Driving Recorder Image
Home » Case Study » Driving recorder image
Project Overview:
Objective
The main goal of this project is to collect and label images from driving recorders. These images are important for developing advanced driving assistance systems (ADAS) and self-driving technologies. By labeling these images, we aim to boost the accuracy of object detection, lane recognition, and traffic sign interpretation. Consequently, this will enhance road safety and driving efficiency.
Scope
This project aims to gather a wide range of driving recorder images from various conditions, including urban and rural areas, as well as different weather scenarios. Moreover, these images will be annotated to identify several elements such as vehicles, pedestrians, road signs, and lane markings.
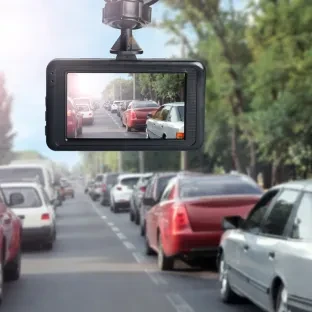
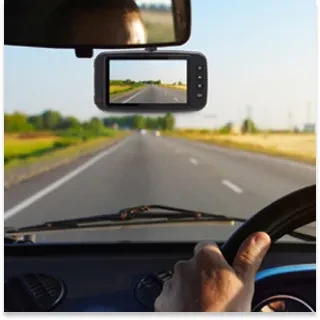
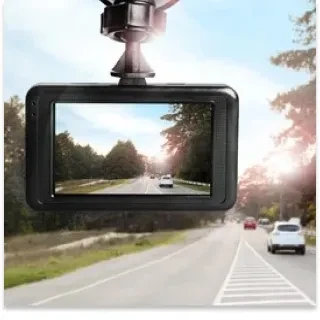
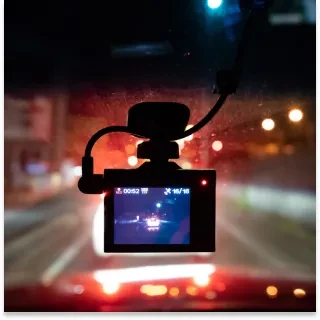
Sources
- Automotive Partnerships: We collaborated with numerous automotive companies, acquiring 12,000 high-quality images from their driving recorders.
- Real-World Drives: Our team conducted extensive real-world driving sessions, adding 5,000 unique images depicting a variety of road situations and obstacles.
- Public Resources: We further enriched our collection with 3,000 carefully selected and annotated images from public databases to ensure a well-rounded dataset.
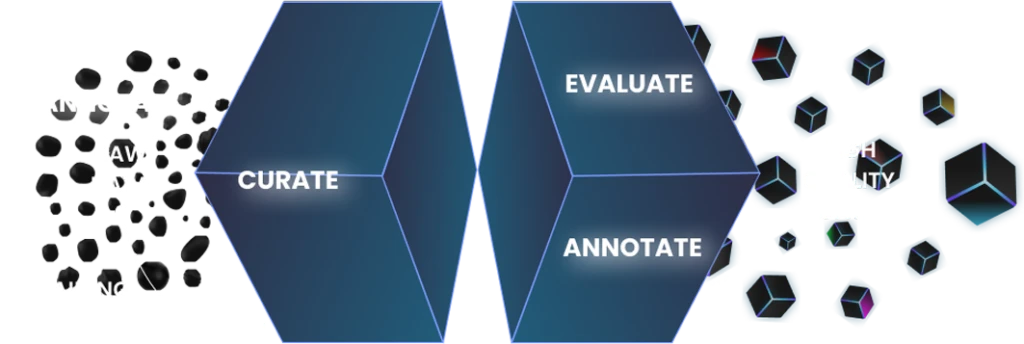
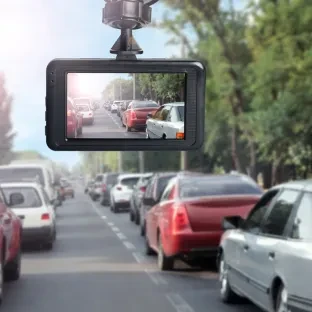
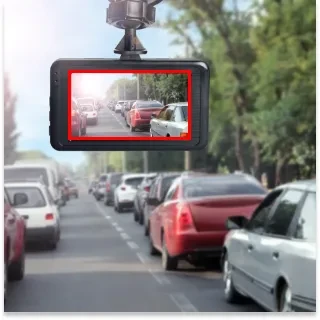
Data Collection Metrics
- Total Images: 20,000
- From Automotive Partnerships: 12,000
- Real-World Driving Sessions: 5,000
- Public Databases: 3,000
Annotation Process
Stages
- Event Categorization: Each image was meticulously tagged with relevant events such as traffic light recognition, pedestrian detection, and road sign identification.
Environmental Context: We included detailed information for each image, such as weather conditions, time of day, and GPS coordinates.
Obstacle identification: primarily focused on marking various obstacles, including static ones like potholes and dynamic ones like pedestrians.
