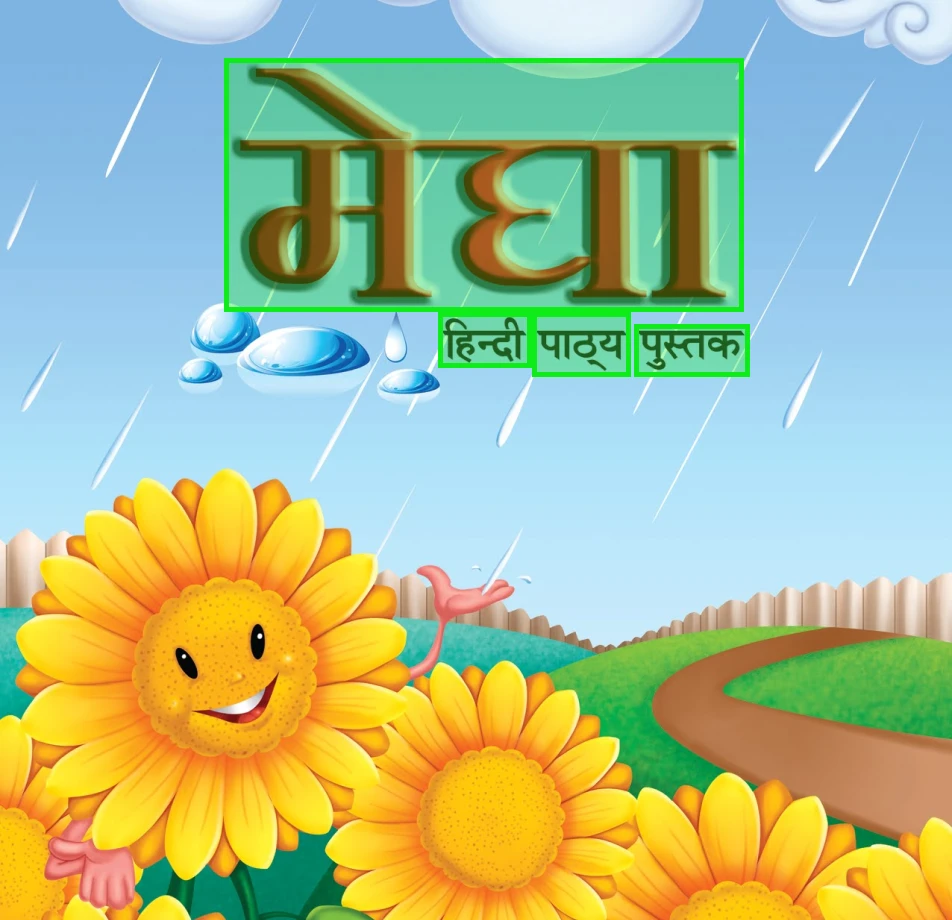
Sources
- Scanned documents, such as reports, books, and forms, were obtained, along with street signs and billboards. Additionally, these documents included handwritten notes.
- The other collected receipts were primarily printed receipts. Additionally, there were invoices. Furthermore, digital displays were used. Moreover, screens were also included.
