Image Annotation for Agricultural Yield Prediction
Home » Case Study » Image Annotation for Agricultural Yield Prediction
Project Overview:
Objective
Image Annotation for Agricultural Yield Prediction: Our initiative in agricultural yield prediction is poised to revolutionize farming methods. By delivering actionable, data-backed insights to farmers and industry stakeholders, we aim to boost productivity and contribute significantly to global food security. Moreover, our approach integrates cutting-edge technology with traditional farming practices, ensuring a seamless transition to more efficient methods. Additionally, our platform empowers farmers with real-time information, enabling them to make informed decisions throughout the growing season.
Scope
Transition words help guide the reader through your content, making it smoother and easier to follow. Here’s your text with transition words added and in active voice. Our project utilized computer vision and machine learning techniques to analyze and categorize agricultural imagery. This approach was instrumental in enabling accurate predictions of crop yields, which are a key factor in optimizing agricultural productivity.
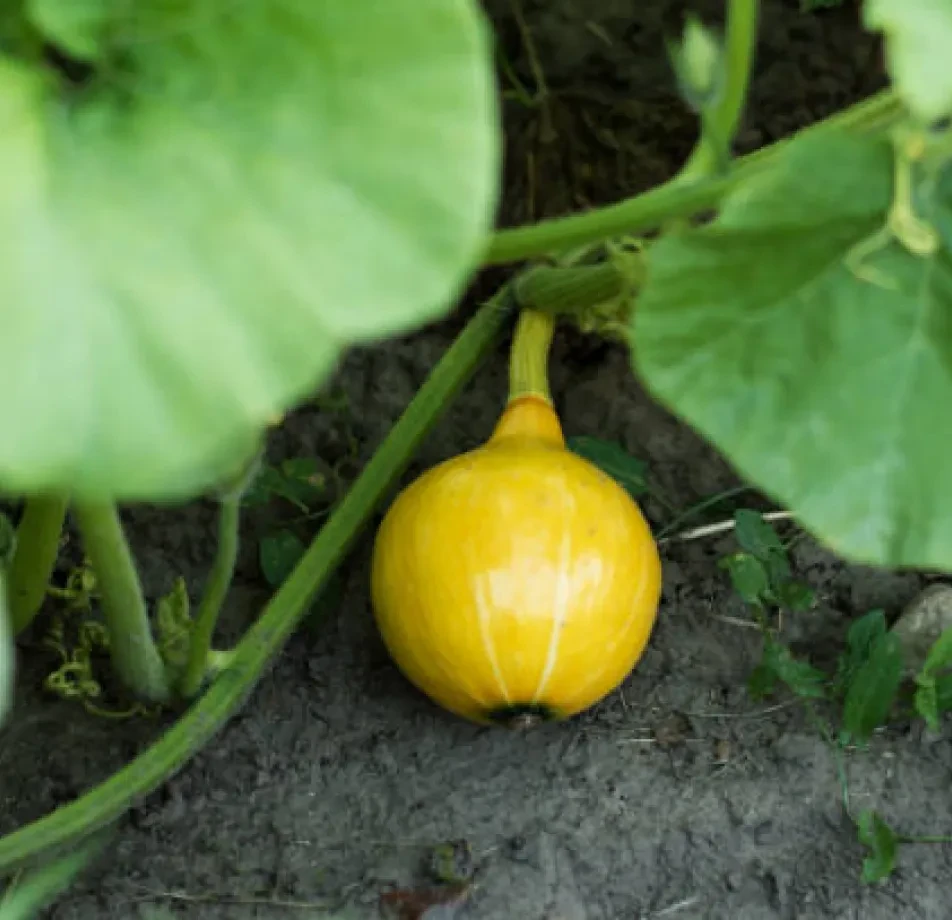
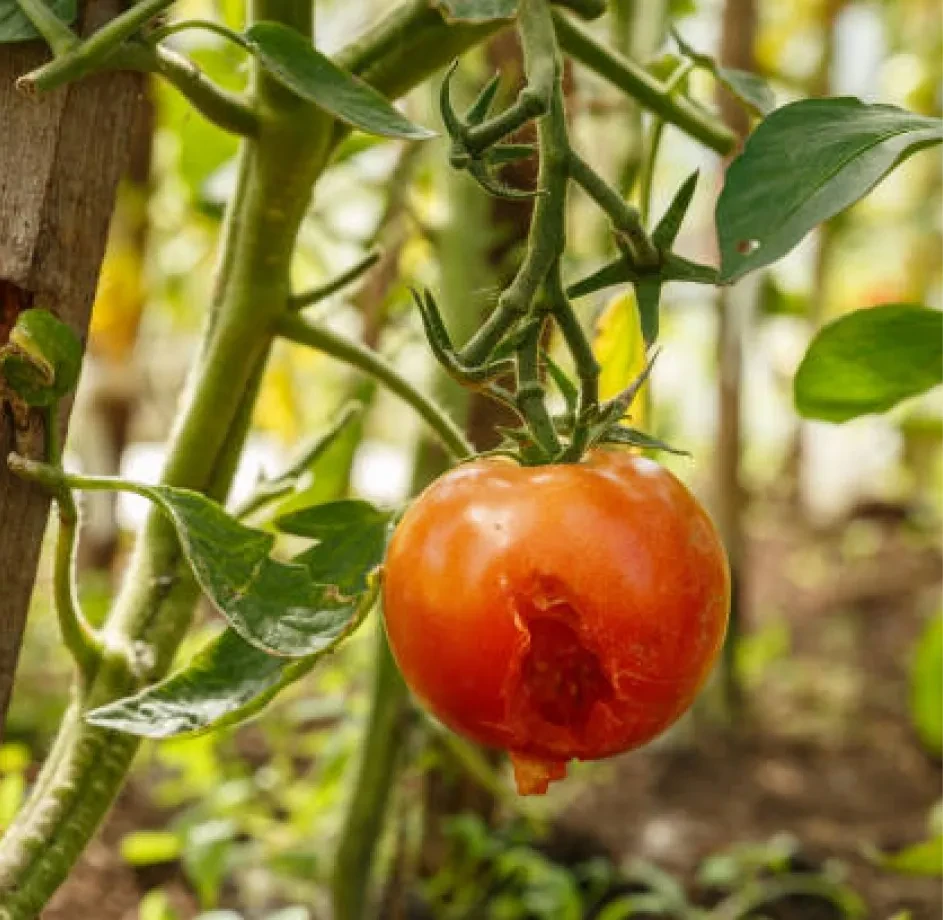
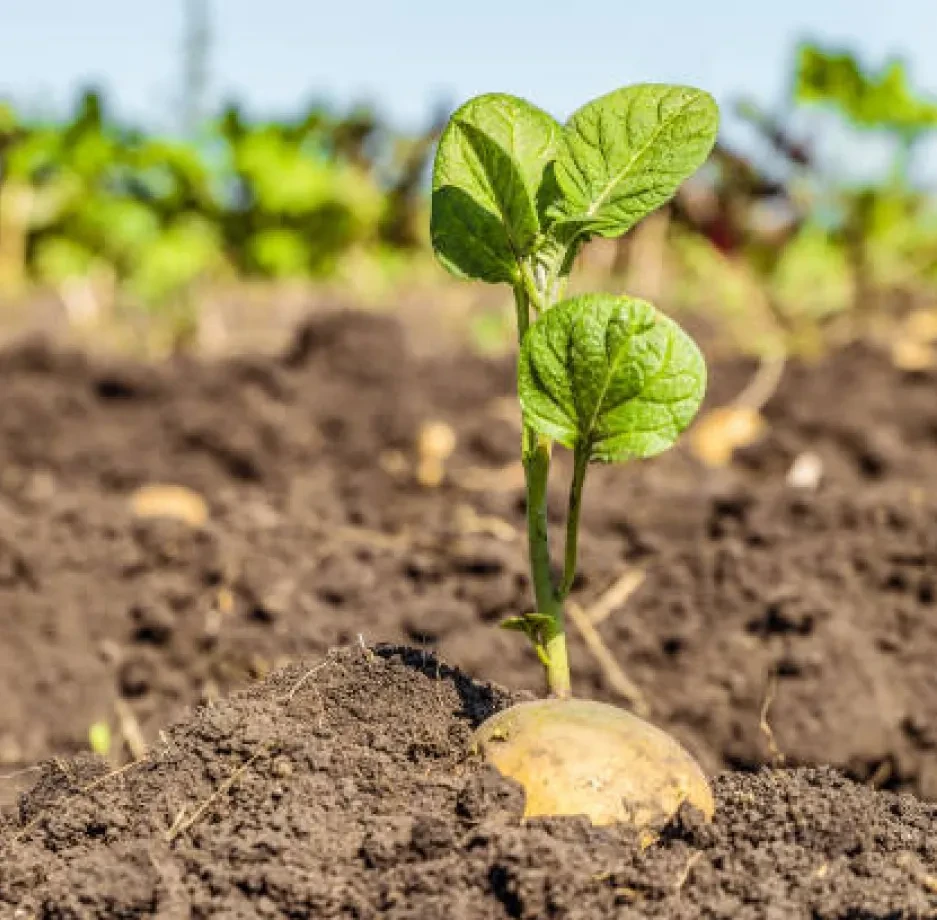
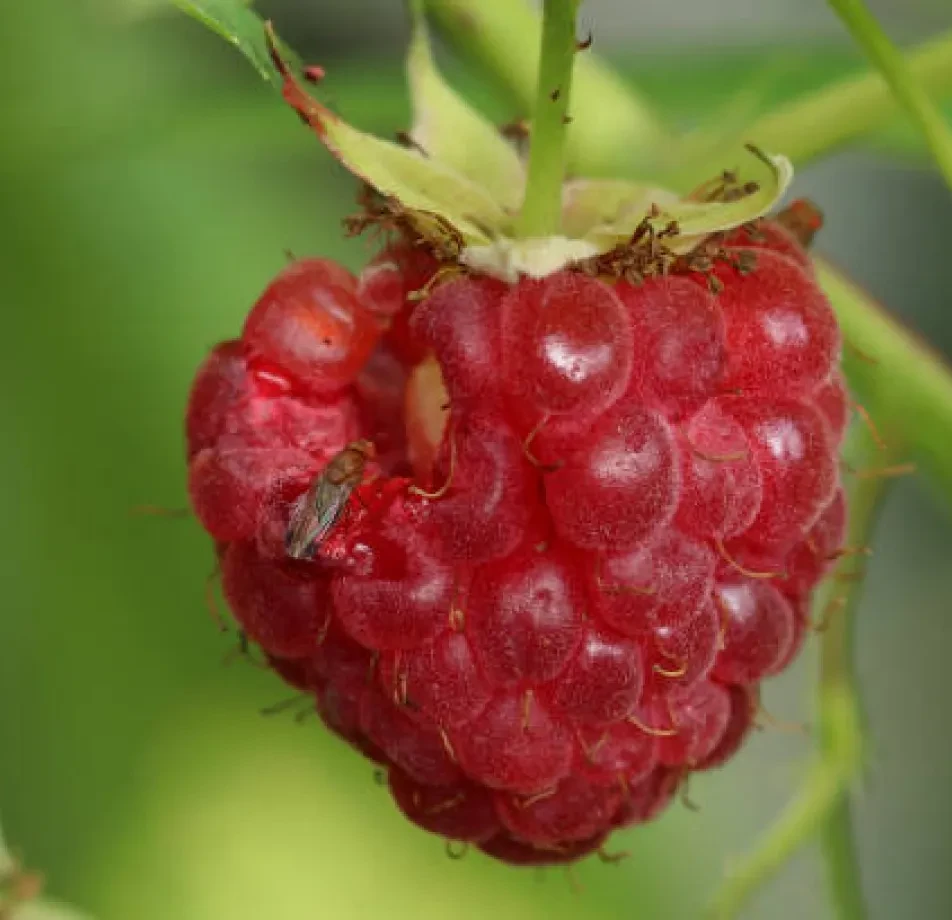
Sources
- Agricultural Research Institutions: Collaborating with agricultural research organizations provides valuable insights and datasets.
- Remote Sensing Providers: Partnering with remote sensing data providers offers access to valuable imagery.
