Emotion Detection in Video Content
Home » Case Study » Emotion Detection in Video Content
Project Overview:
Objective
Emotion detection in video content uses AI to analyze human emotions, offering value across multiple sectors. While its accuracy is increasing, it also brings forth ethical concerns about privacy and cultural sensitivity. Proper data diversity and transparency are essential for responsible use.
Scope
As technology advances, its applications could extend to virtual reality and gaming. Ethical considerations, including privacy and cultural inclusivity, remain crucial.
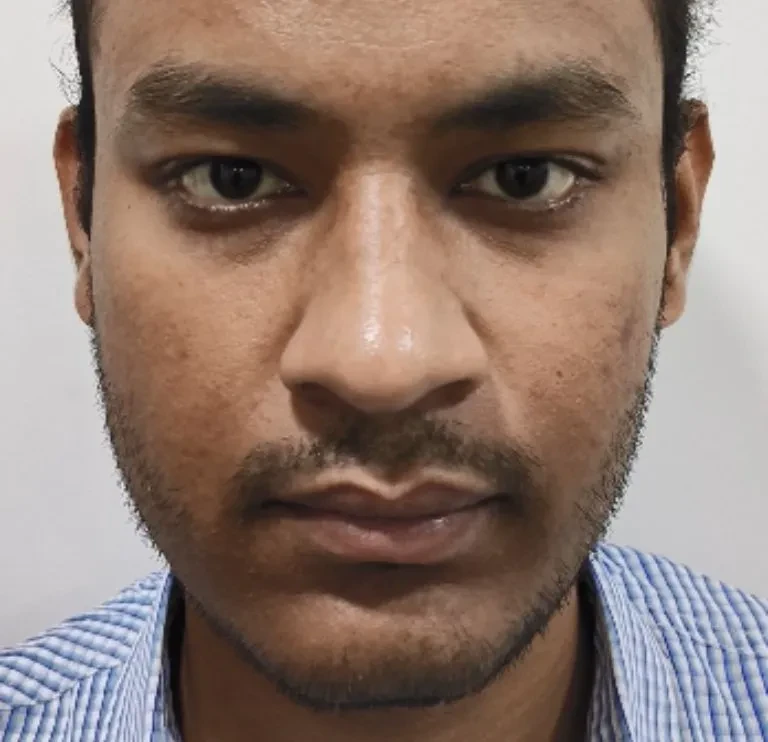
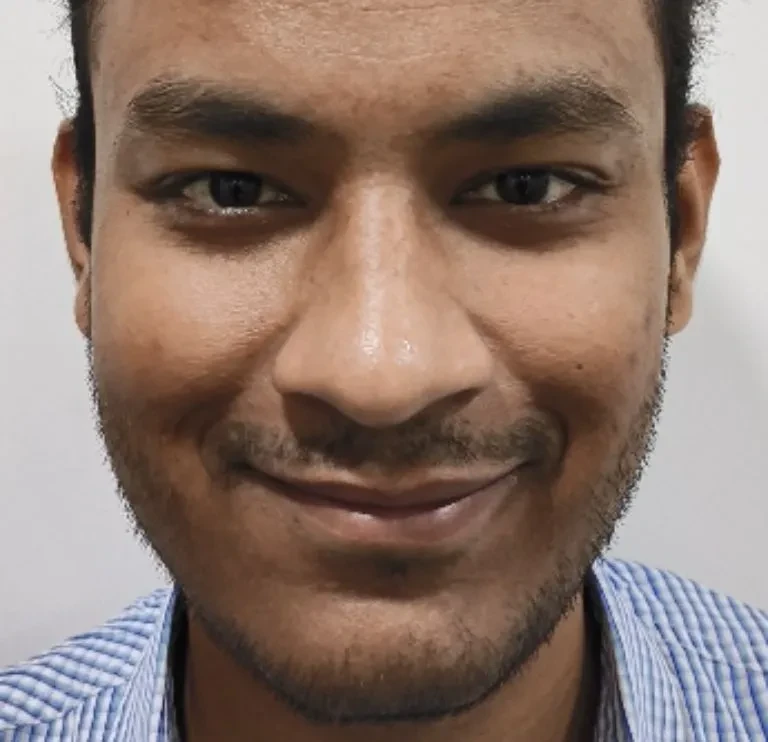
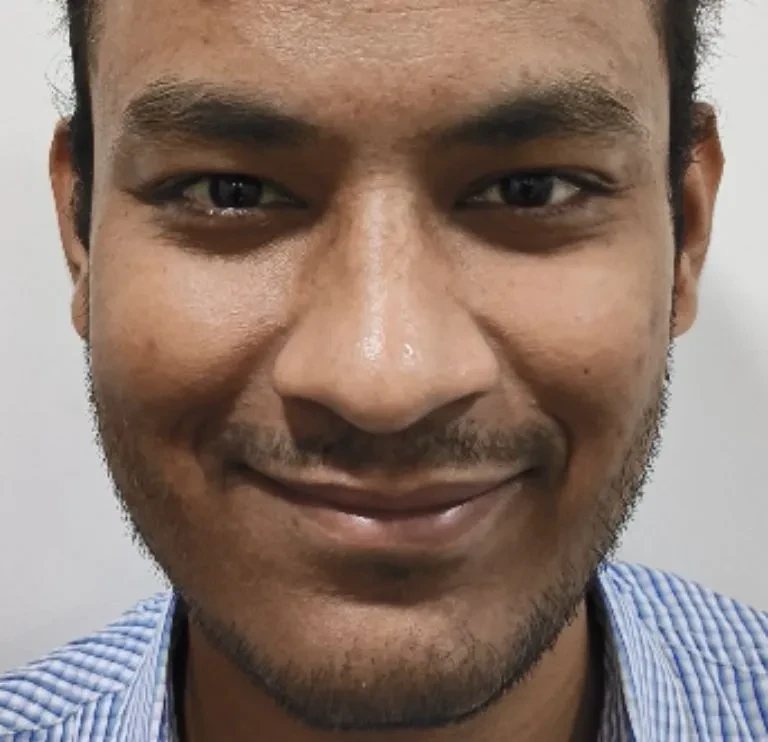
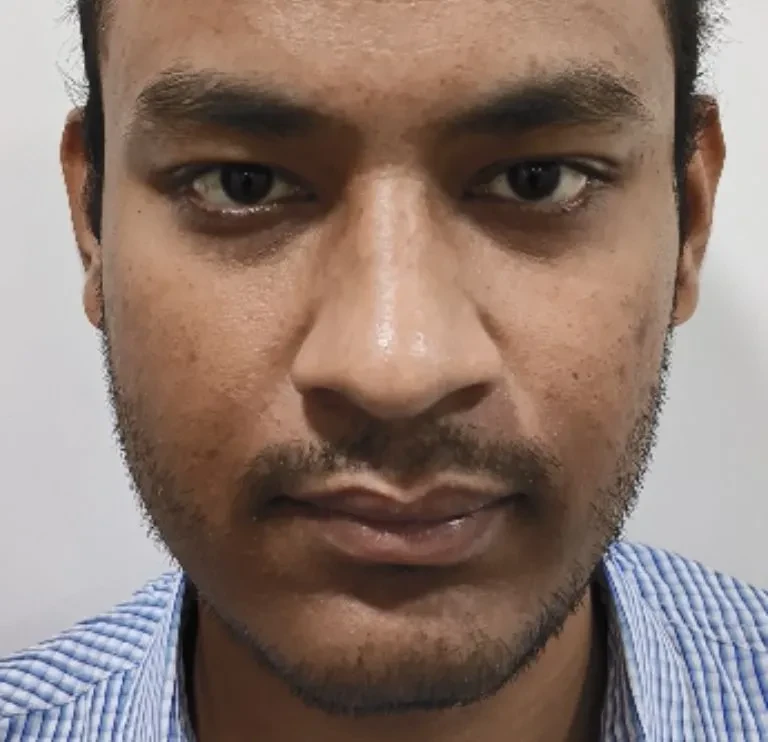
Sources
- Emotion detection leverages AI techniques to analyze visual and auditory cues from video content.
- Its applications span diverse sectors, but ethical concerns, particularly around privacy and inclusivity, need to be addressed.
