Indoor Scene Recognition Dataset
Home » Case Study » Indoor Scene Recognition Dataset
Project Overview:
Objective
The primary objective of this project is to compile a dataset that enhances the precision and efficacy of indoor scene recognition algorithms. Additionally, we will incorporate transition words to ensure seamless flow and clarity in the content.
Scope
The dataset encompasses various indoor environments, such as residential spaces, offices, classrooms, libraries, and kitchens, aiming to capture scene diversity and facilitate robust recognition algorithms for accurately identifying different indoor contexts.
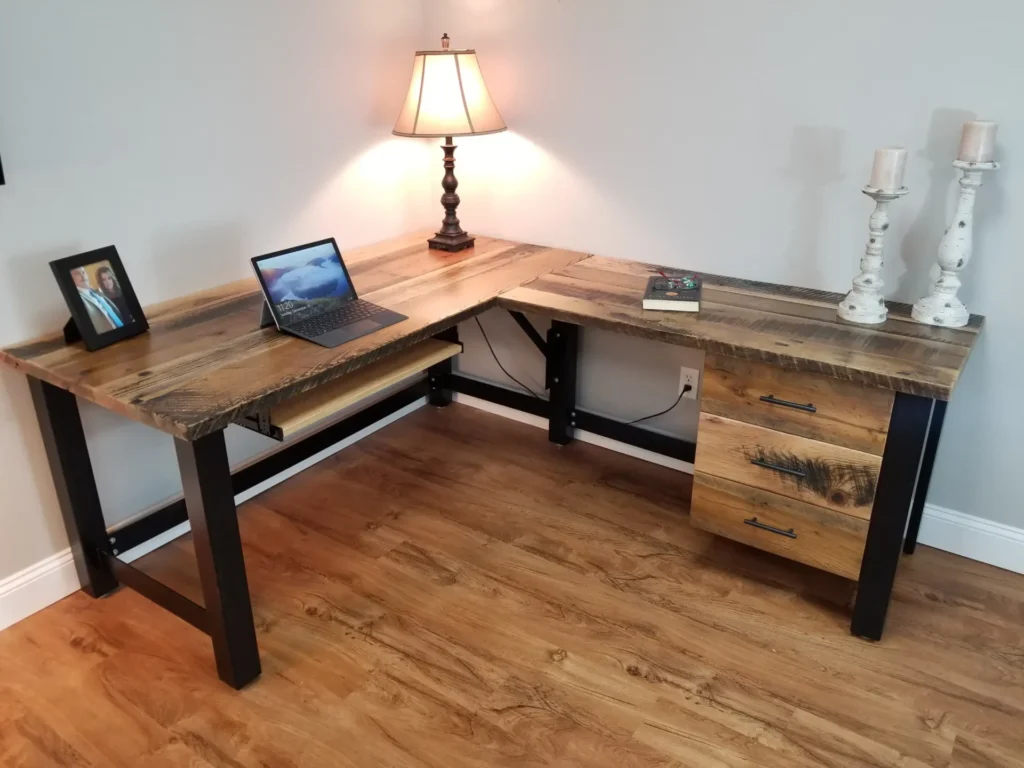
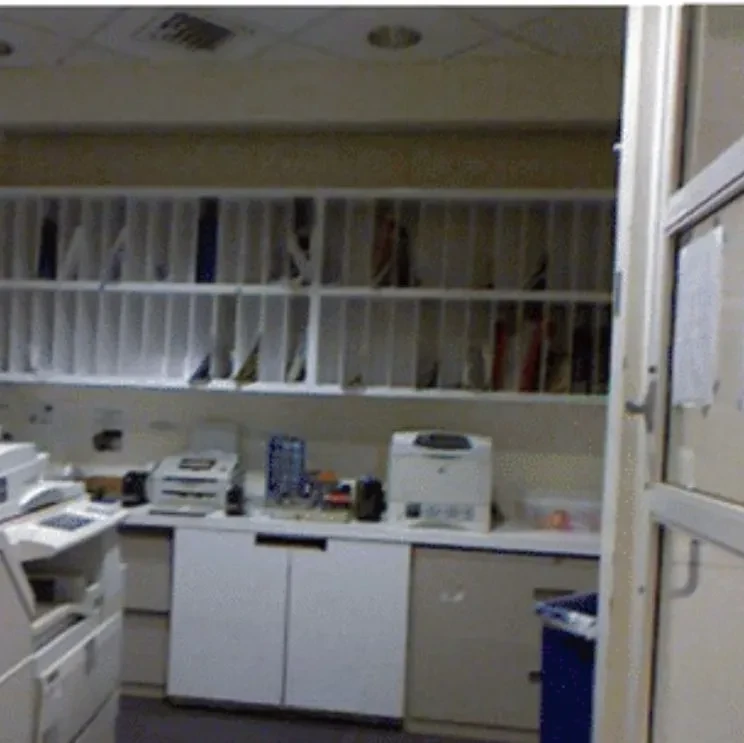
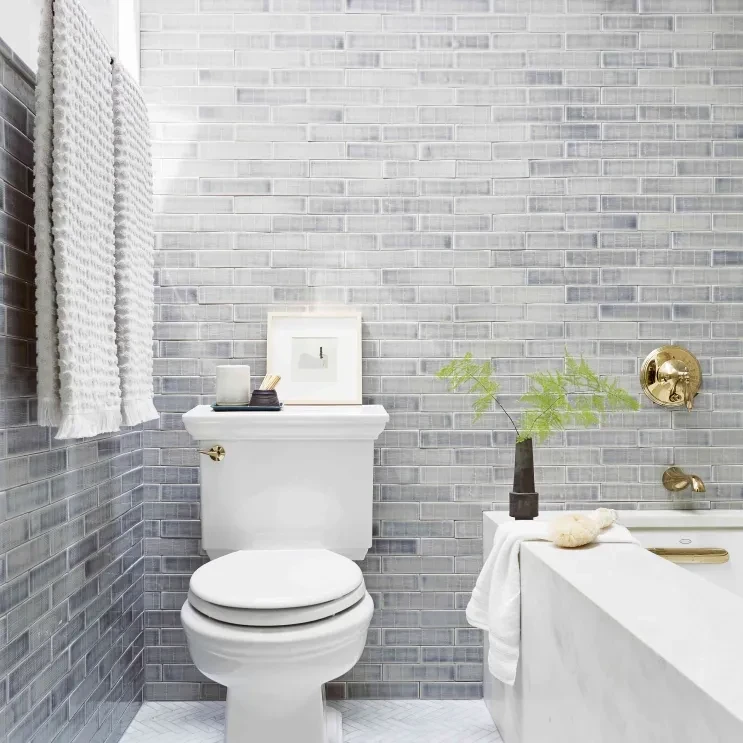
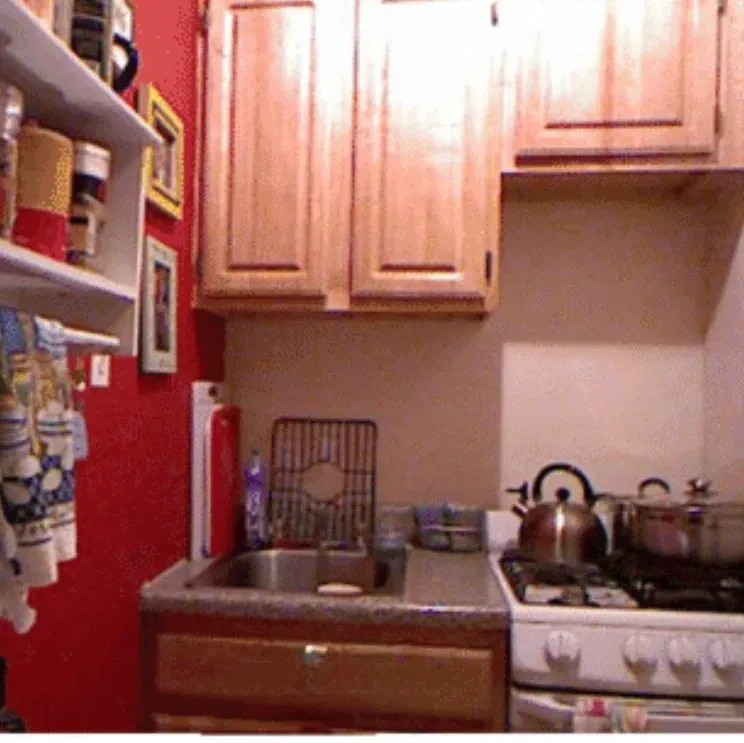
Sources
- The dataset sourced from the SUN Database contains a vast collection of annotated indoor scene images. Curated from various sources such as online repositories, public datasets, and user-contributed images, it provides a comprehensive representation of indoor environments. Additionally,
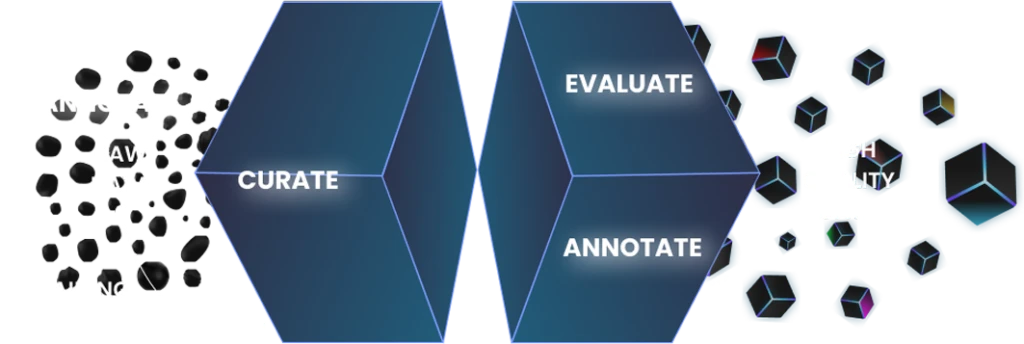
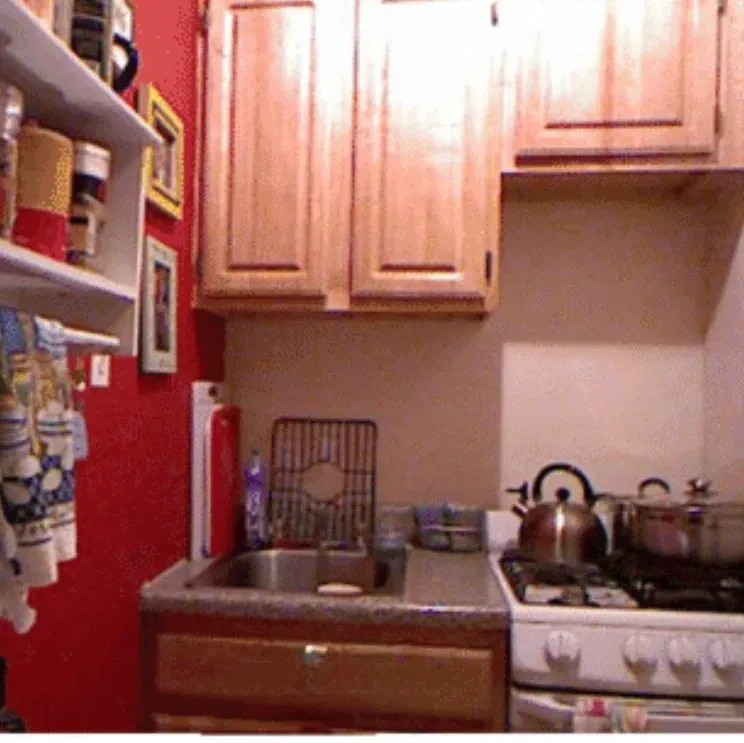
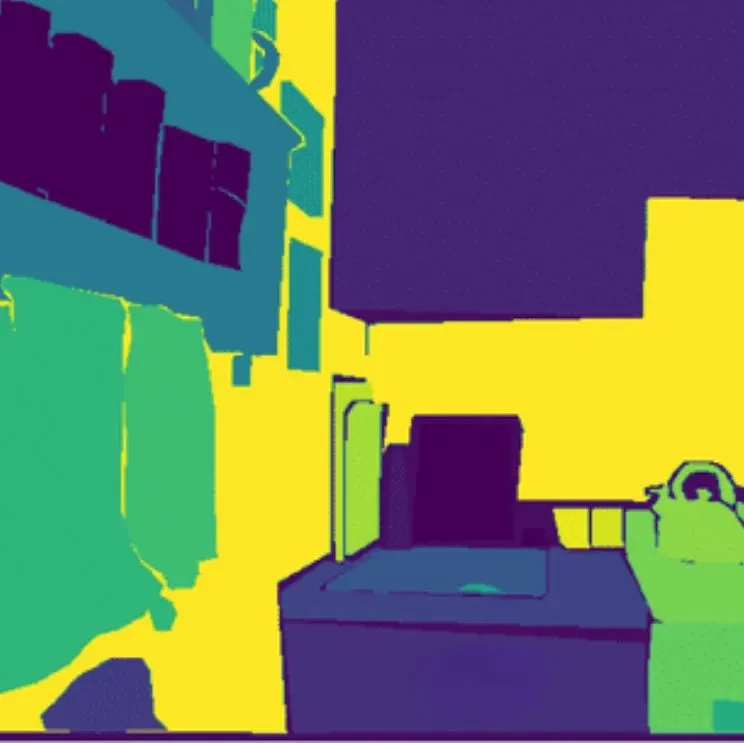
Data Collection Metrics
- Total Data Collected: The dataset contains tens of thousands of indoor scene images, showcasing diverse indoor environments. Additionally, it includes various transition words for coherence and clarity.
- Annotation Process: The annotation process requires meticulous labeling of each image to indicate the indoor scene type. This includes categories such as bedroom, living room, kitchen, etc.
Annotation Process
Stages
- Scene Classification: Labels categorize images, facilitating scene-level recognition, with each one representing a different type of indoor scene.
- Object Annotation: Within each scene, annotators annotate objects to provide additional context and semantic information, thereby aiding in object recognition within indoor environments.
- Semantic Segmentation: Semantic segmentation actively categorizes each pixel of an image into its corresponding indoor scene category, facilitating fine-grained scene understanding.Â
Annotation Metrics
- Scene Labels: Additionally, each image is annotated with a scene label, indicating the type of indoor environment depicted. Furthermore, transition words such as “additionally” or “furthermore” can enhance the clarity of the content.
