Hair Semantic Segmentation Dataset
Home » Case Study » Hair Semantic Segmentation Dataset
Project Overview:
Objective
To assemble a dedicated dataset for the semantic segmentation of hair in human portraits, follow these steps. This dataset will be highly beneficial for various applications, such as digital image editing, virtual hair color testing, augmented reality applications, and improvements in facial recognition algorithms.
Scope
To collect a broad set of portrait images reflecting different hair types, lengths, colors, and styles, we will ensure that the annotations clearly separate the hair from the face and other features.
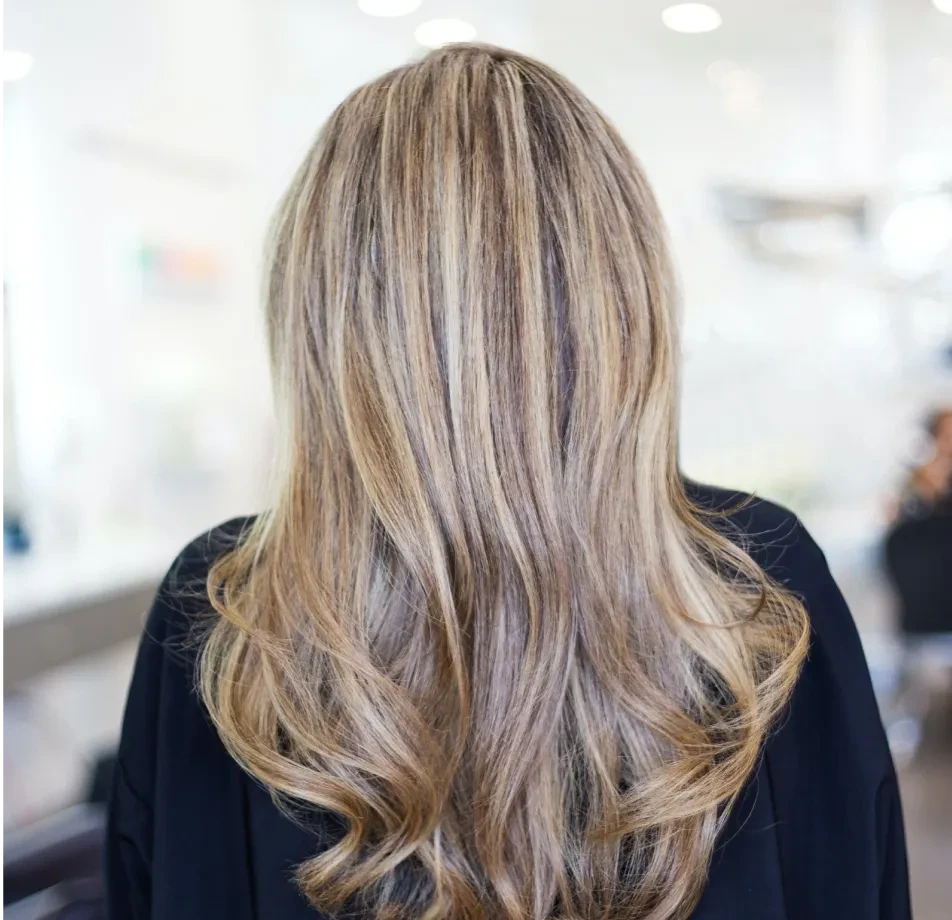
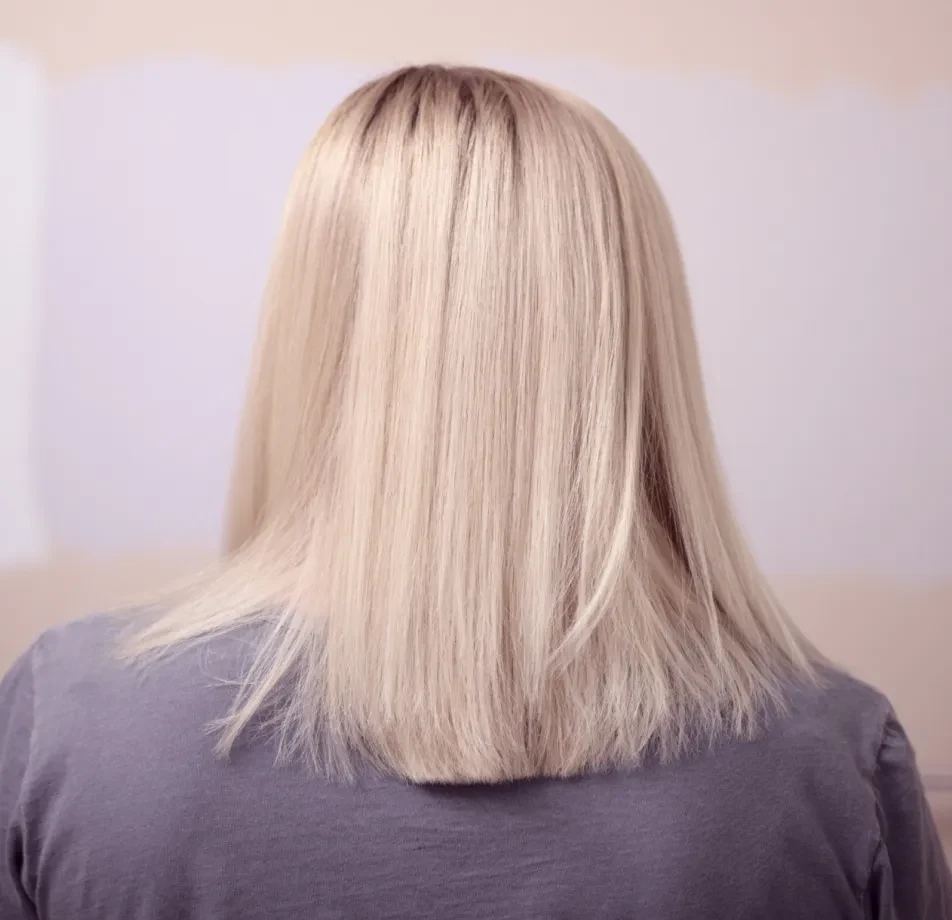
