Large-scene Multi-camera Person Re-identification dataset
Home » Case Study » Large-scene Multi-camera Person Re-identification dataset
Project Overview:
Objective
To create a robust dataset for person re-identification across expansive scenes using multi-camera setups, it is imperative to include transition words for coherence. Consequently, this dataset will be pivotal in advancing security and surveillance systems. Furthermore, it will play a significant role in nurturing smart cities and optimizing crowd management technologies.
Scope
To enhance person re-identification accuracy, we need to gather visuals from vast areas by utilizing various camera angles and resolutions. Consequently, this ensures comprehensive coverage of different scenarios, lighting conditions, and crowd densities.
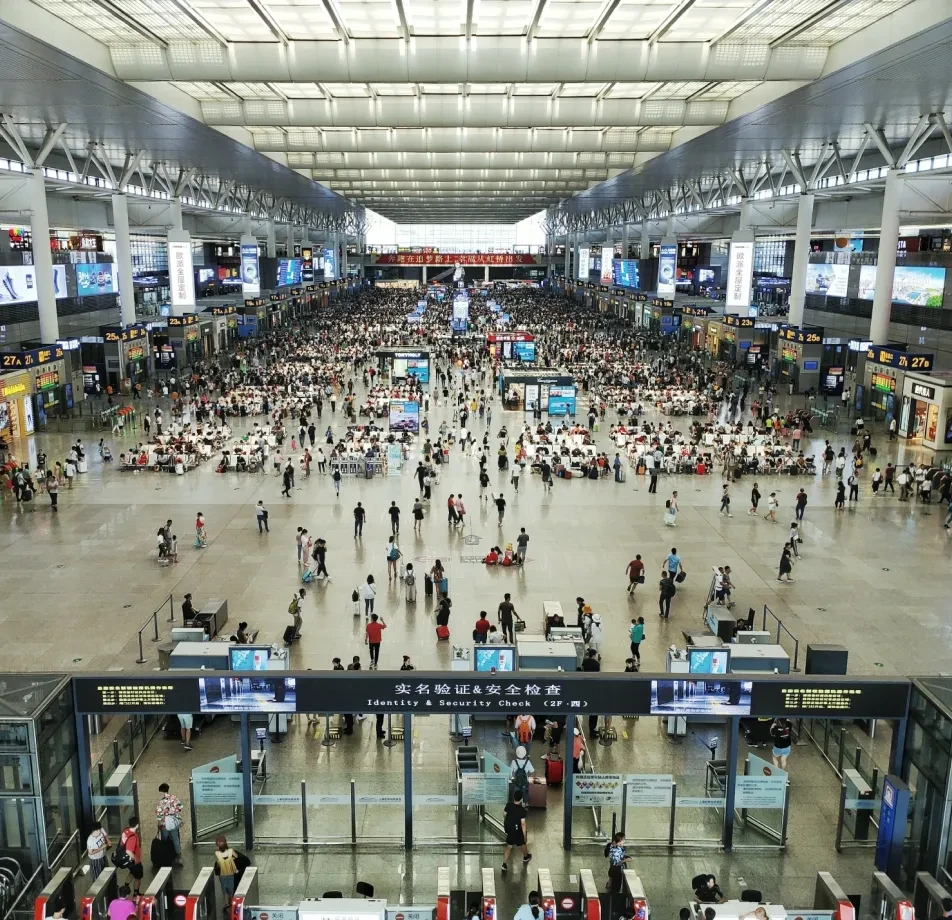
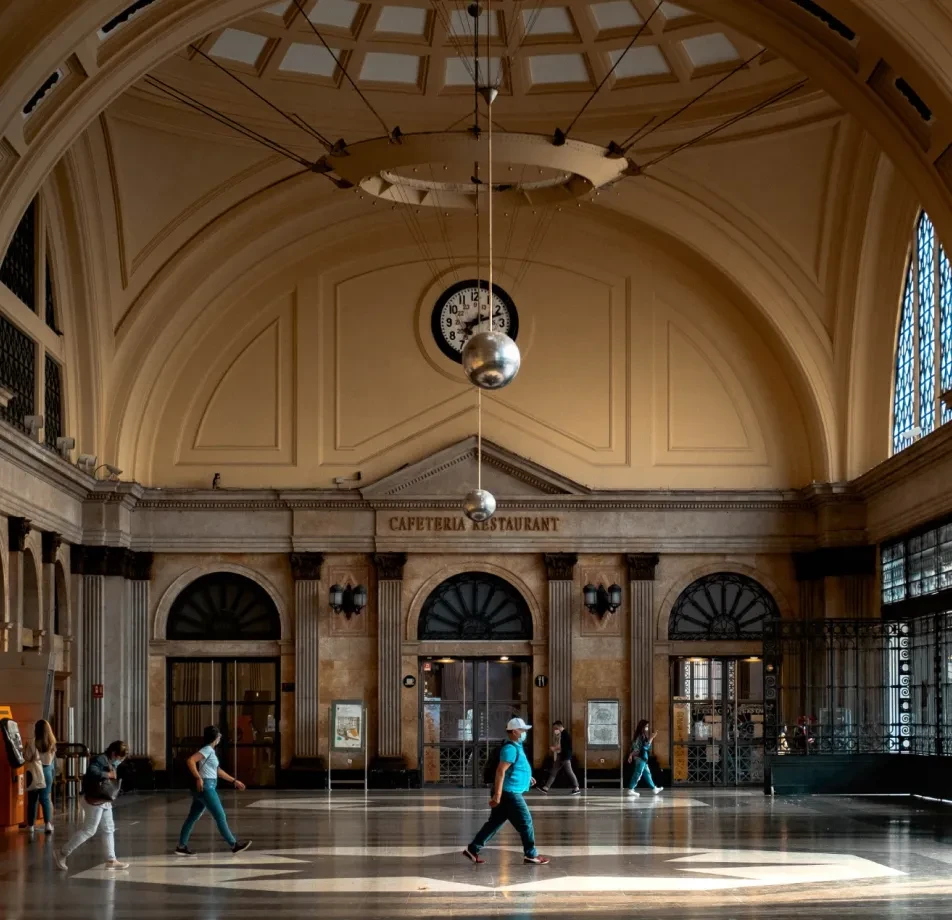