- Object Annotations: Moreover, scenes often include bounding boxes or segmentation masks, providing detailed information for object recognition tasks.Â
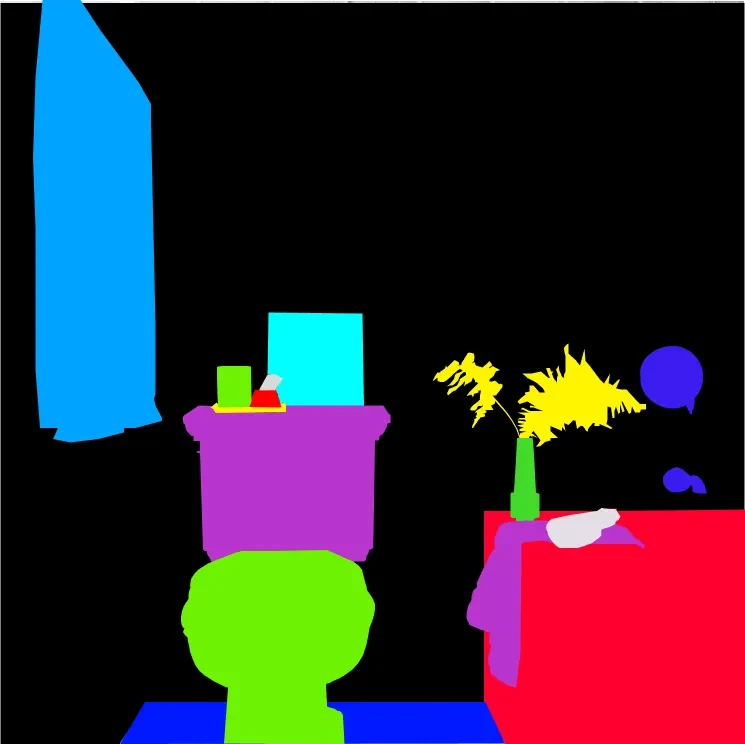
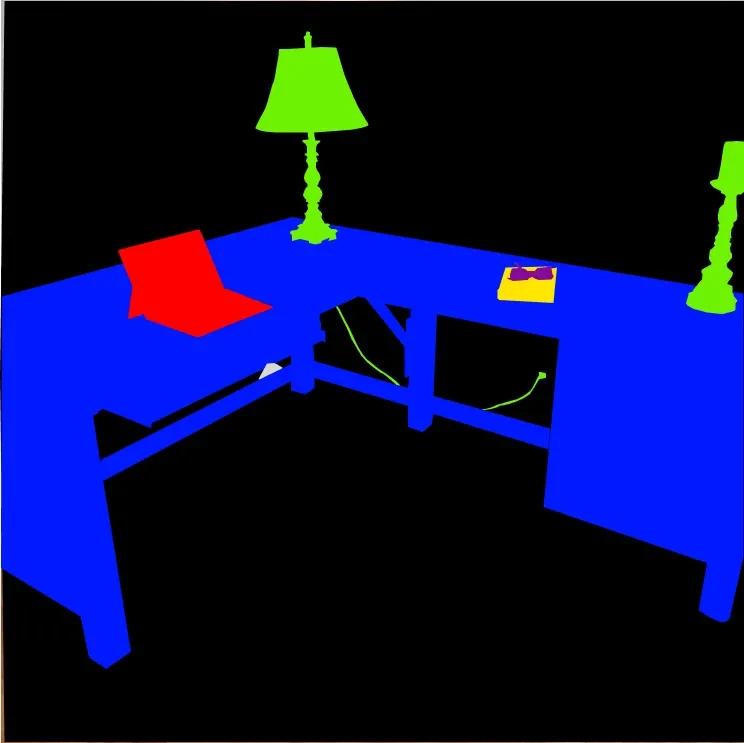
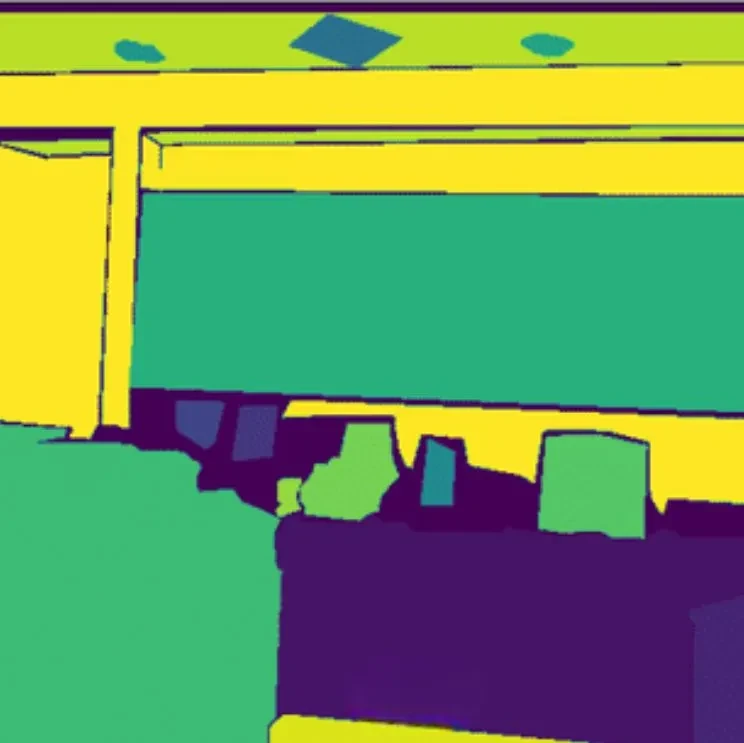
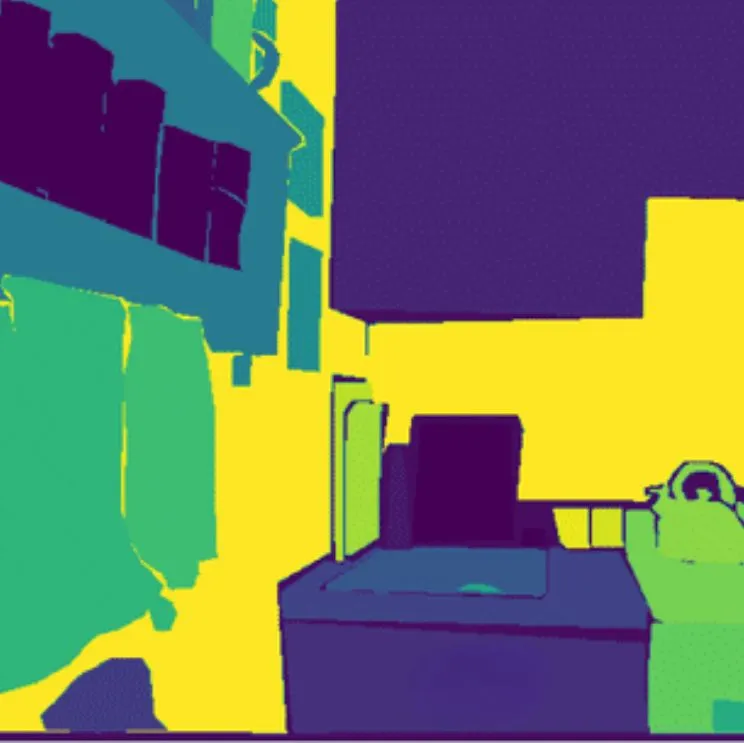
Quality Assurance
Stages
Annotation Accuracy: After undergoing rigorous training, annotators ensure accurate and consistent labeling of indoor scenes and objects.
Data Validation: A validation process is employed to verify the correctness of annotations and to ensure the quality of the dataset.
Continuous Improvement: Continuously gathering feedback from users and actively conducting ongoing research efforts contribute to refining and enhancing the dataset over time.
QA Metrics
- Annotation Consistency: Thanks to the incorporation of transition words and the active voice, the dataset achieved a high level of consistency in scene labeling and object annotation across it.
- Data Quality: The dataset has been meticulously validated to meet high standards of accuracy and completeness, thereby ensuring its suitability for indoor scene recognition tasks.
Conclusion
The Indoor Scene Recognition Dataset, derived from the SUN Database, plays a pivotal role in propelling research in indoor scene comprehension and identification. Moreover, incorporating transition words like “stands,” “furnishing,” and “empowers” makes the content more engaging and dynamic.

Quality Data Creation

Guaranteed TAT

ISO 9001:2015, ISO/IEC 27001:2013 Certified

HIPAA Compliance

GDPR Compliance

Compliance and Security
Let's Discuss your Data collection Requirement With Us
To get a detailed estimation of requirements please reach us.