Image Description for Generative AI
Home » Case Study » Image Description for Generative AI
Project Overview:
Objective
The aim was to produce a comprehensive dataset of 100,000 images paired with rich textual descriptions. This dataset was intended to advance the AI’s proficiency in generating accurate and descriptive image-to-text outputs, thus facilitating more precise and context-aware AI applications.
Scope
The dataset included a wide range of images sourced from various environments and contexts. Each image was accompanied by a detailed textual description, capturing relevant details, contextual information, and potential use cases.
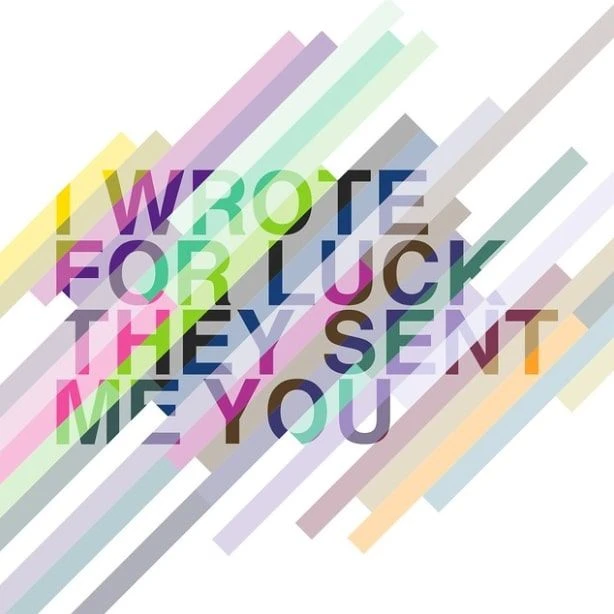
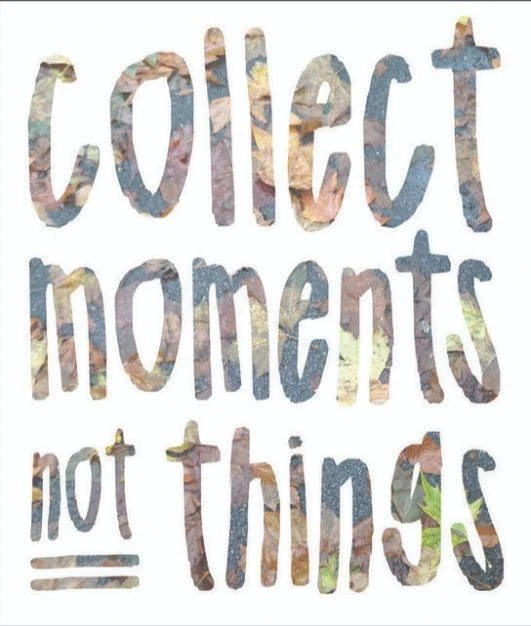
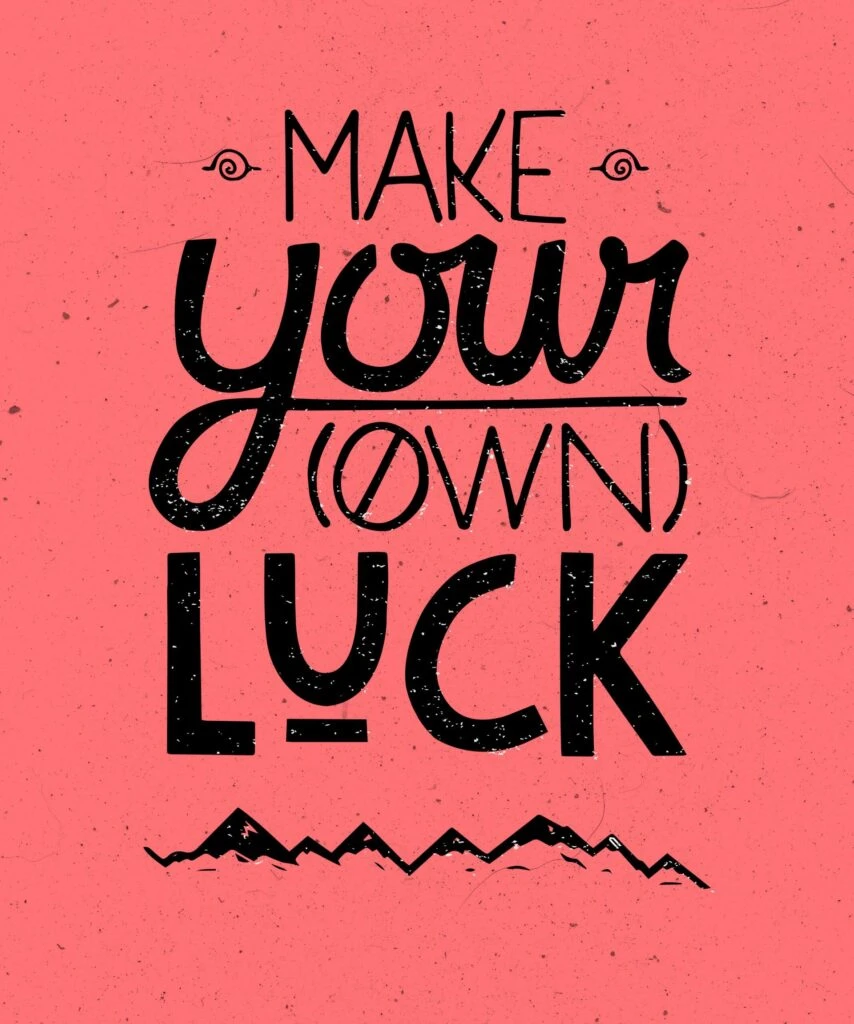
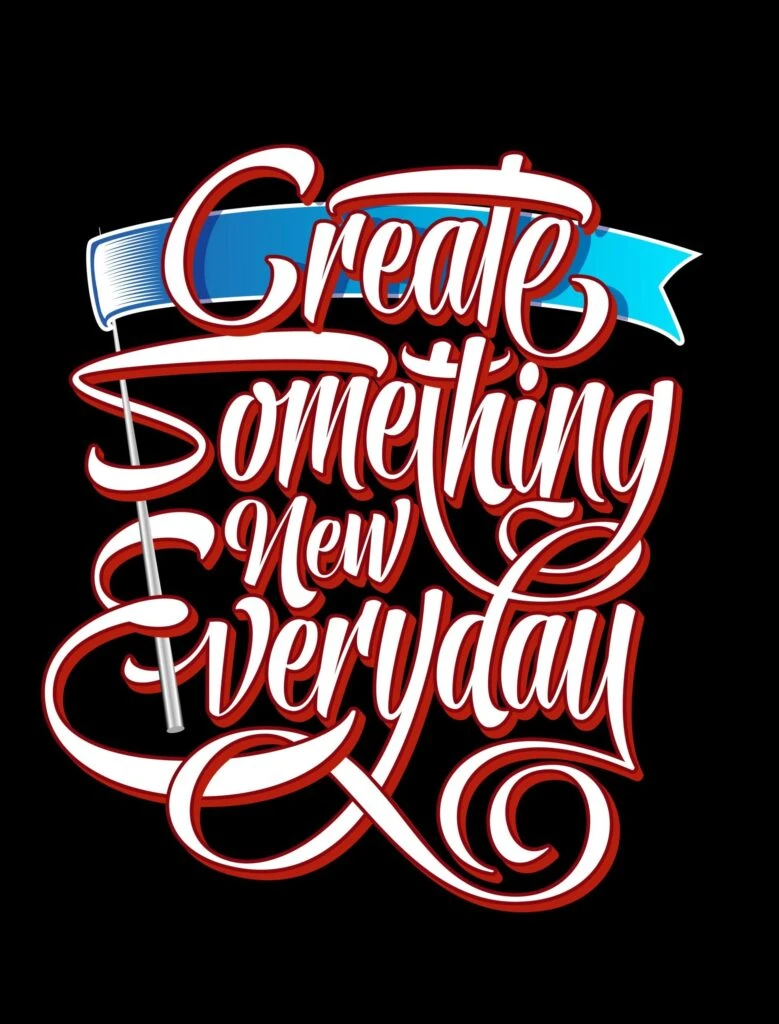
Sources
- Online Image Collection: A diverse collection of 100,000 images was curated from various online sources, ensuring a wide representation of scenarios.
