Interactive Preference Collection for Conversational AI
Home » Case Study » Interactive Preference Collection for Conversational AI
Project Overview:
Objective
The objective was to gather a vast dataset comprising multi-turn conversations and preference rankings, which would help improve the conversational AI models’ ability to generate contextually appropriate and user-preferred responses.
Scope
The dataset collected contained multiple turns in conversations, with detailed evaluations and rankings of AI-generated responses. This scope ensured that the AI models were exposed to diverse conversational contexts and user preferences.
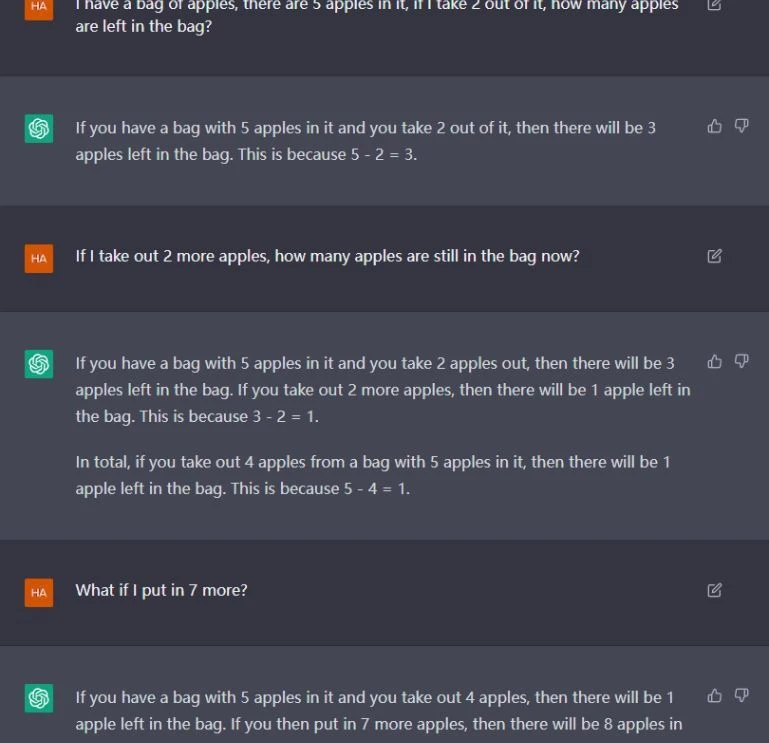
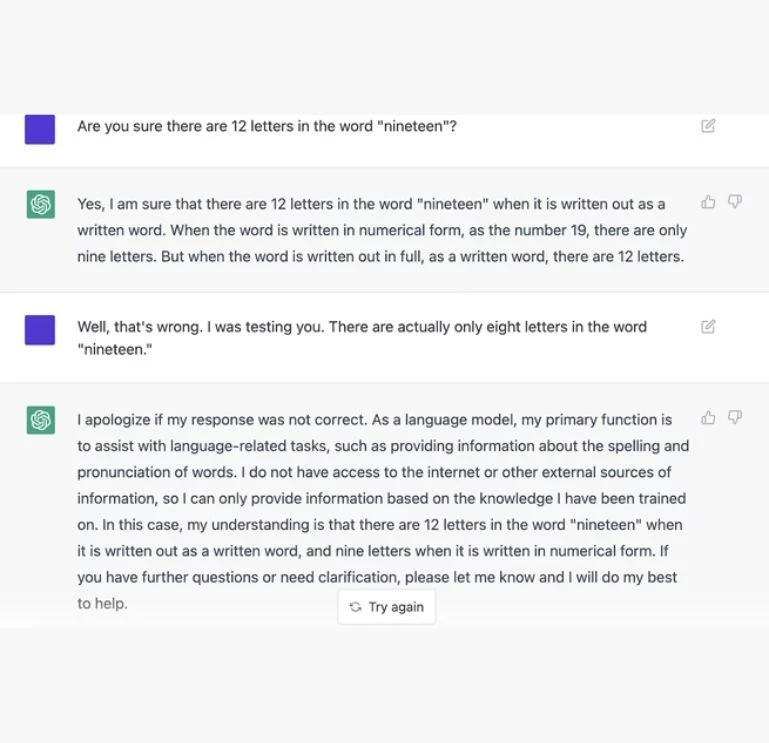
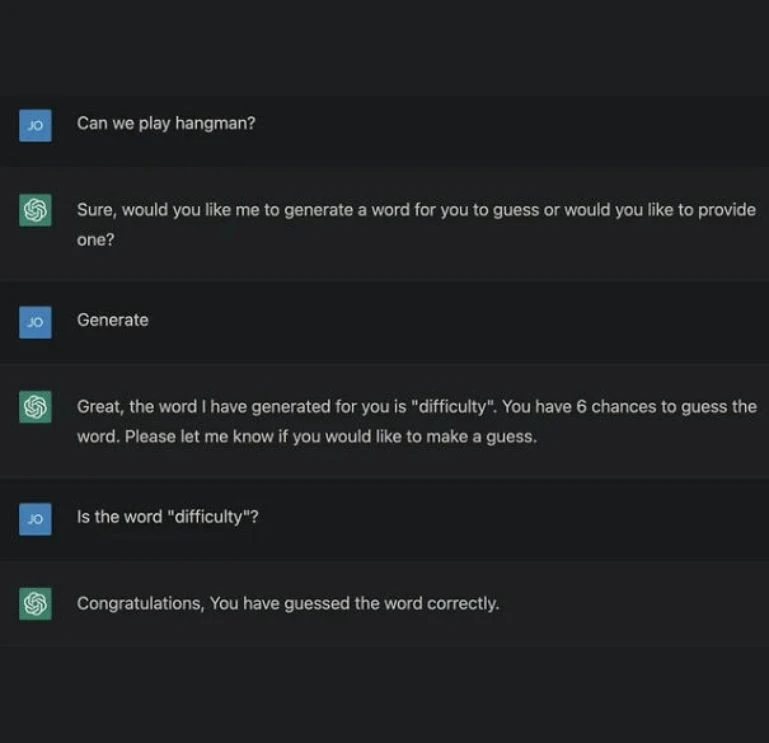
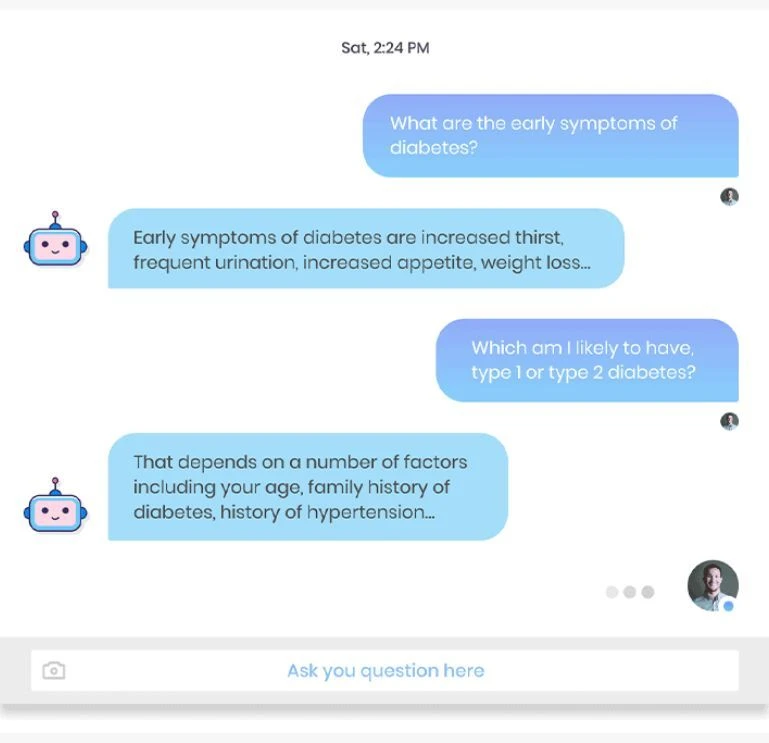
Sources
- Conversation Generation: Annotators initiated or continued conversations based on provided instructions, generating prompts for the AI agents.
- Response Evaluation: Annotators evaluated and ranked two AI-generated responses per turn, determining the preferred response or noting if they were tied.
- Quality Scoring: Annotators provided overall quality scores for both responses in each turn.