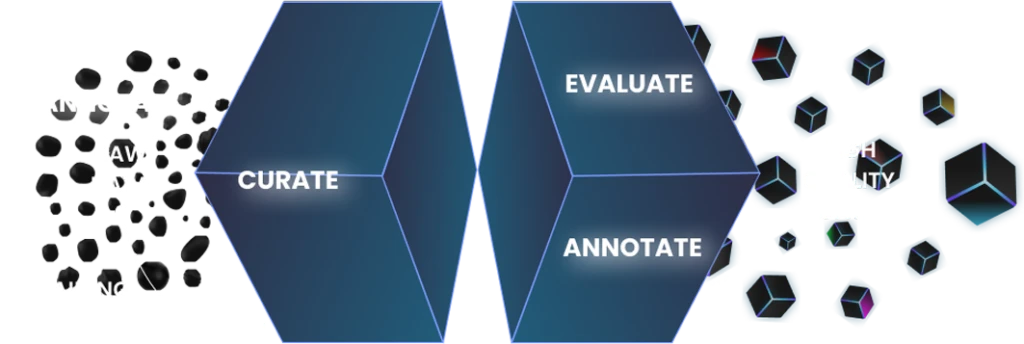
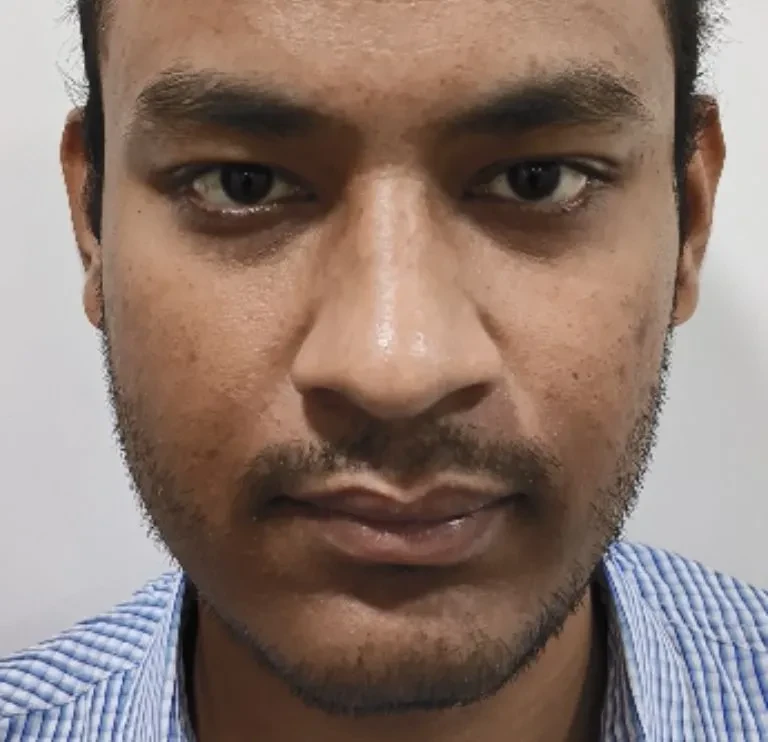
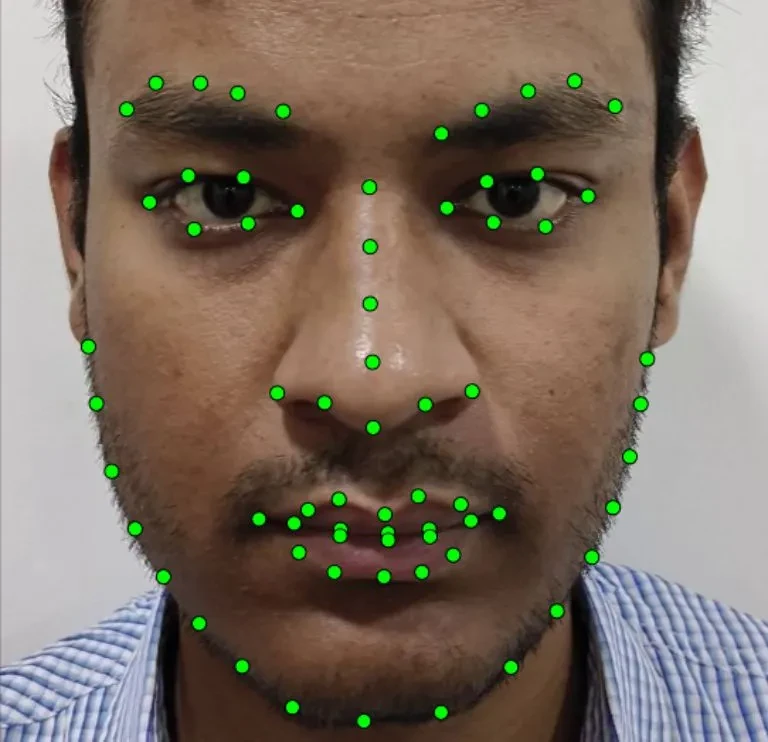
Data Collection Metrics
- Video Quantity: Total videos collected.
- Annotation Consistency: Ensuring uniform emotion annotations for reliable training data.
Annotation Process
Stages
- Data Collection: Gather video content and relevant metadata.
- Preprocessing: Clean, format, and organize the data.
- Annotation: Manually or automatically label emotions in the video content.
