Gesture Recognition for Human-Computer Interaction
Home » Case Study » Gesture Recognition for Human-Computer Interaction
Project Overview:
Objective
Gesture Recognition for Human-Computer Interaction technology aims to enhance user experiences, improve accessibility, and broaden the range of applications across various fields, including gaming, healthcare, and automation, by providing a more seamless and efficient mode of interaction.
Scope
From gaming and entertainment to healthcare and robotics, this tech’s applications are vast. It even enhances accessibility and user experiences, truly transforming our interaction with the digital world. From gaming and movies to health services, industrial processes, and even robotics, this tech is making waves in a ton of areas. However, businesses must get strategic with their limited budgets to achieve ambitious goals and thin profit margins.
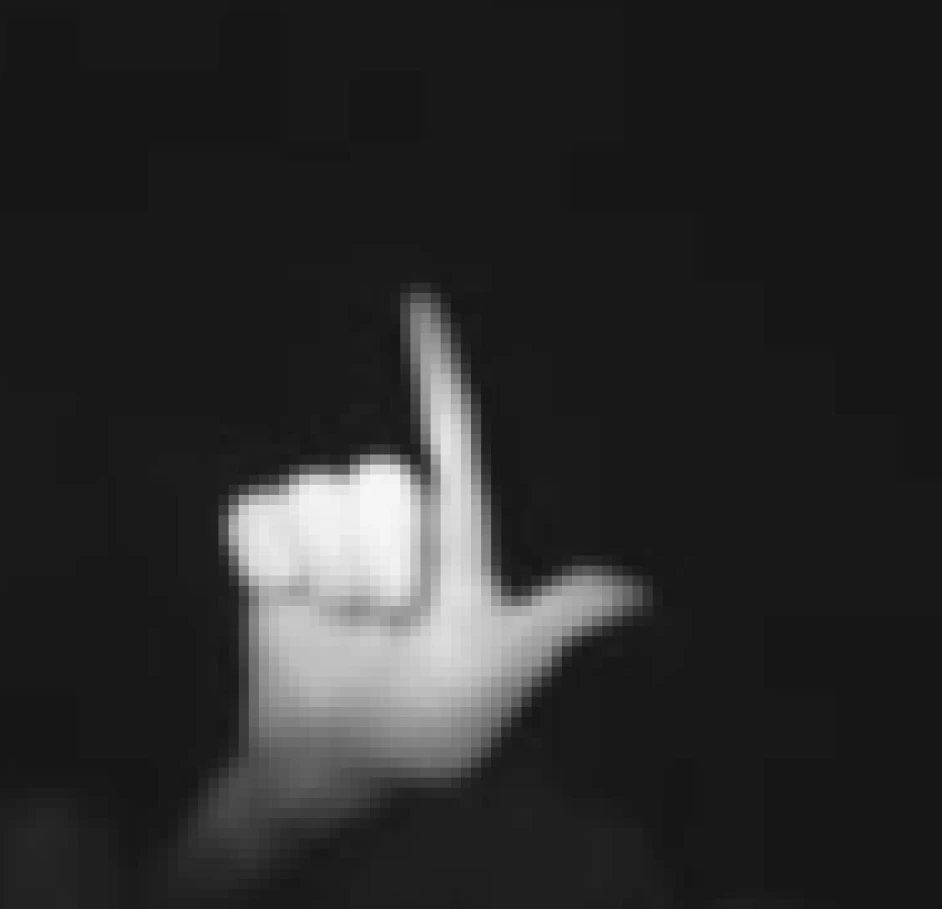
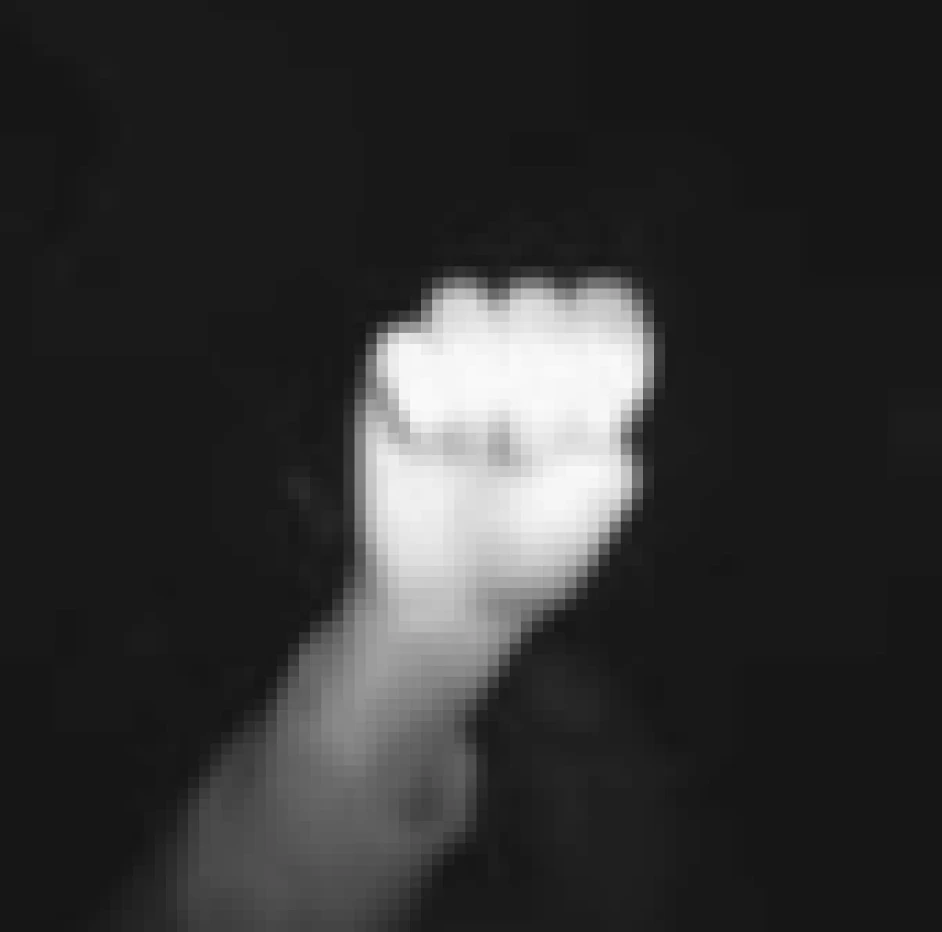
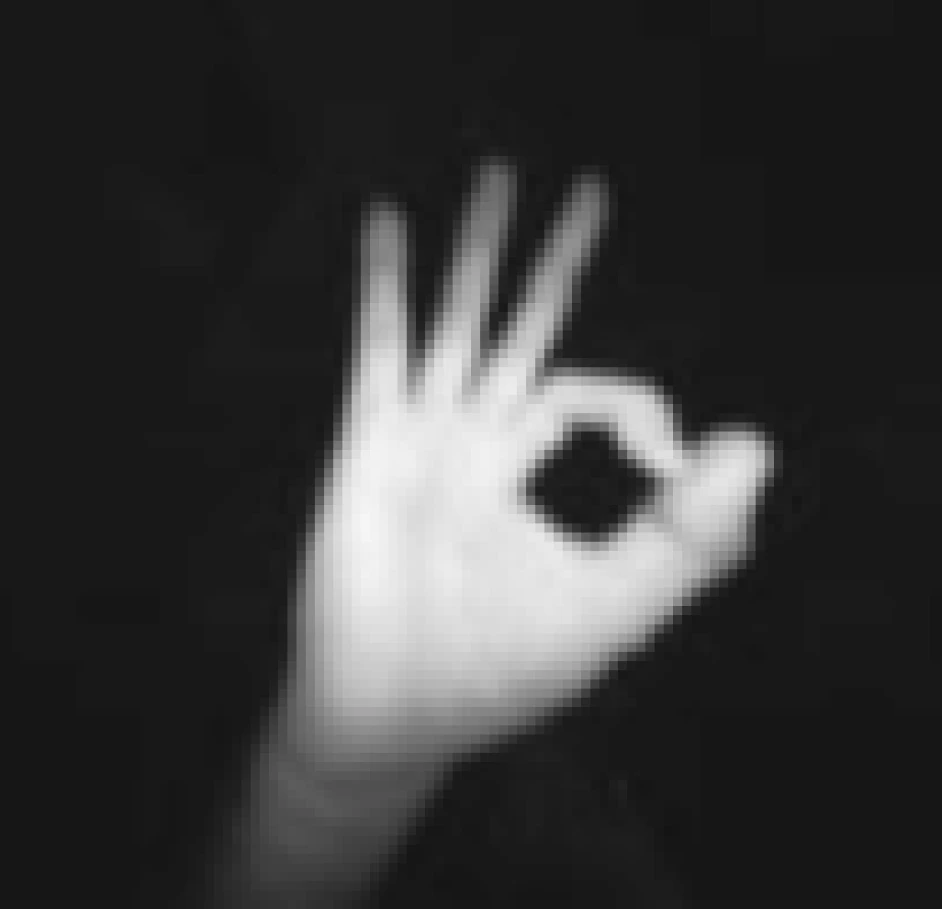
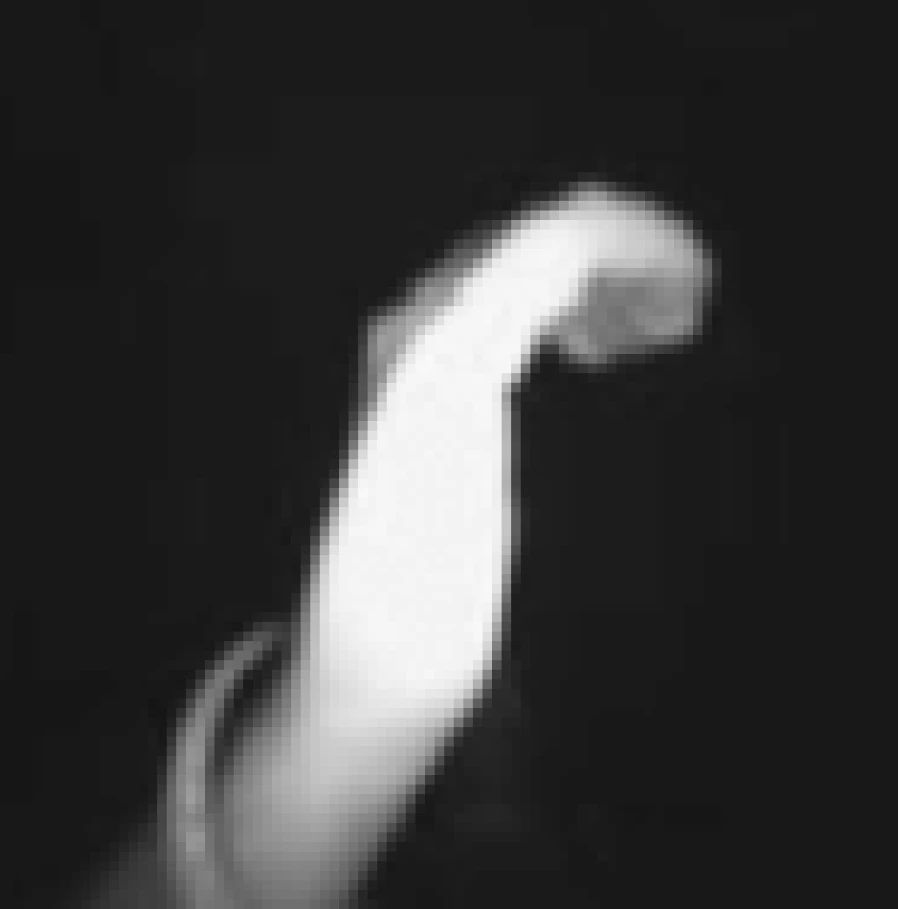
Sources
- Sensors and Cameras: Infrared sensors, depth-sensing cameras, and other hardware components capture and interpret user gestures.
- Machine Learning Models: Algorithms and models trained on gesture data to recognize and interpret a wide range of gestures.
