Lips Segmentation Dataset
Home » Case Study » Lips Segmentation Dataset
Project Overview:
Objective
Scope
Collate a vast range of images that highlight lips in various lighting conditions, emotions, orientations, and lipstick applications. Each image will be finely annotated to delineate the boundaries of the lips. Moreover, collect images of lips in different lighting conditions to show how shadows and highlights affect their appearance. Additionally, gather images capturing a spectrum of emotions, from smiles to pouts, to depict how lips change with facial expressions.
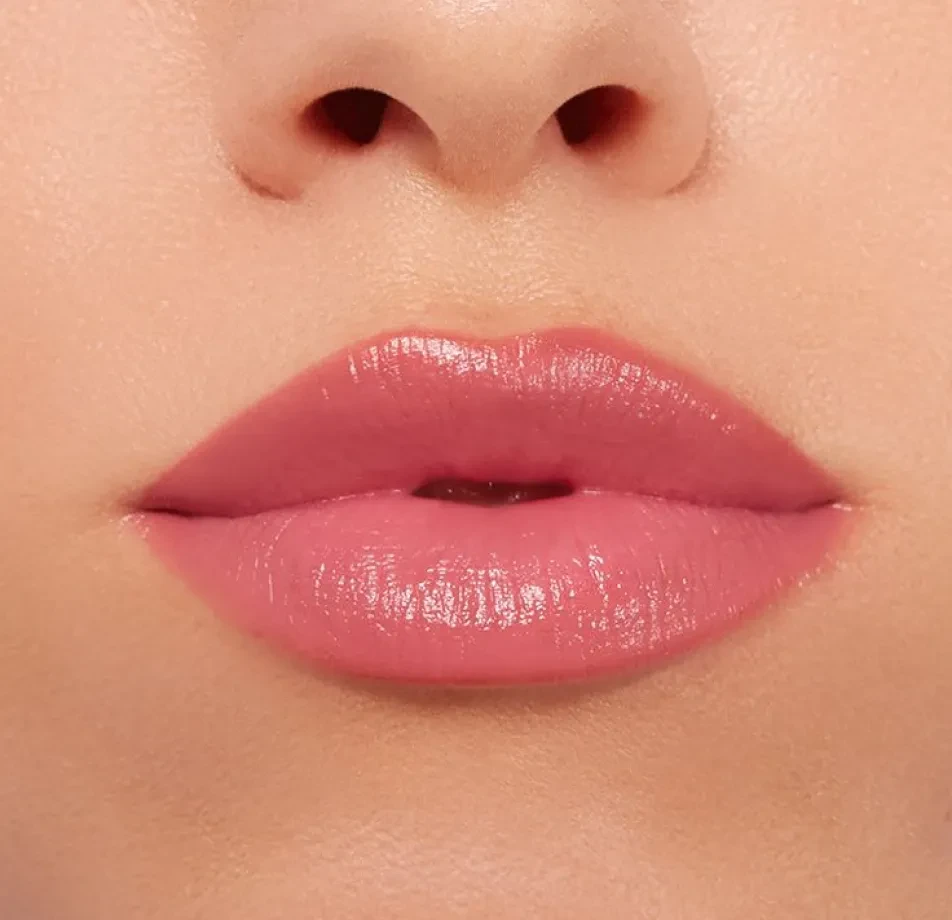
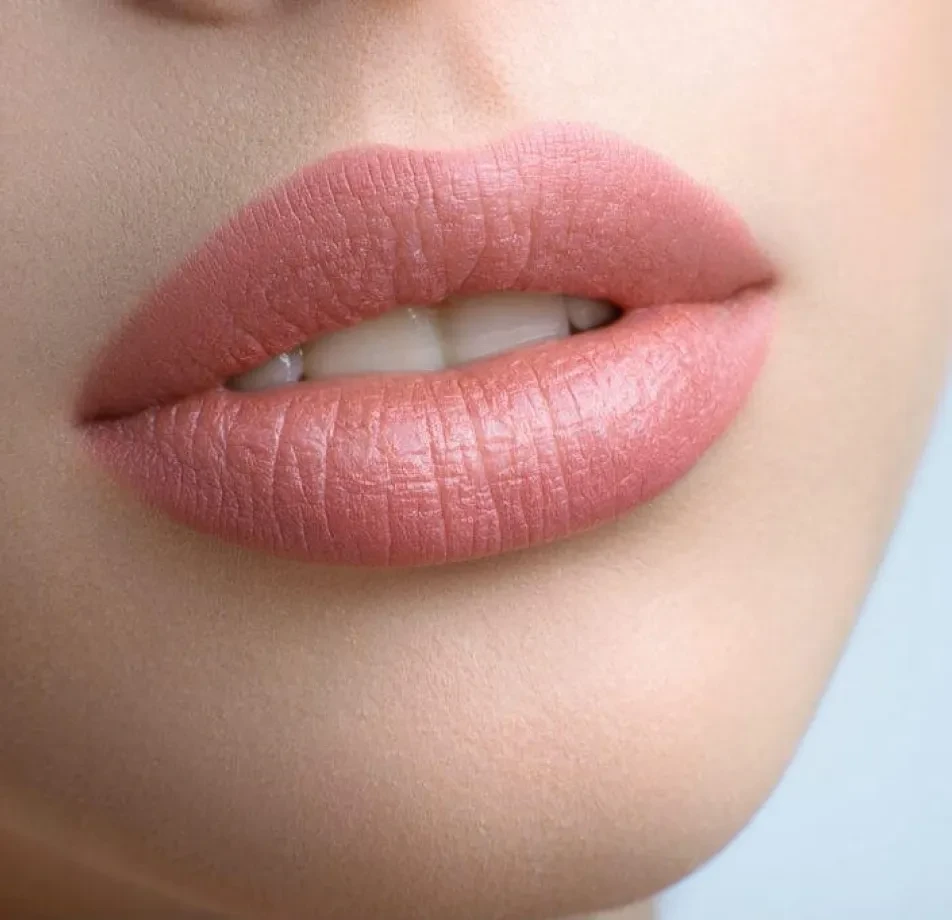
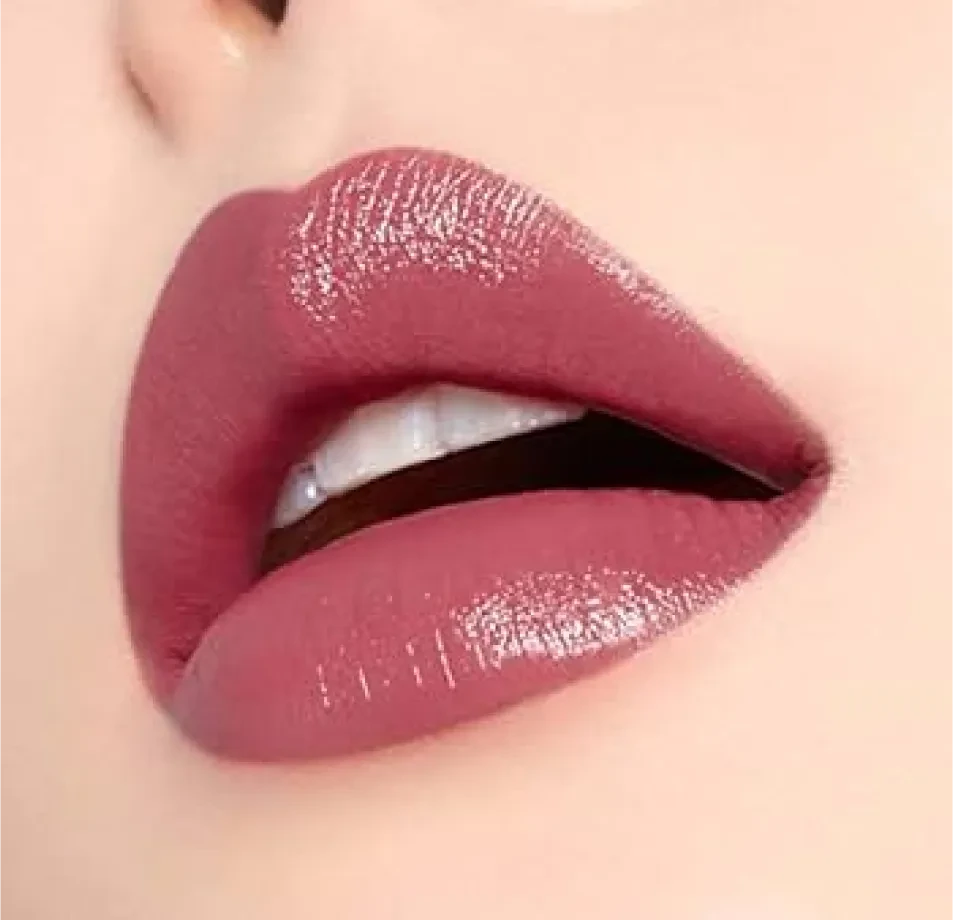
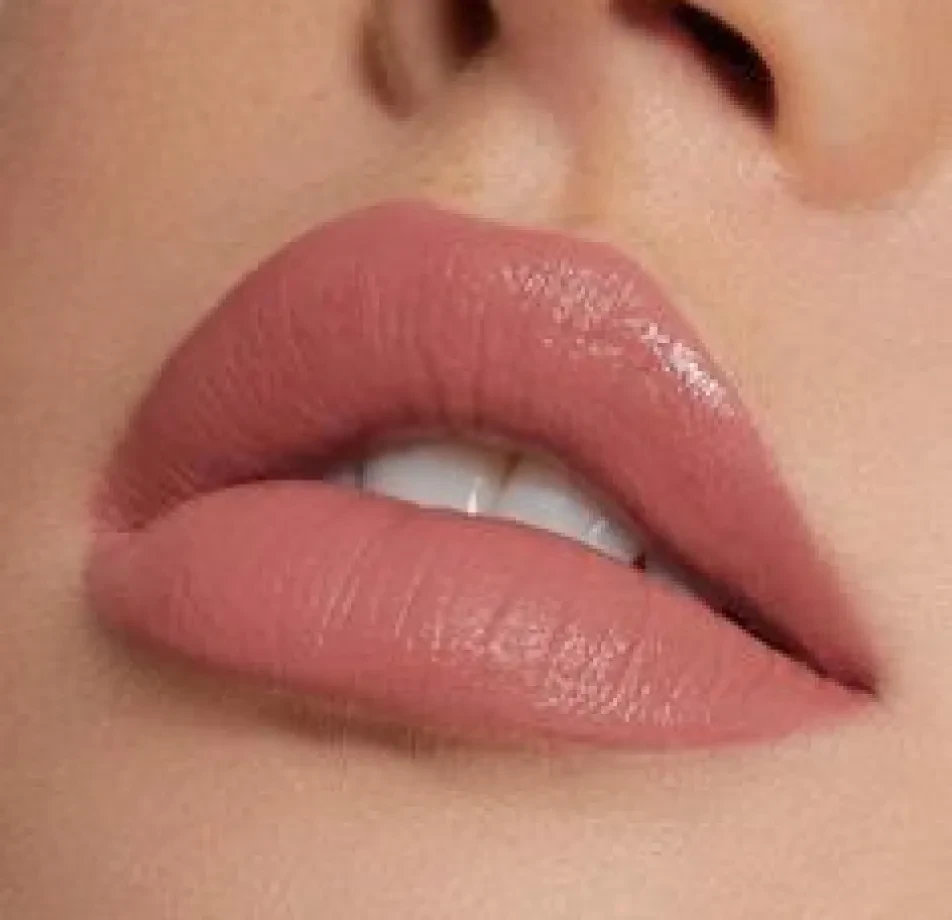
Sources
- Engaged in collaborative initiatives with dermatologists and cosmetic surgeons, thereby resulting in a meticulously collected and successfully curated range of resources.
- Crowdsourced inputs from global participants have been meticulously gathered, consequently ensuring diversity in lip shapes, sizes, and colors within the dataset.
- Worked closely with the movie and entertainment industries to capture a wide array of emotional expressions. This collaboration significantly contributed to the successful collection and thoughtful curation of a diverse set of visuals.