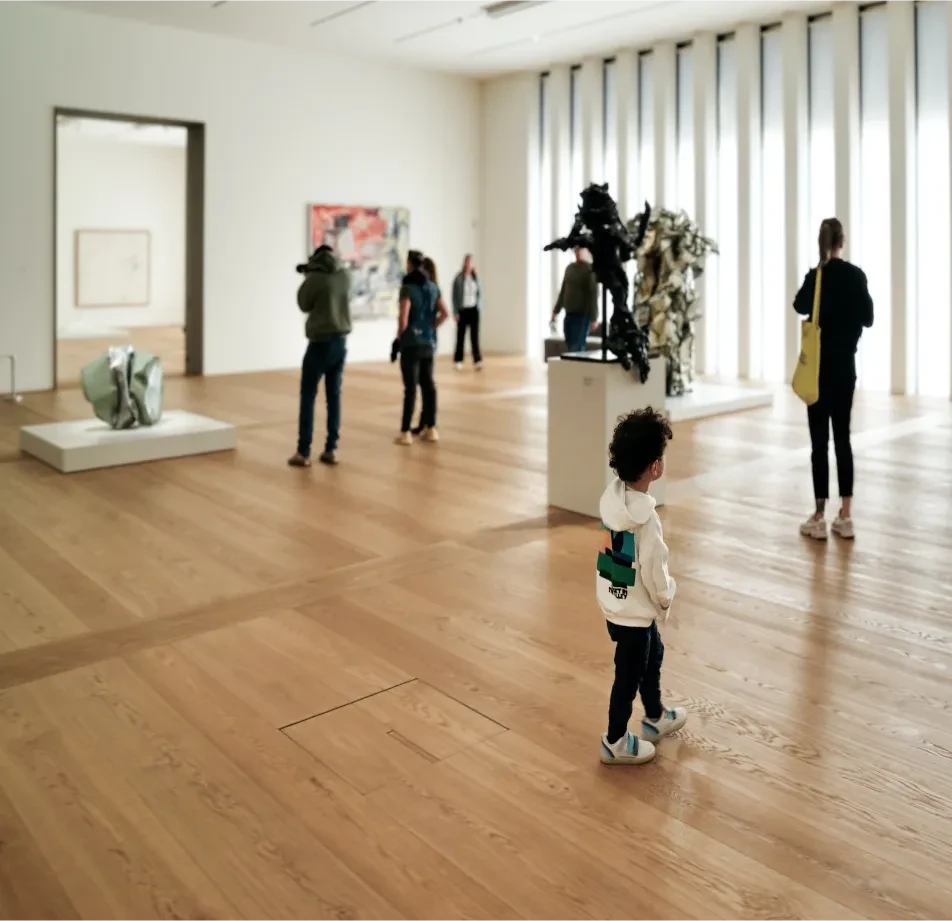
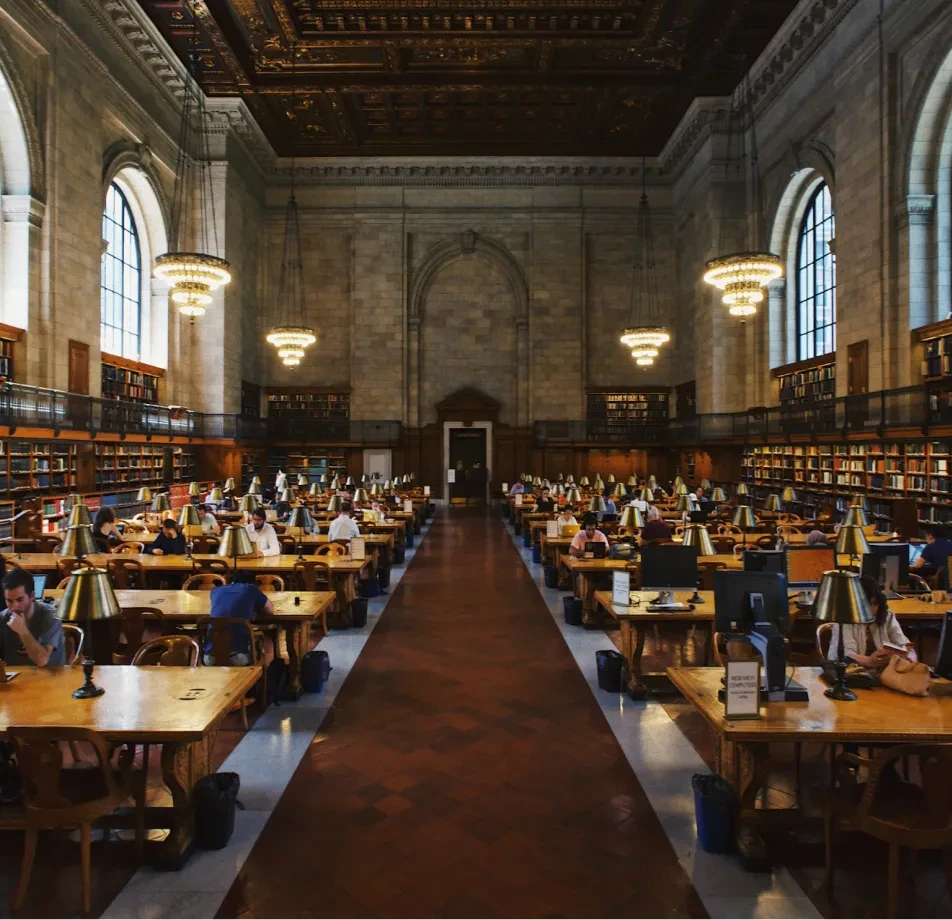
Sources
- Large public spaces: airports, train stations, shopping malls, and parks.
- Events: concerts, sports events, and conventions.
- Different camera brands and resolutions for diverse image qualities.
- Daytime and nighttime captures.
- Collaboration with security firms for accessing pre-recorded surveillance footage (ensuring privacy and permissions).