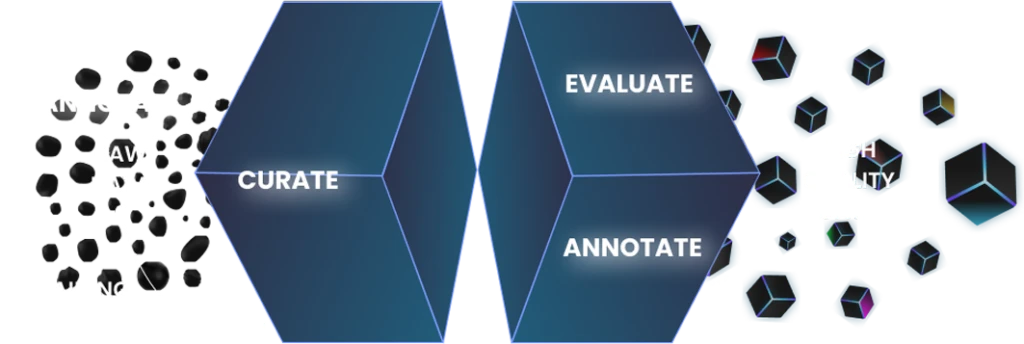
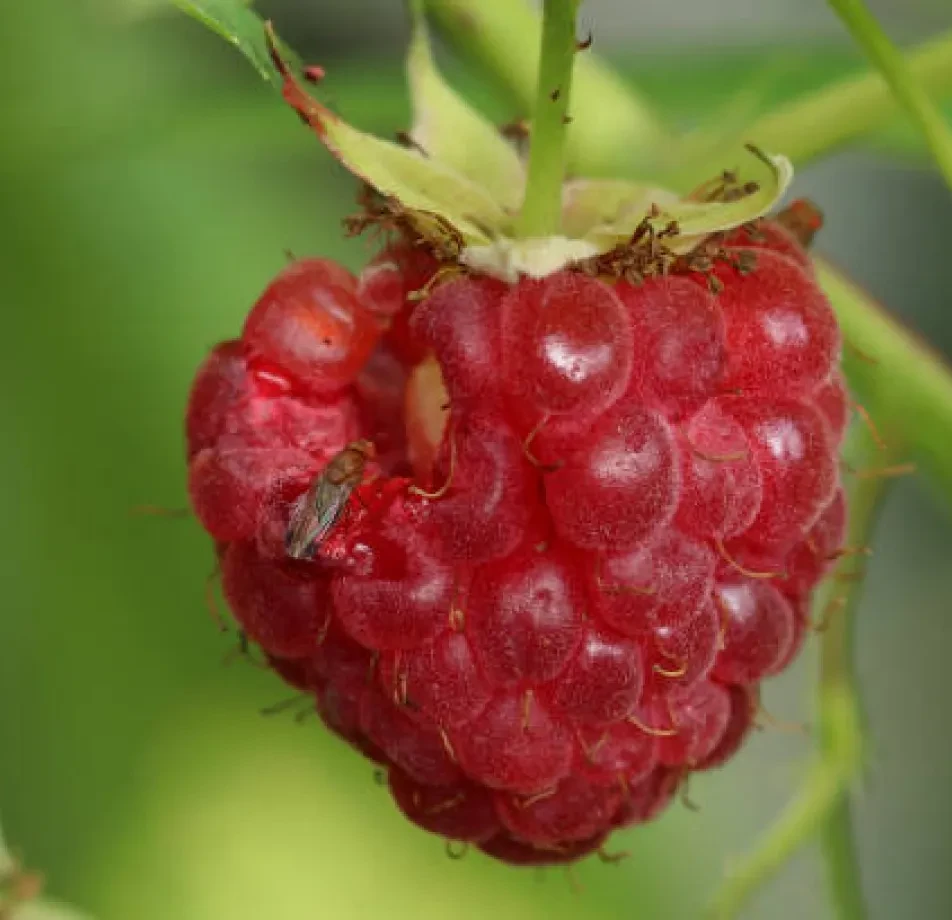
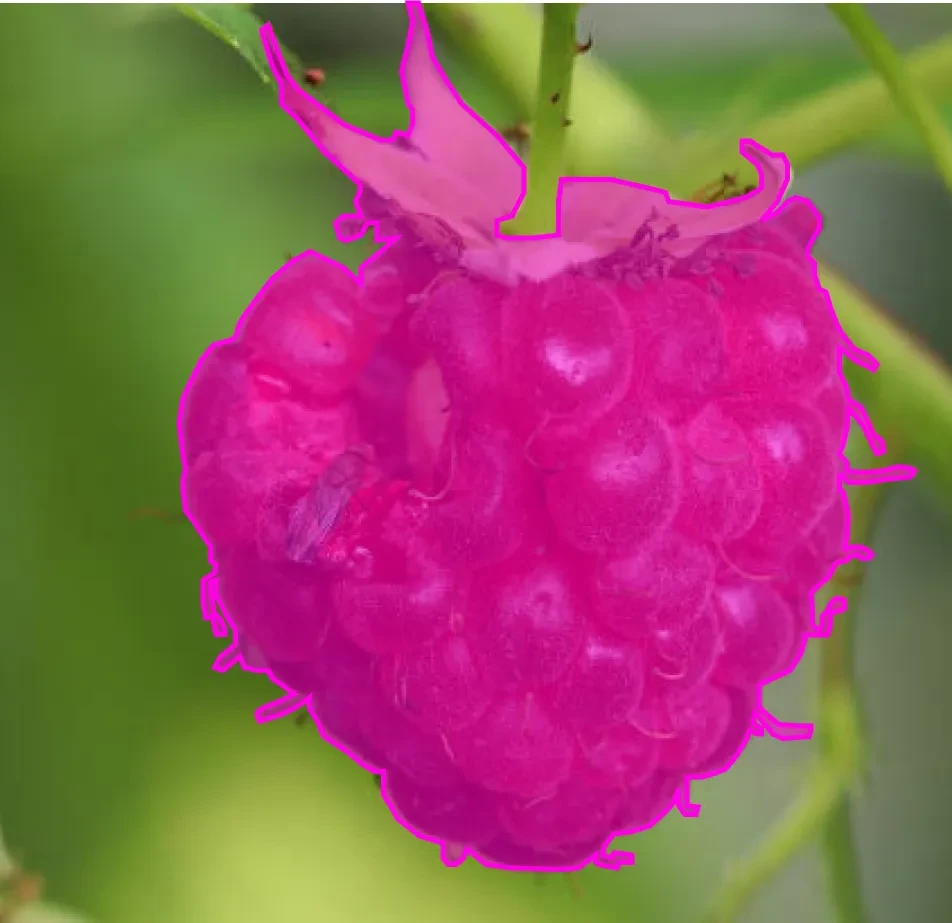
Data Collection Metrics
- Data Volume: We have successfully collected and annotated over 150,000 high-quality agricultural images. These images encompass a wide range of crops, growth stages, and environmental scenarios. Additionally, our dataset includes diverse agricultural landscapes and conditions. Moreover, each image has been meticulously annotated, providing valuable insights for various applications in agricultural research and technology development.
- Data Quality: We prioritized ensuring the highest accuracy and reliability in our annotations, thereby forming a robust foundation for precise yield predictions. Additionally, by implementing stringent quality control measures, we fortified the integrity of our data. Furthermore, through meticulous analysis and verification processes, we solidified the credibility of our predictions. In conclusion, our focus on accuracy and reliability underscores our commitment to delivering precise yield projections.
Annotation Process
Stages
- Data Gathering: We meticulously compiled a comprehensive dataset featuring a variety of agricultural images.
- Annotation: Our team expertly labeled each image with relevant details like crop types and growth stages.
- Preprocessing: We standardized this data to maintain consistency in format and quality.
- Model Training: Utilizing advanced machine learning algorithms, we trained models for yield prediction.
- Validation: We rigorously tested these models to guarantee accurate yield forecasts.
- Integration: The models were then seamlessly integrated into agricultural systems for real-time predictions.
- Monitoring and Maintenance: Continuous performance monitoring ensures our models stay relevant and effective.
Annotation Metrics
- Inter-Annotator Agreement (IAA): Additionally, this metric played a pivotal role in ensuring uniformity and reliability in our image labeling process. Moreover, we achieved a high percentage of correctly labeled features, thereby underlining the precision of our annotations.
- Annotation Accuracy: We achieved a high percentage of correctly labeled features, thereby highlighting the precision of our annotations. Moreover, our team meticulously curated the data, ensuring accuracy at every step. Additionally, we employed advanced algorithms to further validate the annotations, bolstering their reliability. Furthermore, through rigorous testing, we confirmed the robustness of our annotation process.