- Partnered with makeup artists and beauty schools, we collaborated to gather a carefully collected and successfully curated assortment of beauty-related content.
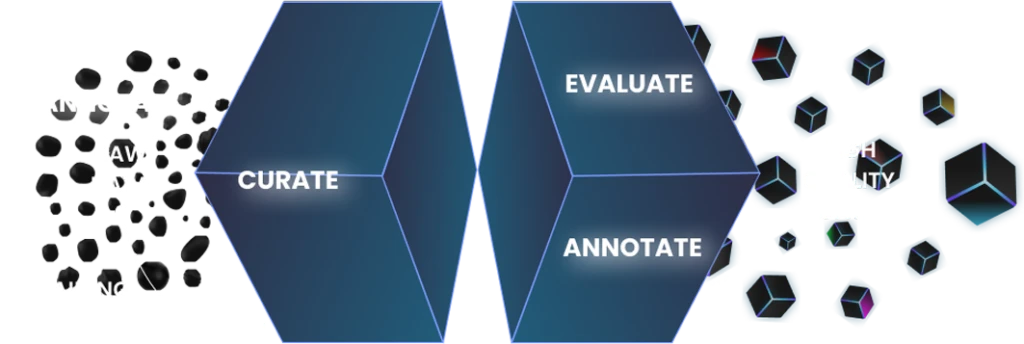
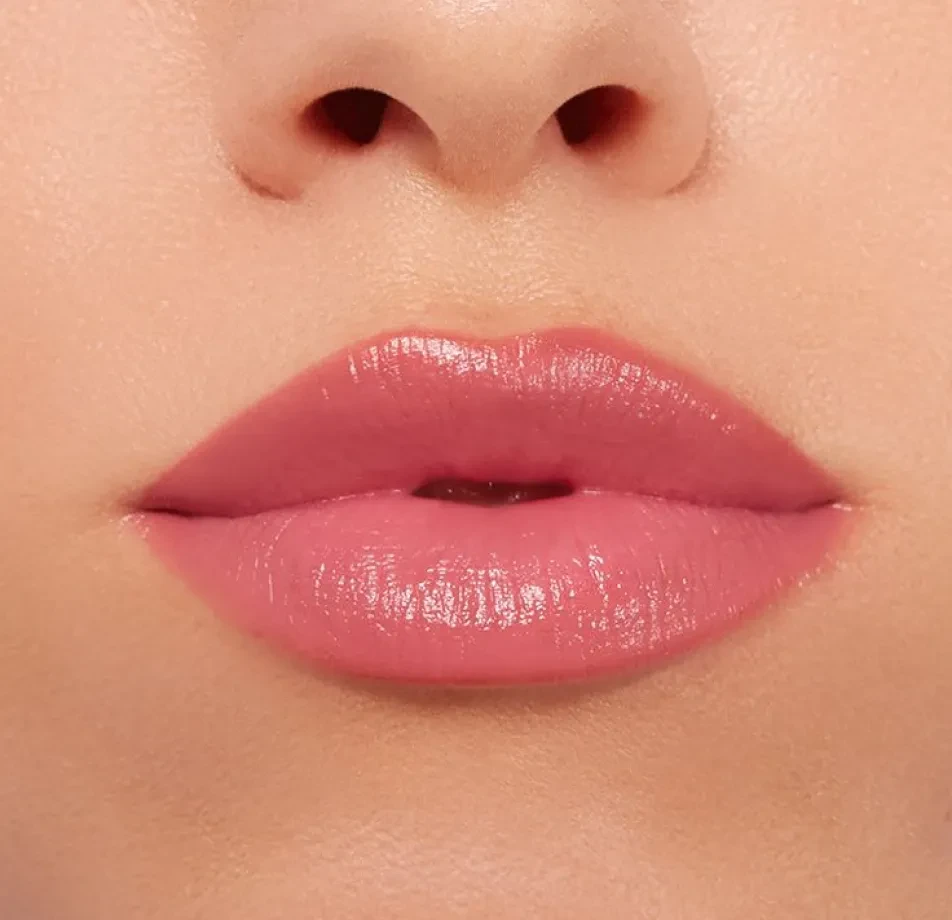
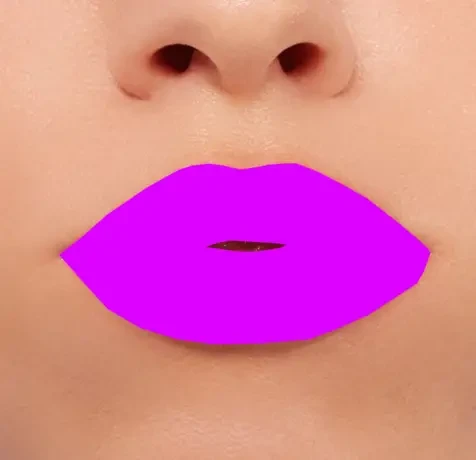
Data Collection Metrics
- Total Images: 25,000
- Neutral Expression: 10,000
- Varied Emotions: 8,000
- Lipstick Applications: 5,000
- Medical Cases: 2,000
Annotation Process
Stages
- Image Pre-processing:
Adjustments for clarity, contrast, and color are necessary to ensure consistent quality across all images. Additionally, incorporating more transition words can help maintain a smooth flow and coherence in the content while achieving the same overall effect.
