Fashion Article Image Dataset
Home » Case Study » Fashion Article Image Dataset
Project Overview:
Objective
Scope
The dataset encompasses a wide range of fashion articles, including clothing, accessories, and footwear. Moreover, this variety extends to the way the items are captured, featuring them in diverse settings and poses. Consequently, this ensures robust model training and testing across various scenarios.
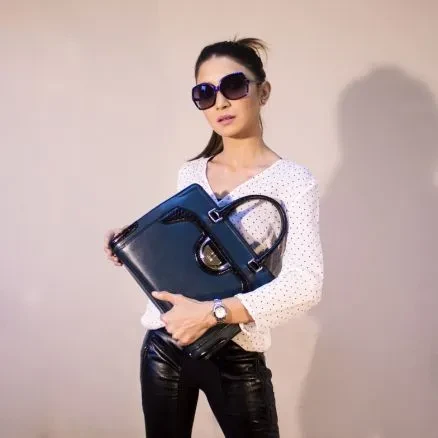
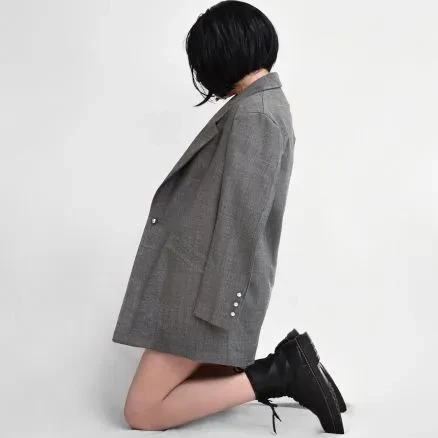
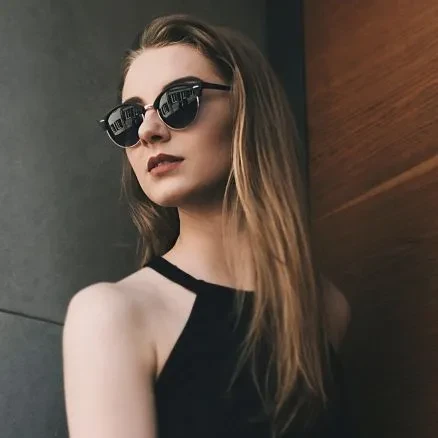
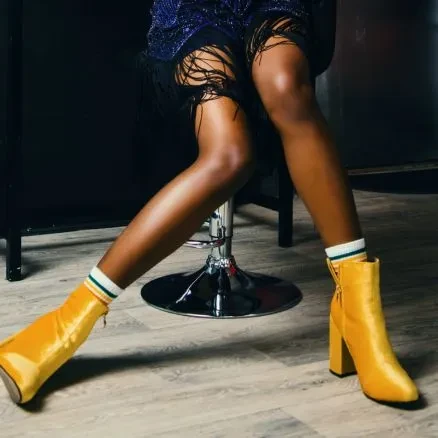
Sources
- The foundation of this dataset is the well-known Fashion-MNIST dataset, which provides a collection of grayscale images of fashion items across ten different categories.
- To further enhance the dataset’s capabilities, we incorporate augmented image data. This involves applying data augmentation techniques to generate additional images from the existing ones. This process effectively increases the dataset’s diversity and improves the model’s ability to generalize to unseen data, leading to better performance.
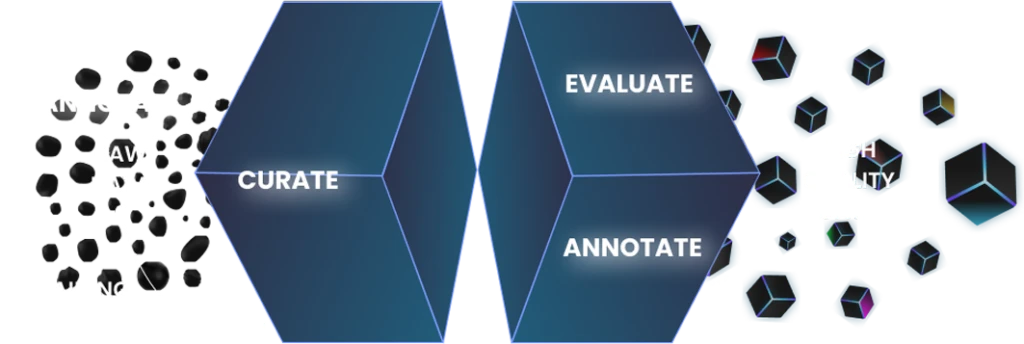
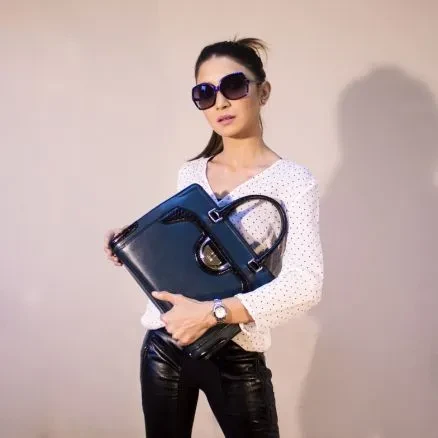
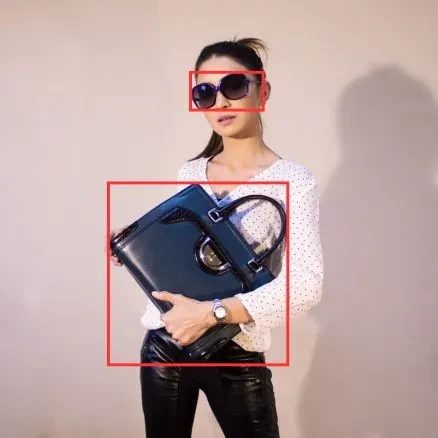
Data Collection Metrics
- Total Data Collected: 150,000 fashion images sourced from the Fashion-MNIST dataset and augmented data.
- Data Annotated for ML Training: 180,000 images with detailed labels and annotations to facilitate machine learning model training.
Annotation Process
Stages
- Item Categorization: Each image is labelled with its corresponding fashion category, including tops, bottoms, dresses, shoes, bags, and accessories. This provides a foundational understanding of the content within the image for the machine learning model.
- Additionally, Attribute Recognition: Beyond categories, attributes such as color, pattern, texture, and style are also annotated. This injects richer information into the data, allowing the model to learn more nuanced details about the fashion items.
- Moreover, Pose and Orientation: To further enhance the model’s robustness, annotations include information on the pose and orientation of fashion items within the image. This equips the model to handle variations in how clothing is presented, improving its ability to recognize items regardless of their position or angle.