- In-House Image Creation: Additional images were created in-house to fill specific gaps and enhance the diversity of the dataset.
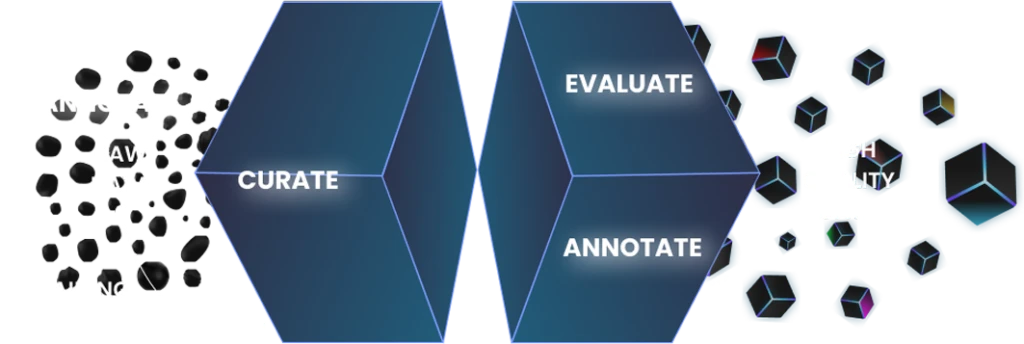
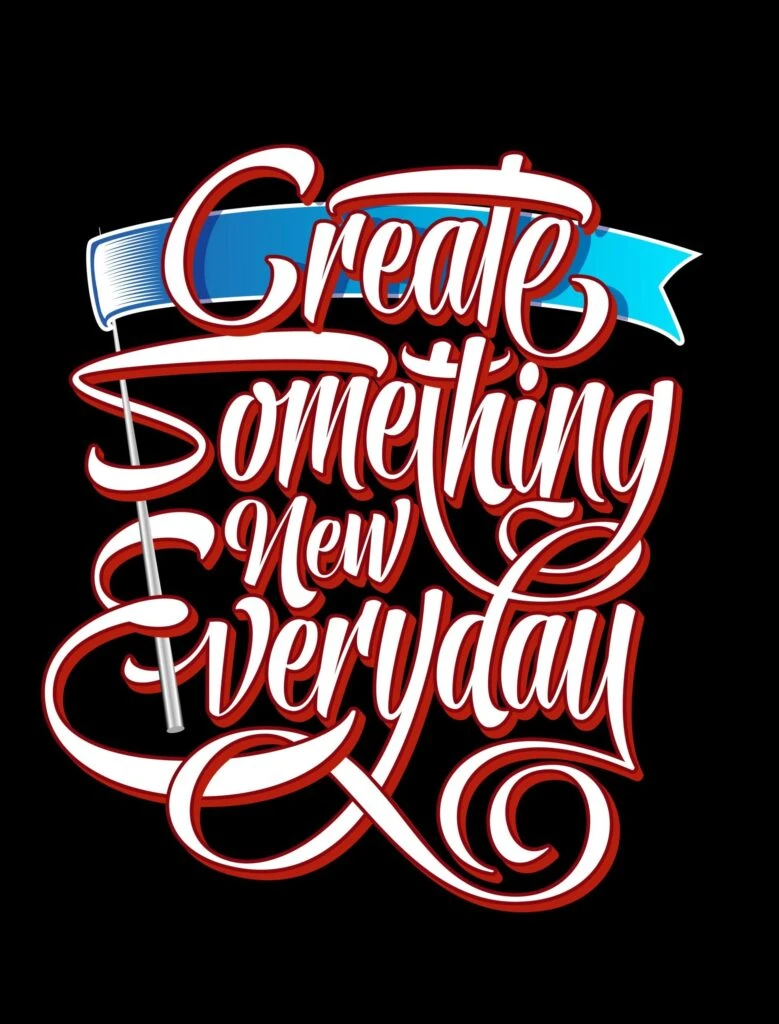
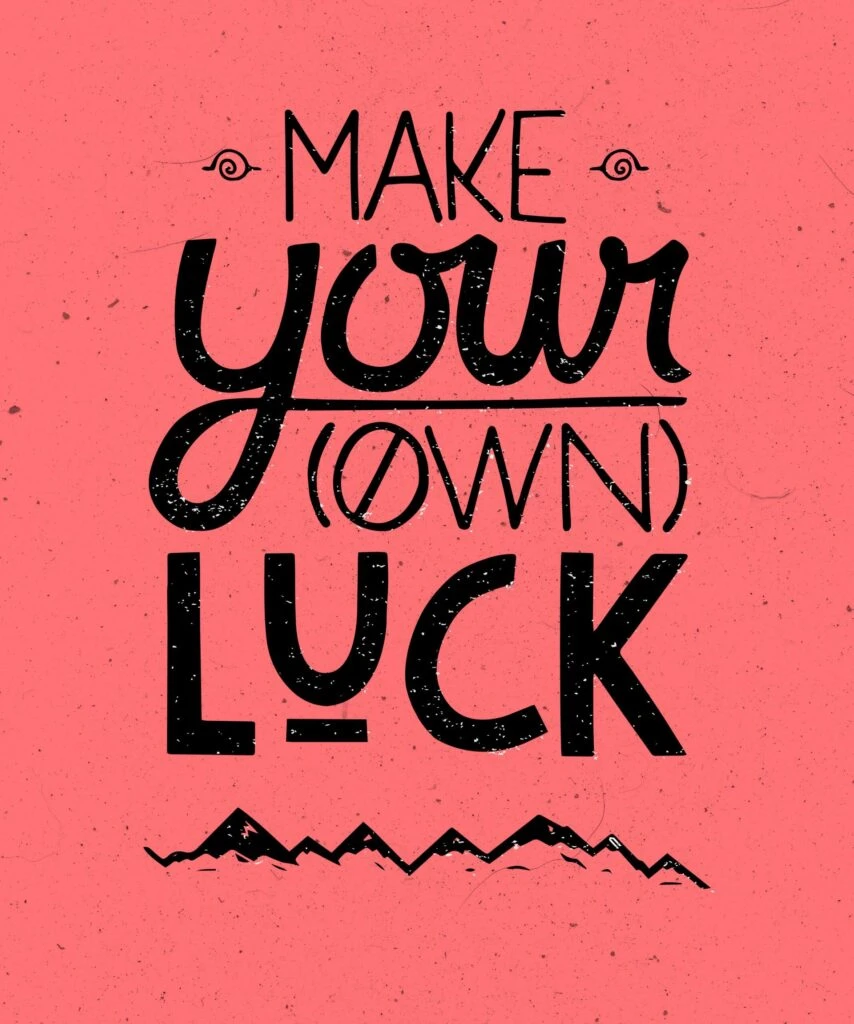
Data Collection Metrics
- Total Images Collected: 100,000 images, including both source and self-created.
- Textual Descriptions: 100,000 detailed descriptions were annotated, one per image, with an average length of 100 words.
Annotation Process
Stages
- Contextual Descriptions: Annotators provided rich textual descriptions for each image, highlighting relevant details, contextual information, and potential applications.
- Detail Capture: The descriptions were crafted to capture the intricate relationships between visual elements and their textual representations, ensuring comprehensive coverage.
Annotation Metrics
- Images Annotated: 100,000 images received detailed descriptions.
- Average Description Length: Each description averaged 100 words, ensuring sufficient detail and context.
