Lane Line Segmentation Dataset
Home » Case Study » Computer Vision » Lane Line Segmentation Dataset
Project Overview:
Objective
We aimed to create a comprehensive dataset essential for developing advanced driver assistance systems (ADAS), autonomous vehicles, and traffic management technologies. This dataset will improve the accuracy and dependability of lane line segmentation. By focusing on these improvements, we can significantly enhance the precision and reliability of ADAS, autonomous vehicles, and traffic management systems.
Scope
Our team embarked on an ambitious project to capture a variety of images from different road scenarios. We meticulously annotated these images to accurately identify and segment lane lines.
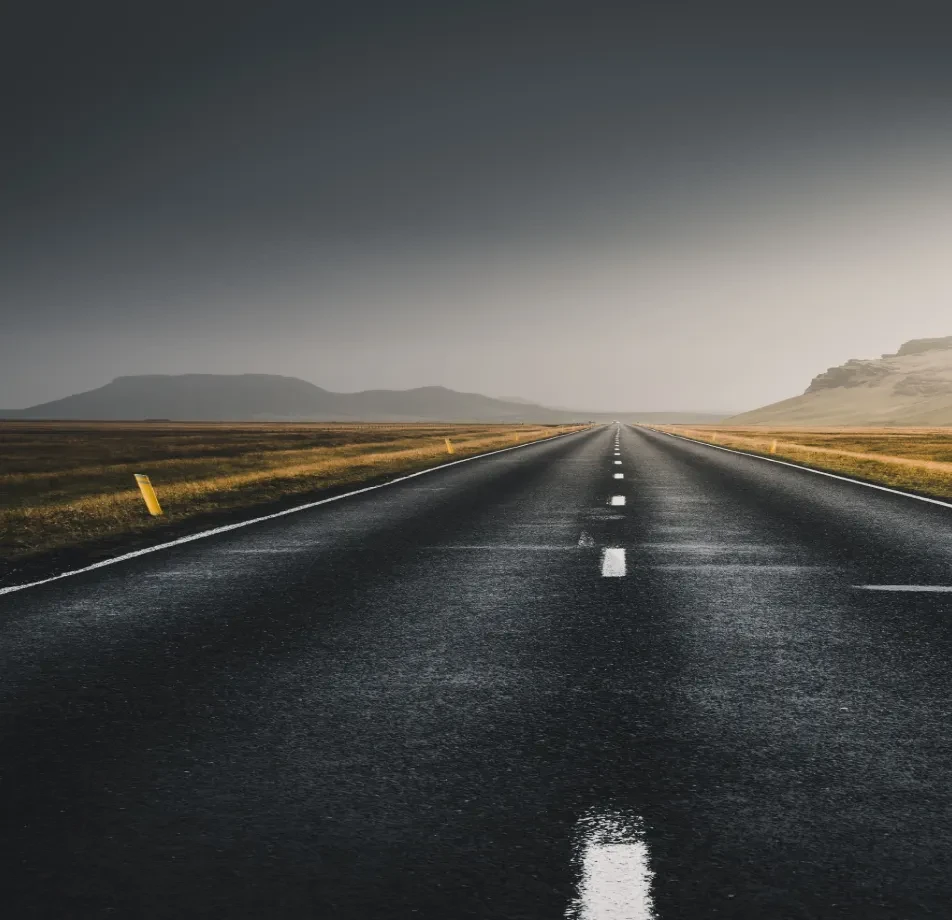
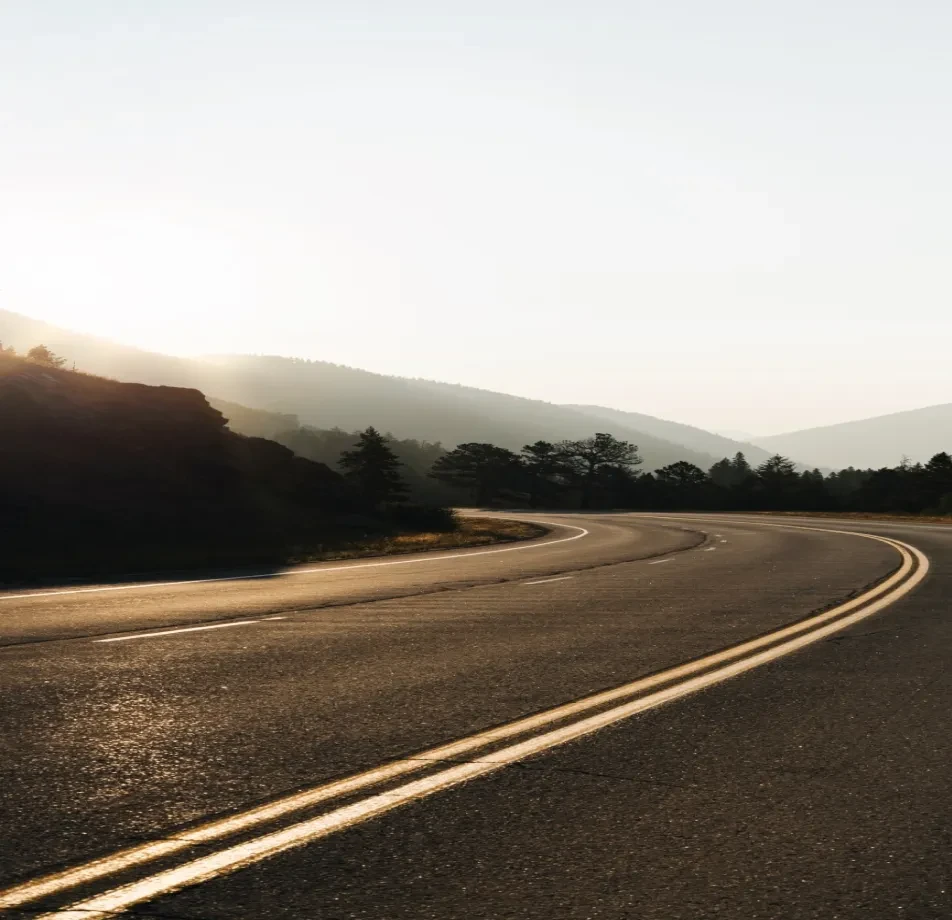
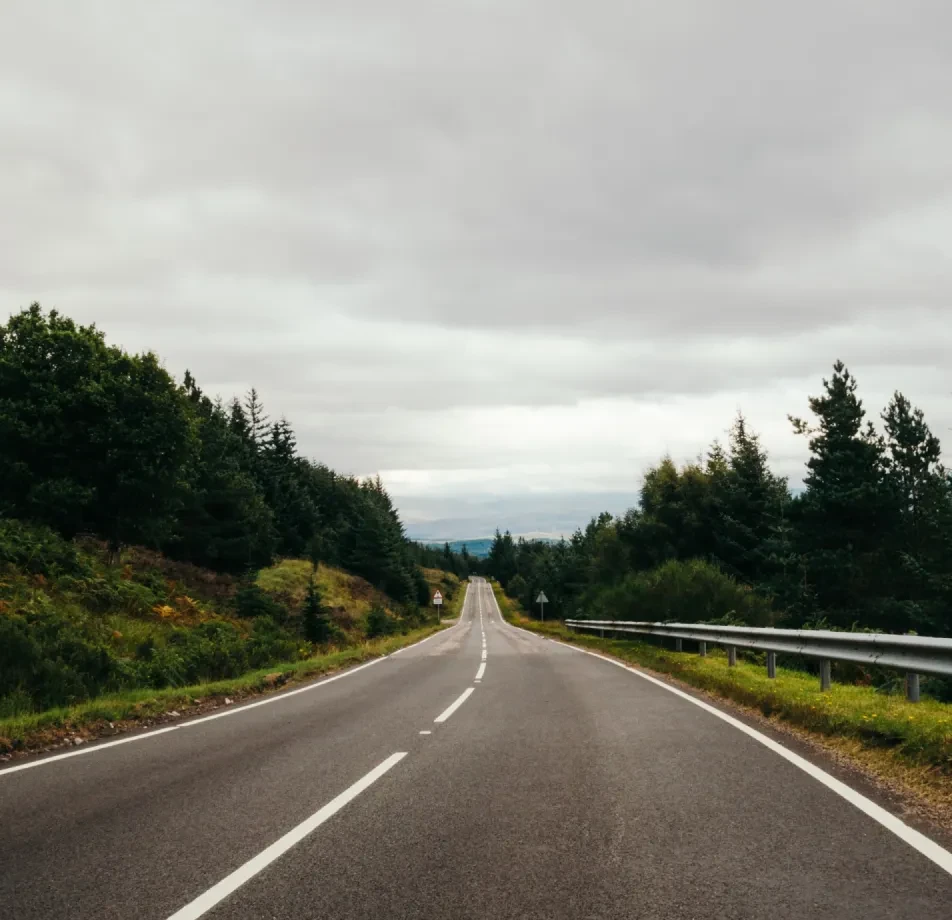
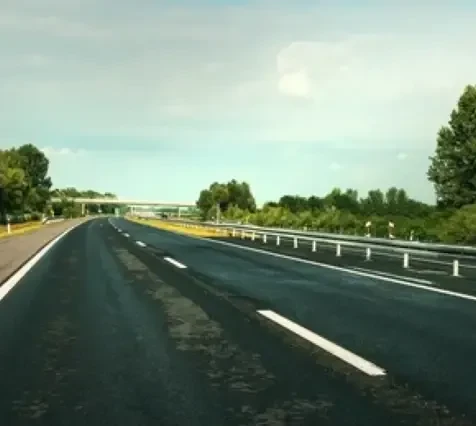
Sources
We have meticulously collected and curated bustling city streets from various cities. Furthermore, we ensured diversity in our selection. In addition, each street was chosen for its unique vibrancy.