Annotation Metrics
- Images with Event Annotations: 20,000
- Contextual Data Enriched: 20,000
- Obstacle Tags Added: 20,000
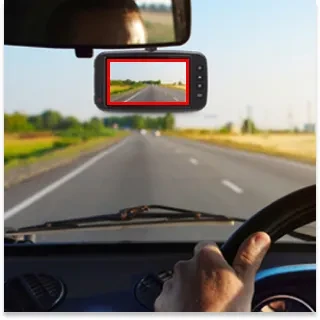
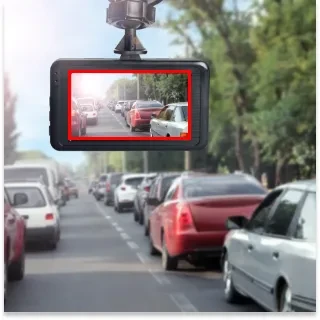
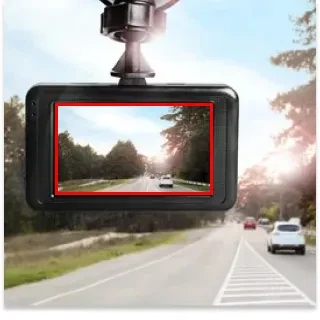
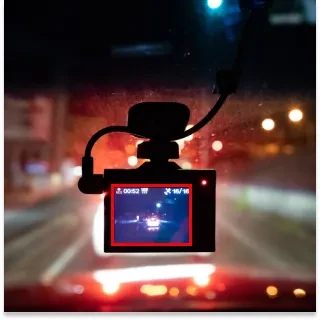
Quality Assurance
Stages
- Expert Review: Traffic and vehicle experts often review a subset of annotated images to ensure accuracy and reliability.
- Continuous Improvement: We regularly update and enhance our annotation techniques. These updates take into account the ever-changing road and driving conditions.
- Feedback Loop: We work closely with developers of autonomous driving systems to gather their feedback. Consequently, we make ongoing improvements based on their insights.
QA Metrics
Expert Review Cases: To maintain high-quality annotations, 15% of the annotated images are reviewed by experts. This helps in identifying and correcting any inconsistencies or errors.
Accuracy Improvement Rate: We track and aim to improve the accuracy of annotations on a quarterly basis. Regular reviews and feedback loops ensure continuous enhancement of our annotation quality.
Conclusion
The “Driving Recorder Image Collection and Annotation” project is essential for improving ADAS and autonomous driving technologies. By collecting and labeling driving images, it gives important data for machine learning models. As a result, these models can better understand and navigate real-world driving situations. In addition, this project represents a big step toward safer and more efficient driving. Moreover, this initiative ensures the data is broad and varied, covering different driving conditions and scenarios. Thus, the project contributes to making driving experiences safer and more reliable.

Quality Data Creation

Guaranteed TAT

ISO 9001:2015, ISO/IEC 27001:2013 Certified

HIPAA Compliance

GDPR Compliance

Compliance and Security
Let's Discuss your Data collection Requirement With Us
To get a detailed estimation of requirements please reach us.