Glasses Segmentation Dataset
Home » Case Study » Glasses Segmentation Dataset
Project Overview:
Objective
To curate a dedicated dataset for segmenting glasses on human faces, we aim to advance augmented reality applications, virtual try-ons for eyewear shopping, and facial recognition improvements, among other uses. However, there are some areas that need improvement.
Scope
To gather a diverse set of facial images showcasing various types of eyewear, including sunglasses, prescription glasses, reading glasses, and specialty eyewear, we need to ensure the images are well-annotated. These annotations should clearly segment the glasses from the rest of the face. This will involve careful planning and execution to achieve the best results.
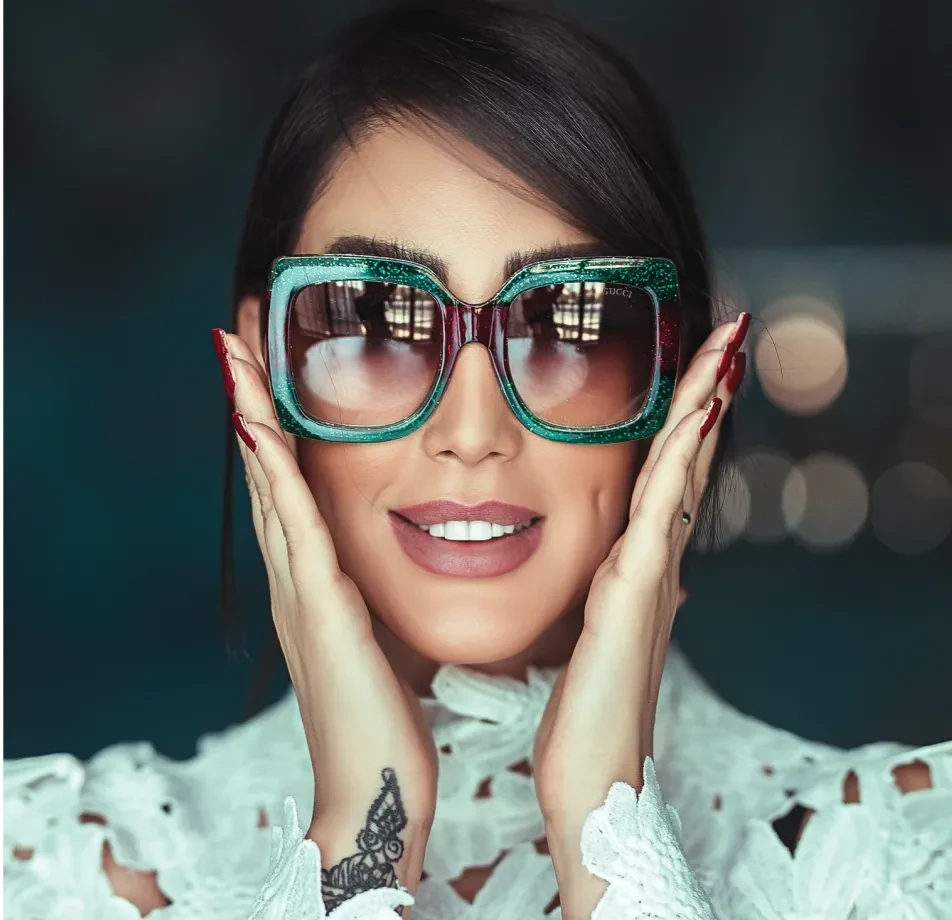
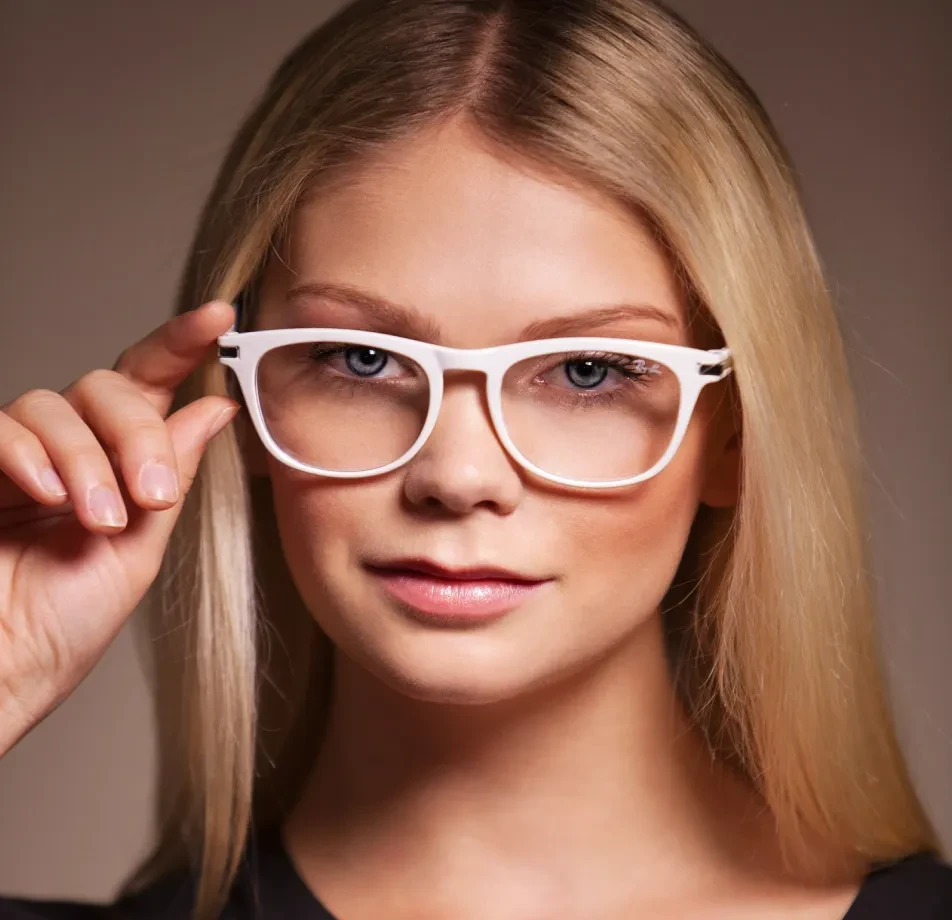
