Medical Imaging Dataset
Home » Case Study » Medical Imaging Dataset
Project Overview:
Objective
Medical Imaging Dataset: The objective is to leverage the CheXpert dataset to train machine learning algorithms that can effectively identify and categorize various thoracic pathologies observed in CXR images. By achieving this, the project aims to provide radiologists with valuable decision-support tools, enabling them to make more informed and timely diagnoses.
Scope
The Chepert dataset covers a wide range of thoracic pathologies, including pneumonia, pneumothorax, pulmonary edema, and nodules, among others. Moreover, it encompasses diverse patient demographics and imaging conditions, thereby offering a comprehensive view of real-world CXR interpretations.
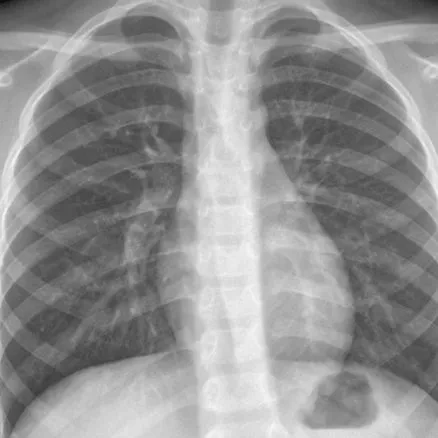
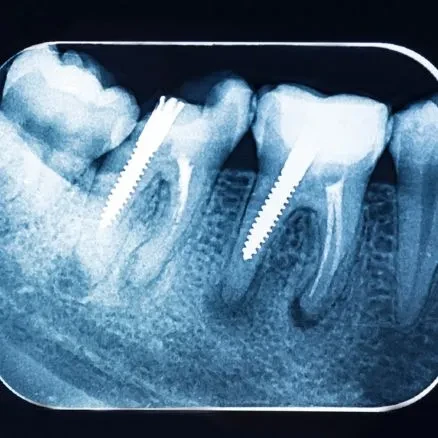
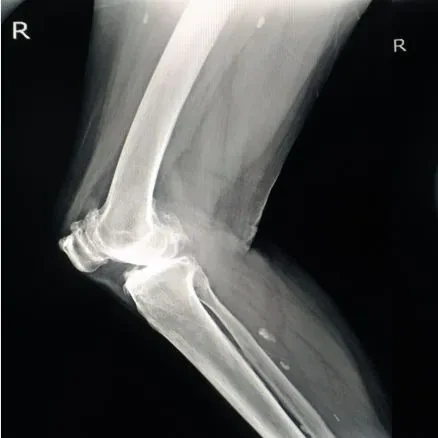
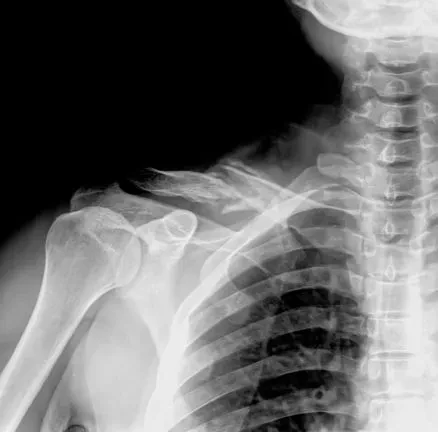
Sources
- The dataset comprises CXR images obtained from various medical institutions, capturing a diverse range of patient cases and imaging settings. Additionally, these images provide a comprehensive view of different pathologies and aid in understanding the complexities of thoracic conditions.
- Annotated Labels: Moreover, detailed labels annotate each CXR image, providing ground truth for model training and evaluation, indicating the presence or absence of specific thoracic pathologies.
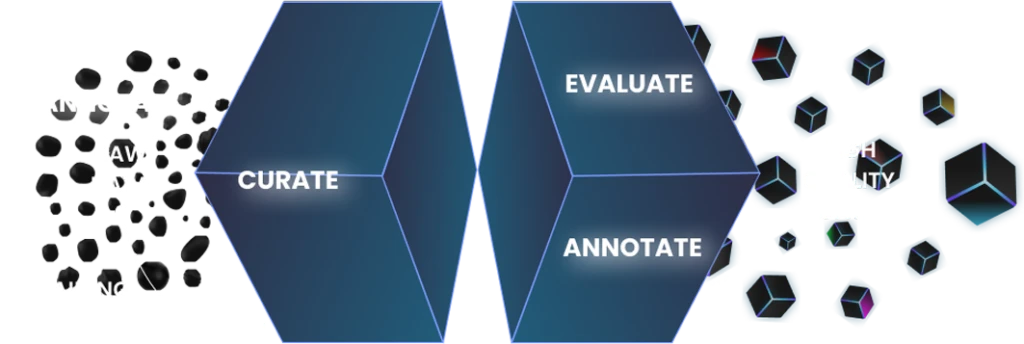
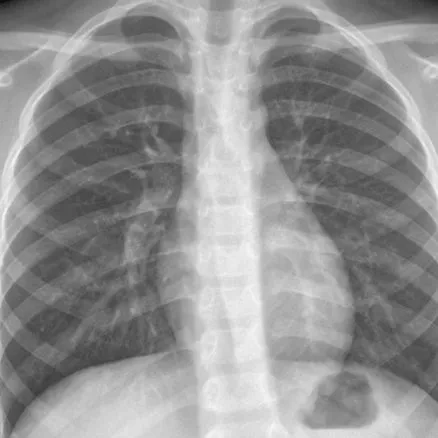
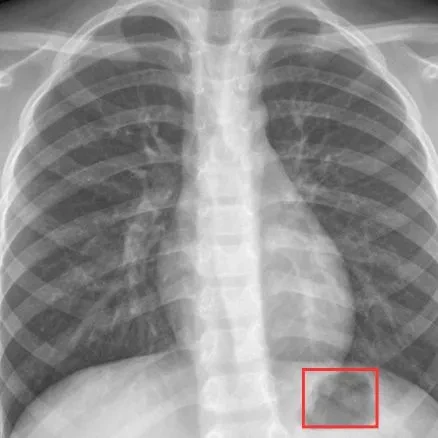
Data Collection Metrics
- Total Data Collected: Over 200,000 CXR images. the dataset provides a robust foundation.