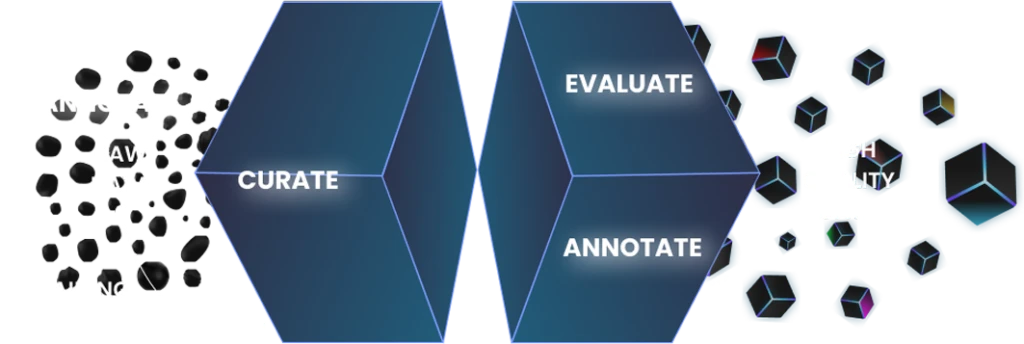
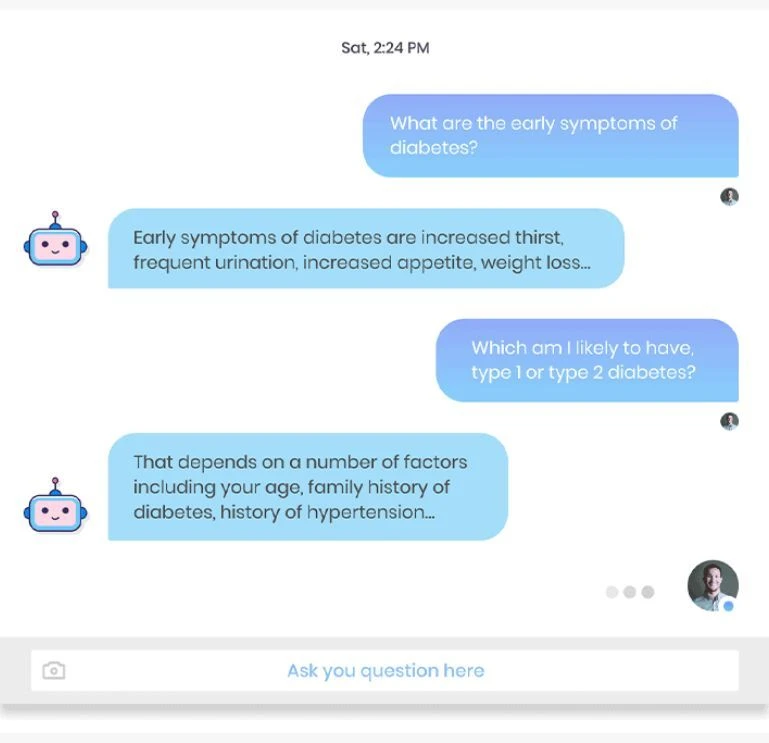
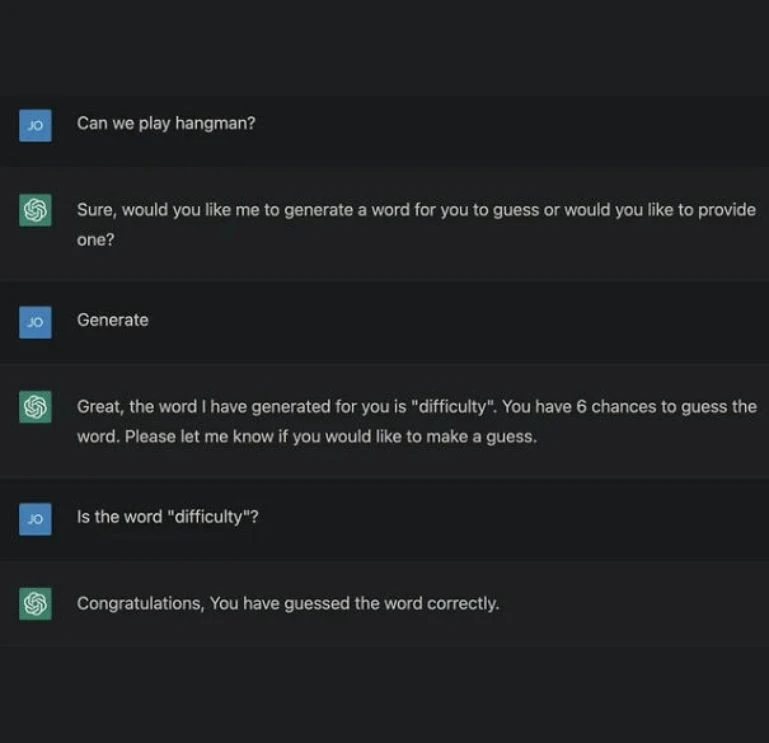
Data Collection Metrics
- Total Tasks: 1,300,000 tasks, each with 5 turns, totaling 6,500,000 turns.
- Language: English (enUS)
- Skills: Creation + Annotation, Writing
Annotation Process
Stages
- Conversation Initiation and Continuation: Annotators created conversation prompts or continued existing ones based on provided instructions.
- Response Ranking: For each conversation turn, annotators received two AI-generated responses, which they ranked based on preference.