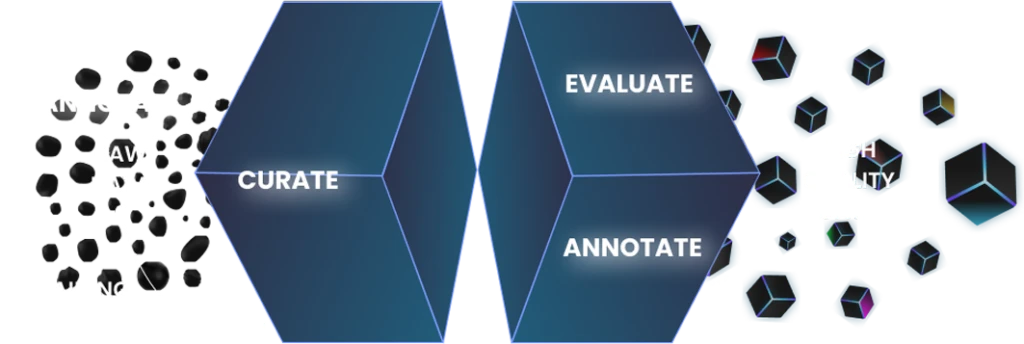
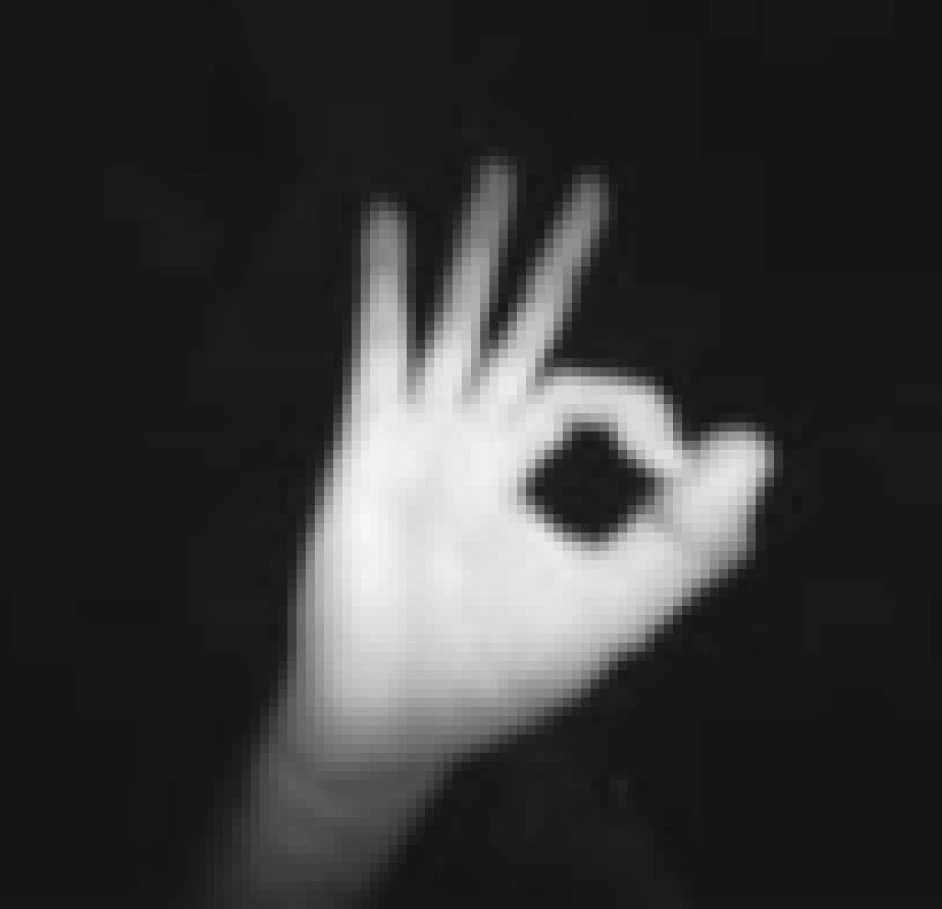
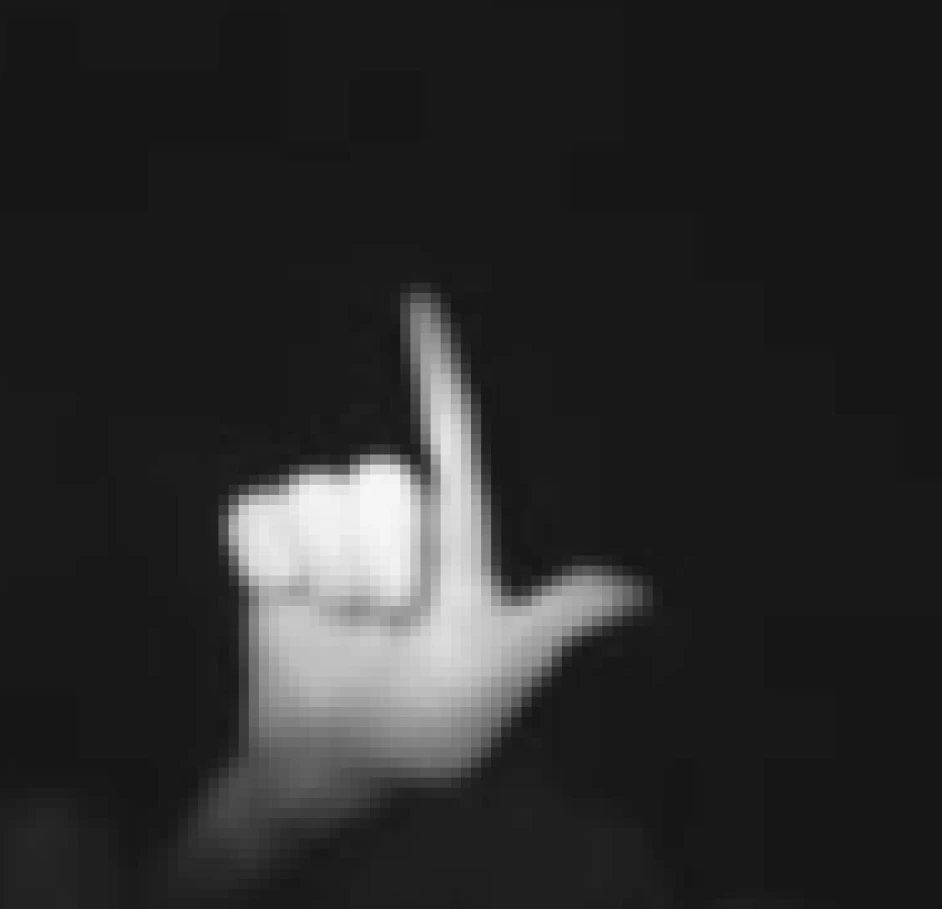
Data Collection Metrics
- Completeness: Measures data gathering efficiency.
- Accuracy: Assesses data correctness and reliability.
Annotation Process
Stages
- Planning: Defining research objectives and data collection methods.
- Data Gathering: Collecting data from various sources using appropriate techniques.
- Validation: Ensuring data accuracy by identifying and correcting errors.
- Reporting: Communicating findings through visualizations, reports, or presentations.
- Analysis: Processing data to extract meaningful insights and patterns.
Annotation Metrics
- Inter-Rater Agreement: Measures the level of consensus among annotators in assessing data, reflecting annotation reliability.
- F1 Score: Combines precision and recall to evaluate the overall accuracy of the annotation process, taking into account both false positives and false negatives.
- Cohen’s Kappa: Quantifies the agreement between annotators while considering chance agreement, an important metric for assessing the reliability and consistency of data annotation.