- Annotated Data for ML Training: Specifically, 180,000 CXR images feature meticulously curated annotations for machine learning training purposes, ensuring precision and relevance.
Annotation Process
Stages
- Total Data Collected: Over 200,000 CXR images.
- Annotated Data for ML Training: Additionally, meticulously curated annotations accompany 180,000 CXR images, making them available for machine learning training purposes.
Annotation Metrics
- In the dataset, a total of 14 thoracic pathologies were annotated, thereby ensuring comprehensive coverage of diagnostic categories.
- Localization Accuracy: The annotations achieved high precision in localizing pathologies within CXR images, thus aiding clinicians in identifying relevant abnormalities.
- Additionally, augmented data variants contributed to improved model performance and resilience to variations in imaging conditions.
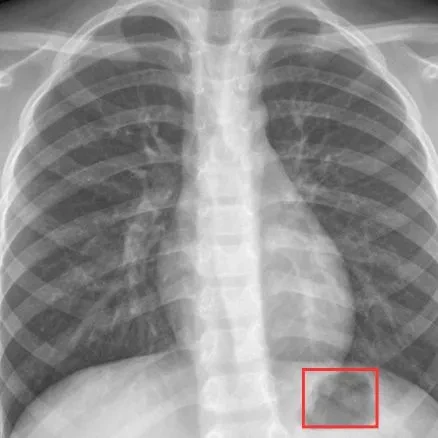
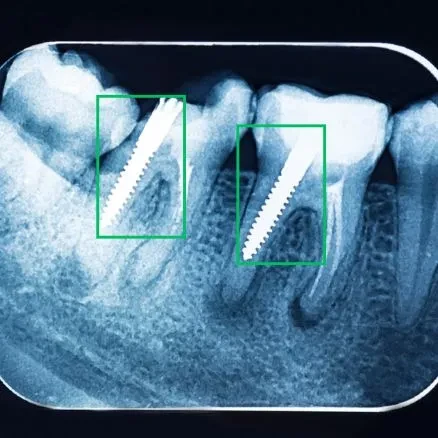
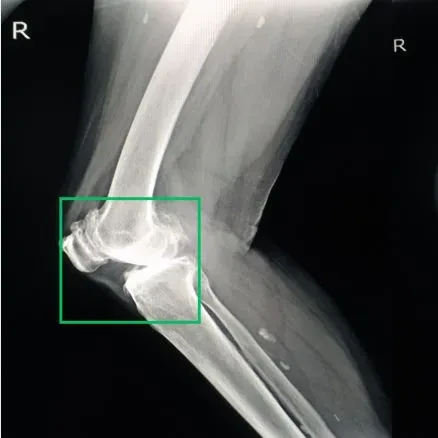
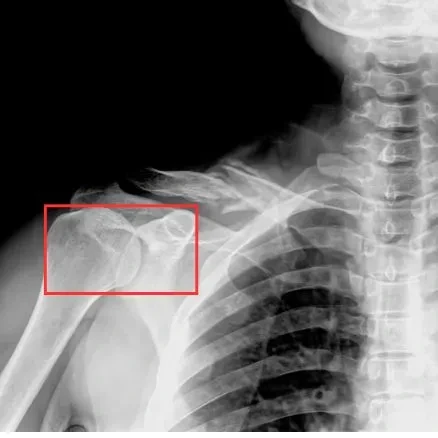
Quality Assurance
Stages
Continuous Model Evaluation: The project implemented thorough testing and validation protocols to ascertain the accuracy and dependability of the trained models in detecting thoracic pathologies. Regular assessments were conducted to monitor model performance and identify areas for refinement.
Clinical Validation: To validate the diagnostic accuracy and clinical relevance of the models, their predictions were meticulously compared with interpretations made by expert radiologists. This comparative analysis provided valuable insights into the models’ efficacy in assisting healthcare professionals in making accurate diagnoses.