We have thoughtfully gathered and professionally curated quaint residential and suburban roads.
We have meticulously collected and successfully curated expansive high-speed highways and motorways.
Charming rural pathways and country roads have been successfully collected and organized.
Improving the conditions during nighttime can be challenging, especially when dealing with diverse street lighting. These lighting setups have been carefully collected and curated to address various needs.
A range of weather scenarios, from clear skies to snowy conditions, has been carefully gathered and organized to provide a comprehensive representation.
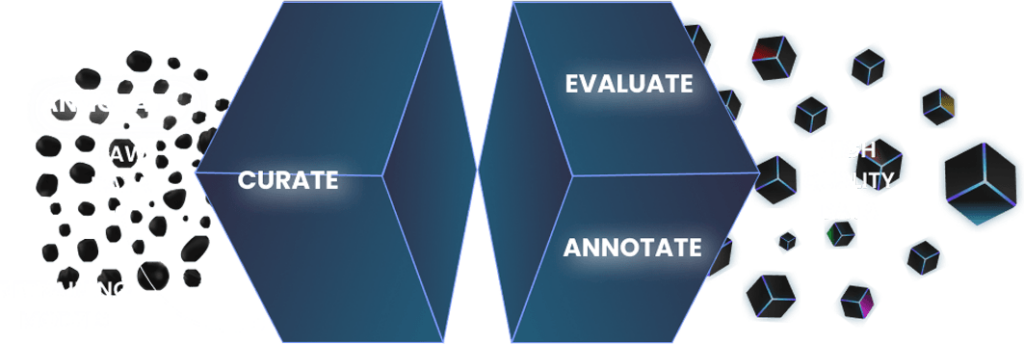
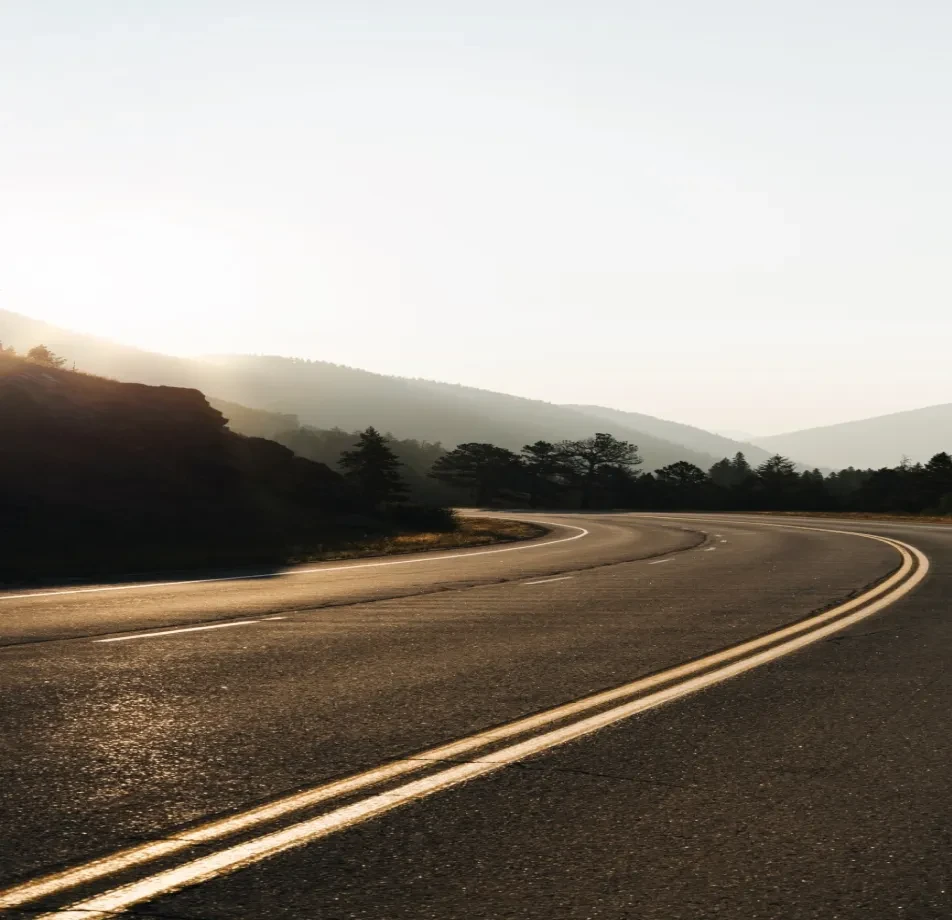
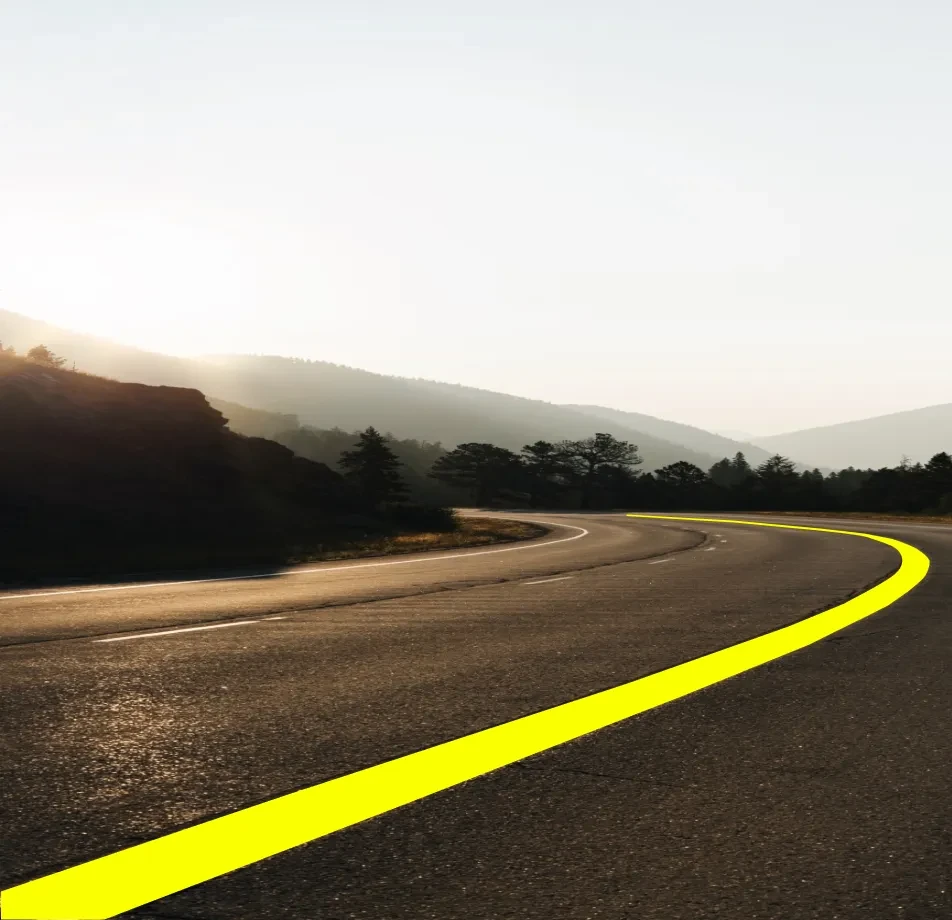
Data Collection Metrics
- Total Data Points: 500,000 images
- City Streets: 180,000
- Residential Roads: 120,000
- Highways/Motorways: 150,000
- Rural Roads: 40,000
- Nighttime Scenarios: 10,000
Annotation Process
Stages
- Raw Data Refinement: We meticulously filtered out any images that did not meet our high standards. Consequently, the quality of our dataset was significantly enhanced.