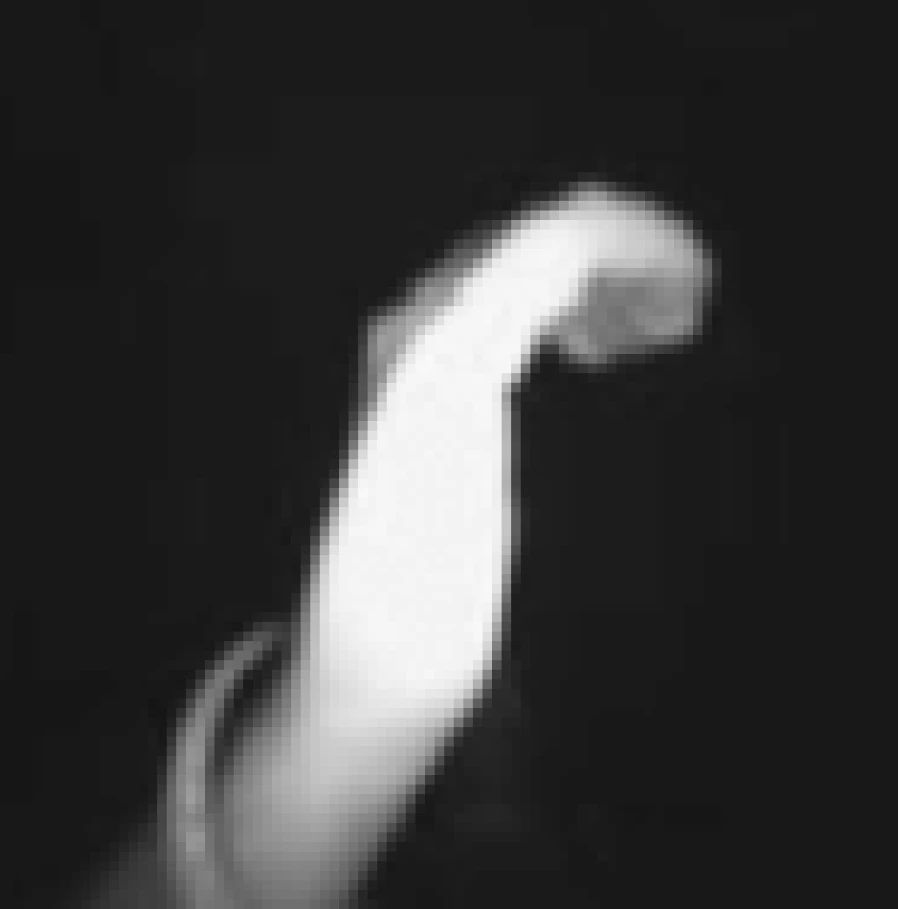
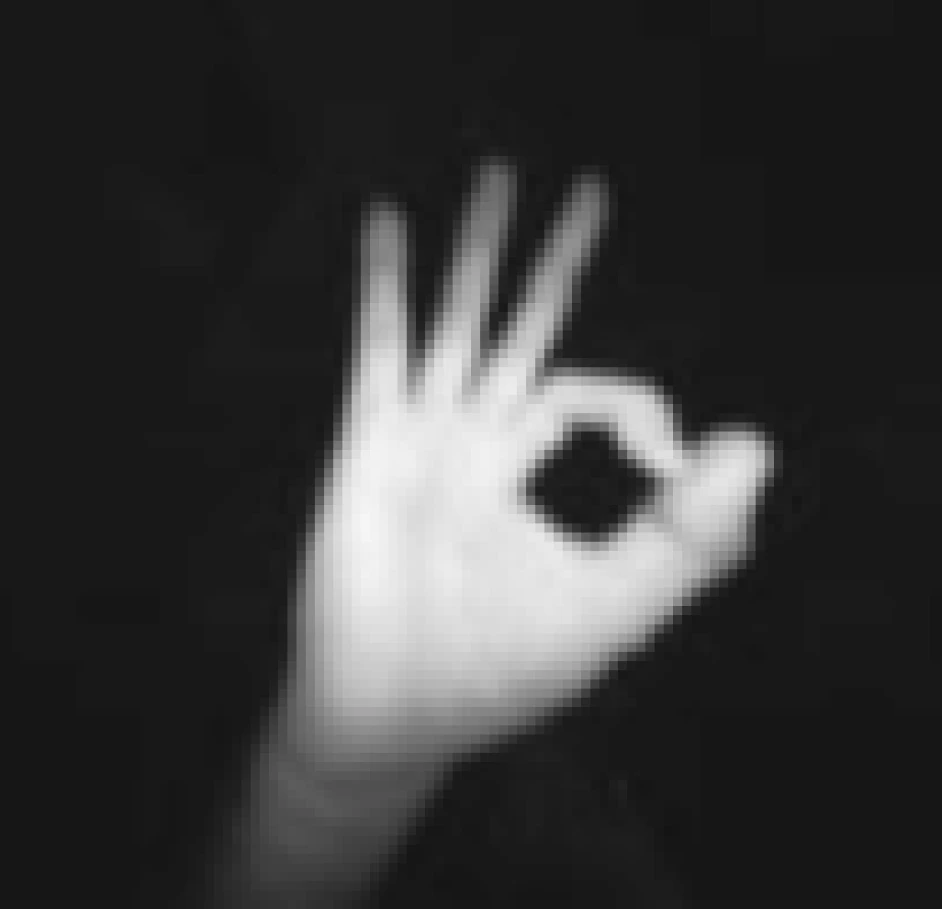
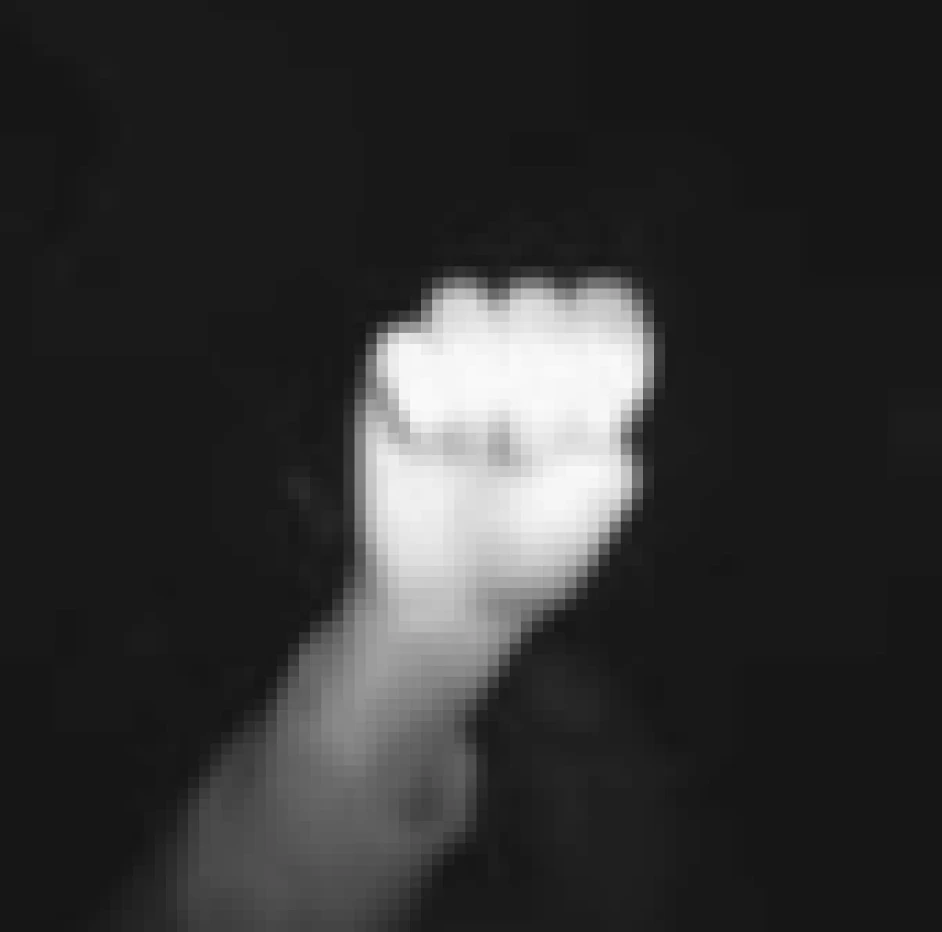
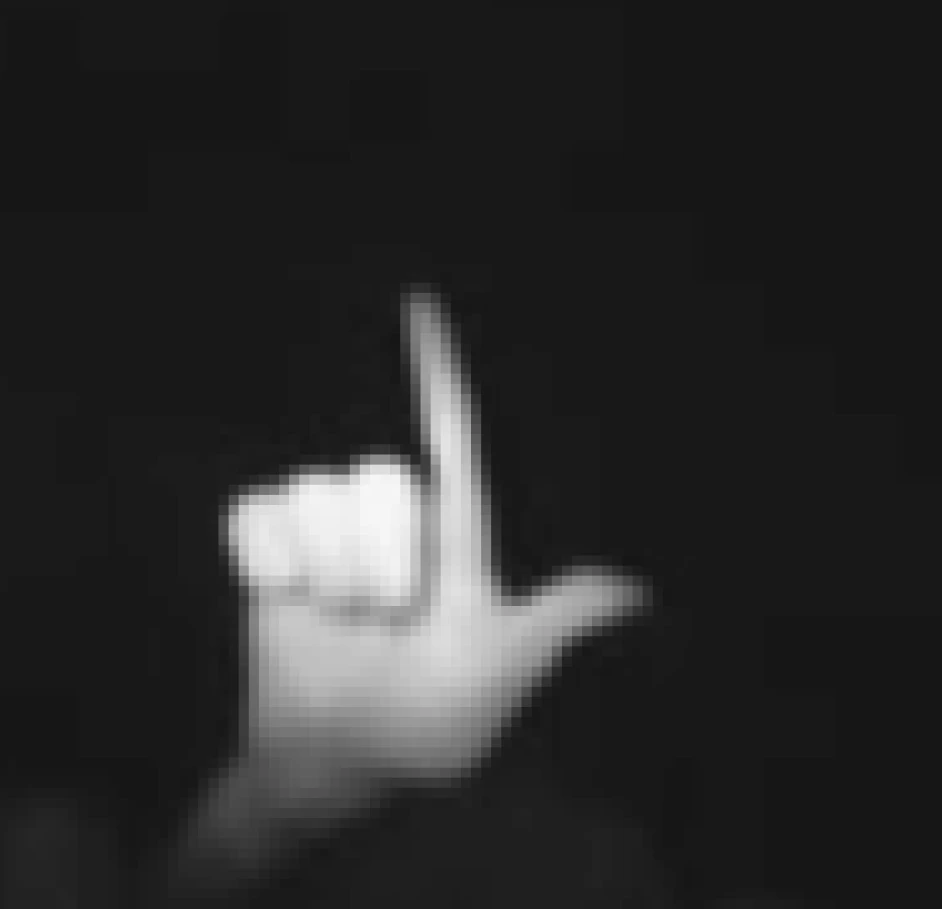
Quality Assurance
Stages
Data Quality:Â Involves accurate, complete, and reliable data essential for informed decision-making and effective data-driven processes.
Privacy Protection:Â Personal information from unauthorized access, ensuring confidentiality and compliance with privacy laws to maintain trust and data security.
Data Security:Â Protects data from unauthorized access through encryption and access controls, ensuring confidentiality and integrity, crucial for businesses and information safety.
QA Metrics
- Defect Rate: Measures the number of defects or errors in a product or process, reflecting overall quality.
- Privacy Compliance: Protect personal data, build trust, and avoid legal problems, especially in a data privacy-regulated environment.
Conclusion
while gesture recognition opens exciting doors for applications ranging from VR to healthcare, we’ve still got some hurdles like standardization and privacy issues that need tackling. By understanding and reacting to human gestures, it’s like we’ve got a golden ticket to a whole bunch of cool applications, spanning from gaming and VR all the way to healthcare and automating factories. But, we’re still just scratching the surface with gesture recognition – it’s got heaps more potential. To fully tap into this though, we need to sort out a couple of big issues like setting standard gestures and tackling privacy worries.

Quality Data Creation

Guaranteed TAT

ISO 9001:2015, ISO/IEC 27001:2013 Certified

HIPAA Compliance

GDPR Compliance

Compliance and Security
Let's Discuss your Data collection Requirement With Us
To get a detailed estimation of requirements please reach us.