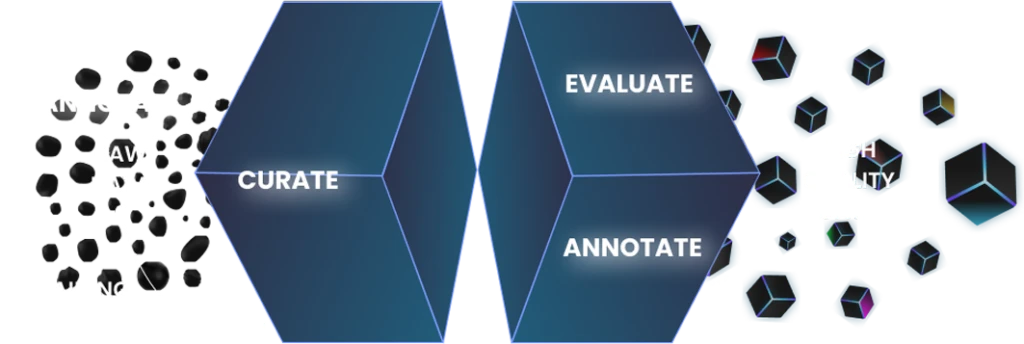
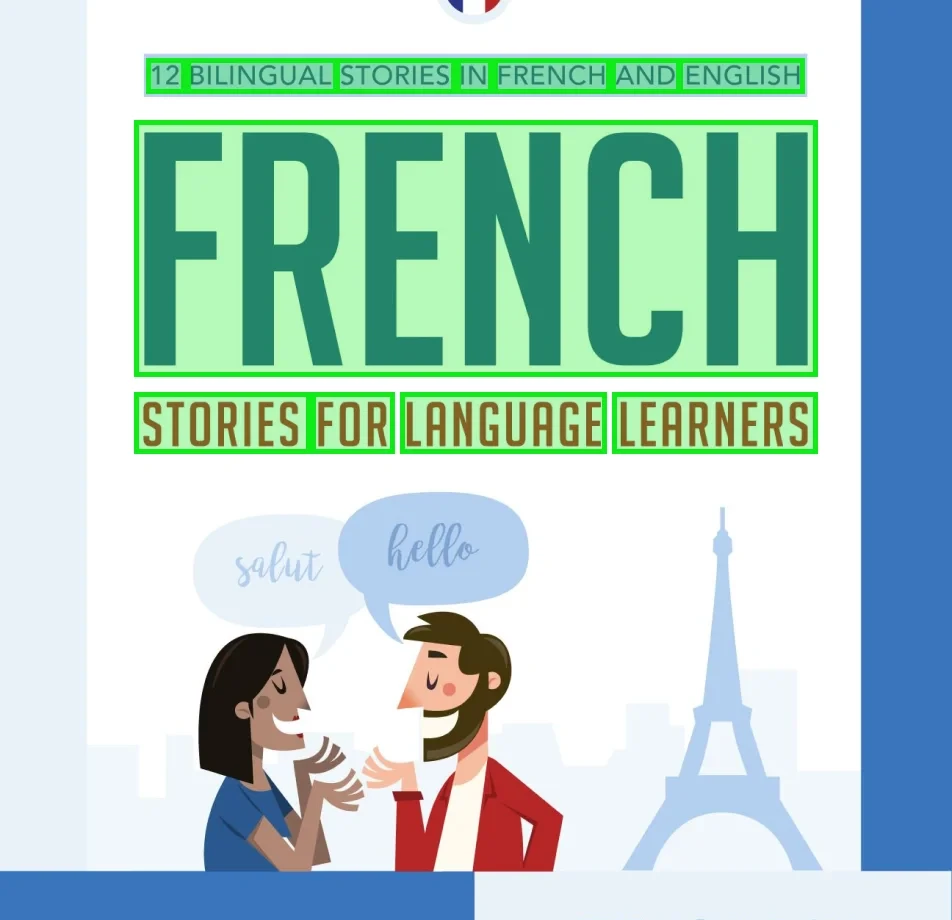
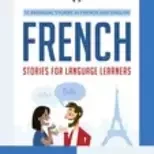
Data Collection Metrics
- Total Image Samples: 400,000
- Scanned Documents: 150,000
- Street Signs & Billboards: 50,000
- Handwritten Notes: 90,000
- Receipts & Invoices: 80,000
- Digital Displays: 30,000
Annotation Process
Stages
- Bounding Boxes: Drawing rectangles around individual words or characters helps in isolating each element for better analysis.
- Transcription: Providing textual equivalents for the identified textual images ensures the text is understandable in a digital format.
- Font & Style Tagging: Marking text based on its font type, size, and style (italic, bold) helps distinguish different parts of the text visually.
- Handwriting Classification: Distinguishing between cursive, print, and mixed styles makes it easier to analyze handwritten text accurately.
- Quality Labeling: Noting the clarity and quality of text (blurry, clear, distorted) helps in assessing the readability and usability of the text.