- Basic Lane Line Segmentation: Firstly, we concentrated on accurately marking the primary lanes, ensuring clear and precise segmentation.
- Advanced Lane Line Segmentation: Subsequently, we differentiated between various lane types, including special lanes for bicycles and buses. This step was crucial for detailed lane identification.
- Adjacent Feature Annotation: In addition, we marked related road markers and signs, providing comprehensive annotation of all adjacent features.
- Annotation Review: Finally, our team conducted a thorough review to ensure consistency and accuracy across all annotations.
Annotation Metrics
- Total Annotations: 2,500,000 (5 segments per image on average)
- Basic Lane Line Segmentation: 500,000
- Detailed Lane Line Segmentation: 1,500,000
- Adjacent Feature Annotations: 500,000
- Annotations Reviewed: 250,000
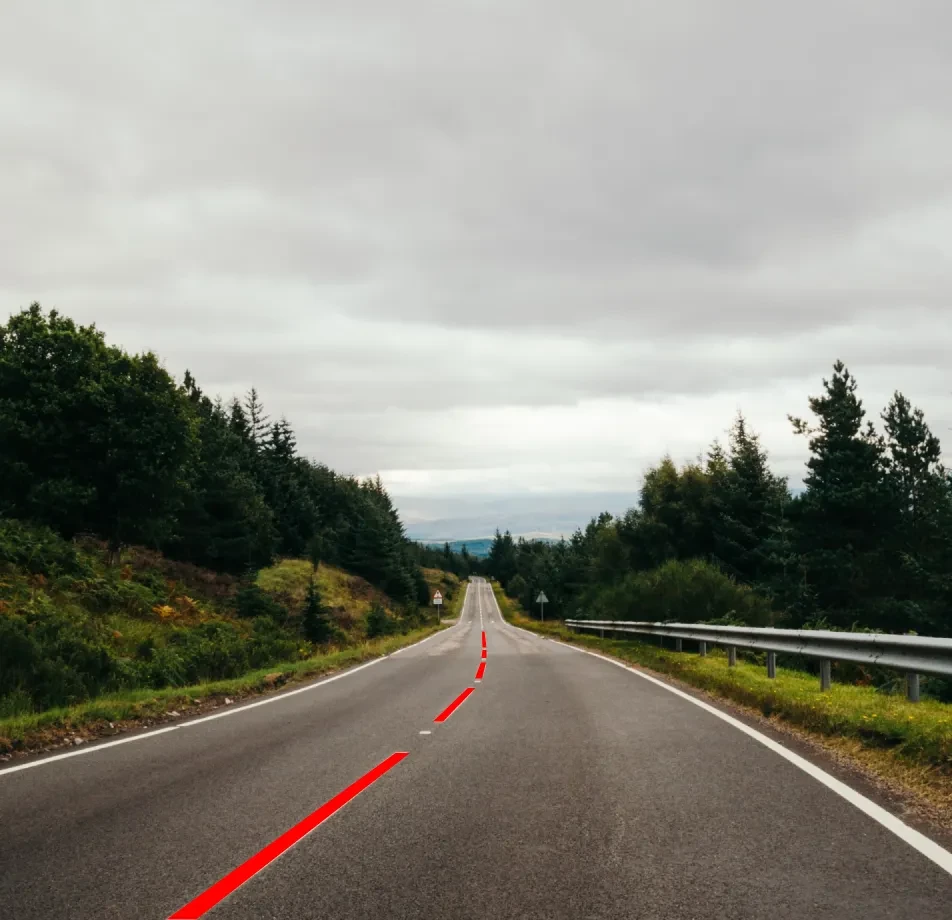
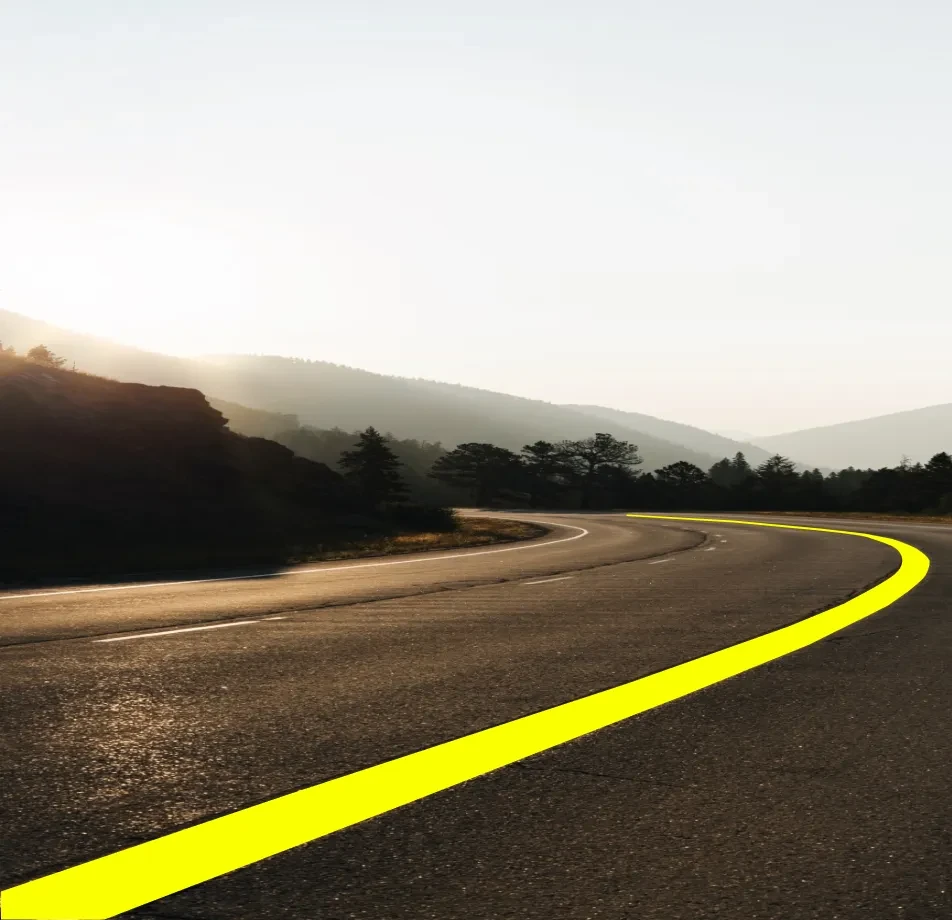
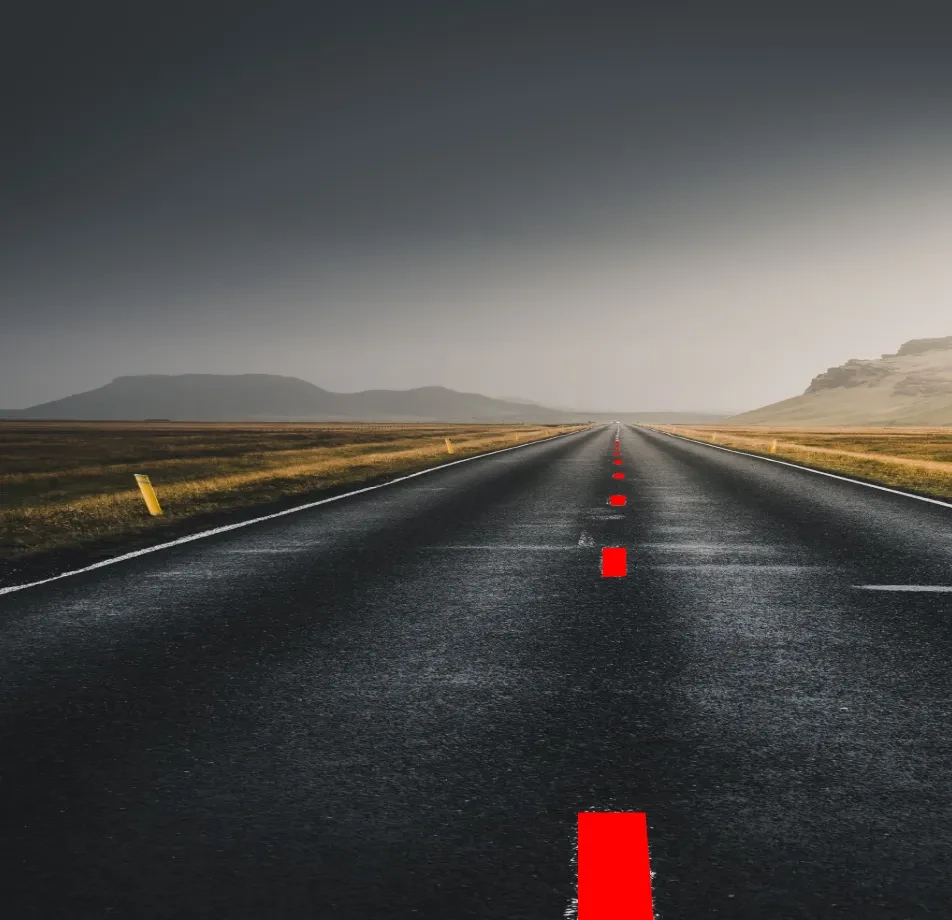
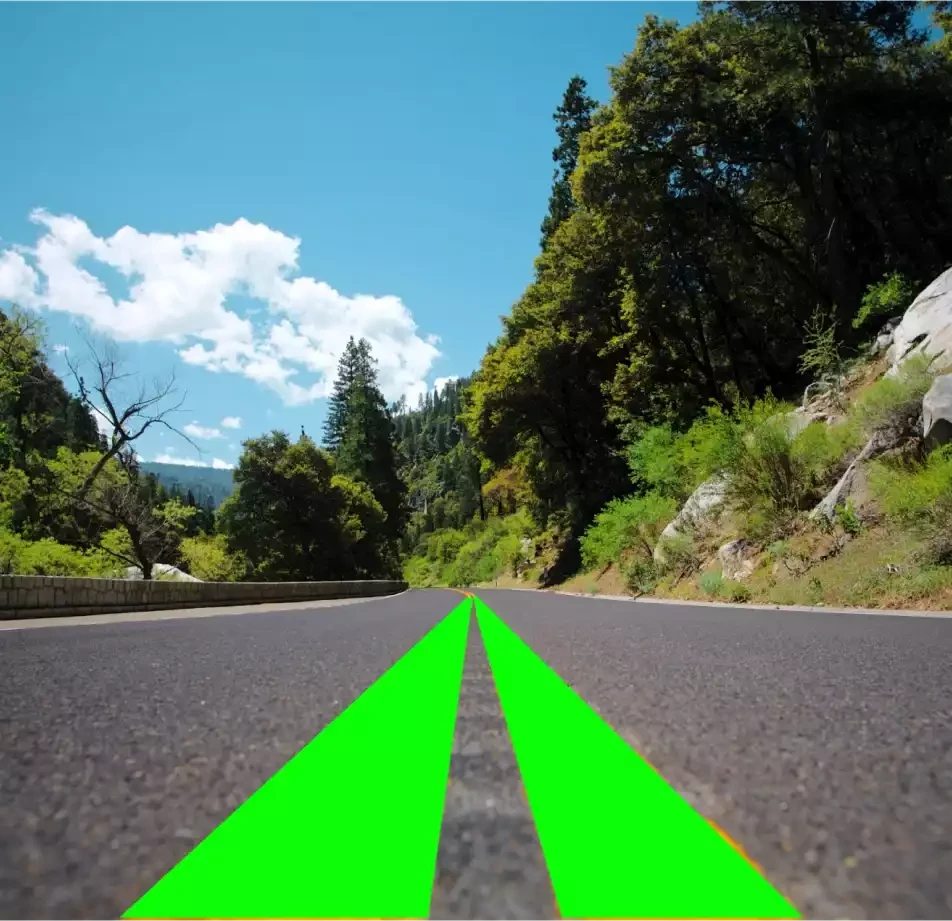
Quality Assurance
Stages
Expert Review: Specialized reviewers with expertise in traffic markings meticulously inspected a subset of the dataset.
Automated Consistency Checks: Moreover, algorithms were employed to highlight potential mismatches or missed segments, thereby ensuring the dataset’s accuracy.
Inter-annotator Agreement: Additionally, to ensure standardization, multiple annotators worked on overlapping sets, cross-checking their annotations.
QA Metrics
- Annotations Reviewed by Experts: 250,000 (10% of total annotations)
- Inconsistencies Identified and Rectified: 25,000 (1% of total annotations)
Conclusion
The Lane Line Segmentation Dataset is a valuable resource that significantly enhances the reliability of autonomous and semi-autonomous driving systems. Furthermore, it captures the complexity of real-world driving conditions, making it highly relevant for practical applications. Additionally, the dataset includes detailed notes that help improve vehicle safety and navigation.

Quality Data Creation

Guaranteed TAT

ISO 9001:2015, ISO/IEC 27001:2013 Certified

HIPAA Compliance

GDPR Compliance

Compliance and Security
Let's Discuss your Data collection Requirement With Us
To get a detailed estimation of requirements please reach us.