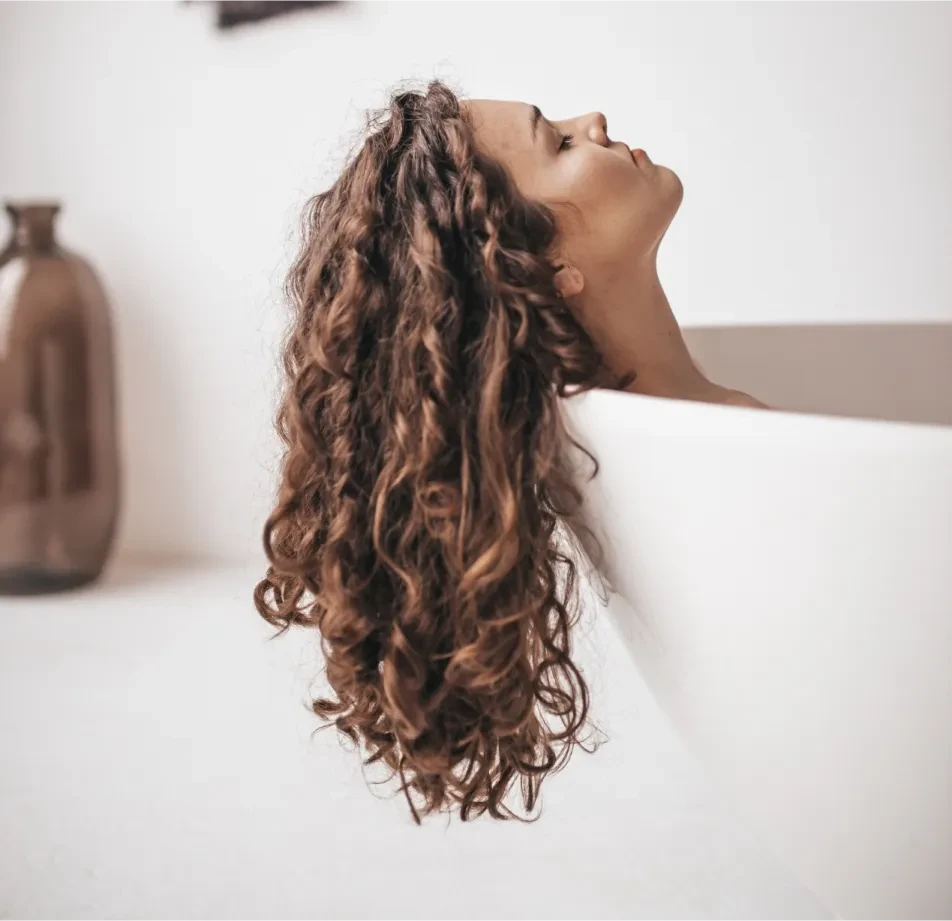
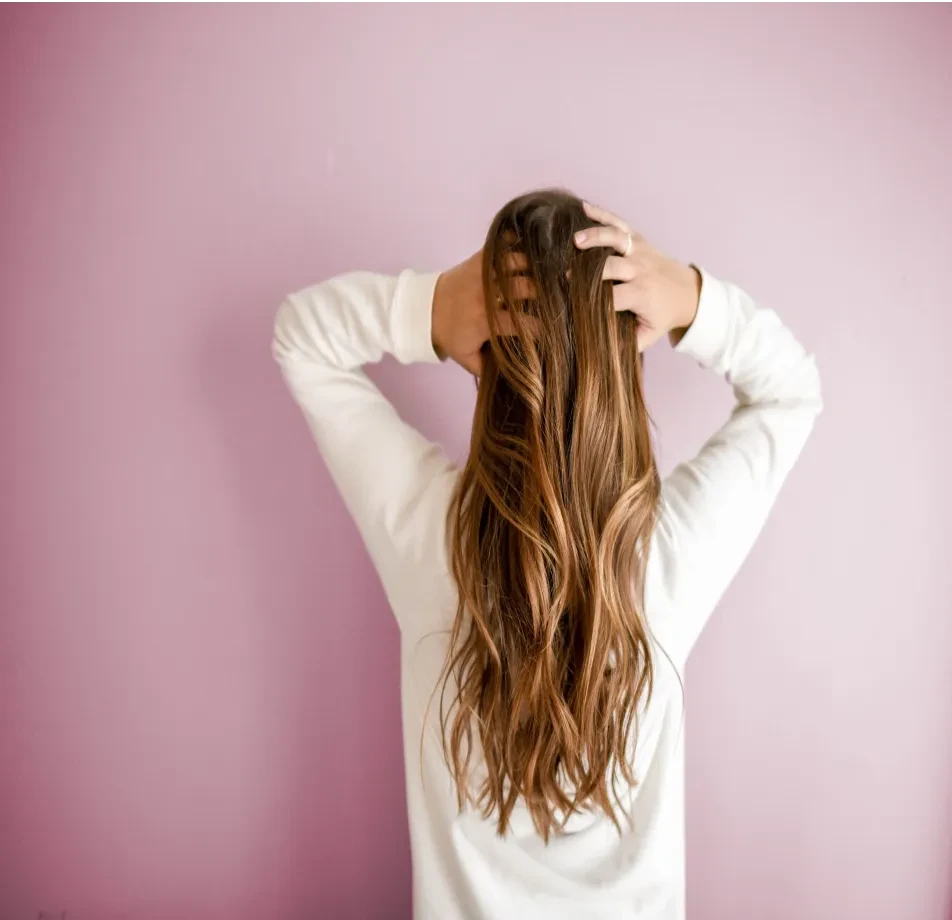
Sources
- Studio portraits capturing diverse hair styles and types.
- Outdoor images under different lighting conditions.
- Candid shots from social gatherings and events.
- Selfies from varied demographics.
- Images showcasing hair accessories, hats, and other headwear.