Annotation Metrics
- Total Annotations: 1,600,000
- Bounding Boxes: 1,000,000
- Transcriptions: 400,000
- Font & Style Tags: 100,000
- Handwriting Classifications: 50,000
- Quality Labels: 50,000
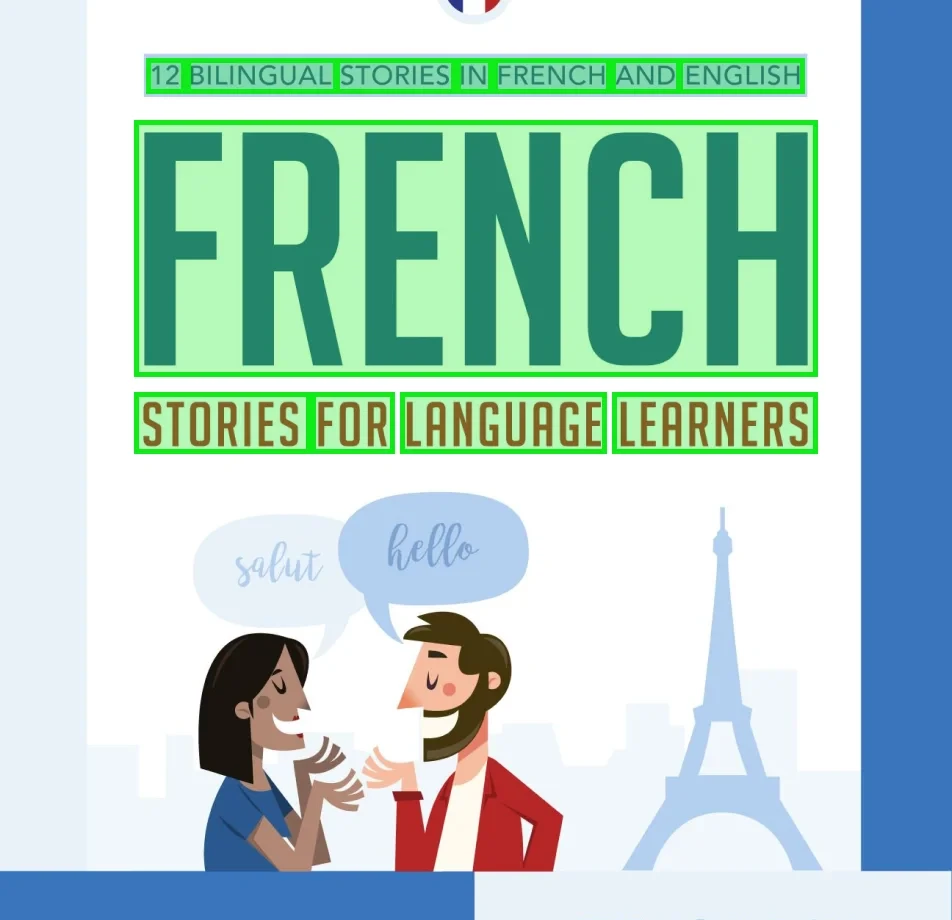
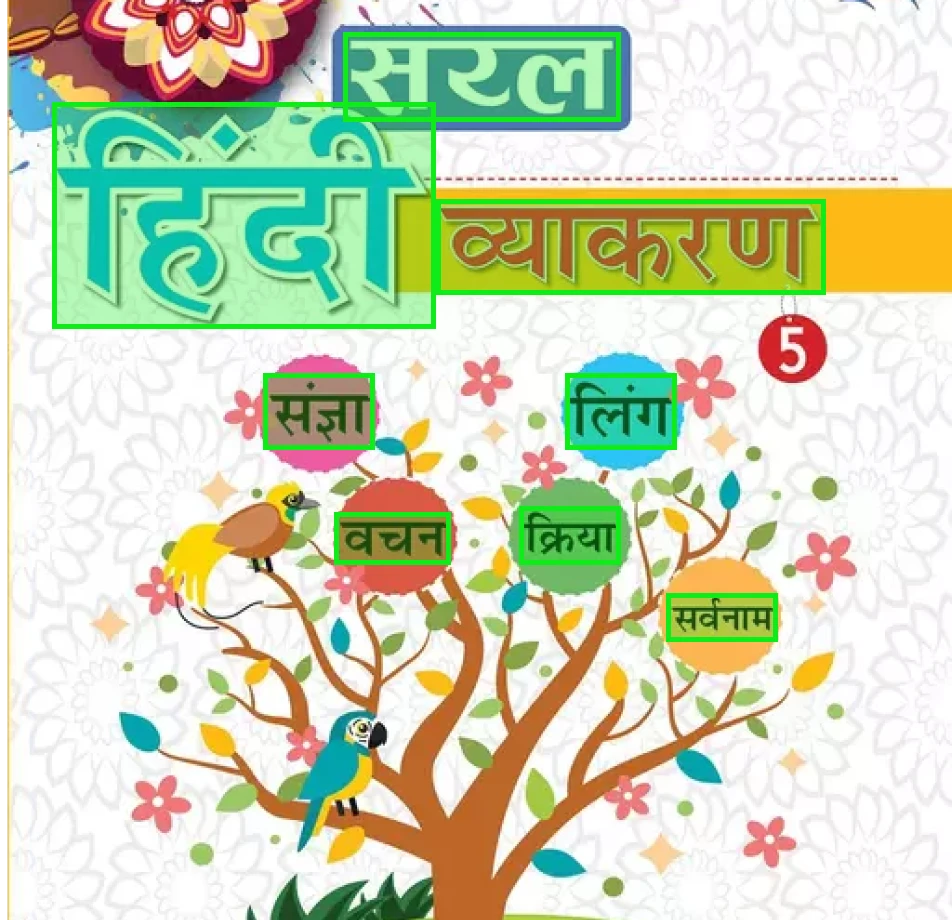
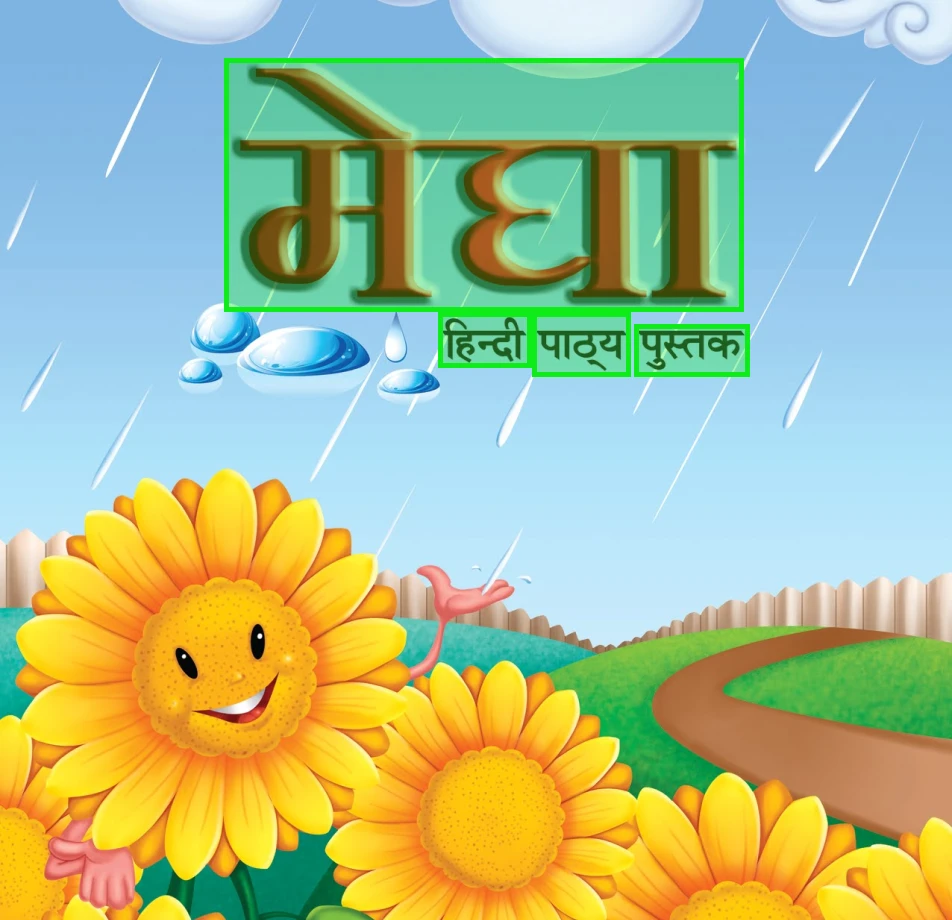
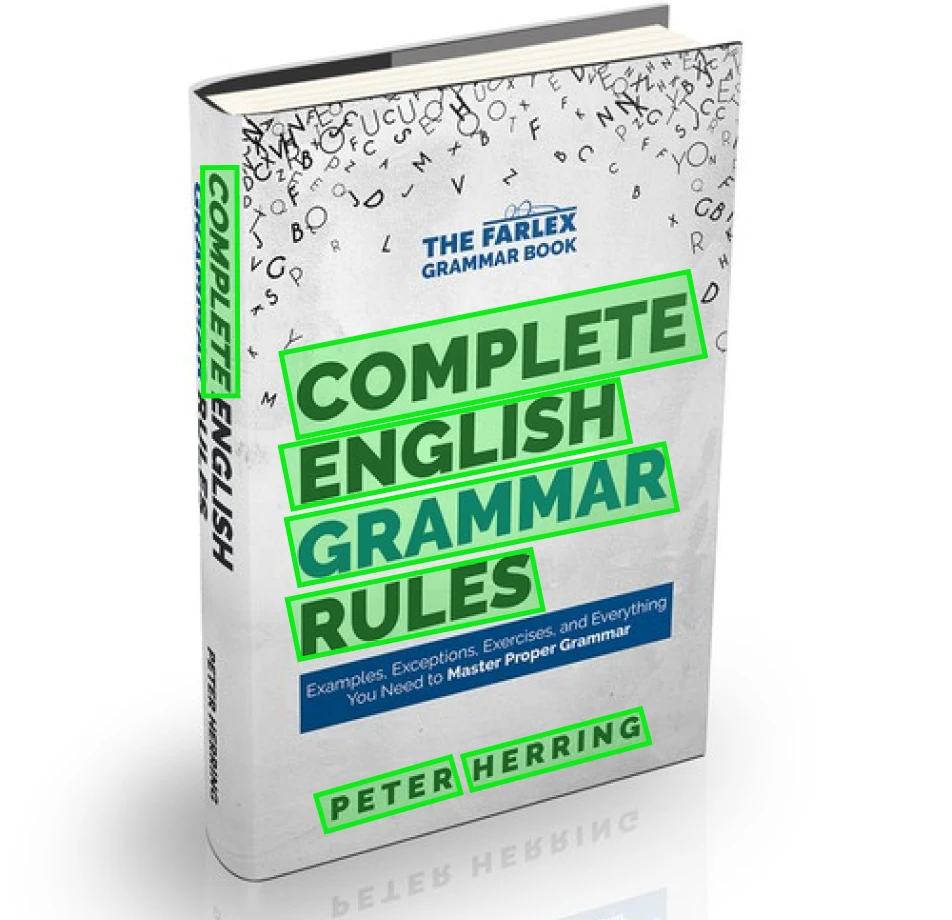
Quality Assurance
Stages
Expert Review: Moreover, we hire OCR specialists to review annotations, thus ensuring high-quality output.
Consistency Checks: Furthermore, automated checks are in place to check text and box placements.
Inter-annotator Agreement: Additionally, to maintain uniformity, multiple annotators review overlapping portions of the dataset.
QA Metrics
- Annotations Reviewed by Specialists: 160,000 (10% of total annotations)
- Inconsistencies Detected and Corrected: 32,000 (2% of total annotations)
Conclusion
The development of this comprehensive OCR dataset, created through meticulous collection and annotation methods, is poised to significantly boost text recognition capabilities. Furthermore, the dataset’s diversity ensures its applicability across various OCR scenarios. Consequently, this makes it a valuable resource for the AI and machine learning community.

Quality Data Creation

Guaranteed TAT

ISO 9001:2015, ISO/IEC 27001:2013 Certified

HIPAA Compliance

GDPR Compliance

Compliance and Security
Let's Discuss your Data collection Requirement With Us
To get a detailed estimation of requirements please reach us.