- Feature Extraction: Extract relevant features from video frames or audio.
- Model Training: Train machine learning models on annotated data for emotion detection.
Annotation Metrics
- Accuracy Rate: Measures correctness compared to a reference or gold standard.
- Inter-annotator Agreement: Evaluates consistency among different annotators.
- Annotation Speed: Tracks the time taken for each annotation task.
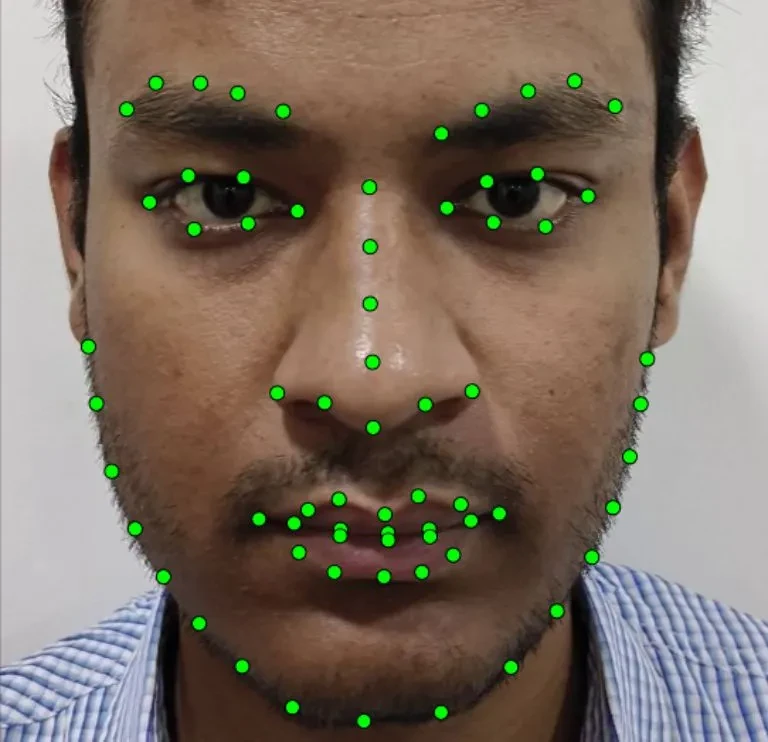
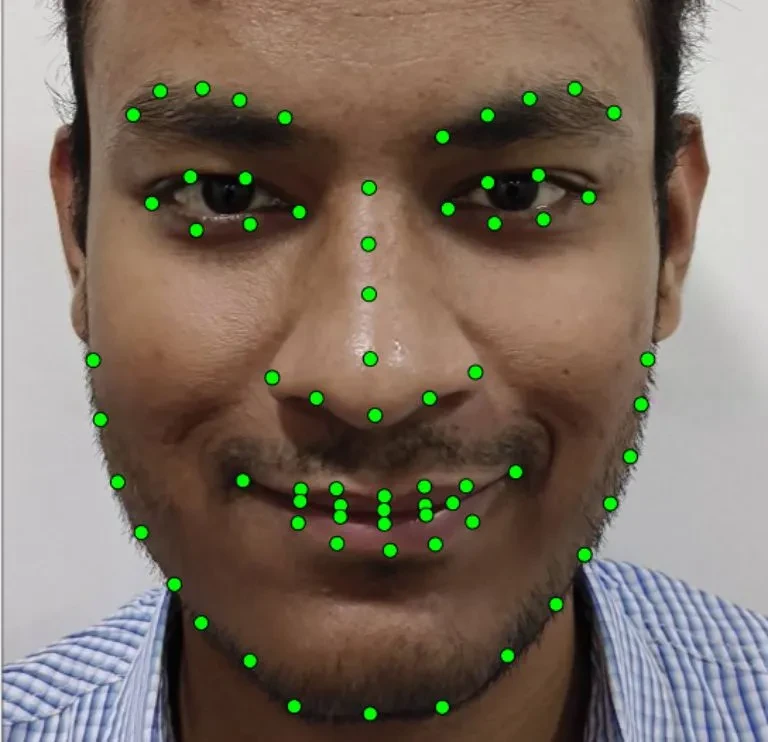
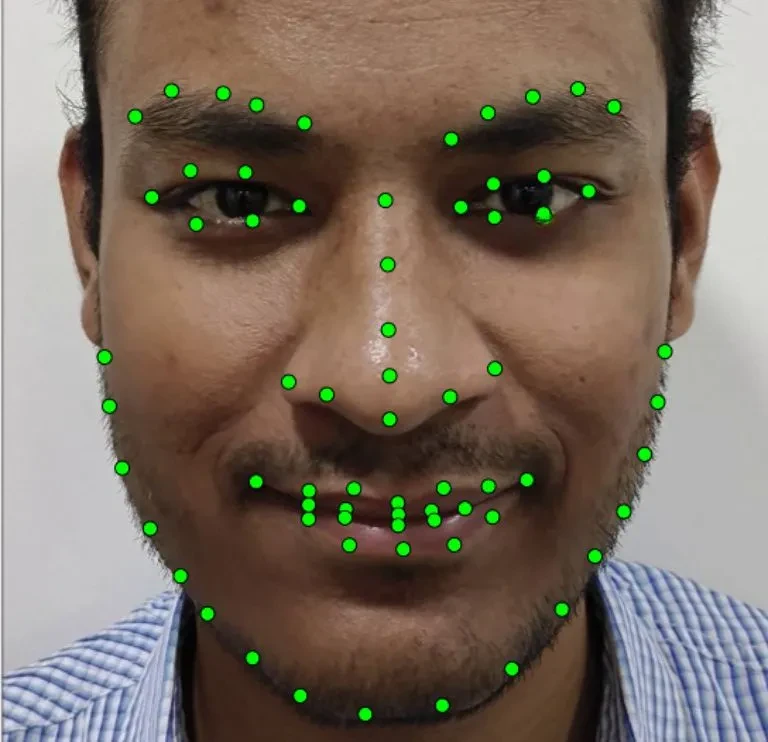
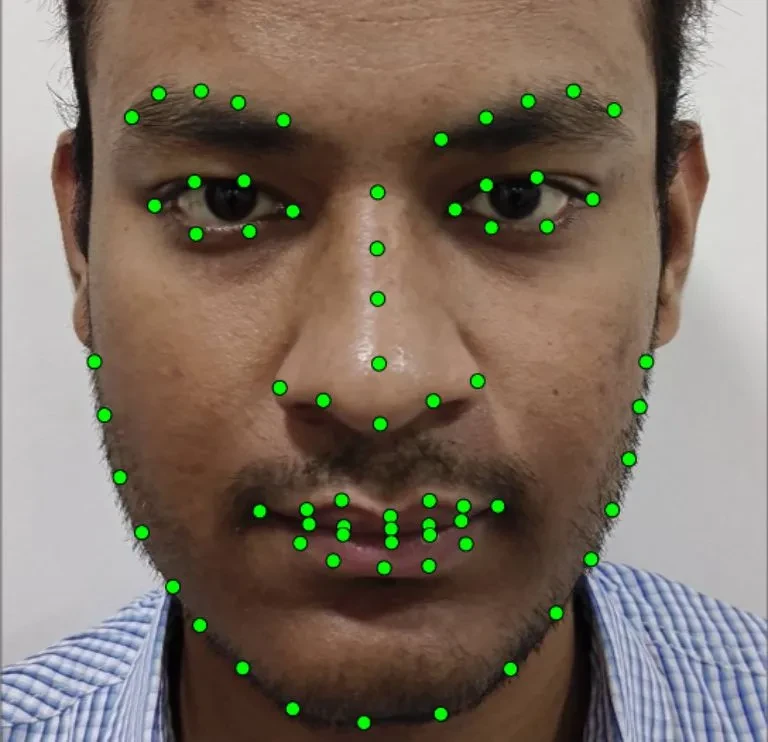
Quality Assurance
Stages
Policy Compliance: Ensuring that privacy protection measures align with relevant laws and regulations, such as GDPR or HIPAA, to avoid legal issues.
Regular Audits: Conducting periodic audits and assessments of data handling practices to identify and rectify privacy vulnerabilities.
User Education: Educating employees and users about privacy policies and best practices to minimize data breaches and privacy violations.
QA Metrics
- Defect Density: Measures the number of defects per unit size, indicating software or product quality.
- Test Pass Rate: Evaluates the percentage of tests successfully passed, indicating the reliability and effectiveness of testing processes.
Conclusion
Emotion detection in video content has emerged as a pivotal area of study and application, leveraging advanced machine learning and artificial intelligence techniques to decipher human emotions from visual and audio cues. This technology has profound implications across various domains, from entertainment and advertising to healthcare and education.

Quality Data Creation

Guaranteed TAT

ISO 9001:2015, ISO/IEC 27001:2013 Certified

HIPAA Compliance

GDPR Compliance

Compliance and Security
Let's Discuss your Data collection Requirement With Us
To get a detailed estimation of requirements please reach us.