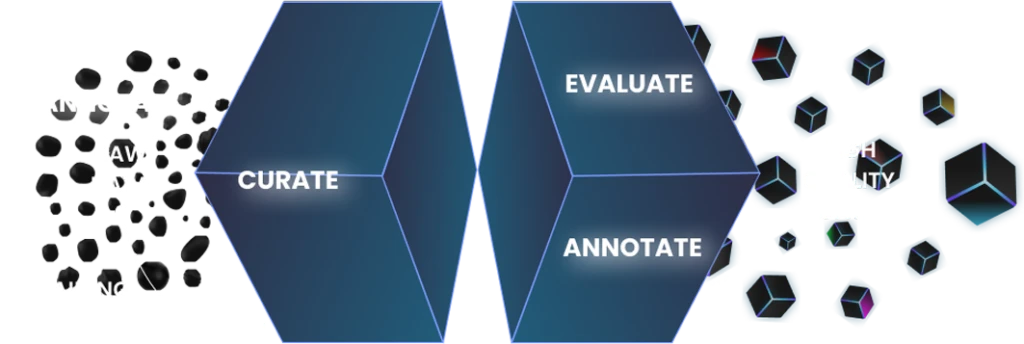
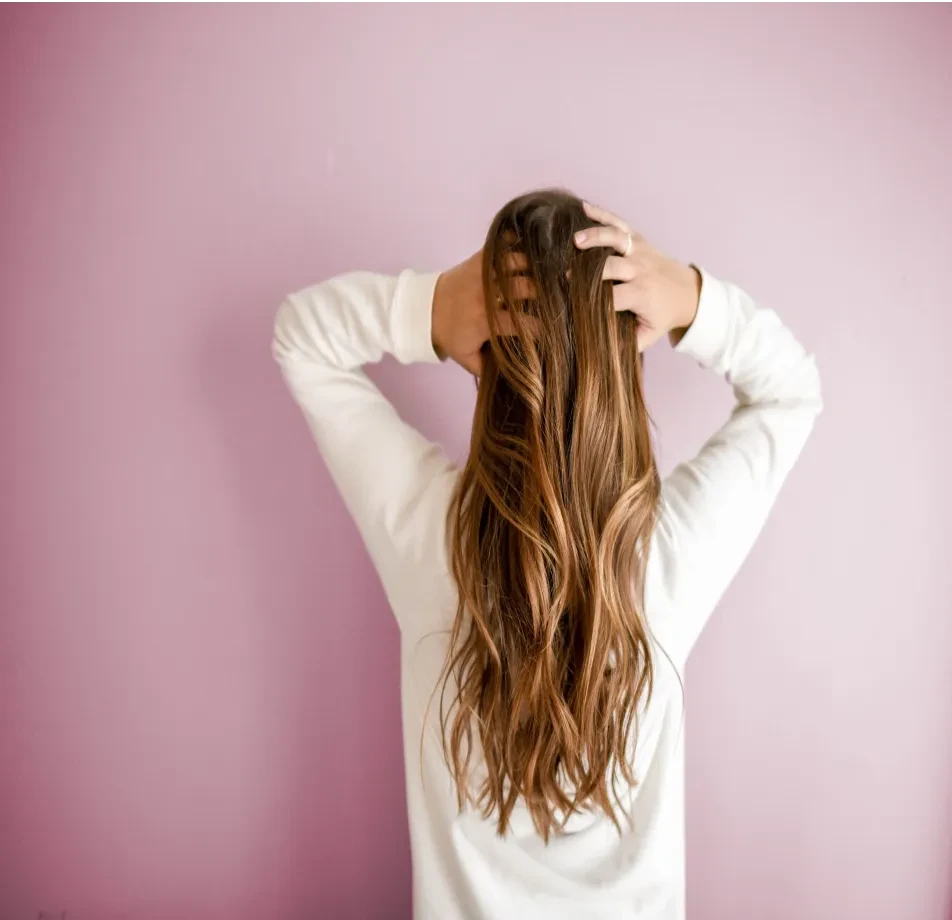
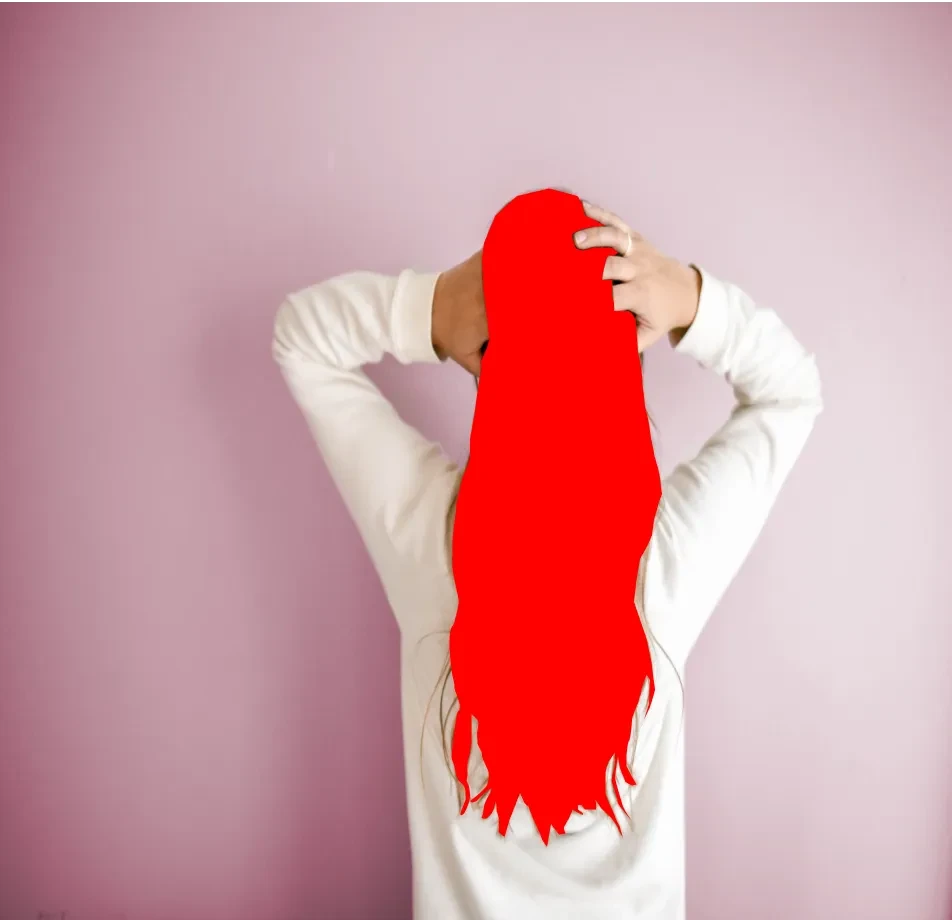
Data Collection Metrics
- Total Data Points: 280,000 images
- Studio Portraits: 80,000
- Outdoor Images: 70,000
- Candid Shots: 60,000
- User-submitted Selfies: 50,000
- Headwear and Accessories: 20,000
Annotation Process
Stages
- Raw Data Cleaning: First, remove any images that are blurry, have obstructed views of hair, or contain redundant data.
- Hair Segmentation: Next, annotate and distinguish the hair region from the rest of the image. Pay special attention to details like flyaway hairs.
- Sub-segmentation (where applicable): Additionally, further segment the image to differentiate between primary hair, sideburns, and beards/moustaches in subjects.
- Quality Review: Finally, ensure that all annotations are accurate and consistent across the dataset.