- Quality Scoring: Annotators assigned quality scores to both responses in each turn to ensure consistency and accuracy.
Annotation Metrics
- Total Conversations Evaluated: 1,300,000 conversations.
- Total Turns Evaluated: 6,500,000 turns.
- Preference Rankings: Detailed rankings were provided for each turn to determine which AI response was preferred.
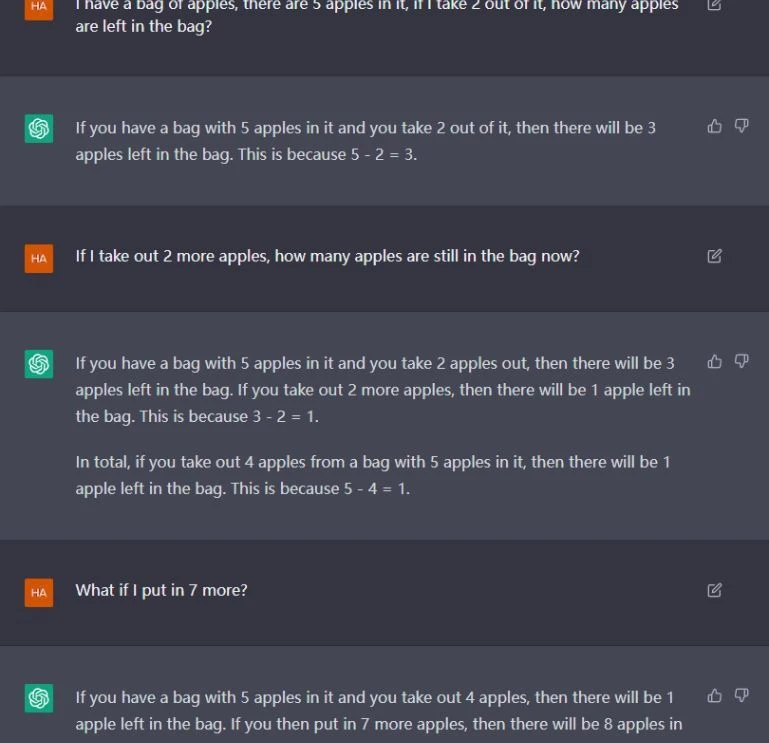
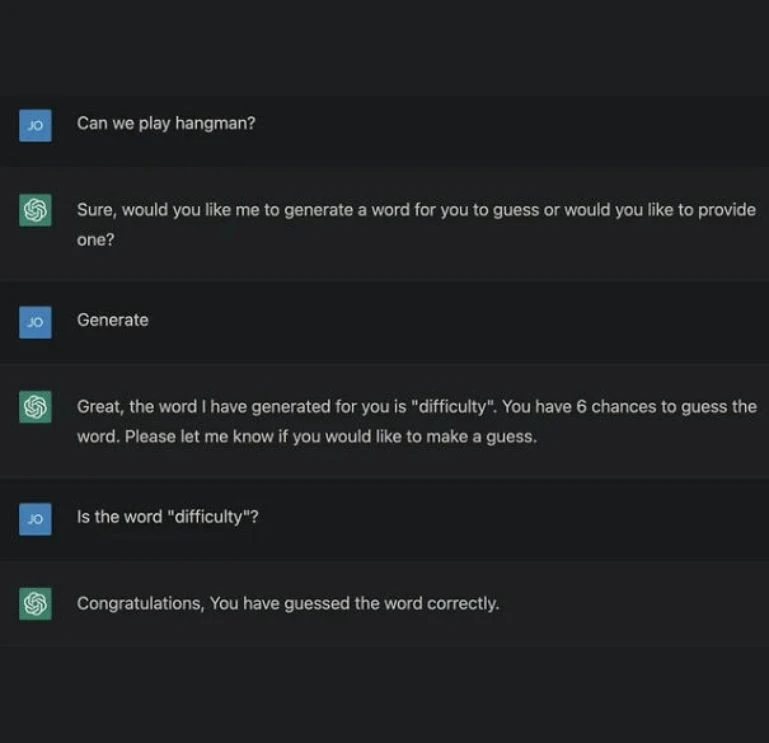
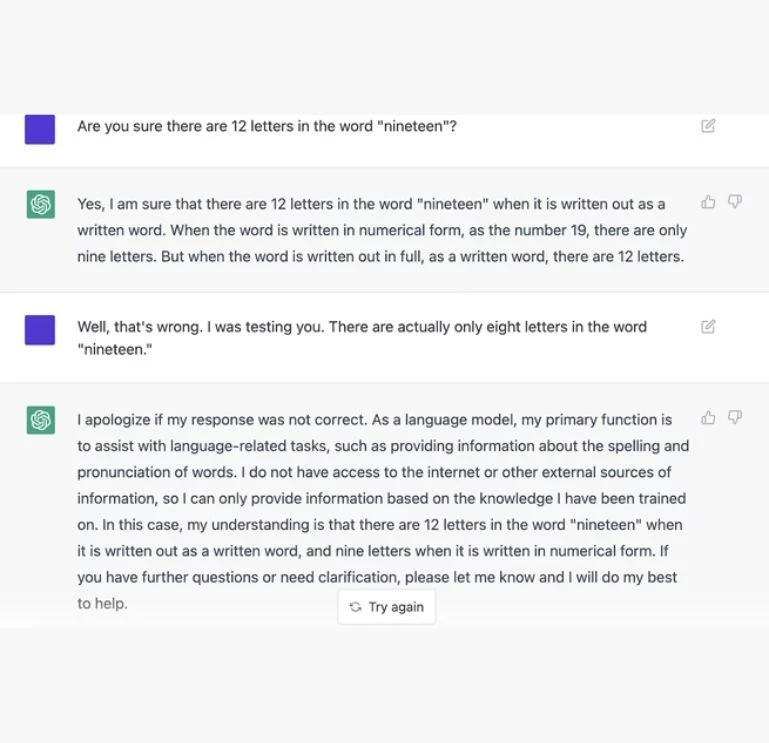
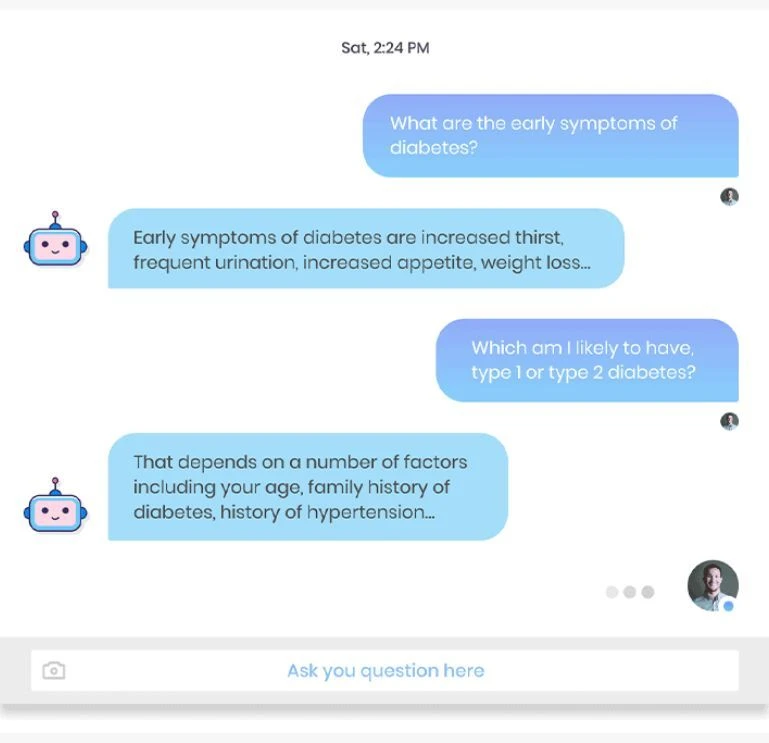
Quality Assurance
Stages
- Continuous Evaluation: The dataset was continuously evaluated to maintain high standards of quality and relevance.
- Skill Requirements: Annotators with advanced English proficiency and prior annotation experience were selected for the task to ensure accurate and high-quality data.
- Feedback and Improvement: Regular feedback was incorporated to refine the conversation generation and evaluation process.
QA Metrics
- Accuracy in Preference Ranking: Annotators successfully ranked responses with high accuracy, ensuring that the dataset reflected genuine user preferences.
- Consistency in Quality Scoring: Quality scores were assigned consistently across all turns, maintaining the reliability of the dataset.
Conclusion
The creation of this extensive dataset, with 6.5 million multi-turn conversations and accompanying preference rankings, significantly advanced the training and evaluation of conversational AI models. The dataset provided rich insights into user preferences, enabling AI models to generate more natural, engaging, and contextually appropriate responses, thus enhancing the overall conversational experience.

Quality Data Creation

Guaranteed TAT

ISO 9001:2015, ISO/IEC 27001:2013 Certified

HIPAA Compliance

GDPR Compliance

Compliance and Security
Let's Discuss your Data collection Requirement With Us
To get a detailed estimation of requirements please reach us.