Ethical Compliance: Adhering to ethical guidelines and regulations concerning patient privacy was paramount throughout the project. Stringent measures were implemented to ensure the responsible handling of medical imaging data, safeguarding patient confidentiality and privacy rights.
QA Metrics
- Diagnostic Accuracy: The developed models demonstrated high accuracy in detecting and classifying thoracic pathologies, with performance metrics exceeding industry standards. Moreover, these models showcased performance metrics exceeding industry standards, affirming their reliability and effectiveness.
- Clinical Utility:
Transition words can help connect ideas and improve the flow of the text. Let’s integrate them into the provided content, Moreover, radiologists reported enhanced diagnostic efficiency and confidence when utilizing the model predictions as a supplementary aid in CXR interpretation.
- Patient Privacy Protection: Stringent measures were implemented to anonymize patient data and uphold confidentiality, thereby meeting regulatory requirements.
Conclusion
The utilization of the CheXpert dataset has significantly advanced the field of medical imaging diagnostics, particularly in chest X-ray interpretation. By harnessing machine learning techniques and leveraging annotated CXR data, the project has facilitated more accurate and efficient identification of thoracic pathologies. Consequently, this advancement ultimately contributes to improved patient care and outcomes in clinical practice.

Quality Data Creation

Guaranteed TAT

ISO 9001:2015, ISO/IEC 27001:2013 Certified

HIPAA Compliance

GDPR Compliance

Compliance and Security
Let's Discuss your Data collection Requirement With Us
To get a detailed estimation of requirements please reach us.