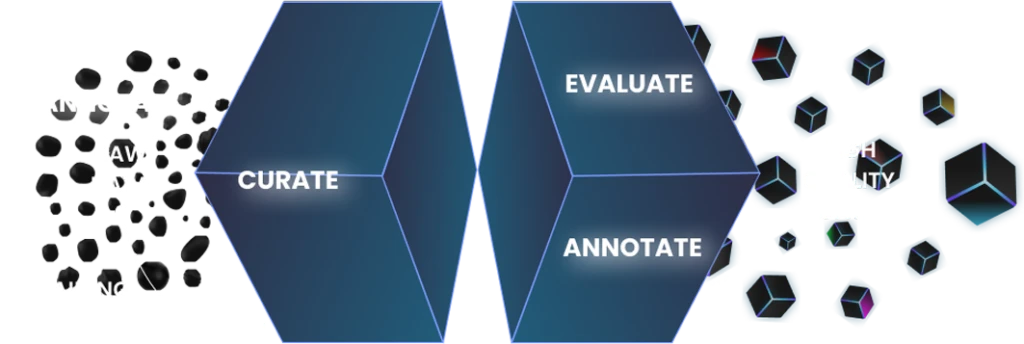
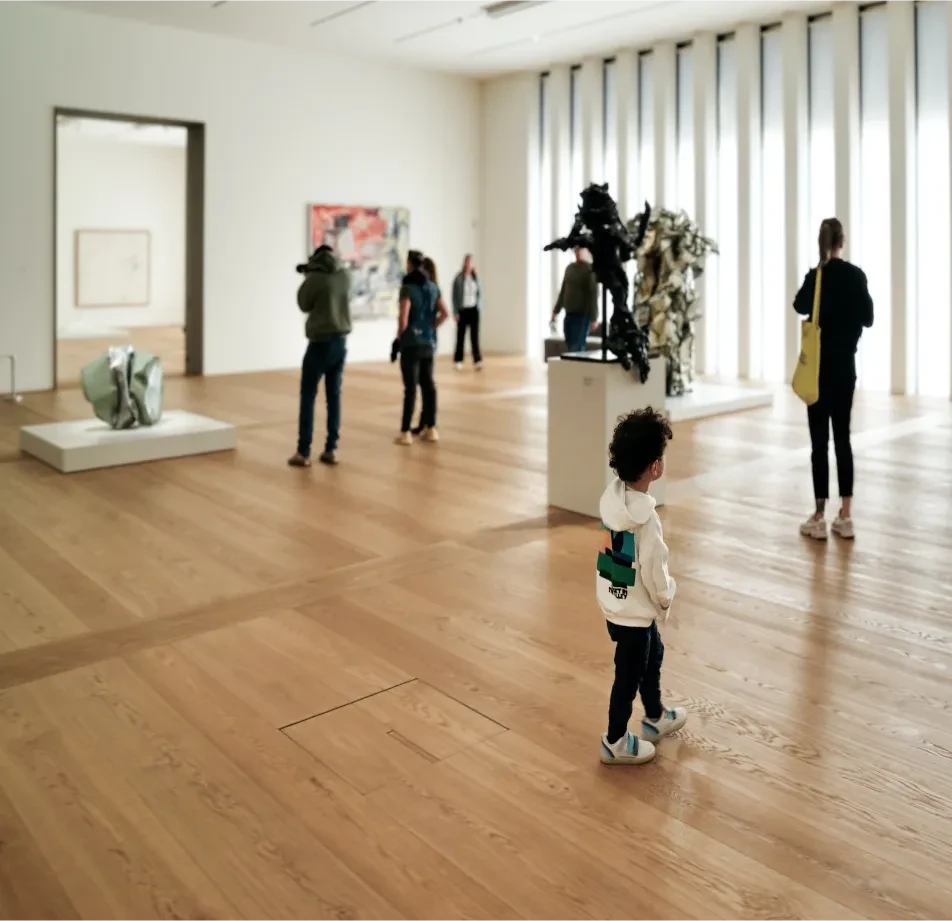
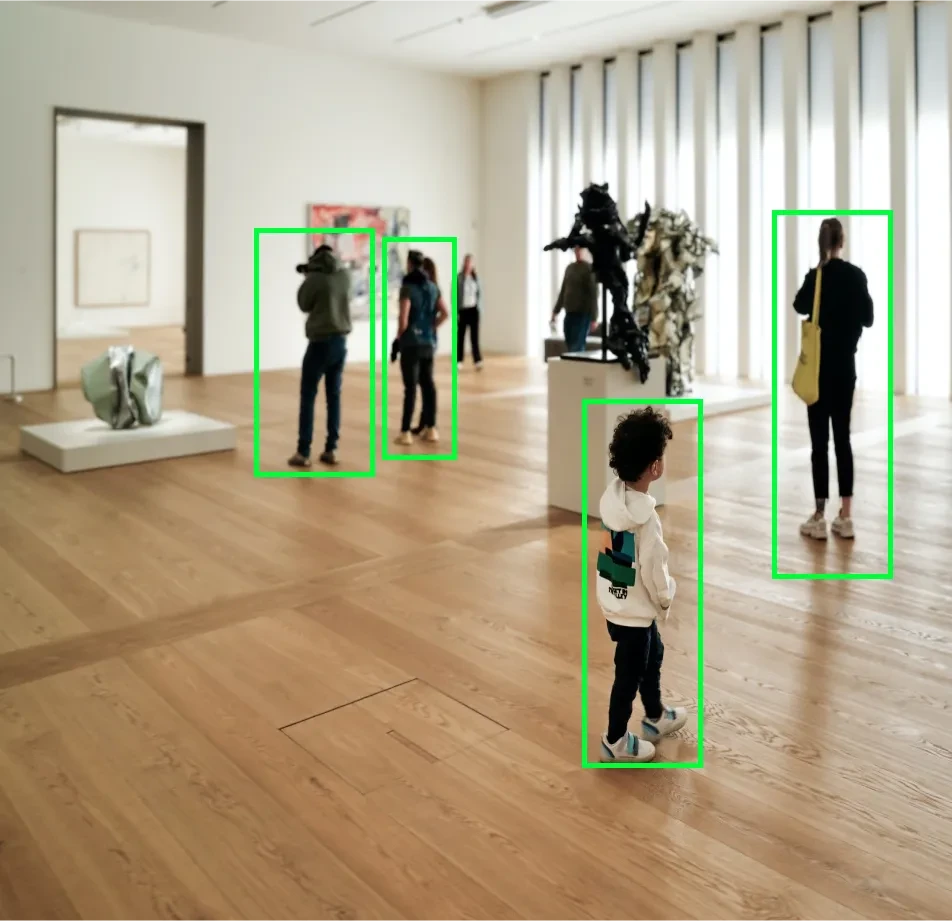
Data Collection Metrics
- Total Data Points: 1,200,000 frames
- Public Spaces: 400,000 frames
- Events: 400,000 frames
- Nighttime Captures: 200,000 frames
- Diverse Camera Sources: 200,000 frames
Annotation Process
Stages
- Refining Raw Data: Initially, we sift through frames, removing those with unclear visibility or obstructions, thereby enhancing overall quality.
- Identifying Persons: Subsequently, we deploy tagging and tracking systems to identify individuals across frames and camera feeds.
- Linking Across Cameras: Moreover, we establish mechanisms to connect sightings of the same individual from different angles across various cameras.
- Annotating Accessories: Additionally, we annotate distinct identifiers like bags, hats, or specific clothing items to aid in re-identification processes.
- Ensuring Quality: Finally, we conduct meticulous quality inspections to ensure precise person tagging and linking.