 - Pixel-wise Segmentation: Following this step, pixel-wise segmentation is conducted. Here, annotators employ advanced tools to meticulously trace the exact contour and interior of the lips. This process effectively differentiates them from the surrounding facial regions.
- Validation: A validation step is conducted subsequently, where a secondary set of experts reviews each annotation to confirm its accuracy.
Annotation Metrics
- Total Pixel-wise Annotations: 25,000 (One for each image)
- Average Annotation Time per Image: Given the detailed nature of lip boundaries, it typically takes approximately 7 minutes to complete the process.
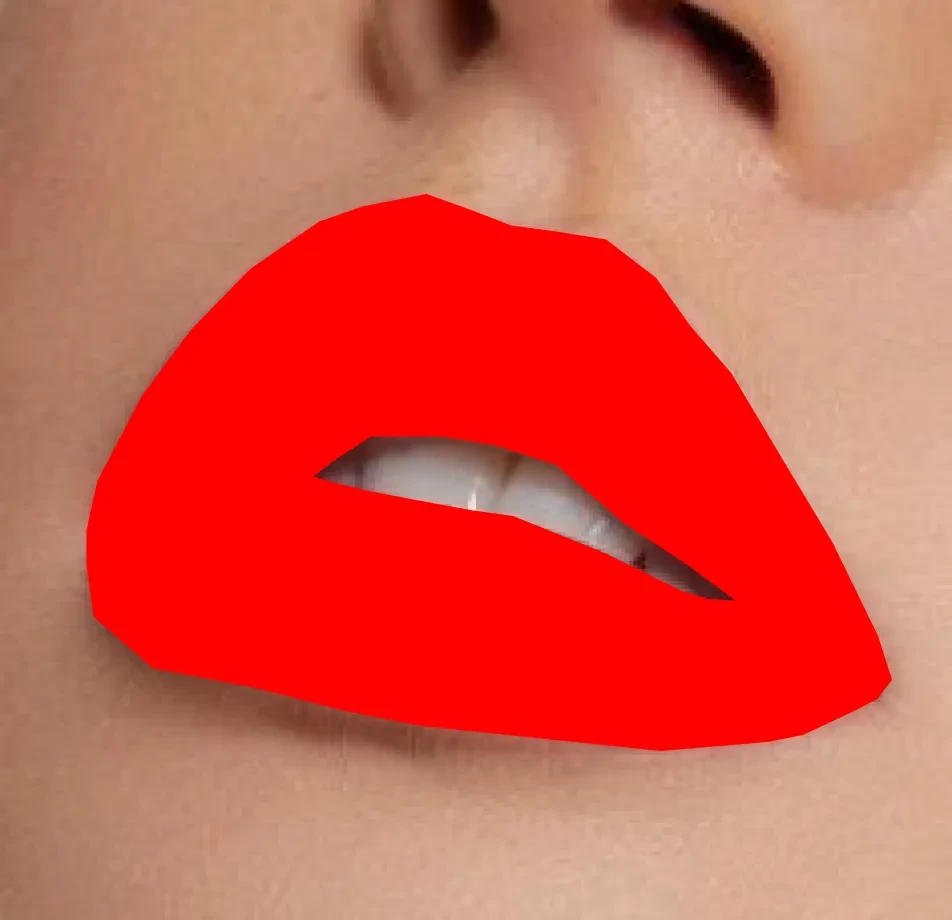
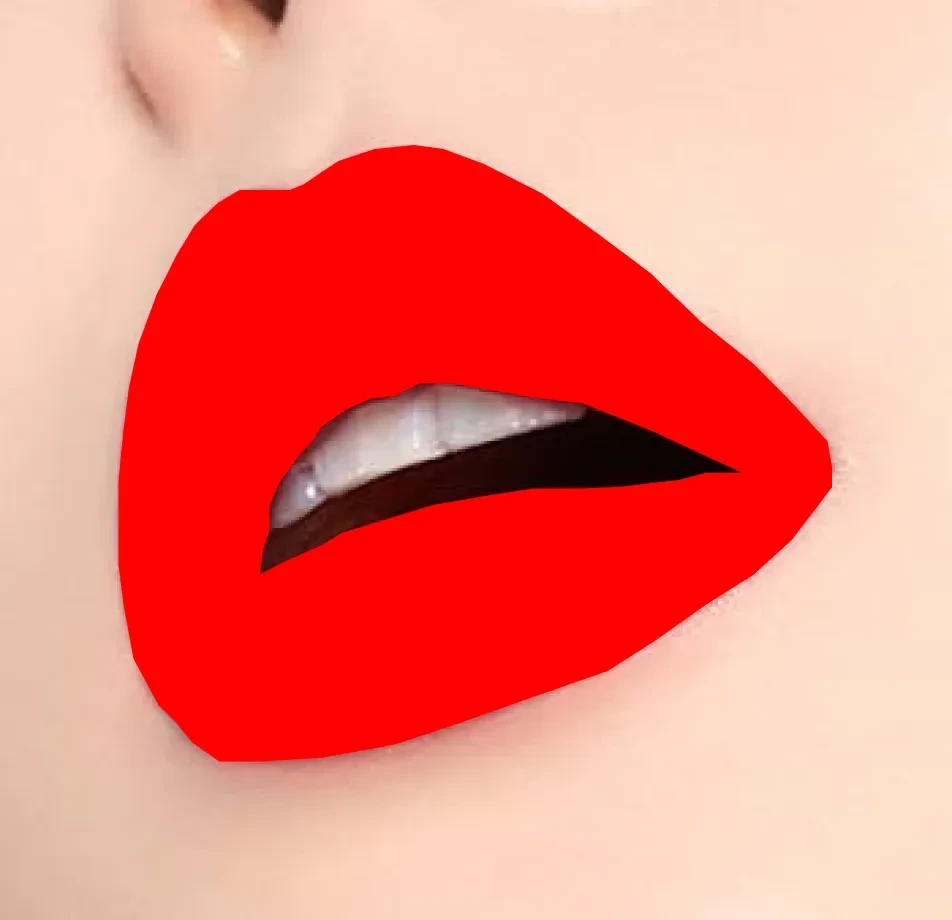
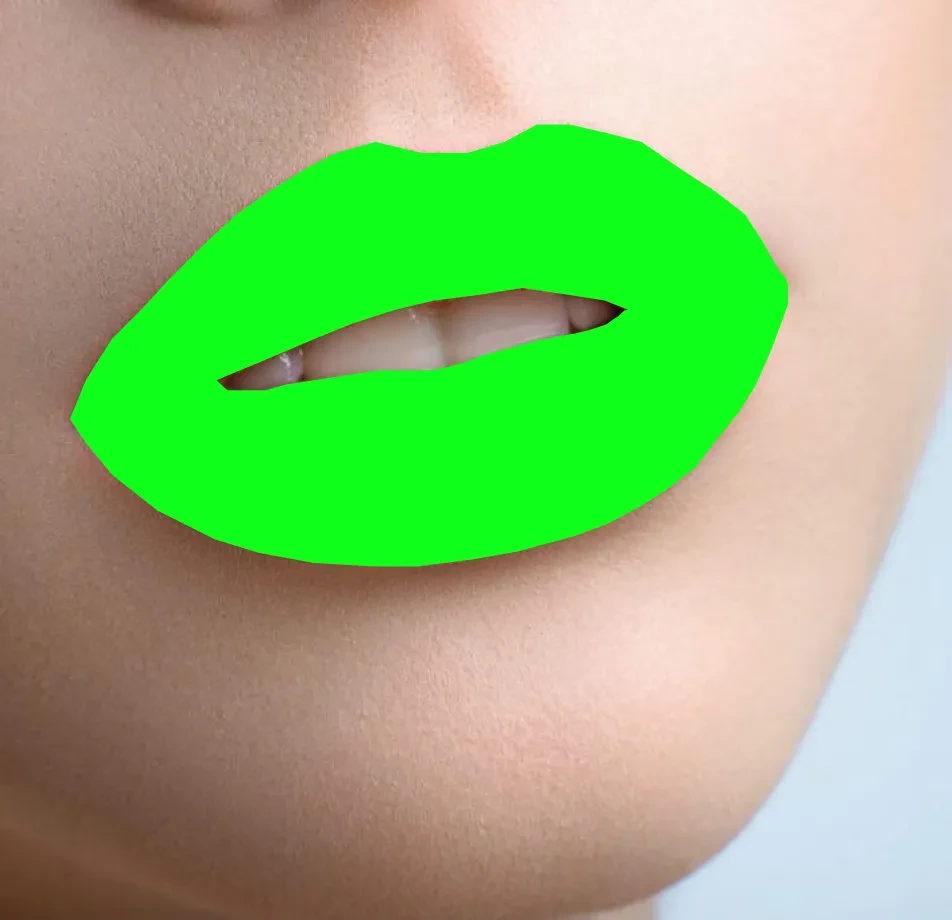