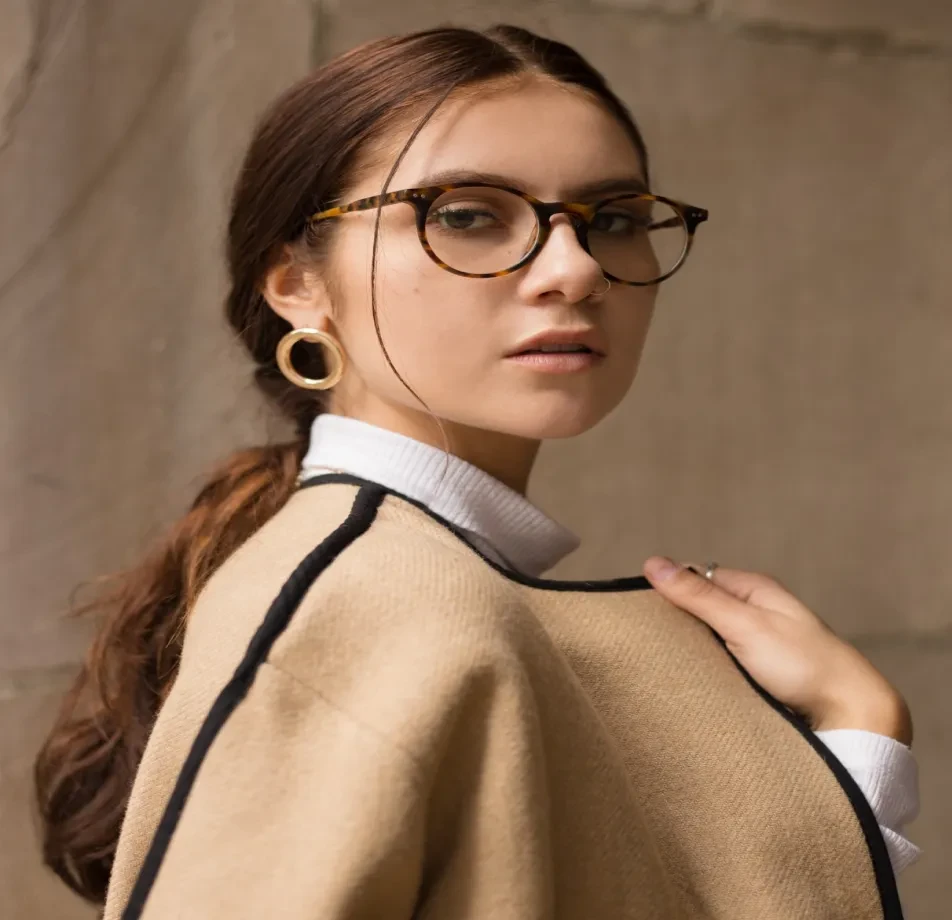
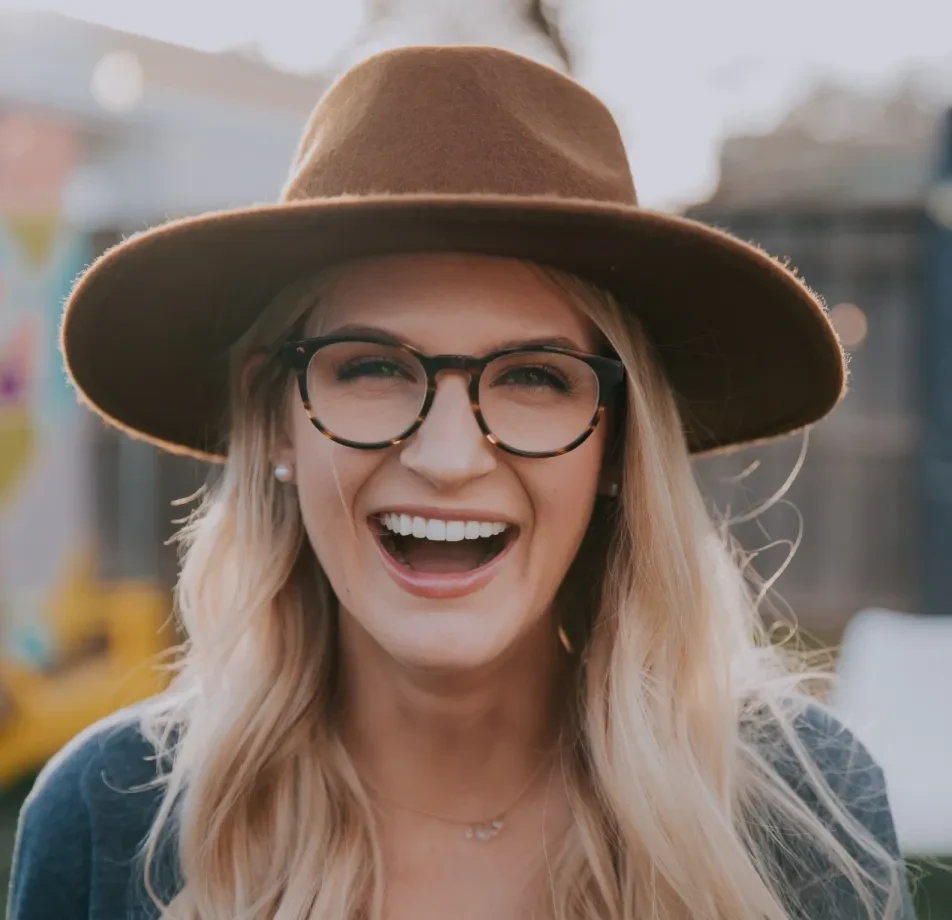
Sources
- Studio portraits with and without glasses, under varying lighting conditions.
- Public events, gatherings, and outdoor settings.
- User-submitted images and selfies.
- Images encompassing different face shapes, ages, ethnicities, and skin types.
- Variety of eyewear styles: rimless, full-rim, half-rim, oversized, etc.