Annotation Metrics
- Individual Persons Tagged: 2,000,000 instances (some individuals appear in multiple frames)
- Cross-camera Annotations: 500,000 links made
- Accessory Annotations: 800,000 instances
- Annotations Reviewed: 240,000 (20% of total for quality assurance)
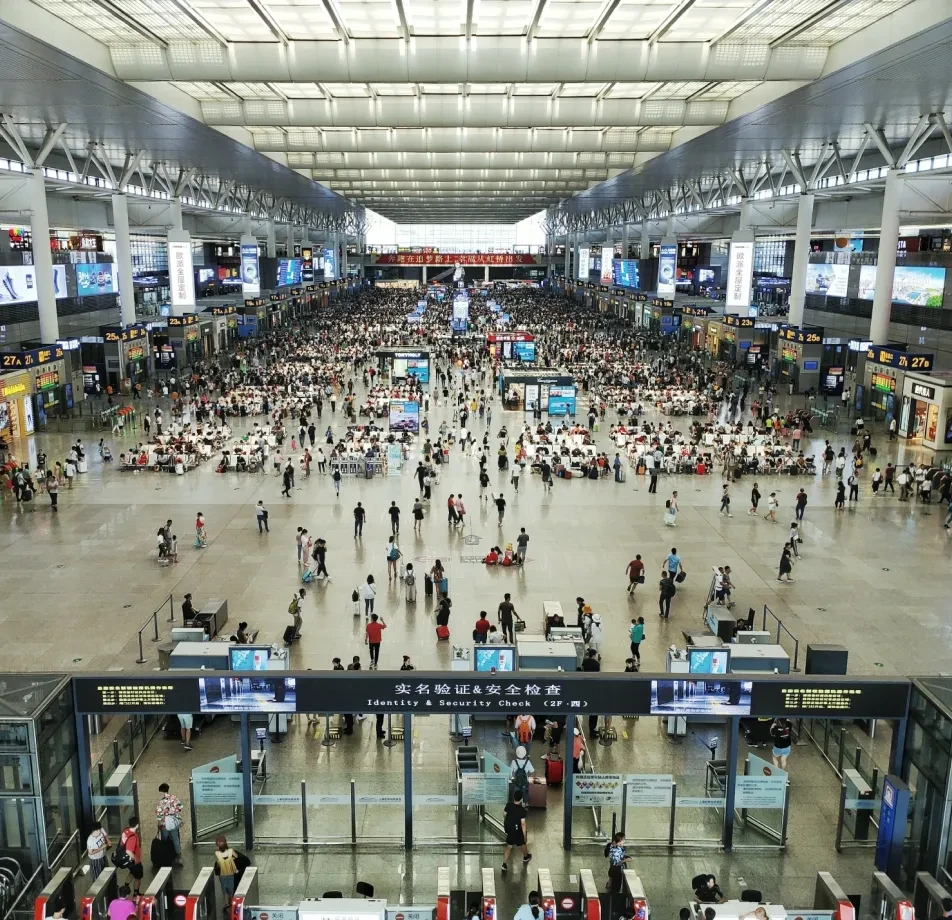
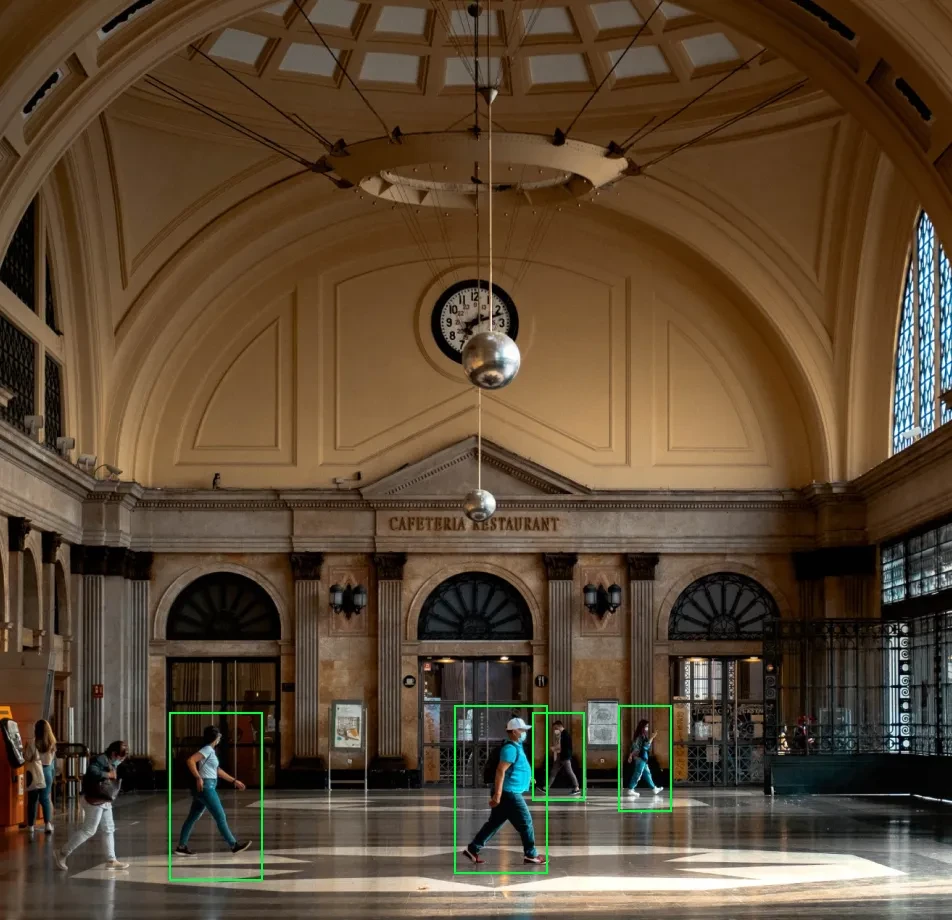
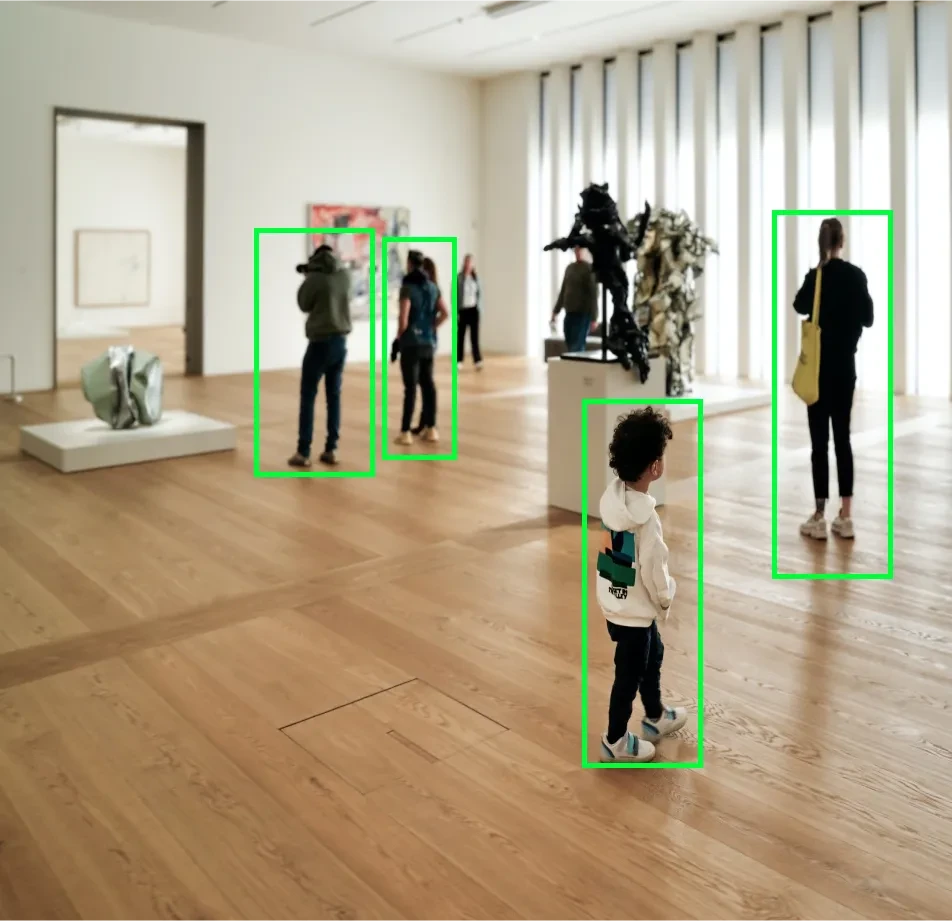
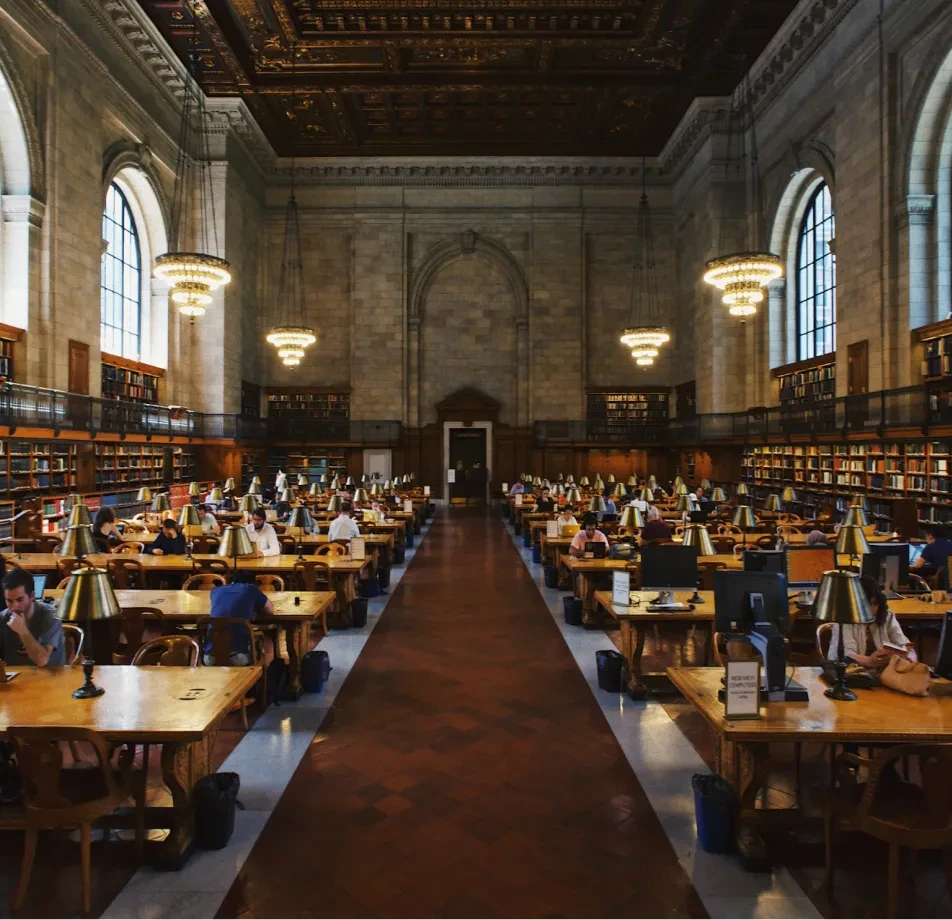
Quality Assurance
Stages
Expert Consultation: Moreover, surveillance and security specialists conducted thorough assessments to ensure the annotations’ real-world relevance and accuracy.
Automated Consistency Verifications: Additionally, software cross-referenced linked annotations to detect any inconsistencies.
Inter-annotator Reliability Checks: Additionally, collaborative reviews involving multiple annotators were undertaken on shared datasets to enhance annotation consistency.
QA Metrics
- Annotations Verified by Experts: 240,000 (20% of total annotations)
- Identified and Corrected Mismatches: 24,000 (2% of total annotations)
Conclusion
The Large-scene Multi-camera Person Re-identification Dataset marks a significant leap forward in surveillance technology. Consequently, it offers tailored solutions for advanced surveillance, crowd control, and seamless integration into smart city systems. With its expansive coverage and meticulously detailed annotations, this dataset has the potential to revolutionize person re-identification. Therefore, it ushers in a new era of accuracy and efficiency in security and urban management solutions.

Quality Data Creation

Guaranteed TAT

ISO 9001:2015, ISO/IEC 27001:2013 Certified

HIPAA Compliance

GDPR Compliance

Compliance and Security
Let's Discuss your Data collection Requirement With Us
To get a detailed estimation of requirements please reach us.