- Annotation Efficiency: We achieved a high percentage of correctly labeled features, thereby highlighting the precision of our annotations. Moreover, our team meticulously curated the data, ensuring accuracy at every step. Additionally, we employed advanced algorithms to further validate the annotations, bolstering their reliability. Furthermore, through rigorous testing, we confirmed the robustness of our annotation process.
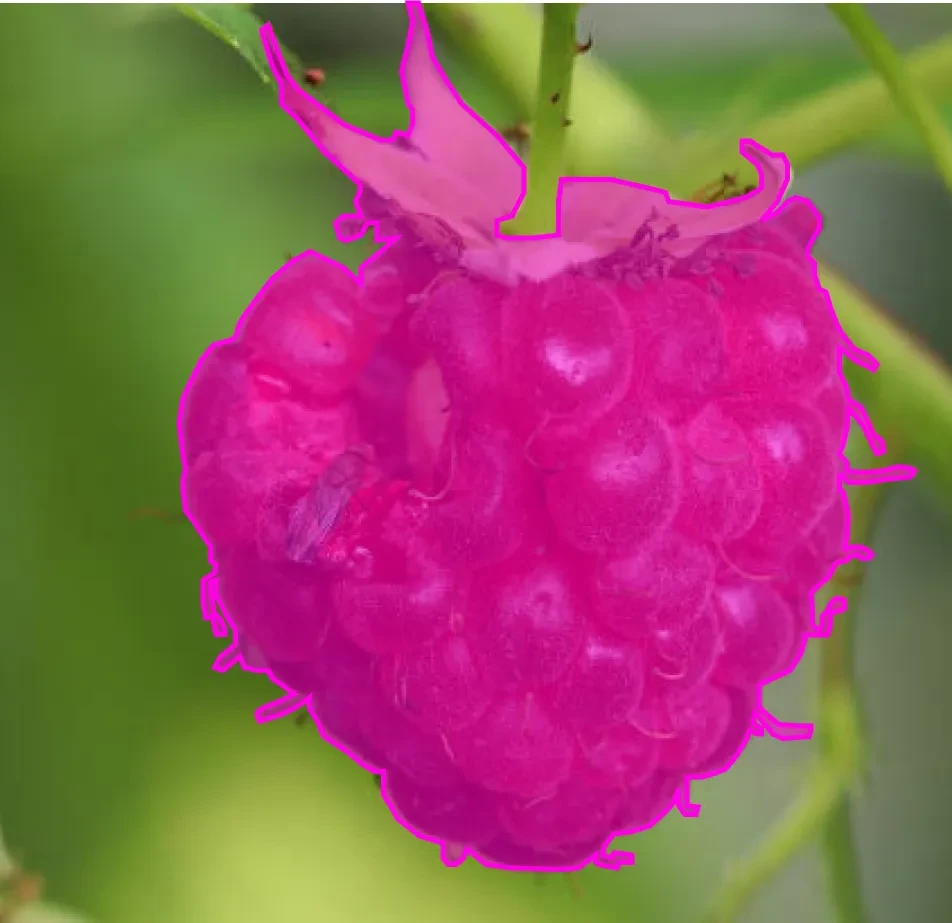
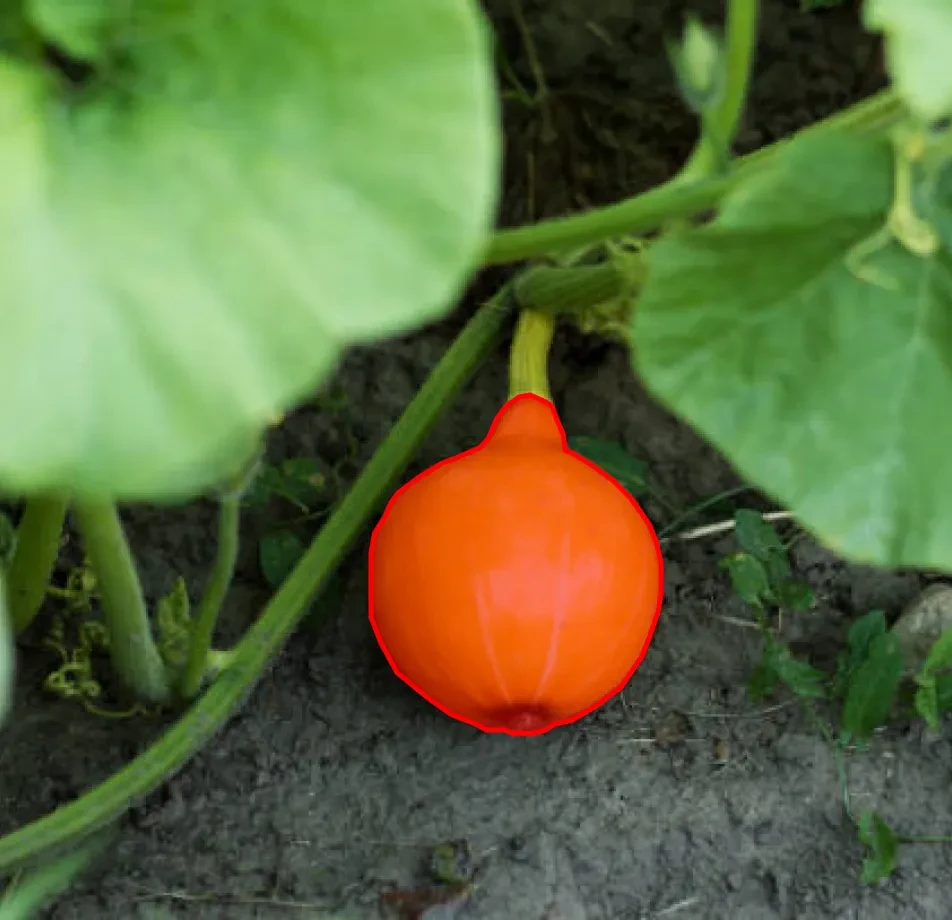
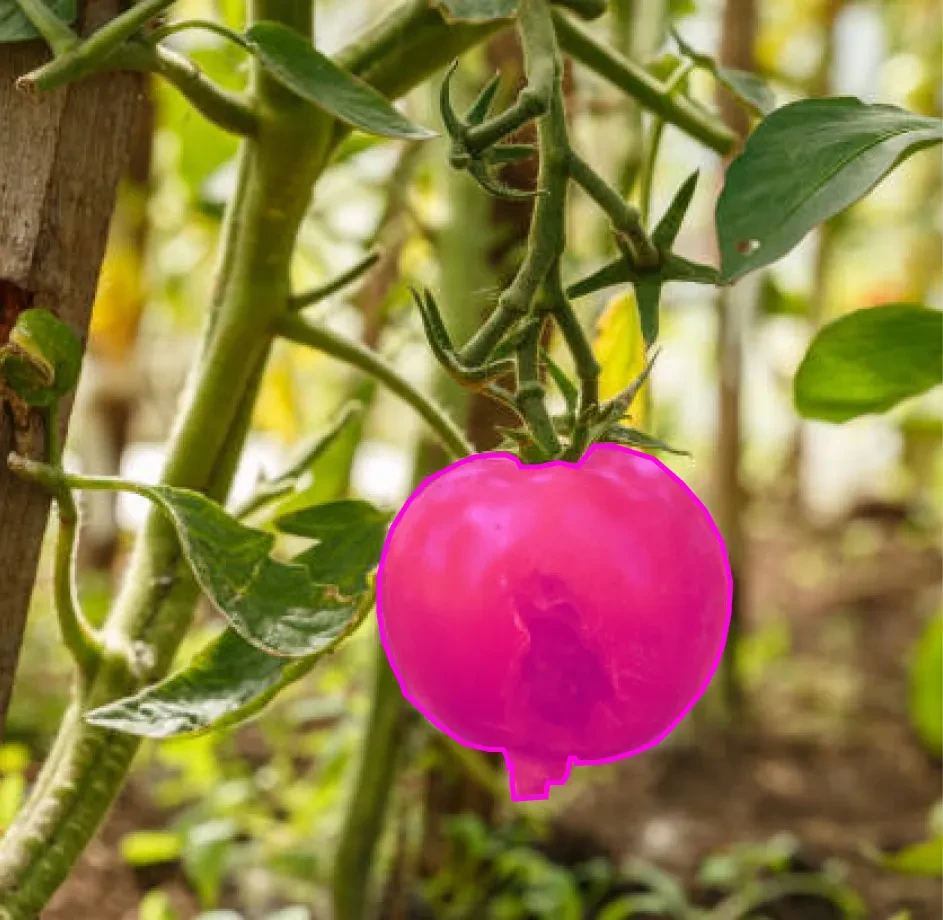