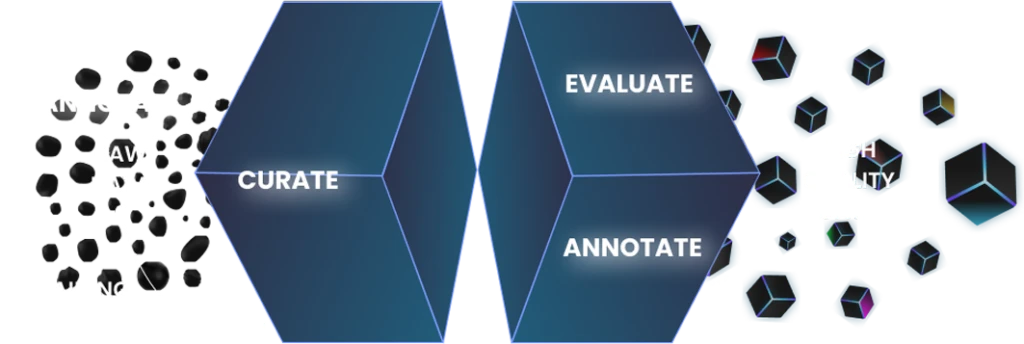
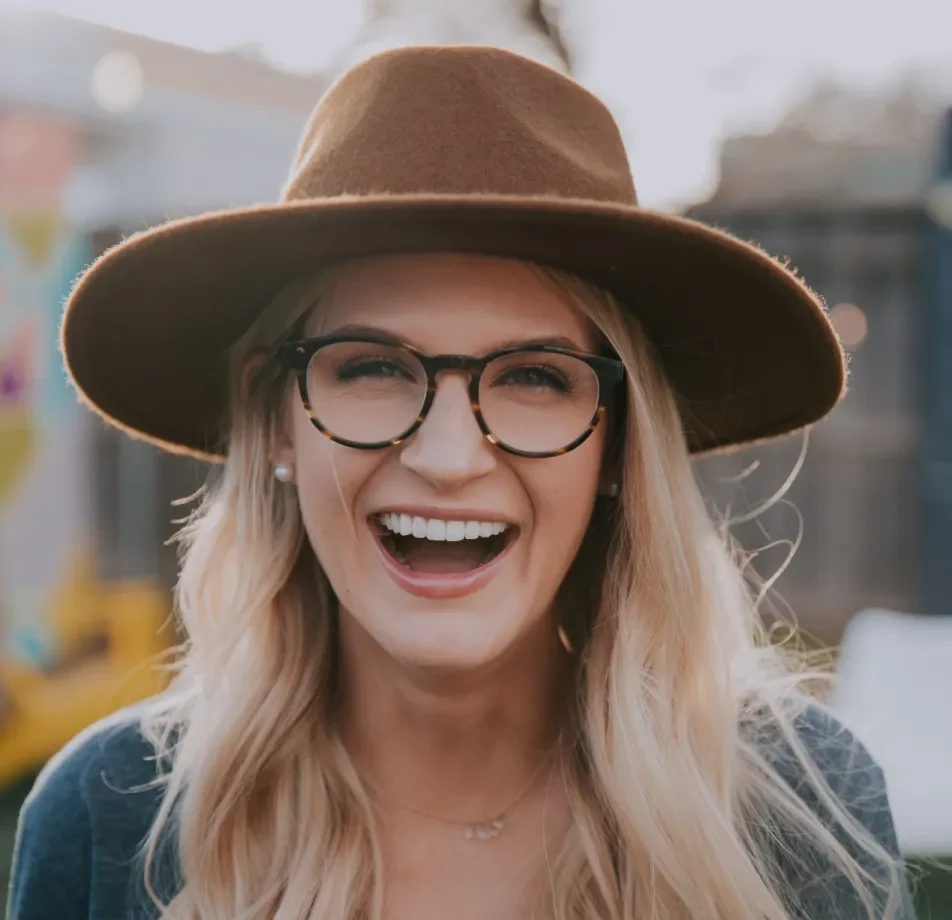
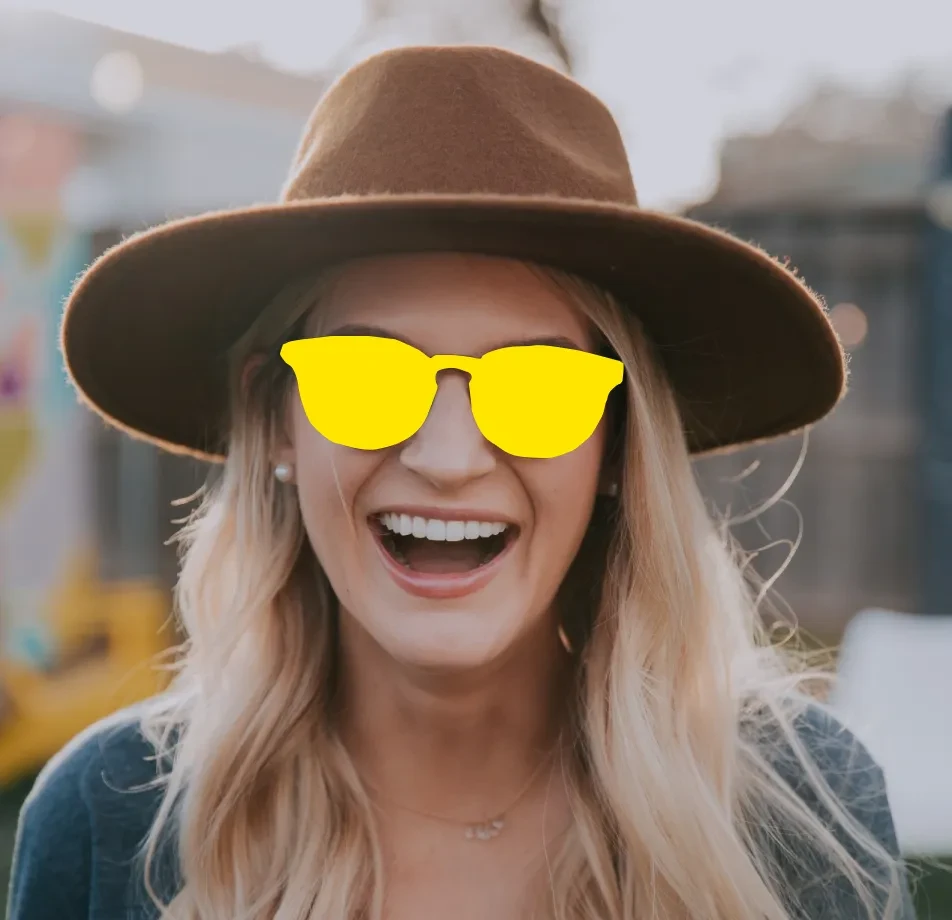
Data Collection Metrics
- Total Data Points: 250,000 images
- Studio Portraits: 60,000
- Public Event Shots: 70,000
- User-submitted Selfies: 90,000
- Diverse Eyewear Styles: 30,000
Annotation Process
Stages
- Raw Data Cleaning: Firstly, we will exclude images that show signs of blurriness, obstructions, or poor focus on the eyewear. This step is essential to ensure that only high-quality images proceed to the next stages.
- Glasses Segmentation: Next, we will annotate and segment the glasses in each image. By doing this, we can differentiate the glasses from the face and other facial features, ensuring clear boundaries between them.
- Frame and Lens Differentiation: After segmenting the glasses, we will further distinguish between the frames and the lenses. This additional segmentation helps in accurately identifying and separating these components.
- Quality Review: Lastly, we will conduct comprehensive checks to validate the consistency and accuracy of our annotations. This quality review ensures that all segments are correctly identified and marked.