Annotation Metrics
- Categorized Items: A total of 180,000 fashion items categorized across different classes for model training.
- Attribute Annotations: Detailed attribute annotations for 150,000 fashion images to aid in fine-grained recognition tasks.
- Pose Annotations: Pose and orientation annotations for 120,000 images to enhance model understanding of spatial relationships.
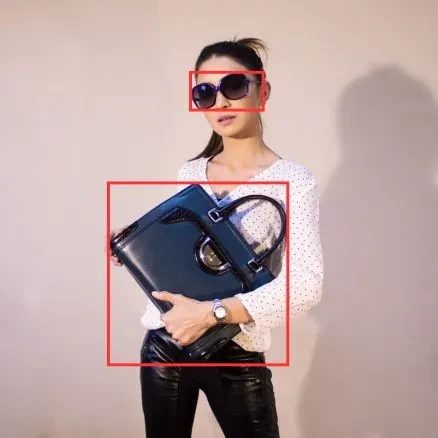
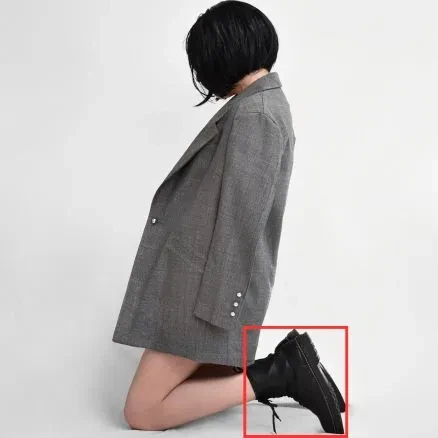
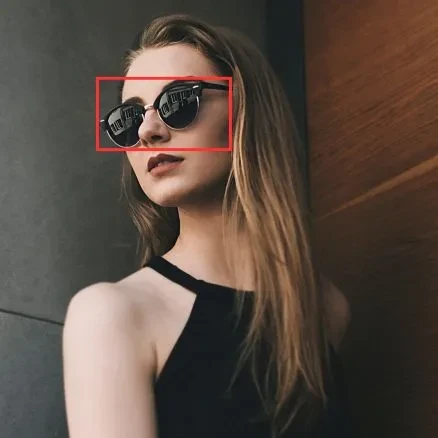
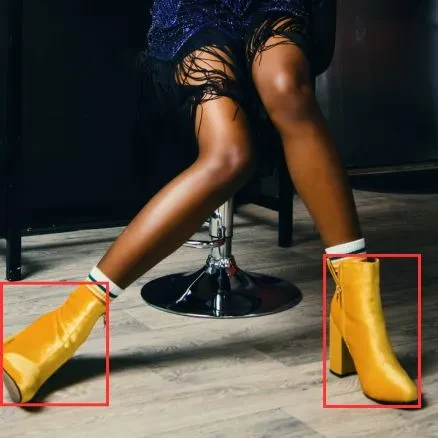
Quality Assurance
Stages
Model Validation: Rigorous testing is conducted to ensure dataset quality and compatibility with various machine-learning models and algorithms.
Additionally, Data Integrity Checks: This process is complemented by regular audits to verify the accuracy and consistency of annotations and labels across the dataset.
Furthermore, Feedback Integration: Finally, we incorporate feedback from model performance and user evaluations. This iterative approach allows us to continuously improve dataset quality and usability.
QA Metrics
- Classification Accuracy: Benchmark machine learning models achieve a classification accuracy of 98% on this dataset, indicating high-quality annotations and labels.
- Attribute Recognition Accuracy: Attribute recognition tasks further demonstrate the dataset’s effectiveness. Here, models achieve an accuracy of 95%, highlighting the value of detailed annotations in capturing fashion item attributes.
- Data Consistency: To ensure uniformity across the dataset, consistency checks were conducted. These checks revealed a high agreement rate of 99% among annotators.
Conclusion
The creation of the Fashion Article Image Dataset, built upon the foundation of the Fashion-MNIST dataset, marks a significant advancement in fashion-related machine learning research. Consequently, this dataset offers a diverse and well-annotated collection of fashion images. In turn, this rich resource facilitates the development of robust and accurate machine-learning models for various applications within the fashion domain.

Quality Data Creation

Guaranteed TAT

ISO 9001:2015, ISO/IEC 27001:2013 Certified

HIPAA Compliance

GDPR Compliance

Compliance and Security
Let's Discuss your Data collection Requirement With Us
To get a detailed estimation of requirements please reach us.