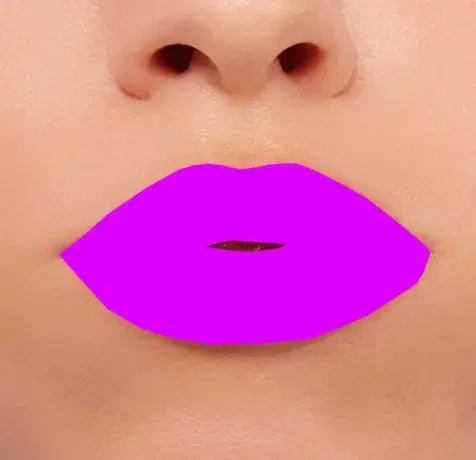
Quality Assurance
Stages
Automated Model Verification: Preliminary lip segmentation models, in addition, cross-check annotations to identify potential discrepancies.
Peer Review: Additionally, experienced annotators oversee a random sample to maintain a consistent quality benchmark.
Inter-annotator Agreement: Moreover, a subset of images is annotated multiple times to ensure consistency and clarity in the dataset’s guidelines.
QA Metrics
- Annotations Reviewed using Models: 12,500 (50% of total images)
- Peer-reviewed Annotations: 7,500 (30% of total images)
- Inconsistencies Identified and Addressed: 250 (1% of total images)
Conclusion
The Lips Segmentation Dataset serves as a cornerstone for a myriad of applications. From virtual makeup trials to health diagnostics, its extensive array of images and meticulous annotations ensure that AI models trained on it will have an unparalleled understanding of human lips in all their multifaceted roles and expressions. Consequently, this dataset significantly enhances the precision and reliability of AI applications. Additionally, the diversity of images included provides a comprehensive representation of various lip shapes, colors, and conditions.

Quality Data Creation

Guaranteed TAT

ISO 9001:2015, ISO/IEC 27001:2013 Certified

HIPAA Compliance

GDPR Compliance

Compliance and Security
Let's Discuss your Data collection Requirement With Us
To get a detailed estimation of requirements please reach us.