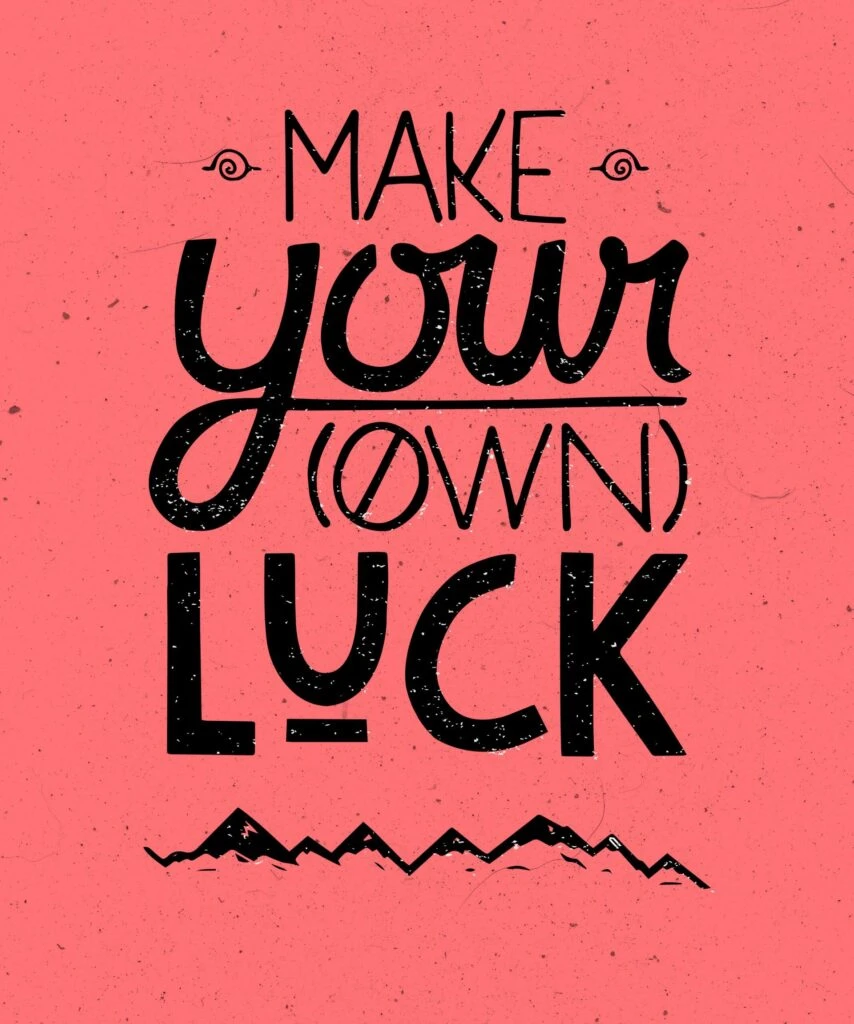
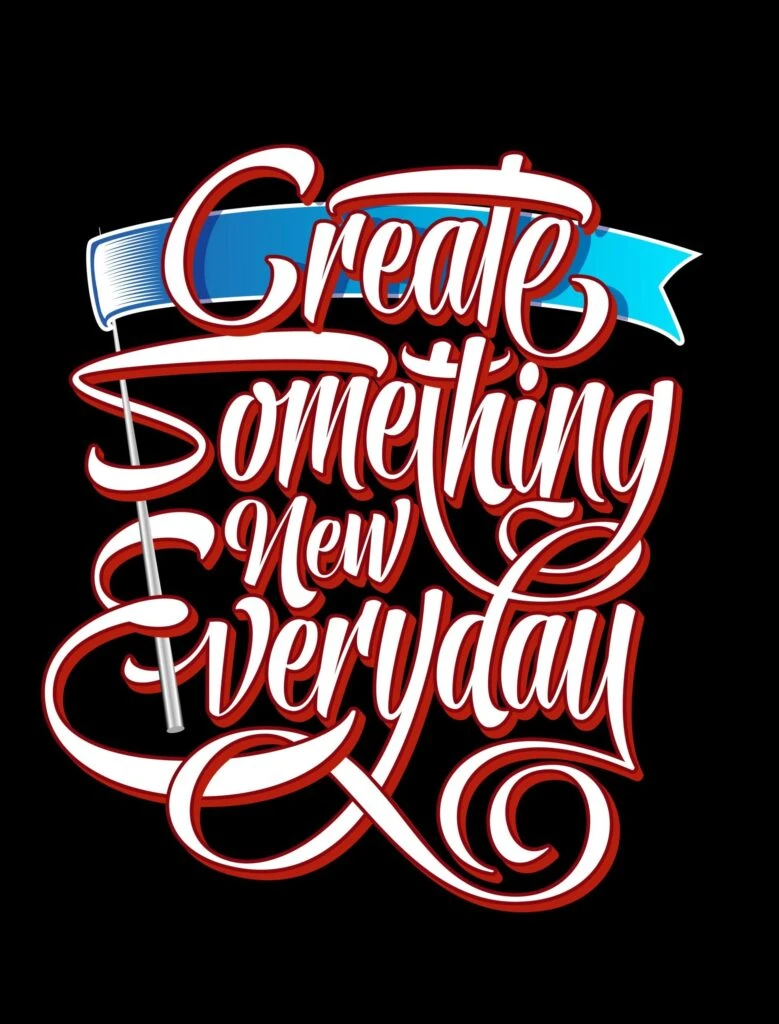
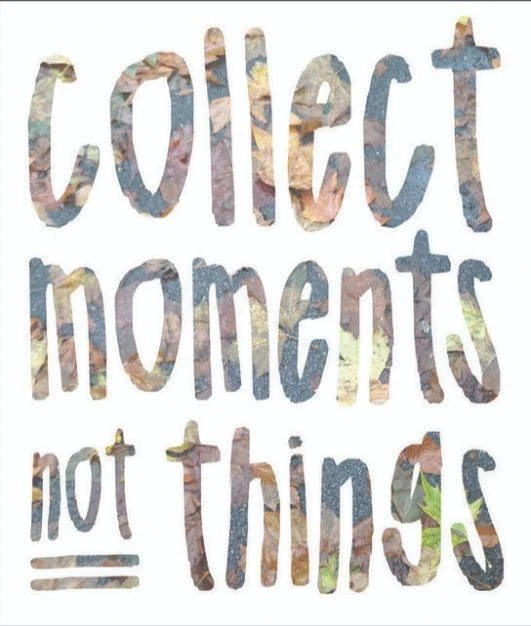
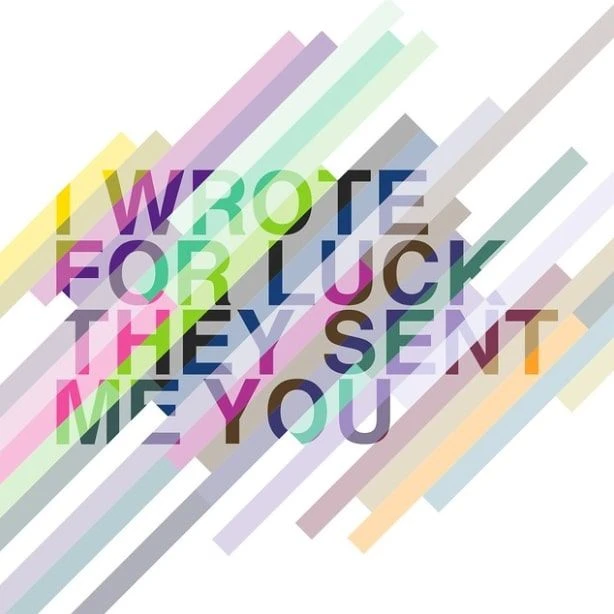
Quality Assurance
Stages
- Annotation Accuracy: Continuous review and feedback loops were implemented to maintain high standards of description accuracy and relevance.
- Consistency Checks: Regular checks were conducted to ensure uniformity in the style and depth of the descriptions across the entire dataset.
- Improvement Process: Feedback from the model’s performance was used to refine and improve the annotation process.
QA Metrics
- Description Accuracy: The project achieved a high level of accuracy in capturing the intended details and contexts within each image description.
- Consistency Rating: The consistency of annotations across the dataset was maintained at a high standard, ensuring uniform quality.
- Feedback Utilization: Continuous improvements were made based on feedback, enhancing the overall quality of the dataset.
Conclusion
The creation of the 100,000-image dataset with detailed textual descriptions represents a significant advancement in the training of Generative AI models. This dataset serves as a crucial resource for improving image-to-text generation, enabling the development of more accurate and context-aware AI applications.

Quality Data Creation

Guaranteed TAT

ISO 9001:2015, ISO/IEC 27001:2013 Certified

HIPAA Compliance

GDPR Compliance

Compliance and Security
Let's Discuss your Data collection Requirement With Us
To get a detailed estimation of requirements please reach us.