Annotation Metrics
- Total Hair Segmentations: 280,000
- Sub-segmentations (like beards): 40,000
- Annotations Reviewed: 56,000 (20% of total segmentations for quality assurance)
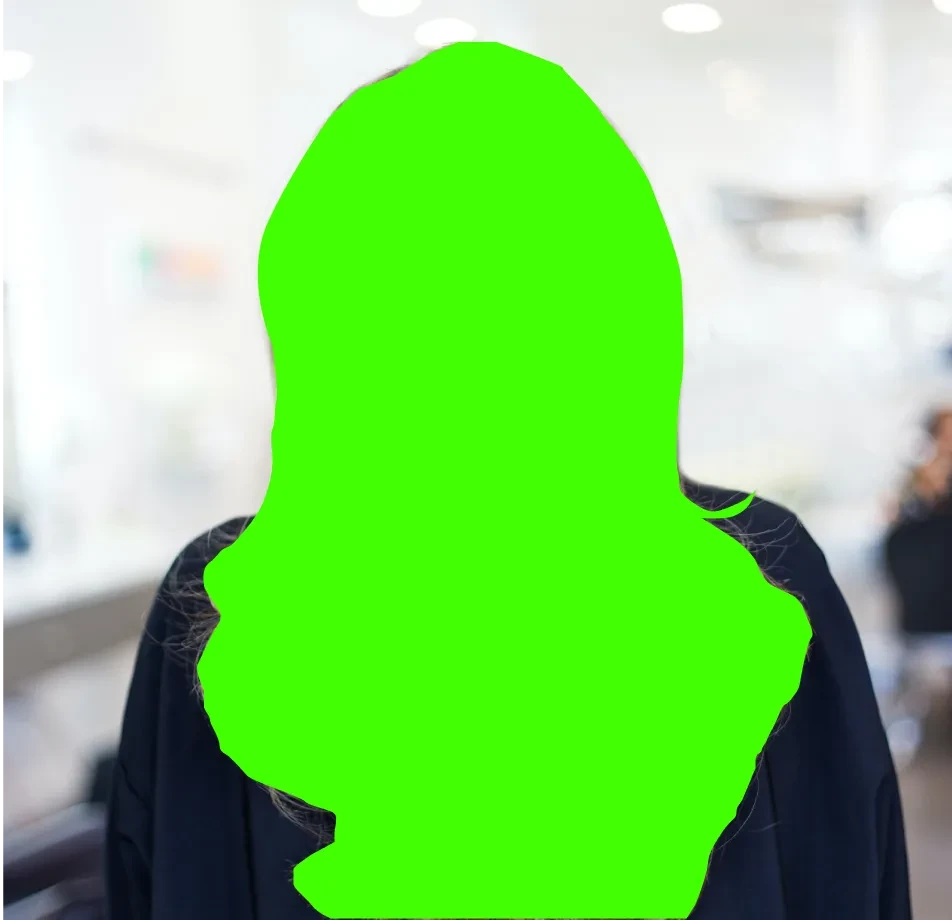
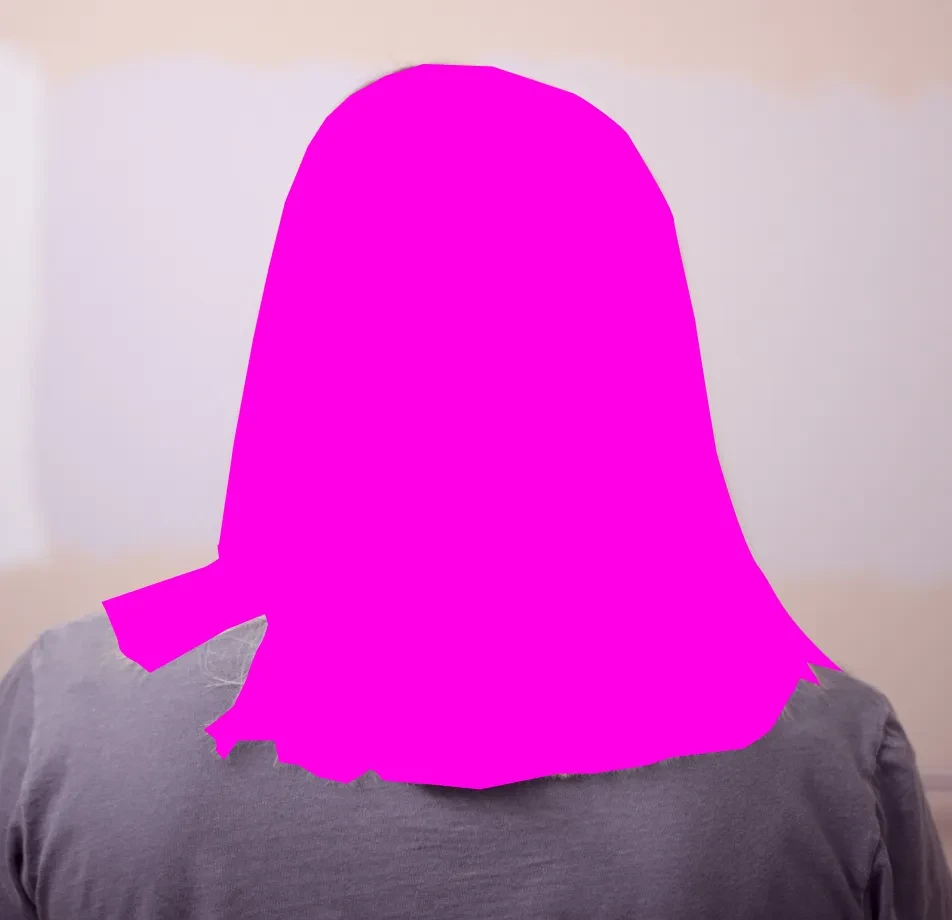
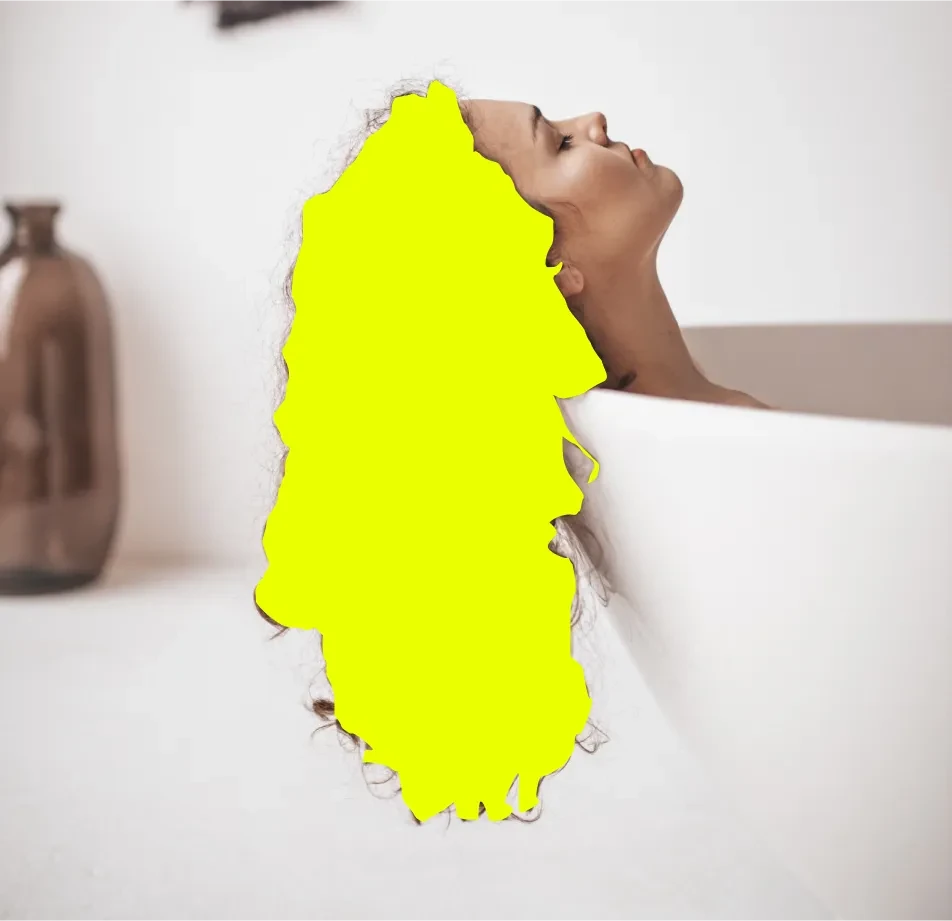
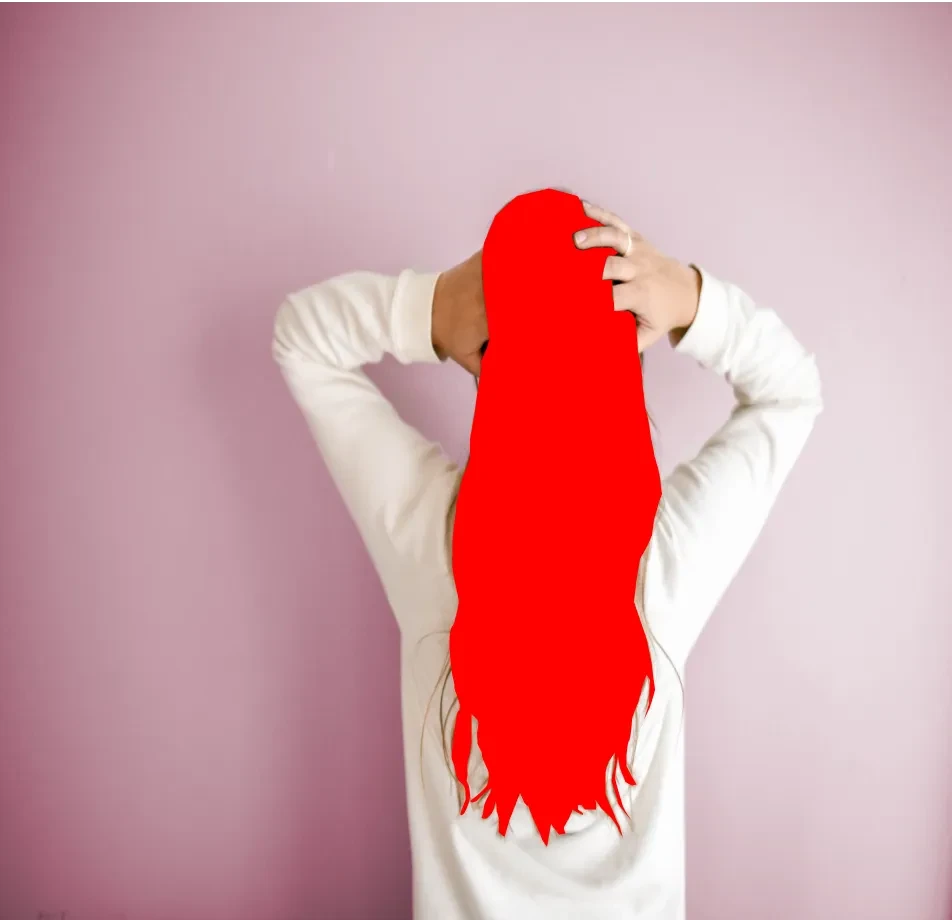
Quality Assurance
Stages
Expert Review: Hairstylists and digital artists reviewed parts of the dataset to check the accuracy of annotations.
Automated Consistency Checks: Additionally, tools were used to find any discrepancies in the annotations.
Inter-annotator Agreement: Furthermore, several annotators worked on the same image sets to ensure the annotations were consistent.
QA Metrics
- Annotations Reviewed by Experts: 56,000 (20% of total annotations)
- Inconsistencies Identified and Rectified: 5,600 (2% of total annotations)
Conclusion
The Hair Semantic Segmentation Dataset is poised to become a vital resource for various tech domains, particularly in the beauty and entertainment industry. By focusing on the intricate details and varied manifestations of human hair, this dataset stands ready to facilitate numerous applications. For instance, it can be used in virtual beauty trials, as well as in enhanced AI-driven photo editing tools.

Quality Data Creation

Guaranteed TAT

ISO 9001:2015, ISO/IEC 27001:2013 Certified

HIPAA Compliance

GDPR Compliance

Compliance and Security
Let's Discuss your Data collection Requirement With Us
To get a detailed estimation of requirements please reach us.