Annotation Metrics
- Total Glasses Segmentations: 250,000
- Frame and Lens Differentiations: 500,000 (2 segments per image on average)
- Annotations Reviewed: 50,000 (20% of total segmentations for quality assurance)
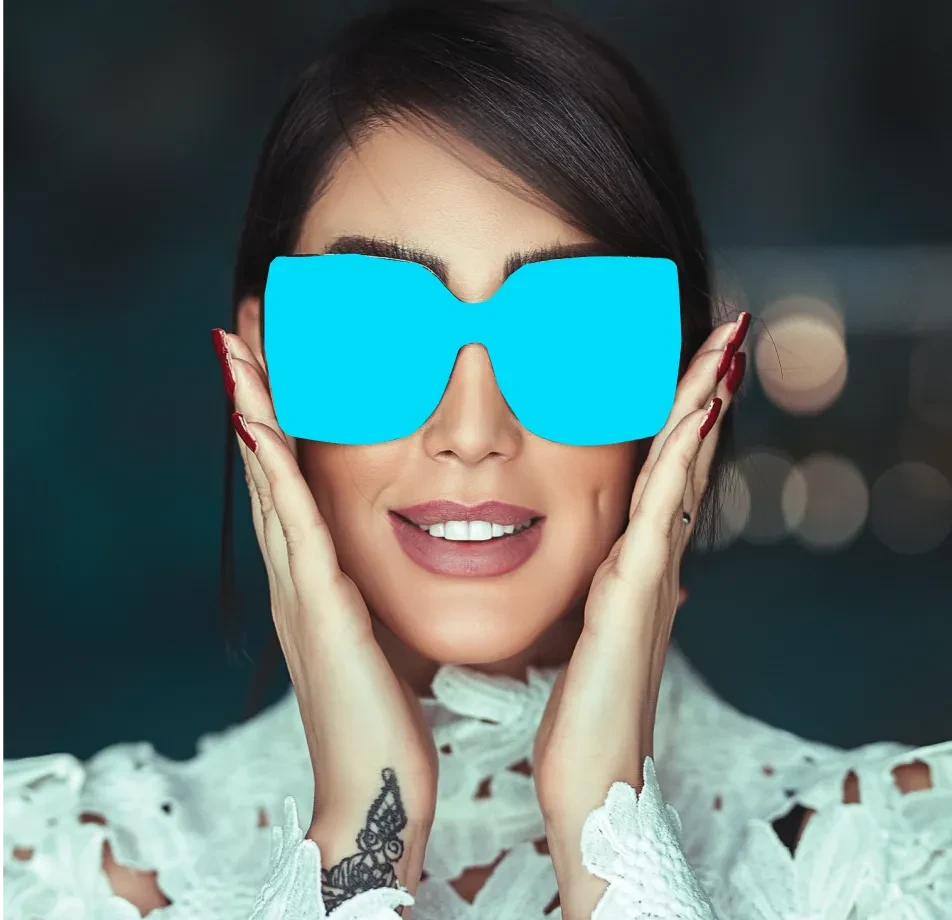
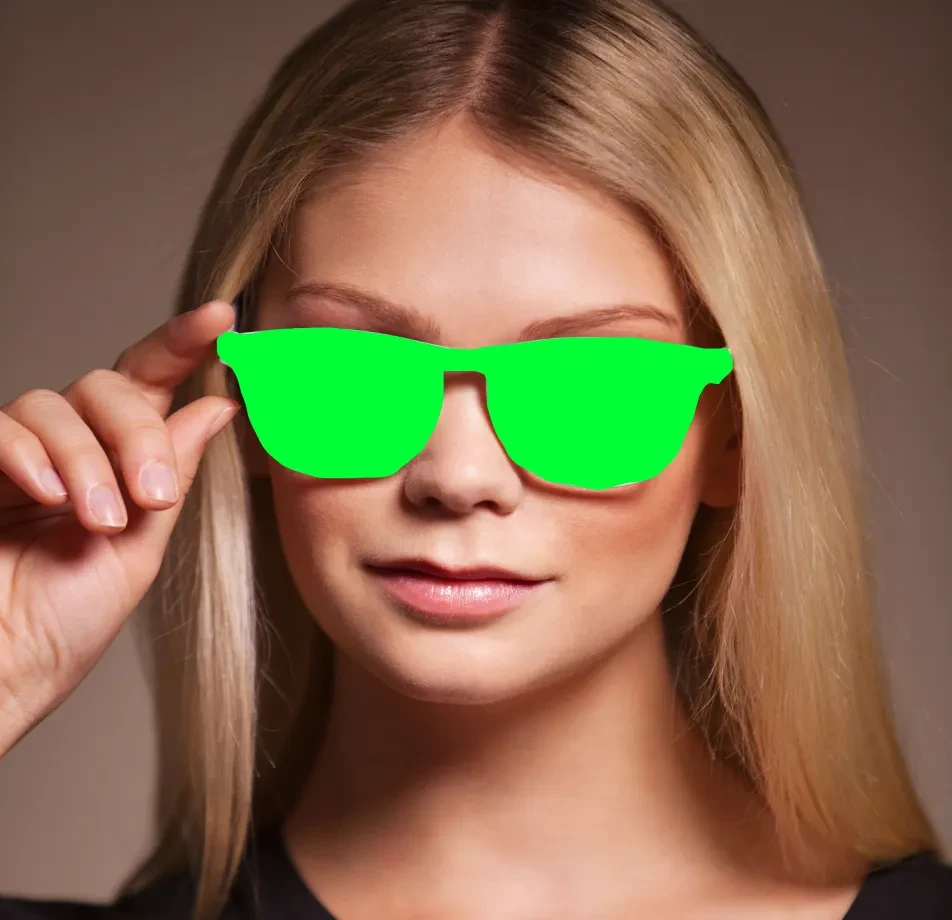
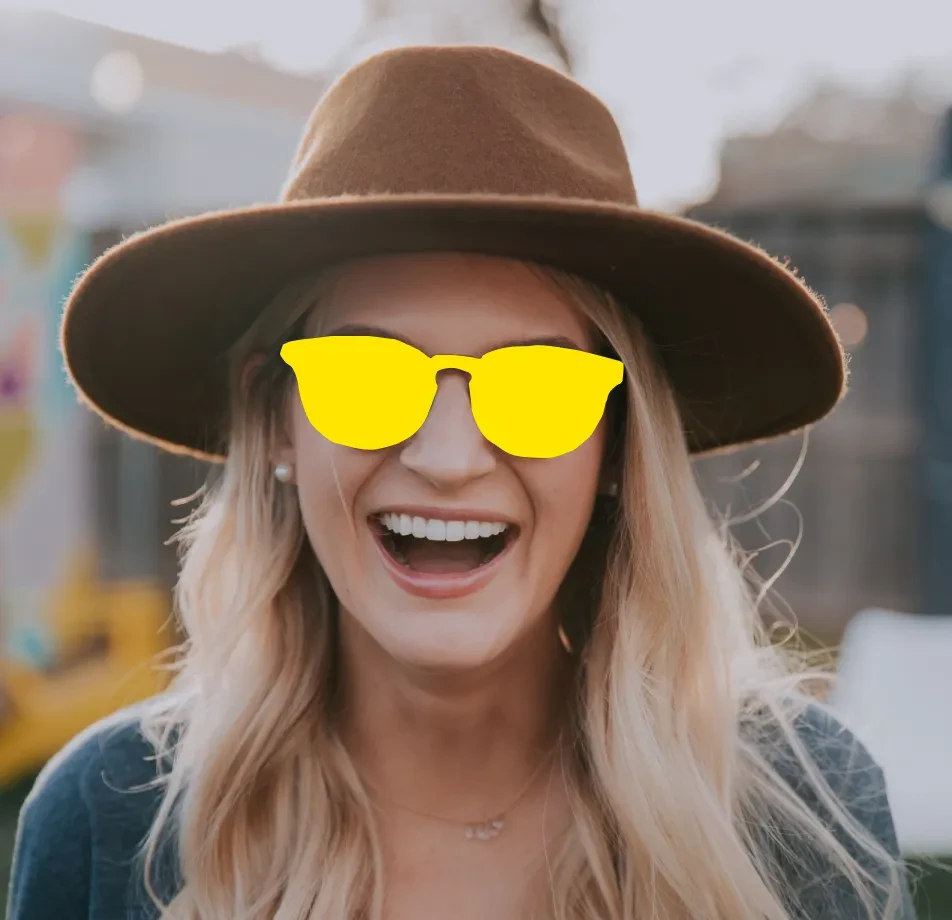
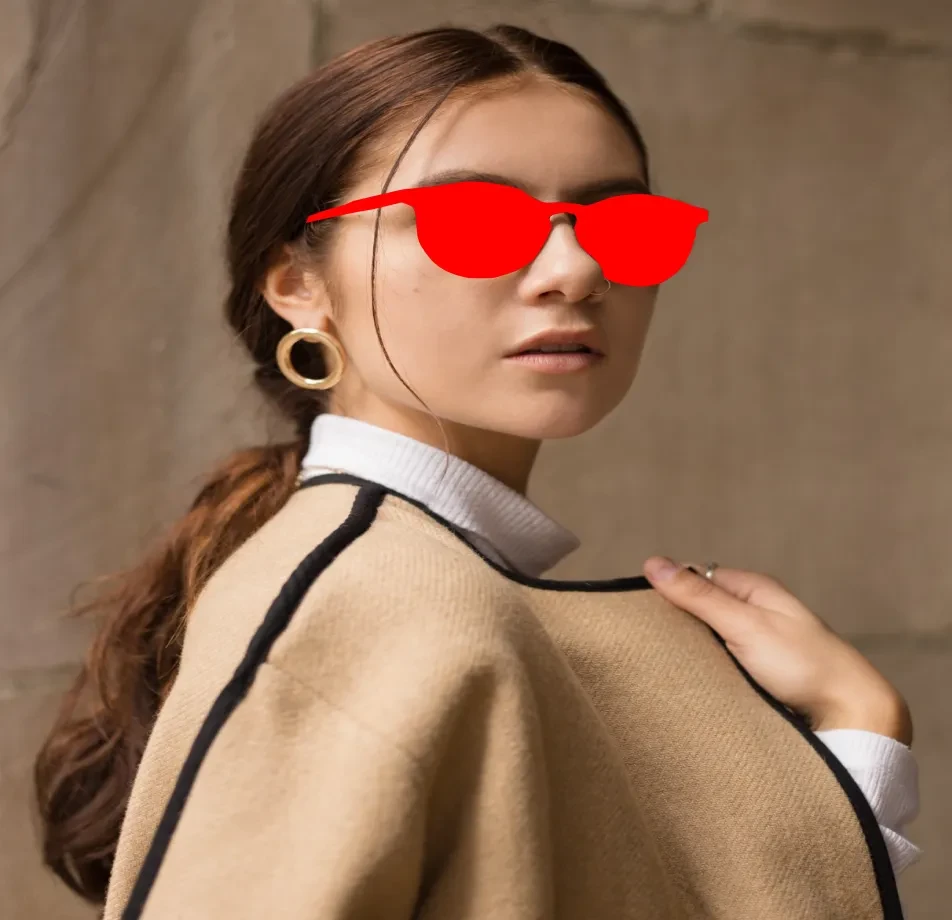
Quality Assurance
Stages
Expert Review: Opticians and eyewear designers carefully reviewed a subset of annotations for accuracy. Additionally,
Automated Consistency Checks: Software tools highlighted potential mismatches or missed sections.
Inter-annotator Agreement: Multiple annotators examined overlapping datasets to ensure a consistent approach.
QA Metrics
- Annotations Reviewed by Experts: 50,000 (20% of total annotations)
- Inconsistencies Identified and Rectified: 5,000 (2% of total annotations)
Conclusion
The Glasses Segmentation Dataset is a key resource for many applications, especially in the eyewear industry and facial recognition technology. By focusing on different types of eyeglasses and offering detailed segmentation, this dataset can drive new ideas in virtual try-on technologies and augmented reality apps.

Quality Data Creation

Guaranteed TAT

ISO 9001:2015, ISO/IEC 27001:2013 Certified

HIPAA Compliance

GDPR Compliance

Compliance and Security
Let's Discuss your Data collection Requirement With Us
To get a detailed estimation of requirements please reach us.