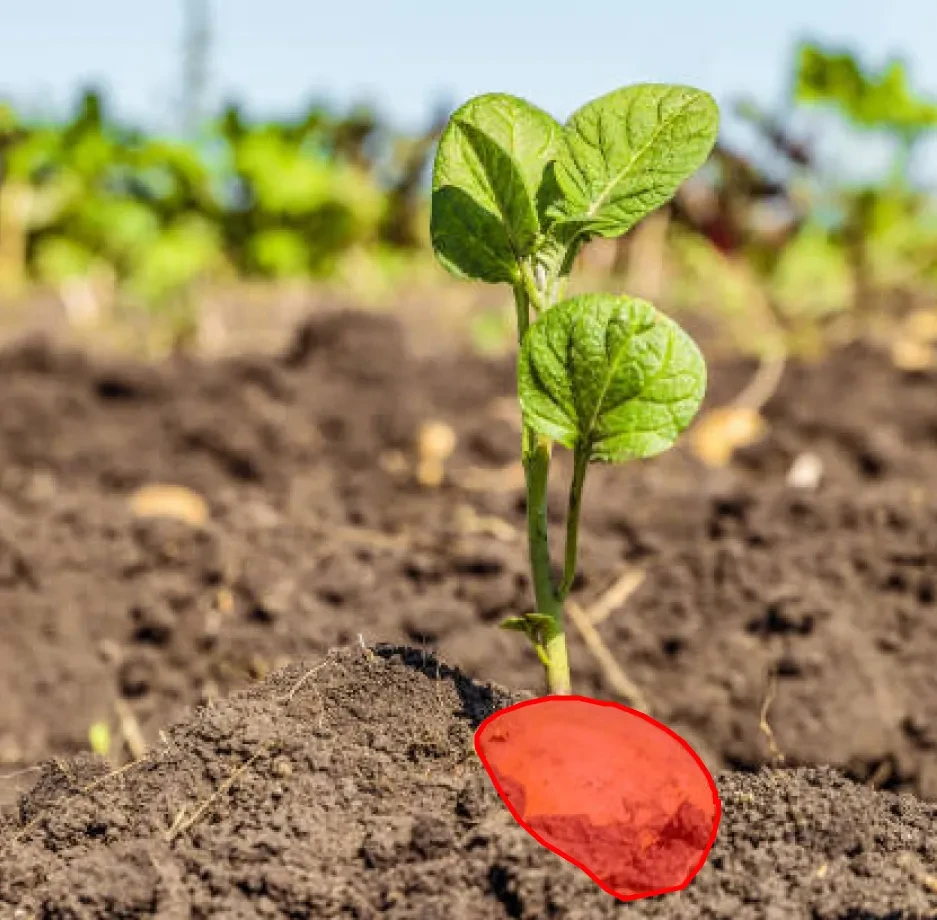
Quality Assurance
Stages
Data Privacy: Ensure data security and compliance with privacy regulations.
Quality Control: Maintain high annotation accuracy through rigorous quality checks.
Ethical Practices: Adhere to ethical guidelines in data collection and annotation.
QA Metrics
- Prediction Accuracy: Evaluate yield prediction precision.
- Model Reliability: Assess consistency and trustworthiness.
Conclusion
In the context of agricultural yield prediction, image annotation emerges as a valuable tool that harnesses the power of computer vision to analyze and categorize agricultural imagery. Additionally, by providing labeled data for machine learning models, image annotation facilitates accurate predictions of crop yields, thereby assisting farmers in making informed decisions about planting, harvesting, and resource allocation.

Quality Data Creation

Guaranteed TAT

ISO 9001:2015, ISO/IEC 27001:2013 Certified

HIPAA Compliance

GDPR Compliance

Compliance and Security
Let's Discuss your Data collection Requirement With Us
To get a detailed estimation of requirements please reach us.